ABSTRACT
Does language influence how we perceive the world? This study examines how linguistic encoding of relational information by means of verbs implicitly affects visual processing, by measuring perceptual judgements behaviourally, and visual perception and attention in EEG. Verbal systems can vary cross-linguistically: Dutch uses posture verbs to describe inanimate object configurations (the bottle stands/lies on the table). In English, however, such use of posture verbs is rare (the bottle is on the table). Using this test case, we ask (1) whether previously attested language-perception interactions extend to more complex domains, and (2) whether differences in linguistic usage probabilities affect perception. We report three nonverbal experiments in which Dutch and English participants performed a picture-matching task. Prime and target pictures contained object configurations (e.g., a bottle on a table); in the critical condition, prime and target showed a mismatch in object position (standing/lying). In both language groups, we found similar responses, suggesting that probabilistic differences in linguistic encoding of relational information do not affect perception.
Introduction
How do we perceive the world? Visual science research has proven that perception is not a passive, purely bottom-up driven process; it involves interpretation and instant categorization based on prior knowledge and experience (Abdel-Rahman & Sommer, Citation2008; Gilbert & Li, Citation2013; Summerfield & de Lange, Citation2014). Over the course of our experiences with the world, we learn to associate certain cues with certain types of sensory input, and hence learn to categorize the visual world automatically. Importantly, human beings use language to talk about the things they perceive, and linguistic cues are acquired from very early in life. Language thus provides us with a rich source of experience in associating referential cues with visual information. In the same way that we are experienced in linking the sound of a dog’s bark to the visual encounter of a four-legged furry creature, we are entrained to immediately relate the word “dog” to the image of a dog. Recent research specifically targeting effects of language on perception has shown that linguistic labels in fact provide better cues to perception than nonverbal cues, such as sounds (e.g., Lupyan & Thompson-Schill, Citation2012). It is argued that the function of words is to generalize across individual exemplars and activate a categorical representation, and with that, general – rather than exemplar-based – visual properties of a concept (Boutonnet & Lupyan, Citation2015; Edmiston & Lupyan, Citation2015; Lupyan & Bergen, Citation2015).
Recent studies have used cross-linguistic differences in terminology as test cases for language-on-perception effects. In these approaches, visual stimuli are manipulated along a specific dimension that varies across languages (e.g., a distinction that is lexicalized in one language, but not in another). Participants perform a purely perceptual task, without any linguistic cues or instructions requiring overt use of language, allowing for an investigation of implicit language effects on perception. For instance, a study by Winawer and colleagues (Citation2007) investigated effects of colour terminology on colour perception using a speeded colour discrimination task. They found that native speakers of Russian, who obligatorily distinguish between light blue (goluboy) and dark blue (siniy), were faster to discriminate two colours when they belonged to different linguistic categories (one light blue and one dark blue) than when they belonged to the same linguistic category (both light blue or both dark blue); for native English participants (who do not lexically distinguish between these colour categories) no such effect was found. A study by Thierry, Athanasopoulos, Wiggett, Dering, and Kuipers (Citation2009) investigated effects of colour terminology on earlier visual processing stages using EEG methodology. Greek participants (who lexically distinguish between ghalazio “light blue” and ble “dark blue”), and English participants (who do not) performed a shape detection task (“press a button if you see a square”). Both shape (task-relevant) and colour (task-irrelevant) properties of the visual stimuli were manipulated following an oddball paradigm: participants were presented with a series of frequently occurring stimuli (Standards, e.g., light blue circles) alternated with infrequent stimuli (Deviants) that differed from the Standard in shape (square; Response condition) and/or luminance (dark blue). They found that blue colour deviants elicited a larger visual N100 component (negative wave form occurring 100–200 ms after stimulus onset)Footnote1 in Greek participants than in English participants, whereas no difference between groups was found for green colour deviants (control condition). These results suggest a stronger perceptual discrimination of a linguistically encoded, but task-irrelevant visual contrast. In a similar vein, Boutonnet, Dering, Viñas-Guasch, and Thierry (Citation2013) studied effects of language on object perception. Whereas English distinguishes between cups and mugs, Spanish has one label for both items (taza). Participants were asked to detect an infrequent target object (a bowl) among a series of standards. The standard was either a cup (alternated with bowl and mug deviants), or a mug (alternated with bowl and cup deviants). Again, it was found that perceptual discrimination between standards and non-target deviants was enhanced in participants who linguistically distinguish between cups and mugs, i.e., enhanced N100 amplitude for task-irrelevant object deviants in English, but not Spanish participants.Footnote2
The above studies show that frequent use of linguistic labels reinforces the perceptual distinction between referents that are labelled distinctly (e.g., the distinction between ble/siniy and ghalazio/goluboy, the distinction between cups and mugs): it leads to the formation of two distinct categories, based on specific perceptual dimensions that speakers of the respective language are highly trained on. Findings show that this enhanced saliency is detectable in these populations even when they are not using the labels online; linguistic category effects were found during post-perceptual processing stages (behavioural responses in perceptual judgement tasks), as well as during early perceptual discrimination (N100 effects). The current study aims to examine the generalizability of previously reported language-on-perception effects in two ways. First, we investigate whether language effects on perception extend from labelling conventions that involve a clear one-to-one mapping between a label and a percept (e.g., colour terms, object labels) to more complex linguistic categories, i.e., categories expressing relational information. Second, we examine language-on-perception effects in cases where cross-linguistic differences are probabilistic rather than categorical. We will use a variety of measurements, tapping into different levels of cognitive processing (perceptual judgements, attention, perception), with the aim to provide a complete picture on the role of language in vision for this specific test case (see discussion in Firestone & Scholl, Citation2016). The outcome of this study will thus substantially advance our understanding of the nature of language-perception interactions.
Regarding the first question, to date the bulk of research has focused on linguistic categories at the word level, such as colours and objects. Only few studies have looked at how more complex linguistic categories may influence perceptual processing. Prior studies have demonstrated that verbs expressing relational information can facilitate category learning (Gentner & Namy, Citation2006) and that, in a pre-cueing paradigm, verbs can influence the detection of visual events (e.g., motion verbs influencing detection of up-/downward motion in random dot motion stimuli, Francken, Kok, Hagoort, & de Lange, Citation2015; Meteyard, Bahrami, & Vigliocco, Citation2007). However, studies of implicit effects of verbs on perceptual categorization and discrimination are lacking.
We specifically focus on posture verbs (e.g., “to stand” and “to lie”) denoting object configurations, that is the relation between a figure and a ground. These verbal labels can apply to any object in different instances; therefore, they evoke less clear perceptual representations. For example, the image of a standing bottle, extending on the vertical axis, looks much different from that of a standing plate, typically extending along the horizontal axis. Despite low perceptual similarity at first sight, the objects share an important feature: they have a natural base, and if for a given configuration, they rest on this base (the base of a bottle, for example), these configurations are described with the verb “staan” in Dutch (Lemmens, Citation2002). This configurational property can be deduced from viewing the objects in relation to their surface, but it is a not a straightforward perceptual feature of the objects themselves. The crucial question is thus, whether such verbally encoded distinctions, based on higher-level, perceptually less salient information, may influence visual processing implicitly. If we find a language-perception interaction driven by cross-linguistic differences in the verbal domain, it would show that even such abstract high-level knowledge accumulated through linguistic experience can influence perception in a top-down manner.
With respect to the second question, the effects of language on perceptual discrimination so far have concerned discrete distinctions in linguistic encoding between the respective language systems, i.e., having a label for a particular category or not. Yet, there are many cross-linguistic differences in linguistic encoding that are not categorical. Languages may share a label to encode the same category but differ in the frequency with which that particular category is referred to. For instance, the word polder exists both in English and in Dutch, but in Dutch speaking communities people talk about polders more often than in English-speaking communities. This raises the question whether cross-linguistic differences in linguistic encoding need to be categorical in order to affect perceptual processing. To what extent does the perceptual system draw on statistical regularities and generalizations based on previous linguistic experience and exposure? In other words, is the habitual encoding of a specific visual contrast the formative force in the creation of perceptual biases, rather than the mere presence of a linguistic category in a language system? These questions are at the heart of the language and thought debate: the availability of a word or a structure in a language system does not necessarily result in the perceptual highlighting of this feature in the minds of the speakers of that language; it has been hypothesized that habitual and frequent use of these linguistic means causally leads to perceptual “warping”, i.e., this feature becoming especially salient to language users (Whorf, Citation1976). By contrasting a linguistic category that exists in two languages, but which is encoded habitually in one and only rarely in another, we can directly tap into this question and test the original hypothesis on the influence of habitual language encoding on perception.
A recent EEG study going beyond both labels and categorical encoding variation (in terms of presence vs. absence of a label) between languages is reported in Flecken, Athanasopoulos, Kuipers, and Thierry (Citation2015), who investigate the perception of motion events. The cross-linguistic contrast under investigation concerned the use of grammatical aspect, conveying a given viewpoint on a situation (Comrie, Citation1976). This has consequences for the extent to which languages encode the goal of an ambiguous motion event. Speakers of English, an aspectual language, typically encode an event by zooming in on the specific phase that is in focus. So, when an entity in motion is shown as on its way towards a goal, but the entity does not reach it in the time window of the event depicted, a typical conceptualization in English would involve the trajectory of motion (a woman is walking along the road), and not so much the goal. A holistic perspective is typically taken in German, a language without grammatical means to mark viewpoint aspect (a woman is walking to a bus stop ). Flecken et al. (Citation2015) investigated whether participants’ perception of motion events was biased towards the dimension typically expressed in their respective language. They found a language effect on the P300 component, reflecting task-relevant attention processing. The stimuli were complex (showing a ball moving towards a specific shape, e.g., a square) and participants performed a matching task, i.e., they had to match dynamic animations of motion events with subsequent static depictions of these motion events. The task demands thus ensured conscious monitoring of both trajectory and endpoint, and specifically probed attentional focus to the dimensions of interest. Findings showed a language-specific boost on attention, demonstrating an effect of probabilistic linguistic categorization on visual processing. Moreover, the linguistic difference concerned people’s habitual perspective-taking on entire situations, hence substantially extending effects of labelling conventions in terms of complexity.
The present study will use a cross-linguistic approach to investigate whether the probability of verbally encoding the configuration of an object relative to a surface affects how people see object configurations (e.g., a suitcase on a table). In Dutch, whenever the location or state of an object is verbalized, linguistic specification of its configuration is mandatory (e.g., de koffer staat/ligt op bed “the suitcase stands/lies on the bed”; Ik heb mijn sleutels op de tafel laten liggen “I left my keys lying on the table”). In English, the configuration can in principle be encoded, but typically it is not (“the suitcase is on the bed”; for detailled linguistic analyses, see Ameka & Levinson, Citation2007; Lemmens, Citation2002; Newman, Citation2002). We will investigate whether this difference in probabilistic encoding, that is, high probability (Dutch) as opposed to low probability (English) of encoding of object configurations, leads to a difference in visual processing. Moreover, we will examine at what level of processing these effects may arise, i.e., at the level of judgement, attention or core perception (see Firestone & Scholl, Citation2016; Raftopoulos, Citation2017). In order to fully exploit the categorizing function of language, we will adopt a picture-matching paradigm, rather than a target detection paradigm (used in Boutonnet et al., Citation2013; Thierry et al., Citation2009). These latter studies involved paradigms with only one exemplar of the category of interest (e.g., a circle, a cup) and participants were exposed to this exemplar for a large number of trials, limiting the generalizability of their results (see Maier, Glage, Hohlfeld, & Abdel Rahman, Citation2014). In our study we will present participants with more complex pictures of a variety of objects on surfaces in diverging configurations, by which we reduce the ability to rely on a one-to-one mapping between a label and a percept. Moreover, each condition will contain multiple object configurations, thereby limiting the possibility of item-specific learning effects. This will provide a more complete and ecologically valid test of the relationship between language and perception. We use a picture-matching paradigm which encourages overt stimulus discrimination. In addition, experimental manipulations of the stimulus dimensions are relevant to the matching task, in contrast to Thierry et al. (Citation2009) and Boutonnet et al. (Citation2013) who analyzed a task-irrelevant perceptual feature. This design allows us to inspect potential language-specific patterning of conditions within the P300 time window (as reported previously for complex language-perception interactions; Flecken et al., Citation2015), as well as for the N100 realm (reported for terminological differences; Boutonnet et al., Citation2013; Thierry et al., Citation2009), reflecting discriminatory processing, in addition to post-perceptual judgements in a separate behavioural task. The approach will provide insight into multiple levels of cognitive processing at which language effects may arise.
First, we report a behavioural picture matching task (Experiment 1) in which Dutch and English native speakers were asked to judge whether two pictures presented shortly after each other contained the same or a different object. The picture pairs contained either two different objects, e.g., a bottle on a table followed by a cup on a table (full mismatch), or the same objects, where the second object appeared either in an identical (full match) or a different configuration than the first. Object configurations differed either along the left/right axis, e.g., a cup with the handle facing right, followed by a picture of a cup facing left (orientation mismatch), or along the vertical axis, e.g., a standing bottle following a lying bottle (position mismatch; critical manipulation). We hypothesize no language differences concerning the full match, full mismatch and the orientation mismatch conditions. If the probability of verbal encoding affects perception, then we will find lower accuracy and/or slower RTs on the position mismatch condition in Dutch participants, compared to English. To shed more direct light on visual perception and attention, the same task was conducted in EEG settings (Experiments 2 and 3), this time under an oddball manipulation (see below). Here we expect the largest N100 and P300 effects for the full mismatch (response deviant) condition in both groups. Under the hypothesis of a language-perception interaction, we expect enhanced modulations of N100/P300 effects for the position mismatch condition in Dutch participants only.
Experiment 1
Method
Participants
20 Dutch native speakers (15 female, age M = 21.8, SD = 2.4, range 18–26 years) and 24 English native speakers (11 female, age M = 22.9, SD = 4.6, range 18–35 years) participated in the experiment. Dutch participants were tested at the Max Planck Institute for Psycholinguistics in Nijmegen, the Netherlands; English participants were tested at the University of Bangor, UK. They completed the task as part of another experimental session which they were compensated for.
Materials, design and procedure
Stimuli were created with the open source 3D animation software Blender (we used a subset of the items in van Bergen & Flecken, Citation2017). They consisted of pictures representing one of ten household objects placed on a table. Each object’s configuration was manipulated by rotating it 90° along the horizontal and/or the vertical axis, yielding 4 pictures per object, and 40 unique pictures in total. With these 40 pictures we constructed 160 prime-target picture pairs, occurring in four conditions. In the Full Match condition, prime and target picture were identical. In the Full Mismatch condition, the target picture contained a different object than the prime picture, and it appeared in a different configuration than the prime object. The other two conditions consisted of a partial mismatch: in the Position Mismatch condition, prime and target objects were the same but differed in vertical orientation with respect to the table; in the Orientation Mismatch condition, prime and target objects were the same but differed in horizontal orientation vis-à-vis the table. An example of an experimental item in all conditions is presented in .
The Full Match condition contained 40 unique prime-target pairs. To create 40 unique prime-target pairs in the Full Mismatch condition, each of the four configurations of the prime object was combined with a different target object, such that participants never saw two objects paired twice. For both the Position Mismatch and the Orientation Mismatch condition, there were two unique pairs per object, making 20 unique pairs per condition. These pairs were repeated once to arrive at 40 trials. For repeated pairs, the prime picture in the first half was the target picture in the second half and vice versa.
Pictures were presented in the centre of the screen on a white background on a 24-inch LCD monitor. After a 500 ms fixation cross, the prime picture was presented for 300 ms, followed by a blank screen for 500 ms. Next, the target picture was displayed. Participants were instructed to press the right button on a button box with their right index finger if the target picture displayed the same object as the prime picture (i.e., the Full Match, Orientation Mismatch, and Position Mismatch condition), and to press the left button with their left index finger if the target picture contained a different object than the prime picture (Full Mismatch condition). The target picture stayed on the screen until the participant provided a response; the button press triggered the start of the next trial. To ensure that participants could not rely purely on their visual-spatial working memory, prime and target pictures differed in size: Prime pictures were 960 by 960 pixels, target pictures were half this size. Participants were seated about 1 m from the screen; target pictures subtended approximately 8 × 8° of visual angle. An example of a trial sequence is presented in .
Experimental conditions were presented in a pseudo-randomized order. The experiment consisted of 160 trials; the full experiment took approximately 10 min.
Analyses
Accuracy and reaction times were analyzed with mixed-effects regression models using the lmerTest package (Kuznetsova, Brockhoff, & Christensen, Citation2017) in R (R Core Team, Citation2008). Models included the maximal random effects structure justified by the design (Barr, Levy, Scheepers, & Tily, Citation2013).
Results and discussion
Five participants (four English, one Dutch) had to be excluded from analyses because of poor performance, likely due to a misinterpretation of the task. Response latencies faster than 200 ms and longer than 2.5 SD above participant means were removed, yielding 2.6% data loss.
Results are presented in . A logistic mixed-effects analysisFootnote3 predicting the probability of a correct response on the basis of Condition (4 levels, Full Match as reference category), Language (Dutch vs, English, centred), and their interaction revealled that accuracy for Full Match pairs was significantly higher than for Full Mismatch pairs, β = 0.83, SE = 0.19, p < .001. We found no evidence for a difference in accuracy between Full Match and Orientation Mismatch pairs, β = −0.10, SE = 0.21, p = .64, but accuracy for Position Mismatch pairs was significantly lower than for Full Match pairs, β = −0.85, SE = 0.19, p < .001. These results indicate that correctly identifying an object match was more difficult if the target object was rotated along the vertical axis, but not if it was rotated along the horizontal axis. Crucially, we found no evidence for a Condition by Language interaction effect (comparing models with vs. without the interaction effect: X2 (3) = 6.05, p = .11), suggesting that Dutch and English participants were similarly affected by the position distinction when performing the matching task. A linear mixed-effects regression analysis of (log-transformed) reaction timesFootnote4 revealled a similar pattern. Correct responses in the Full Match condition were significantly faster than correct rejections in the Full Mismatch condition, β = −0.18, SE = 0.02, p < .001. There was no evidence for a difference in reaction times between Full Match and Orientation Mismatch pairs, β = 0.01, SE < 0.01, p = .28, but responses were significantly longer in the Position Mismatch condition relative to the Full Match condition, β = 0.03, SE < 0.01, p < .001. There was no evidence for a Condition by Language interaction effect (X2 (3) = 4.95, p = .18), again suggesting that the position distinction affected Dutch and English participants’ response behaviour to the same extent.
Figure 3. Accuracy (left panel) and reaction times (right panel) per condition, split up by language group. Error bars represent +/− 1 SE.
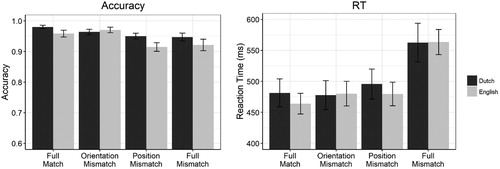
In sum, our results demonstrate that the difference in probabilistic verbal encoding of object position between Dutch and English is not reflected in behaviour on a perceptual task. These findings contrast with earlier studies showing cross-linguistic differences in colour discrimination tasks (e.g., Regier & Kay, Citation2009; Winawer et al., Citation2007). Our results possibly suggest that probabilistic verbal encoding differences are not as entrenched as categorical distinctions encoded in terminology. However, behavioural measures reflect for a large part conscious decision making, rather than perception per se (see Firestone & Scholl, Citation2016). To investigate whether the cross-linguistic contrast in verbal encoding probability affects perception or attention more directly, we performed an EEG version of the same experiment.
Experiment 2
Method
Participants
33 Dutch native speakers (26 female; age: M = 22.0 years, SD = 2.60, range 19–32) and 28 native speakers of English (21 female; age: M = 21.4 years, SD = 2.66, range 18–31) participated. All were tested in Nijmegen, the Netherlands (Max Planck Institute for Psycholinguistics). Dutch participants were students from Radboud University; English participants were exchange students temporarily studying at Radboud University, originating from the US, the UK or Canada. They reported to have no or very poor knowledge of Dutch (or any other language displaying a similar semantic contrast, such as German). They were recruited in the week of arrival in the Netherlands and tested within a month after arrival, ensuring they were in an English-dominant language mode at the time of testing. All participants received course credits or cash for participation in the experiment, which lasted approximately 1.5 h.
Materials and procedure
Stimuli were the same as those used in Experiment 1. Using the 40 pictures outlined above we constructed 400 prime-target picture pairs occurring in the same four conditions as in Experiment 1 (see ), but now following a visual oddball paradigm: a Standard condition occurring 70% of the time (280 trials) and three Deviant conditions, each occurring in 10% of the cases (40 trials). The Full Match condition was used as the Standard; the Full Mismatch condition occurred as the Response Deviant. The Position Mismatch condition served as the Critical Deviant, and the Orientation Mismatch condition as the Control Deviant. During the experiment, all 40 pictures occurred both as primes and targets. Each configuration of each object occurred only once as the target picture in every Deviant condition (40 trials per deviant), whereas it occurred as the target in the Standard condition seven times (ensuring 280 trials for the full match condition).
Participants performed a go/no-go task: they were instructed to press a button if and only if the second picture displayed a different object than the object shown in the preceding picture (Response Deviant). They were asked to withhold their response until a question mark appeared on the screen. The prime picture was presented for 200 ms, followed by a fixation cross for 500 ms. Next, the target picture was displayed for 200 ms, followed by a blank screen for 800 ms. Then, a question mark appeared in the centre of the screen for 1000 ms, prompting a response if required by the trial. The inter-trial interval (a blank screen) was 500 ms. An example of a trial sequence is presented in .
ERPs were time-locked to target picture onset. To avoid eye movements during the critical ERP-window, as well as to ensure that participants could not rely purely on their visual-spatial working memory, target pictures were again half the size of prime pictures.
Experimental conditions were presented in a pseudo-randomized order such that rare trial types (Deviants) occurred once in 10 trials and were never presented in immediate succession. The experiment consisted of 4 blocks of 100 trials, with self-timed breaks in between. After the experiment, participants performed a short picture description task. They were asked to describe in one sentence what they saw for 10 of the experimental pictures. All participants were presented with each household object once, in one of its four configurations; object configurations were counterbalanced between participants. Pictures were presented for 200 ms followed by a blank screen, during which the participant verbally described the picture. The experiment leader immediately transcribed the participant’s description; when the transcription was completed, the next picture was presented.
EEG recording and analyses
EEG data were recorded with BrainVision Recorder 1.20.041™ in reference to the left mastoid. Sampling was set to 500 Hz (online a low cut-off [0.016 Hz] and high cut-off [125 Hz] filter was applied) and data were recorded from 32 active electrodes, 27 of which were placed on the scalp according to the 10–20 convention (ActiCAP system). In addition, an electrode was placed above and below the left eye to monitor blinks, plus 2 electrodes to the left/right of the left/right eye to monitor horizontal eye movements (additional electrodes were placed on the mastoids). Impedances were kept below 10 kΩ. EEG activity was re-referenced offline to the average of both mastoids and filtered with a low pass zero phase-shift filter (30 Hz, 48 dB/oct). Eye movement artefacts were corrected on the basis of the four eye electrodes using the Gratton & Coles automatic ocular correction procedure, implemented in BrainVision Analyzer 2™. Individual ERPs for each condition were computed from epochs ranging from −100 to 1000 ms after stimulus onset and baseline corrected in reference to 100 ms of pre-stimulus activity. Automatic artefact rejection discarded all epochs with an activity exceeding +/−75 microvolts. The remaining segments were averaged per participant and per condition.
The N100 analysis concentrated on a time window ranging from 110 ms to 160 ms after target picture onset (+/−25 ms from the N100 peak, which was found at 135 ms) at three occipital electrodes (O1/2, Oz) which were predicted to be maximally sensitive to the effects of interest (Boutonnet et al., Citation2013; Thierry et al., Citation2009). The P3 was analyzed at central-parietal electrodes (CP1/CP2, Pz, P3/P4) between 350 and 700 ms after target picture onset, following Flecken et al. (Citation2015).
First, we analysed raw amplitudes with an omnibus Condition (Exp. 2: 4 levels; Exp. 3: 3 levels) by Language (2 levels) ANOVA. In case of a significant interaction, we computed difference waves by subtracting the standard full match condition from each deviant condition, within each group (the deviancy effects). Each deviant-minus-standard was then compared between groups using independent samples t-tests. In addition, we examined whether the deviant conditions (object mismatch, position mismatch, and additionally in Exp. 2 orientation mismatch) differed significantly from the standard (full match) condition within each language group, to see whether the deviants elicited a N100/P300 effect respectively (Condition ANOVAs for each group separately).
Results and discussion
Six participants had to be excluded from the analyses due to poor behavioural performance (i.e., an exessive number of false alarms: 2 Dutch; 1 English), bad EEG recording quality (1 Dutch participant with more than 25% trial removal due to artefacts on one of the conditions) or their language history (1 Dutch participant who was adopted and did not learn Dutch until the age of 3; 1 German-Dutch early bilingual).Footnote5
Behavioral results
Response accuracy was very high for both groups (Dutch: 98%, English: 98% correct button presses).
ERP results
and below shows the grand averaged ERP waveforms for all conditions, on a total of 9 electrodes on frontal, central and parietal scalp regions for the entire segment, for Dutch () and English () participants.
N100
Unexpectedly, N100 activity was maximal at frontal-central electrodes, rather than in occipital scalp regions typical for the discriminatory N100 and reported previously for related paradigms (Boutonnet et al., Citation2013; Thierry et al., Citation2009); this prevented us from performing the analysis planned a priori. The anterior distribution more closely resemblled the N100 reported in Vogel and Luck (Citation2000) for a task requiring overt responses on part of participants, not straightforwardly linked to perceptual discrimination. Although the matching task in the current experiment did not require an overt response in the critical conditions, all manipulations were in fact task-relevant; in this respect, the current paradigm differed from the previously discussed N100 paradigms in which critical manipulations were task-irrelevant (Boutonnet et al., Citation2013; Thierry et al., Citation2009). To explore potential modulations of this anterior N100, we analyzed average voltage activity on electrodes Fz, F3/F4, FCz, FC1/FC2, Cz, C3/C4 (following Vogel & Luck, Citation2000). An omnibus mixed ANOVA of Condition by Language on raw amplitudes showed no main effect of Condition (F (3,159) = .73, p = .54 ns), but a significant main effect of Language (F (1,53) = 6.66, p < .05) and an interaction between Language and Condition (F (3,159) = 2.97, p < .05). Follow-up independent samples t-tests compared difference waves of each deviant-minus-standard condition between language groups. We found no Language effect for Full Mismatch deviants, t (53) = 0.99, p = .32, nor for Orientation Mismatch deviants, t (53) = 0.41, p = .69. Difference waves of Position Mismatch (minus Full Match) trials did reveal a Language effect, t (53) = 2.37, p < .05: the N100 difference between Position Mismatch trials and Full Match trials was significantly larger in Dutch participants relative to English participants. However, a one-way ANOVA of mean raw amplitudes showed no significant effect of Condition in the Dutch dataset, F (3,81) = 1.31, p = .28 nor in the English dataset, F (3,78) = 2.32, p = .08: we hence found no evidence for the presence of an anterior N100 effect in any condition.
shows the difference waves of each deviant condition minus the standard Full Match condition for the N100 realm on the selected subset of electrodes in each language group.
P300
An omnibus mixed ANOVA of Condition by Language on raw amplitudes showed a main effect of Condition (F (3,159) = 70.69, p < .001), no main effect of Language (F (1,53) = 2.56, p = .12 ns), and no interaction between Language and Condition (F (3,159) = .18, p = .91 ns). The main effect of Condition was explored further by comparing the difference wave for Position mismatch (minus Full match) against the two other deviants, collapsed across language groups: Position mismatch (minus full match) deviants differed significantly from Full mismatch deviants (t (54) = 7.13, p < .05), with the latter response condition eliciting the most positive P300 wave. Position mismatch trials were significantly more positive than Orientation mismatch trials (t (54) = −2.39, p < .05), suggesting that Position Mismatch trials were processed with a higher degree of attention, regardless of participants’ language background.
Analyses of raw condition amplitudes, collapsed across language groups, showed a significant P300 effect for the response condition (Full Mismatch vs Full Match: t (54) = 11.16, p < .001), as well as for the Orientation Mismatch condition (Orientation Mismatch vs Full Match: t (54) = 8.35, p < .001), and the critical Position Mismatch condition (Position Mismatch vs Full Match: t (54) = 7.64, p < .001). These analyses show that all Deviant conditions elicited a significant P300 effect compared to the Standard condition.
below plots the mean difference waves of each Deviant-minus-Standard condition for the P300 time window per language group on the above-defined subset of electrodes.
Figure 8. Mean amplitudes for each Condition (Deviant – Standard) per Language in the P300 time window (average of a subset of central-parietal electrodes) in Experiment 2. Error bars represent +/− 1 SE.
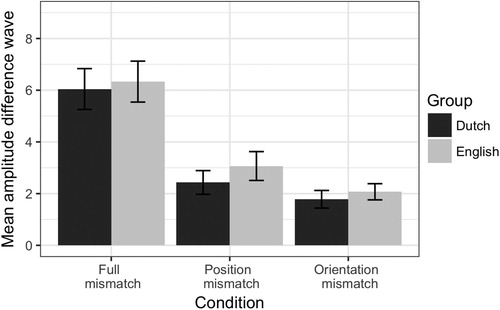
In sum, findings from Experiment 2 provided no evidence for a cross-linguistic difference in perceptual attention to object configurations (P300). The results regarding the N100 were less straightforward. Different from what was expected, the N100 peak was not found on occipital regions, but showed a frontal-central distribution instead (in line with Vogel & Luck, Citation2000). An exploratory analysis of N100 activity on frontal-central electrode sites revealled a marginal language effect: the N100 difference between Position Mismatch trials and Full Match trials was enhanced in Dutch participants compared to English participants. However, we found no evidence for a difference in N100 amplitude between any deviant condition and the standard condition in either of the language groups. Further, the unusual scalp topography does not allow us to argue for an effect on a discriminatory process (see Vogel & Luck, Citation2000). Thus, in order to further investigate the suggestive cross-linguistic difference found in this experiment, we ran a second EEG experiment with two different groups of English and Dutch native speakers, including only the Object mismatch and Position mismatch conditions as deviants (hence taking out the Orientation mismatch condition). We reasoned that if the previously attested N100 difference between language groups is indeed a genuine effect, a design with fewer deviants should boost effects of Position Mismatch deviants, and hence enhance potential cross-linguistic differences. We thus predicted a larger N100 difference between Position Mismatch and Full Match trials in Dutch participants compared to English participants.
Experiment 3
Method
Participants
32 Dutch native speakers (23 female; age: M = 21.7 years, SD = 1.79, range 19–25) and 35 native speakers of English (22 female; age: M = 23.1 years, SD = 3.38, range 19–35) participated in the experiment. All were tested in Nijmegen, the Netherlands (Max Planck Institute for Psycholinguistics). Dutch participants were again students from Radboud University; English participants were exchange students temporarily studying at Radboud University, originating from the US, the UK or Canada. They reported to have no or very poor knowledge of Dutch or German. All participants received course credits or cash for participation in the experiment, which lasted approximately 1.5 h.
Materials and procedure
The same materials and procedure were used as in Experiment 1, except this time, the Orientation mismatch condition was excluded from the experiment entirely. The remaining conditions were again manipulated following a visual oddball paradigm: The Standard condition now occurred 80% of the time (320 trials) and the two Deviant conditions each occurred in 10% of the cases (40 trials).
EEG recording and analyses
The same recording and analyses procedures as described above were adopted. Both the N100 and the P300 analyses concerned the same time windows as denoted above. Also in this Experiment, N100 activity was maximal at frontal-central scalp sites; the grand averaged N100 peak was again found at 135 ms.
Results
A total of 11 participants had to be excluded due to poor behavioural performance (two Dutch and one English participant; nearly all full mismatch trials were misses), bad EEG data quality (five English and two Dutch participants; more than 25% trial loss due to artefacts in at least one of the conditions), or their language background (one English participant was a German-English early bilingual).Footnote6
Behavioral results
Overall, participants performed the task with high accuracy (Dutch participants: 99% accuracy; English participants: 98.8% on average).
ERP results
and below depict the grand averaged ERP waveforms for all conditions, on a total of 9 electrodes on frontal, central and parietal scalp regions for the entire segment, for Dutch () and English () participants.
N100
An omnibus mixed ANOVA of Condition by Language on raw amplitudes revealled no main effect of Language, F (1,54) = 1.34, p = .25, nor of Condition, F (2,108) = .85, p = .43, but a significant Language by Condition interaction, F (2,108) = 3.60, p < .05. Follow-up independent samples t-tests of each difference wave (Deviant-minus-Standard) showed no Language difference for Full Mismatch deviants, t (54) = −0.40, p = .69, but Position Mismatch (minus Full Match) trials did differ between language groups, t (54) = 2.66, p = .02: this time, however, the difference between Position Mismatch and Full Mismatch trials was enhanced for English participants compared to Dutch participants.
A one-way ANOVA on the Dutch raw data showed no main effect of Condition, F (2,54) = 1.17, p = .32, suggesting that none of the deviants elicited a significant N100 effect in Dutch participants. The English raw data, on the other hand, did show a significant Condition effect, F (2,54) = 3.906, p < .05; a paired samples t-test revealled that amplitudes in the Position Mismatch condition were significantly enhanced relative to the Full Match condition, t (27) = −3.35, p < .05. However, amplitudes in the Full Mismatch condition did not significantly differ from the Full Match condition, t (27) = −0.61, p = .55.
shows the difference waves between each deviant condition and the standard Full Match condition for each language group in the N100 time window.
P300
An omnibus Condition by Language mixed ANOVA on raw amplitudes showed no main effect of Language (F (1,54) = .94, p = .34 ns), but a main effect of Condition (F (2,108) = 99.91, p < .001). The interaction was not significant, F (2,108) = 1.02, p = .37 ns. The main effect of Condition indicated enhanced P300 effects for the Full Mismatch (minus Full Match) condition compared to the Position Mismatch (minus Full Match) condition, irrespective of language group (independent t-test comparing difference waves of the two deviant conditions: t (55) = 6.50, p < .001).
Analyses of raw amplitudes collapsed across Language groups showed P300 effects (relative to the Full Match condition) for the Full Mismatch deviant (t (55) = 11.69, p < .001) as well as the critical Position Mismatch condition (t (55) = 12.54, p < .001). shows mean amplitudes of each deviant condition minus the standard full match condition in each language, for the P300 window and region.
Figure 12. Mean amplitudes for each Condition (Deviant – Standard) and language in the P300 time window (average of a subset of central-parietal electrodes) in Experiment 3. Error bars represent +/− 1 SE.
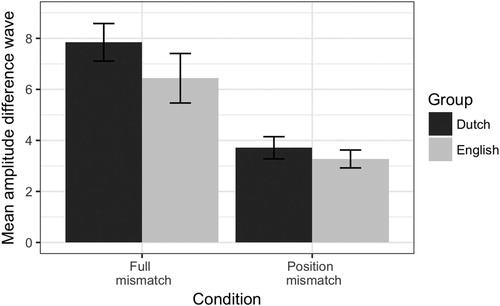
Taken together, findings from Experiment 3 do not confirm our hypothesis of enhanced attention and perceptual discrimination of object position in Dutch relative to English participants. As in Experiment 2, the P300 results showed no evidence for a language effect on attention. Moreover, we did not find the hypothesized boost in the N100 effect for Position mismatch deviants in Dutch participants; instead, N100 amplitude in this condition was enhanced for English participants. In addition, we found no evidence for a difference in N100 amplitude between Response deviants and standards (which was predicted to elicit the largest N100 effect) in either language group. Again, the N100 scalp topography was frontal-central rather than occipital, making it unclear whether or not the data reflect perceptual discrimination processes. Given that the N100 results were both against our initial hypothesis and inconsistent, we will not interpret these findings any further.
General discussion
This study set out to shed light on the extent to which probabilistic relational categorization on the basis of posture verbs influences perceptual processing, as measured by behavioural responses and modulations of specific ERP components. Using non-verbal perceptual tasks, we targeted implicit language-on-perception effects. By studying cross-linguistic variation in the verbal domain, we were able to study potential penetrability of perception by more complex linguistic categories than previously investigated (e.g., colour terms, object nouns). In addition, by looking at a linguistic distinction that differs in terms of usage frequency, we explored in how far language-perception interactions are driven by statistical knowledge, and hence to what extent the perceptual system relies on generalizations drawn from prior linguistic experiences.
In our cross-linguistic comparison of behavioural and ERP responses to object configurations, we found similar patterns in Dutch and English participants for variations in object position, a dimension encoded obligatorily in Dutch but not in English. These findings suggest that perception of object configurations is not influenced by probabilistic verbal encoding of this dimension, when studying post-perceptual judgements (Experiment 1) and perceptual attention (P300, Experiments 2 & 3). The inconsistent findings on the N100 (Experiments 2 & 3) prevent us from providing a conclusive answer to whether or not there are language effects on early perceptual processing. This is perhaps due to the increased visual complexity of the pictures used, or the use of multiple pictures rather than repeating a single picture in each condition (cf. Boutonnet et al., Citation2013; Thierry et al., Citation2009). However, if there really were language effects on core perceptual processes, we would predict these effects to have a cascading effect on later processing stages. Given that we did not find evidence for language effects on the P300 component in Experiments 2 and 3 (where findings were consistent), we find it unlikely that earlier processing stages would be affected by the probability of verbal encoding of object position. We are aware that absence of evidence is not evidence of absence. However, given the fact that we ran three experiments, one of which (Experiment 3) partly replicated an earlier one (Experiment 2), and found mostly consistent patterns, we feel justified to at least put forward a set of hypotheses on the basis of a solid set of experimental, in part neuroscientific data.
The similar patterns for Dutch and English participants in the present study leave two possible scenarios to the question as to what role language played during the task. Our findings could indicate (1) the absence of a language effect in both Dutch and English participants, or (2) the presence of an effect of language in both groups, meaning that English participants used their knowledge of posture verbs to the same extent as Dutch participants. The first scenario would imply that language-perception interactions do not extend to more complex linguistic categories, i.e., that such effects do not go beyond labels referring to single units: Posture verbs may not strengthen associations with specific percepts (“standing” or “lying” configurations) to the same extent as labels for colours or objects, for instance because different exemplars of standing objects are perceptually less similar than different exemplars of blue, or various exemplars of mugs. Recall, however, that we found overall enhanced processing of Position Mismatch trials relative to Orientation Mismatch trials in both Experiments 1 and 2: we found increased misses for position mismatch in Experiment 1, and enhanced attention in Experiment 2. These findings thus show prioritized processing of object position relative to the other configuration that was manipulated in the stimuli (left-right orientation), suggesting that the categories of standing and lying are perceived as distinct from other visual features. It is therefore unlikely that discriminating pictures on the basis of configurational information is by default “too complex” for the visual system. Moreover, prior studies have reported language-perception effects in relation to similarly complex relational categories as expressed by verbs, both explicitly and implicitly. For example, verbs expressing up- or downward motion have been shown to influence discrimination and detection of visual motion stimuli in a pre-cueing paradigm (Francken et al., Citation2015; Meteyard et al., Citation2007). Likewise, the study of Flecken et al. (Citation2015), targeting implicit effects of grammatical viewpoint aspect, evidenced language effects on attention as reflected in P300 effects. Thus, research in the field to date would not support the simplistic hypothesis that linguistic encoding of complex categories cannot influence visual processing.
The second scenario implies that language-perception interactions may not be sensitive to probabilistic language differences. We believe this scenario to be more likely, given the attested differences between the critical Position Mismatch trials and the control Orientation Mismatch trials in both language groups in the present experiments. The mere existence of posture verbs, and hence familiarity with the categories of “stand” and “lie”, in a language may have been enough to evoke the enhanced Position Mismatch effects we found. Note that in both Dutch and English, posture verbs are present and frequently used; the main difference is that in English, their use is largely restricted to encode human posture, whereas in Dutch, their meanings encompass both human posture and object configurations (see Ameka & Levinson, Citation2007; Newman, Citation2002). This cross-linguistic difference in the semantic criteria for applying posture verbs was hypothesized to lead to different perceptual effects. However, arguably, for a speaker of English to understand that posture verbs can also apply to positions of inanimate entities, does not require the creation of a completely new category; rather, it requires the extension of an existing category. It is arguably not hard to draw an analogy between human posture and the object configurations used in our experiments on the basis of perceptual features, i.e., to classify objects that are higher than wide as standing, and objects that are wider than high as lying. Given the strong perceptual overlap between human posture and object configurations, the cross-linguistic difference in probabilistic encoding of the relational categories may not have led to enhanced effects of Position Mismatch trials in Dutch participants. Instead, the relevant perceptual features may have stood out to English participants in this matching task just as well, based on their knowledge of the posture verb categories (see also Folstein, Palmeri, & Gauthier, Citation2013).
An interesting test case would be to study object configurations for which this perceptual analogy does not hold. In Dutch, posture verbs also mark object configurations that are not solely perceptually based. If objects have a natural base, and if they rest on this base, they are linguistically categorized as “standing”. This means that a plate on a table is described as standing, although perceptually extending along the horizontal axis. If an object does not have a natural base, e.g., a ball, it is categorized as “lying”, even though a ball is as wide as it is high. Previous research has shown that such atypical instances are hard to acquire for second language learners of Dutch (Gullberg, Citation2009). In a visual world eye-tracking experiment on incremental language comprehension (van Bergen & Flecken, Citation2017), native Dutch participants were shown to predict atypical object configurations on the basis of the information encoded in posture verbs (as reflected in anticipatory eye movements to objects depicted in a visual world); these predictive processing effects were not found in English second language speakers of Dutch. Based on these findings, cross-linguistic differences are to be expected in perceptual tasks that involve less typical object configurations: Dutch speakers, compared to English speakers, may show enhanced perceptual processing when dealing with these instances. A picture-matching paradigm as used here would not allow to address this issue, given that for objects that do not have a natural base, a position mismatch condition cannot be created (e.g., a ball cannot “stand” given the lack of a base, according to Dutch logic). Future studies could make use of, for example, a pre-cueing paradigm, in which posture verbs are presented prior to pictures of object configurations, to address explicit effects of language on perception of those pictures.
Another interesting follow-up idea would be to test the extent to which differences in probabilistic encoding can lead to perceptual differences in less complex categories. In the current study, categorizing object configurations as standing and lying may be rare in English, but as described above, there is considerable overlap with the highly familiar and well-established human posture categories. Future studies could target less complex categories that vary across languages in terms of frequency of use: for instance, the word “polder” is ubiquitous in the Dutch language, but probably less frequently used – and less familiar – in English speakers. Such probabilistic differences may not have substantial effects on conscious, high-level cognitive operations, such as perceptual judgements; once you are familiar with a given linguistic category, you are probably also aware of the perceptual information relevant to this category, so frequency of use may not matter for overt judgements. It is an open question whether processing speed (e.g., behavioural response latencies), visual attention (measured with eye tracking or EEG) or early perceptual processes are influenced by such differences in probabilistic encoding.
Generally, it is important for future studies to be clear as to the level of cognitive processing that a given experimental task taps into. It is likely that language plays a different role at different stages of cognitive processing. Therefore finding a null effect in one experiment for a given domain, does not mean that there won’t be language effects on other cognitive processes, and in different experimental tasks. In all, we advocate the use of experiments tapping into different cognitive operations, with different time scales and different measurements in the language and thought debate (see also, Maier et al., Citation2014; discussion in Firestone & Scholl, Citation2016). Only this approach will lead to a clear picture regarding the when and how of language influencing human cognition.
Acknowledgements
We would like to thank Guillaume Thierry for help in collecting the English data of Experiment 1, and for helpful feedback on this project. In addition, we thank Caspar Hautvast for facilitating this collaboration, and Arfryn for their hospitality.
Disclosure statement
No potential conflict of interest was reported by the authors.
Additional information
Funding
Notes
1 Thierry et al. (Citation2009) refer to this component as visual Mismatch Negativity (vMMN).
2 Boutonnet et al. (Citation2013) use the term Deviance-Related Negativity (DRN).
3 The final model included random intercepts for participants and items.
4 The final model included random intercepts for participants and items, as well as random by-participant slopes for Condition.
5 Participants included in the analysis were 28 native speakers of Dutch (26 female; age: M = 21.3 years, SD = 1.60, range 19–25) and 27 English native speakers (20 female; age: M = 21.5 years, SD = 2.69, range 18–31).
6 Participants included in the analysis were 28 native speakers of Dutch (21 female; age: M = 21.5 years, SD = 1.7, range 19–26) and 28 English native speakers (18 female; of 4 participants no age information was recorded; the mean age of the other 24 participants was 22.7 years, SD = 2.1, range 19–27).
References
- Abdel-Rahman, R., & Sommer, W. (2008). Seeing what we know and understand: How knowledge shapes perception. Psychonomic Bulletin & Review, 15(6), 1055–1063. doi: 10.3758/PBR.15.6.1055
- Ameka, F. K., & Levinson, S. C. (Eds.). (2007). The typology and semantics of locative predication: Posturals, positionals and other beasts [special issue]. Linguistics, 45(5).
- Barr, D., Levy, R., Scheepers, C., & Tily, H. (2013). Random effects structure for confirmatory hypothesis testing: Keep it maximal. Journal of Memory and Language, 68(3), 255–278. doi: 10.1016/j.jml.2012.11.001
- Boutonnet, B., Dering, B., Viñas-Guasch, N., & Thierry, G. (2013). Seeing objects through the language glass. Journal of Cognitive Neuroscience, 25, 1702–1710. doi: 10.1162/jocn_a_00415
- Boutonnet, B., & Lupyan, G. (2015). Words jump-start vision: A label advantage in object recognition. Journal of Neuroscience, 35(25), 9329–9335. doi: 10.1523/JNEUROSCI.5111-14.2015
- Comrie, B. (1976). Aspect. Cambridge: Cambridge University Press.
- Edmiston, P., & Lupyan, G. (2015). What makes words special? Words as unmotivated cues. Cognition, 143, 93–100. doi: 10.1016/j.cognition.2015.06.008
- Firestone, C., & Scholl, B. J. (2016). Cognition does not affect perception: Evaluating the evidence for “top-down” effects. Behavioral and Brain Sciences, 39, e229. doi: 10.1017/S0140525X15000965
- Flecken, M., Athanasopoulos, P., Kuipers, J. R., & Thierry, G. (2015). On the road to somewhere: Brain potentials reflect language effects on motion event perception. Cognition, 141, 41–51. doi: 10.1016/j.cognition.2015.04.006
- Folstein, J. R., Palmeri, T. J., & Gauthier, I. (2013). Category learning increases discriminability of relevant object dimensions in visual cortex. Cerebral Cortex, 23(4), 814–823. doi: 10.1093/cercor/bhs067
- Francken, J. C., Kok, P., Hagoort, P., & de Lange, F. P. (2015). The behavioral and neural effects of language on motion perception. Journal of Cognitive Neuroscience, 27, 175–184. doi: 10.1162/jocn_a_00682
- Gentner, D., & Namy, L. L. (2006). Analogical processes in language learning. Current Directions in Psychological Science, 15(6), 297–301. doi: 10.1111/j.1467-8721.2006.00456.x
- Gilbert, C. D., & Li, W. (2013). Top-down influences on visual processing. Nature Reviews Neuroscience, 14(5), 350–363. doi: 10.1038/nrn3476
- Gullberg, M. (2009). Reconstructing verb meaning in a second language. How English speakers of L2 Dutch talk and gesture about placement. Annual Review of Cognitive Linguistics, 7, 221–244. doi: 10.1075/arcl.7.09gul
- Kuznetsova, A., Brockhoff, P., & Christensen, R. (2017). Lmertest package: Tests in linear mixed effects models. Journal of Statistical Software, 82(13), 1–26. doi: 10.18637/jss.v082.i13
- Lemmens, M. (2002). The semantic network of Dutch posture verbs. In J. Newman (Ed.), The linguistics of sitting, standing and lying (pp. 103–139). Amsterdam: John Benjamins.
- Lupyan, G., & Bergen, B. (2015). How language programs the mind. Topics in Cognitive Science, 8, 408–424. doi: 10.1111/tops.12155
- Lupyan, G., & Thompson-Schill, S. L. (2012). The evocative power of words: Activation of concepts by verbal and nonverbal means. Journal of Experimental Psychology: General, 141(1), 170–186. doi: 10.1037/a0024904
- Maier, M., Glage, P., Hohlfeld, A., & Abdel Rahman, R. (2014). Does the semantic content of verbal categories influence categorical perception? An ERP study. Brain and Cognition, 91, 1–10. doi: 10.1016/j.bandc.2014.07.008
- Meteyard, L., Bahrami, B., & Vigliocco, G. (2007). Motion detection and motion verbs: Language affects low-level visual perception. Psychological Science, 18, 1007–1013. doi: 10.1111/j.1467-9280.2007.02016.x
- Newman, J. (2002). The linguistics of sitting, standing and lying. Amsterdam: John Benjamins.
- Raftopoulos, A. (2017). Pre-cueing, the epistemic role of early vision, and the cognitive impenetrability of early vision. Frontiers in Psychology, 8, 1156. doi: 10.3389/fpsyg.2017.01156
- R Development Core Team. (2008). R: A language and environment for statistical computing. Vienna: R Foundation for Statistical Computing. ISBN 3-900051-07-0. Retrieved from http://www.R-project.org
- Regier, T., & Kay, P. (2009). Language, thought, and colour: Whorf was half right. Trends in Cognitive Science, 13(10), 439–446. doi: 10.1016/j.tics.2009.07.001
- Summerfield, C., & de Lange, F. P. (2014). Expectation in perceptual decision-making: Neural and computational mechanisms. Nature Reviews Neuroscience, 15, 745–756. doi: 10.1038/nrn3838
- Thierry, G., Athanasopoulos, P., Wiggett, A., Dering, B., & Kuipers, J. R. (2009). Unconscious effects of language-specific terminology on preattentive color perception. Proceedings of the National Academia of Science, 106, 4567–4570. doi: 10.1073/pnas.0811155106
- van Bergen, G., & Flecken, M. (2017). Putting things in new places: Linguistic experience modulates the predictive power of placement verb semantics. Journal of Memory and Language, 92, 26–42. doi: 10.1016/j.jml.2016.05.003
- Vogel, E. K., & Luck, S. (2000). The visual N1 component as an index of a discrimination process. Psychophysiology, 37(2), 190–203. doi: 10.1111/1469-8986.3720190
- Whorf, B. (1976). Language, thought and reality. Cambridge: MIT Press.
- Winawer, J., Witthoft, N., Frank, M., Wu, L., Wade, A., & Boroditsky, L. (2007). Russian blues reveal effects of language on color discrimination. Proceedings of the National Academy of Sciences, 104, 7780–7785. doi: 10.1073/pnas.0701644104