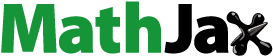
ABSTRACT
Research investigating the drivers of consumers’ engagement with brands on social media is proliferating. However, little is known about how advertising outside social media drives engagement with brands on social media. This study aims to explore the relation between advertising spend in different offline media (TV, radio, newspapers, magazines, out of home), and reach of and engagement with brand pages on Facebook. The study uses a unique real-life data-set containing information about the Facebook pages of 45 brands for approximately three years combined with Nielsen Advertising Spend data. Results showed that while advertising in offline media influenced organic and viral reach, the number of page likes was directly influenced by advertising on Facebook only. It can be concluded that offline advertising is relevant in driving consumers’ online brand engagement; however, there is a unique set of drivers for organic reach, viral reach and likes.
Introduction
Advertisers often aim to drive consumers’ online brand engagement on social media. They try to stimulate consumers to engage with branded content not only because they want to reach these consumers, but also because they believe that the content might spread into the consumers’ social network (Scheinbaum Citation2016). In the last few years, an increasing body of academic research has investigated the drivers of consumers’ engagement with brands on social media. However, existing studies mainly tried to answer this question from a micro-level perspective. For instance, by studying which content characteristics (e.g. positivity, emotionality, and interactivity) of brand posts are related to consumers’ online brand engagement (Berger and Milkman Citation2010; Araujo, Neijens, and Vliegenthart Citation2015; Yuki Citation2015), or by investigating how consumer perceptions of brand pages (e.g. of argument quality or attractiveness) influence consumers’ online brand engagement (Chang, Yu, and Lu Citation2015; Vries and Carlson Citation2014).
This study takes an entirely different perspective by arguing that the drivers of consumers’ engagement with brands on social media should be sought in earlier stages of the customer journey outside of social media. As consumers are exposed to a multitude of branded messages daily, it is likely that these brand activities outside of social media affect the way in which consumers engage with brands on social media. Exploring these drivers from a more macro perspective allows us to generate novel insights into how consumers’ passive exposure to branded messages across offline media relates to their active engagement with brands online. More specifically, this study aims to explore the relation between advertising activities in different offline media (TV, radio, newspapers, magazines, trade publications, and out-of-home) and engagement with brands and their contents on Facebook at varying levels of activeness (organic reach, viral reach, and page likes, similar to Muntinga, Moorman, and Smit [Citation2011]). The study uses a unique real-life data-set containing information about the Facebook pages of 45 brands for approximately three years combined with Nielsen Advertising Spend data.
This study provides several important contributions to the academic literature. First, it adds a new potential type of driver to the literature regarding consumers’ online brand engagement, which has been neglected in the previous research on the topic: advertising activity in different media (Brettel et al. Citation2015). Second, by investigating novel metrics, such as various types of reach, we can capture the concept of engagement from angles that go beyond the traditional metrics, such as likes and shares. In doing so, we investigate how offline advertising affects not only consumers’ likes, but also, how offline advertising influences engagement metrics associated with the consumption of brand content (i.e. reach). Third, this study contributes to a greater understanding of cross-media effectiveness. Cross-media campaign effectiveness has been a key priority in the advertising field for many years, and is still of major importance (Assael Citation2011; Fulgoni and Lipsman Citation2014; Neijens and Voorveld Citation2015). In the academic literature, there is a focus on synergistic effects of combining multiple media in a campaign (e.g. Chang and Thorson Citation2004; Naik and Peters Citation2009; Voorveld, Neijens, and Smit Citation2011; Voorveld and Valkenburg Citation2015; Vandeberg et al. Citation2015). However, there is a lack of research investigating ‘cross-over’ effects of different media especially between offline and online media. This study contributes to this gap in the literature by focusing on the question how advertising activities in a certain medium (e.g. TV) affect consumers’ engagement on social media across different levels of activeness (i.e. different types of reach as well as likes). This is important since advertisers not only need to know the synergistic effects that may exist between online and offline media, but also the potential effects of advertisements across different media to actively drive engagement and reach on Facebook.
Background
Earlier research on drivers of social media engagement
We define consumers’ brand engagement in line with the work of Hollebeek, Glynn, and Brodie (Citation2014, 154), as ‘a consumer's positively valanced brand-related cognitive, emotional and behavioral activity during or related to focal consumer/brand interactions.’ Earlier research on the drivers of consumers’ online brand engagement on social media can be divided into three streams. The first set of studies investigated the content related to drivers of consumers’ online brand engagement. In this stream, factors such as emotions, usefulness, and storytelling (Yuki Citation2015); vividness and interactivity of brand posts (de Vries, Gensler, and Leeflang Citation2012); positivity and emotionality of news stories (Berger and Milkman Citation2010); or informational and emotional cues of brand posts on Twitter (Araujo, Neijens, and Vliegenthart Citation2015) have been identified as important content-related drivers of consumers’ online brand engagement. The second stream of literature focused on the brand-related drivers of consumers’ online brand engagement. In this body of research, factors, such as brand love (Bergkvist and Bech-Larsen Citation2010), warmth of the brand (Bernritter, Verlegh, and Smit Citation2016), brand identity (Hollenbeck and Kaikati Citation2012), and the symbolic value of the brand (Bernritter Citation2016), have been the important predictors of consumers’ online brand engagement. The third stream of research covered the consumer-related drivers of online brand engagement. Here, consumers’ online brand identification (Stokburger-Sauer, Ratneshwar, and Sen Citation2012), motivations (Muntinga, Moorman, and Smit Citation2011), self-representation (Wallace et al. Citation2014), cultural mindsets (Bernritter et al. Citation2017), and identity maintenance and creation (Hollenbeck and Kaikati Citation2012) have been identified as drivers of consumers’ engagement with brands on social media.
The current research complements these streams of research by investigating the issue from a more macro level, exploring cross-media effects driving consumers’ engagement with brands on social media. Moreover, we investigate three different Facebook engagement metrics: organic reach, viral reach, and likes.
Earlier research on cross-media effects
The field of cross-media effects is dominated by research on cross-media synergy. Cross-media synergy refers to the idea that the combined effect of marketing activities in multiple media exceeds the sum of the individual effects with the ability to create a super-additive effect (Havlena, Cardarelli, and Montigny Citation2007; Naik and Peters Citation2009; Naik and Raman Citation2003; Taylor et al. Citation2013; Vandeberg et al. Citation2015; Kumar, Choi, and Greene Citation2017; Voorveld, Neijens, and Smit Citation2011). Earlier empirical research showed that campaigns combining advertising in multiple media can be more effective in terms of cognitive, affective, and behavioural responses than campaigns using only one medium (e.g. Chang and Thorson Citation2004; Naik and Raman Citation2003; Naik and Peters Citation2009; Romaniuk, Beal, and Uncles Citation2013; Steele et al. Citation2013; Voorveld, Neijens, and Smit Citation2011). While research on synergistic effects of social media with advertising in traditional media is still scarce, a body of research on the subject is gradually developing. There are studies showing that combining social and traditional media in one campaign can be more persuasive (Voorveld and Van Noort Citation2014), or even lead to more sales (Kumar, Choi, and Greene Citation2017; Srinivasan, Rutz, and Pauwels Citation2016).
Two recent papers (Neijens and Voorveld Citation2015; Vandeberg et al. Citation2015) concisely sum up several theoretical explanations for these effects such as encoding variability (Stammerjohan et al. Citation2005; Tavassoli Citation1998; Unnava and Burnkrant Citation1991), repetition-variation (Gibson Citation1996; Schumann, Petty, and Clemons Citation1990; Stammerjohan et al. Citation2005; Yaveroglu and Donthu Citation2008), complementarity (Dijkstra Citation2002), differential attention (MacKenzie Citation1986; Stammerjohan et al. Citation2005; Unnava and Burnkrant Citation1991), forward encoding (Dijkstra 2002; Edell and Keller Citation1989; Voorveld, Neijens, and Smit Citation2011), and multiple source credibility (Chang and Thorson Citation2004; Dijkstra 2002; Laroche et al. Citation2013; Voorveld, Neijens, and Smit Citation2011). A careful study of these explanations shows that they are all based on one underlying explanation of variability; processing information across different types of media causing more variability in the encoding; and retrieval of the information than (repeatedly) processing information within a single medium (Vandeberg et al. Citation2015).
In conclusion, while earlier empirical studies and theoretical reasoning provided evidence for the value derived from integrating social media with another type of medium (especially TV) in advertising campaigns, they did not yet give insight into the cross-over effects, so to what extent does advertising in one medium affects online reach and engagement on Facebook?
How advertising in different media might influence consumers’ online brand engagement
Some studies about related topics can be used to argue that advertising in different media influences consumers’ online brand engagement on Facebook. First, we discuss the empirical results of some of these studies, followed by a discussion of the theoretical arguments that may explain these effects, and that can also be used to argue why we expect that advertising in offline media influences reach and engagement with brand pages on Facebook.
Empirical results
Several studies focused on how consumers search online for products or brands. Some of these studies tracked the effects of advertising expenditures in different media on subsequent online search behaviour. For example, the results of Laroche et al. (Citation2013) showed that exposure to advertising in different media outlets increases the likelihood of individual’ follow-up search and showed that radio was less effective than TV and online advertising. The authors indicated that this could be due to the TV's ‘attention-getting’ nature, which stems from its sound and imagery effects (Blackwell, Miniard, and Engel Citation2001; Chang and Thorson Citation2004; Rossiter and Bellman Citation1999), and due to the immediacy effect of the online medium that allowed searches for the brand to occur much more readily than through traditional channels. In the same vein, a relationship between print advertising (Olbrich and Schultz Citation2014), and TV advertising (Joo et al. Citation2014) and consumers’ online search was shown.
It is important to note here that online search behaviour differs from engagement in several important ways. First, while online search behaviour is private in nature, engagement is generally considered to be public (e.g. Bernritter, Verlegh, and Smit Citation2016). This has important downstream consequences for brands such as increased perceived social risk of online engagement compared to more private types of behaviour (cf. Eisingerich et al. Citation2015). Second, online brand engagement has often been associated with consumers’ identity signalling (Hollenbeck and Kaikati Citation2012). Consumers deliberately engage with brands in social media to communicate their identity to others (Wallace et al. Citation2014), while online search behaviour primarily serves informational purposes. These differences in motivational drivers and audience of the target behaviour are likely to play a role in how offline ads affect online consumer behaviour. Effects of offline ads on consumers’ online brand engagement might thus differ from other online activities and should therefore be investigated.
Also, research on ‘social TV’ can be used to argue that there is a relation between offline advertising and consumers’ online brand engagement on social media (e.g. Krämer et al. Citation2015; Buschow, Schneider, and Ueberheide Citation2014). Studies in this area have shown that as much as 85% of the prime-time-active Twitter users report habitually tweeting about things they see on TV (Nagy and Midha Citation2014). This behaviour is triggered further by the general trend towards media multitasking (i.e. engaging in more than one media activity at a time; Voorveld and Van der Goot Citation2013) and multi-screening (i.e. the simultaneous usage of multiple screens such as a TV, smartphone, laptop, and tablet; Segijn, Voorveld, and Smit Citation2016). These types of media-use facilitate the opportunity for cross-over effects for traditional and social media because a simultaneous use of multiple media allows engagement with a brand on social media to occur in a more immediate way. Extending the results of these previous studies to our study, we expect that paid advertising activities in offline media such as TV, magazines, and radio might influence consumers’ behaviour on Facebook.
Theoretical explanations
Unfortunately, not all earlier empirical studies discuss theoretical explanations for the results. However, some arguments for the existence of cross-over effects can be deducted from the earlier studies, which can be related to the different engagement levels that are distinguished in the current study. To start with, advertising may basically increase consumers’ (perceived) knowledge or awareness of product benefits, or the set of brands they can recall and this may influence their search behaviour (Brucks 1985 in Joo et al. Citation2014; Newman and Staelin Citation1973; Moorman et al. 2004 in Joo et al. Citation2014). Consumers might not only use traditional sources of information to satisfy their need to search for information; however, they might use social media as well. This might be further triggered by advertising explicitly referring to social media. For example, by mentioning web addresses in the advertisement (Kanso and Nelson Citation2004; Olbrich and Schultz Citation2014), by using #hashtags, call to actions, or by referring to special content on social media (Nagy and Midha Citation2014). More importantly, simply by consumers being exposed to the brand, advertising in offline media increases the awareness of the brand to the consumer, which may influence the consumer going to Facebook to access more information or to interact with the brand. This action will likely increase the number of people who are exposed to the brand content in their own timelines or by visiting the brand page (i.e. organic reach).
Moreover, without actively searching for it, it is possible for consumers to be exposed to the content from Facebook brand pages. The reason is that an increase in awareness or knowledge of a brand due to advertising in offline media can trigger some consumers to talk about a brand online making them engage with brand-generated content. Media content in general and advertising in specific influences conversation among people (Bronner and Neijens Citation2006). Particularly, television viewing has always been a partly social activity and the emergence of social media has relocated a portion of these activities to online media (Krämer et al. Citation2015). Media producers and advertisers also encourage this behaviour by using the same techniques as discussed in the previous paragraph (Krämer et al. Citation2015; Spotts, Purvis, and Patnaik Citation2014). Engaging with brand content on Facebook (e.g. by liking, sharing, or commenting) indirectly increases the reach of the brand as more users are exposed to the brand content because their friends on Facebook engaged with the content (i.e. viral reach), even if the user does not actively search for the brand page itself.
An increase in organic and viral reach might subsequently increase the likelihood of a page to receive additional likes in two different ways. First, as organic reach includes consumers that have visited the brand page actively, it can be expected that a share of these consumers may not yet have liked the brand page, and they might do so when visiting it, especially as the brand may be more salient because of advertising exposure. Second, for viral reach, as consumers get exposed to the brand content because friends engaged with it, it can also be expected that some of these consumers will go ahead and like the brand page. If ‘friends’ in the network of a consumer like a brand page, this can be seen as an active endorsement towards the brand, and such an endorsement can persuade the consumer who does not yet like the page to like it (Bernritter, Verlegh, and Smit Citation2016). These endorsements might be very effective for several reasons. For instance, consumers will perceive brand information from other consumers as trustworthy (Bickart and Schindler Citation2001), and therefore, they might be less resistant to persuasion (Bernritter, Verlegh, and Smit Citation2016). Otherwise, they may identify the like of a member of their network as a signal for group-membership and like the brand as well making them to affiliate themselves with this group (Escalas and Bettman Citation2003).
Based on previous studies and theoretical arguments, we can assume that advertising in offline media drives consumers’ brand engagement on Facebook; however, it is still hard to make predictions about the exact differences between the effects of different media. Television is regularly credited a powerful role in cross-media synergy and research on consumers’ online search behaviour (Pfeiffer and Zinnbauer Citation2010; Snyder and Garcia-Garcia Citation2016); however, hardly any explicit comparisons to other media are made. Since media differ from each other on many characteristics (modality, push vs. pull; pacing; interactivity; subjective experience; and trust) (Stewart and Cunningham Citation2017; Bronner and Neijens Citation2006; Dijkstra, Buijtels, and Van Raaij Citation2005; Danaher and Rossiter Citation2011; Voorveld et al. Citation2016), it is expected that they do not influence consumers’ online brand engagement in the same manner. Therefore, we pose the following exploratory research question:
RQ1: What are the differential effects of spending in TV, radio, newspapers, magazines, and out-of-home on the reach of and engagement with Facebook brand pages?
Method
Sample
We use daily observations of user's activity on Facebook pages of 45 Dutch brands as our sample. These brands cover a wide variety of sectors ranging from FMCG's to consumer electronics, and from car brands to retailers, with 27 (60%) of the brands primarily selling non-durable products, 9 (20%) services, 7 (16%) durable products, and 2 (4%) were large retailers. These 45 brands, all active in the Dutch market, were present in a larger data-set obtained in collaboration with a media agency responsible for managing the Facebook advertisement and social activities of these brands. To be in this sample, the brands had to (a) focus primarily on the consumer market, (b) have its advertisement activities reported in Nielsen, and (c) be active on Facebook during the period of the study. The Nielsen and Facebook page data were reconciled at page level (i.e. the activities of a brand, or sub-brand in Nielsen were associated with a specific Facebook page). When more than one Facebook page was available for the same brand/sub-brand reported by Nielsen, only the largest brand page was considered. It is important to note that data from multiple brands are included because this might help to control potential biases from brand-specific effects (Klapdor et al. Citation2015). The sample includes activity on the brand pages between 2012 and 2015, covering a period of approximately three years (Mdays per brand page = 800.18, SD = 276.03).
Independent variables
All data are provided through a cooperation with a media agency, and collected directly from Nielsen (advertising data) or Facebook Insights (reach and likes). The data include the measures described below:
Advertising spend per medium
Since expenditures are the acceptable measures for advertising directed to consumers (Laroche et al. Citation2013), Nielsen advertising data were used for the same period as Facebook Insights data. The main independent variables of the study, offline spending, were provided on a daily basis, and separately for each of the following media: magazines (M = 1415.68, SD = 9073.63), radio (M = 3431.65, SD = 11,800.87), out-of-home (M = 2234.36, SD = 11761.66), newspapers (M = 1503.56, SD = 13089.37), trade publications (M = 31.83, SD = 539.04), and television (M = 16582.69, SD = 32801.48). We also included Internet advertising (M = 930.64, SD = 27,692.24) spend from each company as measured by Nielsen as a control variable. This measure includes the spend with display advertising in the largest Dutch websites for the brands in the sample.
Paid reach
Following Facebook's (Citation2017) definitions, paid reach was defined as the total number of unique users who were reached by the page's advertising on Facebook. It is important to note that paid reach was considered an independent variable for this study, as this type of reach was a direct consequence of online advertising by the (brand) pages on Facebook; thus, it could serve as a proxy for the amount of online advertising by the brand on Facebook.
Dependent variables
Organic reach
Organic reach is defined as the total number of unique users who see any content of a page ‘through unpaid distribution,’ that is excluding users who see content from the page based on the advert from the brand (Facebook Citation2017; Mby page/day = 5654.16, SD = 11,395.86).
Viral reach
Viral reach is defined as the total number of unique users who see any specific content of the page because one or more of their friends engage with the content before; so the number of people who have seen a page post in a report of a friend (2017; Mby page/day = 3191.46, SD = 11,699.82). Although Facebook recently stopped to report viral reach, we believe it is still a relevant measure to include in our research because of its practical importance for advertisers.
Page likes
Page likes are defined as the total number of likes that the brand page has in a day and is collected from Facebook daily. Unlike viral reach, it is important to note that page likes are a cumulative metric, i.e. the number of likes of the previous day is a starting point for the following dayFootnote1 (Mby page/day = 70,968.34, SD = 66,286.65).
In this study, these three measures were used as dependent variables: for each of the three dependent variables, we created three models to explore cross-over effects by including the following independent variables: total advertising spend per medium and paid reach. Because our dependent variables (organic reach, viral reach, and page likes) are related, we controlled for the other dependent variables when conducting the analysis for one of the dependent variables. We also controlled for spending on online advertising.
Controlling for Facebook changes
Facebook itself is not a static platform, and via its algorithms, it often changes the priority it gives to the content shown to its users, and more importantly, under which conditions users can be reached or find content from others (Bucher Citation2012). We expect these changes to influence the effect advertising has on users’ engagement on Facebook. We control the year of the measurement, which roughly matched relevant changes on Facebook's algorithms for brand pages reach according to practitioner's blogs and reports at an aggregate level (e.g. Peterson Citation2014).
Analytical strategy
Our data-set has a nested structure with daily observations nested in brands. This panel structure of the data comes with several statistical challenges. First, we expect the presence of strong autocorrelation, i.e. the value of the dependent variable to be strongly affected by the previous values. The second challenge is heterogeneity (i.e. structural differences in the values of the dependent variable across brands that cannot be captured by the independent variables, which is included in our model) that is likely to be present. Therefore, we rely on pooled-time series fixed-effects models while including a lagged dependent variable. The independent variables are expected to have an immediate impact on the dependent variables.Footnote2 Because the levels of the dependent variable can differ across brands in ways that cannot be predicted by the independent variables in our model (unit-level heterogeneity), we ran fixed-effects models with a unique intercept for every brand. By including fixed effects, all inter-unit variances (i.e. variance across different brands/industries/Facebook pages) are removed and the modelling focuses on intra-unit, over-time variance (Wilson and Butler Citation2007). We think it is more valid to use a fixed-effects model than to include a control variable for variables like type of brand, brand size, etc., because categorizing the 45 brands into certain categories is always rather arbitrary and brands differ on many characteristics at the same time. Mathematically, such a model including a single independent variable x is written as follows:(1)
(1) and can be considered a variant of an infinite distributed lag model and specifically a Koyck lag. In this situation, various independent variables have a direct impact on the dependent variable as an indirect, delayed one through the effect of the previous value of yit (De Boef and Keele Citation2008). The total long-term effect of x on y (long-term multiplier) is similar to
(2)
(2) We log-transformed all variables (except for the algorithm changes) given their high skewness levels. We conducted the Fisher unit-root test to assess whether our dependent variables are stationary. Results suggest that series are stationary (chi-squared (90) for organic reach is 2805.73, p < .001; for viral reach 2371.58, p < .001; and 868.22, p < .001 for page likes – in all instances rejecting the null hypothesis of non-stationarity) and need not to be differenced before conducting the analysis.
Results
The trends of reach and likes
The results showed a declining trend of reach of brand pages over the years that were included in our sample. When compared to the period prior to the first large algorithm change by Facebook by the end of 2013, subsequent periods between 2014 and 2015 showed a statistically significant drop in organic and viral reach. This declining trend is in line with Facebook changes to its algorithm that determines which content is shown to the user. In contrast, there is an increase in likes over the years.
Cross-over effects of offline advertising on Facebook reach and engagement
Results in show that advertising spend in different media differentially affects different forms of reach and engagement. We discuss the three metrics below.
Table 1. Time-series analysis for the influence of offline media on organic reach, viral reach, and page likes.
Organic reach
Organic reach is influenced by advertising spend in different offline media. Advertising spend on TV, newspapers, and out-of-home leads to a higher organic reach on Facebook. Thus, if more money is spent on advertising in these media, the total number of unique users who view any content of the page either in their time lines, or by visiting the brand page, is higher. Remarkably, there is a negative relationship for magazines, if more money is spent on advertising, organic reach on Facebook decreases. Paid reach on Facebook and the number of brand page likes were the strongest driver of organic reach on Facebook; thus, the higher the amount of money spent on advertising on Facebook, the higher the number of likes a certain brand page, the higher the organic reach of a brand page on Facebook. The spend on Internet advertising, included as a control variable in this study, has a negative relationship with organic reach, suggesting that display advertisements on other websites (outside of Facebook) do not tend to generate higher activity within Facebook, or even a lower activity, unlike paid reach on Facebook itself which has a positive influence.
Given the lack of empirical research in this topic, we decided to use straightforward models investigating linear effects. However, to begin illustrating the potential for long-term effects, we have added figures that show the immediate and, through the lag-dependent variable in our model, also the long-term effects. shows the change in log-transformed organic reach due to a one unit increase in the log-transformed expenditures at time 0 (only significant results are shown). The effect at t = 0 equals the regression coefficient in the equation, the subsequent days illustrate the cumulative effect, due to indirect effects via the autoregressive component in the models, more specifically the lagged dependent variable.
Viral reach
While organic reach is influenced by advertising spend in several different offline media, viral reach is only influenced by advertising on TV. Yet, it is again clear that the number of page likes and the amount of advertising on Facebook itself are the strongest drivers for the total number of unique users who view the content of the page because one or more of their friends engage with the content. Just as for organic reach, Internet advertising in general has a negative relationship with viral reach.
Again, shows the change in log-transformed viral reach due to a one unit increase in the log-transformed expenditures for TV at time 0 (TV is the only medium with a significant impact). The effect at t = 0 equals the regression coefficient in the equation; the subsequent days illustrate the cumulative indirect effects via the lagged dependent variable.
Page likes
shows that only predictors directly related to Facebook, i.e. the total number of unique users who have been reached via advertising on Facebook (paid reach), organic reach, and viral research are significantly related to the number of page likes. While advertising in offline media could influence organic reach and to a lesser extent viral reach, the number of page likes was only directly influenced by advertising on Facebook itself.
Conclusion
This study is the first to explore the relationship between advertising spend in different media (TV, radio, newspapers, magazines, trade publications, and out-of-home) and reach of and engagement with brand pages on Facebook. It can be concluded that advertising spends in different offline media influence different forms of consumers’ online brand engagement in different ways. A unique pattern was detected for the different engagement metrics such as organic reach, viral reach, and likes.
When it comes to organic reach, different media (TV, newspapers, and out-of-home) have a positive influence, thus, providing evidence to the notion that cross-over effects are relevant for influencing the number of unique users who view any content of the page either in their own timelines, or by visiting the brand page. Magazines seem to have a negative effect on organic reach. Furthermore, advertising on Facebook turned out to be the driver of organic reach on Facebook. When looking at the coefficients, it can be concluded that effects are generally small. Whereas, cross-over effects play a critical role in influencing organic reach on Facebook, advertising on Facebook itself seems to be the strongest driver of organic reach of Facebook brand pages.
For viral reach, cross-over effects seem to be less relevant, and TV is the only offline medium that is a driver of viral reach, next to advertising on Facebook (paid reach). For likes, no cross-over effects could be detected and only advertising on Facebook could influence the number of likes a brand page received from consumers. However, organic and viral reach are also important drivers of the number of likes, so there is a probability of an indirect influence of advertising spend on TV, newspapers, and out-of-home media.
Analysis of secondary data typically does not allow discerning the underlying processes that can explain the different results found for the different indicators of consumers’ online brand engagement; however, we can share some possible explanations based on earlier literature. Television turned out to play a prominent role for both organic and viral reach. This is in line with the powerful role of TV in driving cross-media synergy and in line with earlier research that shows that TV is especially effective in terms of upper-funnel metrics (Pfeifer and Zinnbauer Citation2010; Briggs, Krishnan, and Borin Citation2005). This result might be explained by the characteristics of the medium. Television is a typical broadcasting medium, an internally paced delivery medium: consumers have a low level of control over the speed and timing of information transfer (Dijkstra, Buijtels, and Van Raaij Citation2005). Moreover, TV is often applauded for its attention-getting capabilities due to its sound and imagery effects (Blackwell, Miniard, and Engel Citation2001; Chang and Thorson Citation2004; Rossiter and Bellman Citation1999). These characteristics make TV a medium that can be particularly useful for pushing messages to consumers, and it is effective in building awareness, which is important in early stages of the consumer journey. Television is also experienced as a medium that gives people a subject of conversations (Bronner and Neijens Citation2006). When people talk about brands advertised on TV, this further boosts organic and viral reach as being discussed previously.
Remarkably, magazines had a negative impact on organic reach (not on viral reach and likes). Again, this result might be explained by the characteristics of the medium. Magazines are typically described as a lean-back medium that people read in a rather passive way. People describe reading a magazine as giving them enjoyment, making them forget everything for a moment; a treat, a reward for doing other things, an escape, and suitable for filling an empty moment (Malthouse, Calder, and Tamhane Citation2007; Bronner and Neijens Citation2006). The nature of magazines may make it less likely that people go to Facebook to visit a brand page after they saw an ad for a certain brand in a magazine. In contrast, the way people experience newspapers makes this behaviour much more likely. People experience newspapers as informative, and provide them with subjects for conversation (BronnerandNeijens Citation2006). This might explain the positive impact of newspaper expenditures but negative impact of magazine expenditures on organic reach of Facebook brand pages.
Across all types of engagement investigated in the current study, advertising on Facebook has a positive impact on consumers’ online brand engagement on Facebook. Moreover, when comparing the coefficients of advertising on Facebook with advertising spend in offline media, it can be concluded that the effects of advertising on Facebook are more consistent as they encompass all types of engagement behaviour studied. Earlier research comparing effects of online and offline advertising on online search behaviour already suggested that the immediacy effects of the Internet allow searches for the brand to occur much more readily than through offline media (Laroche et al. Citation2013), which might also partly explain the differences in the current study. More importantly, online advertising on Facebook might not only drive engagement with brands on Facebook due to its immediacy, but also due to its proximity. As advertising on Facebook and engagement with brands take place on the same device and even the same platform, this might induce less switching costs for consumers (Yeykelis, Cummings, and Reeves Citation2014) than switching between different devices or media. Therefore, it is much more convenient for consumers to engage with brands on Facebook if they are exposed to the ads of that brand on Facebook than in other media such as TV and radio.
Whereas Facebook advertising has a strong positive impact on all types of engagement with Facebook, the pattern found for the control variable, online display advertising on websites other than Facebook, was much more counterintuitive. Results showed that the spending on online display advertising (outside Facebook) exerts a negative significant relationship on organic and viral reach. A potential explanationFootnote3 may be that visits to the brand's actual website may be the missing link between offline/online ad spend and social media engagement metrics. It would be possible consumers may forgo visiting a brand's social media pages and directly search and visit a brand's actual website, once they become exposed to any form of that brand's advertising. This is even more likely the case with online display advertising since the majority of banner ads link consumers to the brand website rather than its social media site because brand websites are often where the main online sales takes place, rather than social media brand pages.
Results also showed that organic reach is more strongly affected by advertising in offline media than viral reach. The reason might be that organic reach is independent of consumers’ personal network and the engagement behaviour of their peers. It can be driven by various exogenous factors such as previous knowledge of a brand, personal interest, or exposure to advertising in other media. Contrarily, viral reach is dependent on the behaviour of other peers and how they interact with branded content. Here, different social motivations, which can often be found in the domain of self-representation and impression management (e.g. Wallace et al. Citation2014), might explain a huge portion of the variance of viral reach, while these social factors should play no role in organic reach.
The absence of significant cross-over effects from offline media to likes could possibly be explained by the nature of this metric. Liking a brand page that deals with consumers’ explicit affective responses to online messages is in ways that are visible to others (Alhabash et al. Citation2015). Moreover, it has been shown that consumers have the tendency to like brands on Facebook to signal their identity (e.g. Hollenbeck and Kaikati Citation2012; Wallace et al. Citation2014). However, it could impose a higher risk to consumers than merely visiting a brand page or viewing a brand post in one owns timeline (cf. Eisingerich, Auh, and Merlo Citation2014). Thus, liking brands require an active, rather risky behaviour of consumers, while organic and viral reach is more about passively consuming a brands’ content without any risk. Another explanation for the absence of significant cross-over effects from offline media on likes might be that whereas reach can be seen as a metric representing an earlier stage of the purchase funnel or consumer journey, liking can be seen as a more attitudinal measure (Srinivasan, Rutz, and Pauwels 2016; Spotts, Purvis, and Patnaik Citation2014) and as an indicator of later stages of the purchase funnel or consumer journey. It can be argued that advertising spends in a particular medium might have a rather straightforward influence on early stages of the consumer journey, actually liking a brand page might be more dependent on other factors. Some of these factors are under the control of the advertiser, such as the actual content, however, probably go beyond the amount of money spent on advertising in a typical offline medium (ARF Citation2003).
Limitations and future research
Although the study has a unique approach by using a real-life data-set, it also has some limitations. The first limitation is that we studied consumers’ online engagement from a macro level, by using aggregate ad spend on medium level (e.g. magazines, TV), rather than on medium vehicle level (specific magazines or TV programmes). However, we miss data on a more micro level, for example, about the specific campaigns during those periods and on the exact ad characteristics Therefore, we do not know whether the brands are involved in any other type of activity besides advertising, such as PR events, during these periods which could influence the results, and whether ads with specific characteristics (e.g. an explicit referral to the brands Facebook page), would have a stronger impact on consumers’ online brand engagement on Facebook. Future research could try to answer these questions with additional real-life data, or with experimental studies in which ad characteristics are manipulated.
Second, as discussed earlier, analyses of secondary data typically do not allow discerning the underlying processes that can explain the different results. The nature of our data does not allow us to ask questions to consumers about why they chose to visit a Facebook page, and why they liked a brand on Facebook. Surveys among consumers or experimental studies might be able to give more insight into the underlying mechanisms that can explain the results found in the current study.
The third limitation is that the study was based on time-series analyses and was not based on a single-source panel in which advertising exposure and social media behaviour were observed within a single consumer.
We do believe that the findings are generalizable to other brands and countries, because our data covered a wide variety of brands, ranging from FMCGs to consumer electronics, from car brands to retailers, and both national as international brands. We believe this might help to control for potential biases from brand-specific effects (Klapdor et al. Citation2015).
It is unsure whether the results or the current study are generalizable to other social media platforms. Although social media are often regarded as an umbrella concept, many variations and types of social media exist. Facebook (a social network), Snapchat (an instant photo messaging application), Instagram (a photo sharing application), Twitter (a microblogging application), and Pinterest (a ‘catalogue of ideas’ or photo sharing website) all have different functionalities and other characteristics (Voorveld et al. Citation2018). They differ for example with regards to nature of the connection: (profile-based versus content-based), level of customization of messages (the degree to which a service is customized to satisfy an individual's particular preferences) (Zhu and Chen Citation2015), modality (Waterloo et alCitation. forthcoming), private vs. public access to content (Lapinski and Rimal Citation2005), following mechanism (reciprocal vs. non-reciprocal, Davenport et al. Citation2014), privacy parameters, type of connections (friends/colleagues), and longevity of content accessibility. Recent research also showed that these different functionalities and characteristics translate into different consumer experiences. While Facebook, Instagram, and Snapchat are experienced as suitable for social interaction; YouTube and Pinterest are not. While Facebook and Instagram are used to fill an empty moment, others such as Snapchat, Pinterest, and Twitter are used to a much lesser extent as pastime. Of course, there are also similarities. Except for YouTube and Pinterest, all social media platforms are experienced as a way to remain up to date (Voorveld et al. Citation2018).
The current study focuses on Facebook because advertisers generally spend the largest part of their social media budgets on Facebook (Kollewe Citation2017), and consumers most often encounter advertising on Facebook in comparison to other platforms (Voorveld et al. Citation2018). Therefore, Facebook is a leading platform with respect to social media advertising and consumers’ online brand engagement. Future research should test whether the results of the current study are comparable when other social media platforms than Facebook are studied.
Given the lack of empirical research in this topic, this study uses straightforward models investigating linear effects. Future research could also test more specific models, taking into account, for example, content of the advertisements and specific campaign characteristics, or could include additional variables like brand website visits, online sales, and offline sales to give a more complete picture of the purchase funnel. Future research could also try to formulate specific hypotheses of nonlinear effects, but at this moment, we could not find enough theoretical reasons for nonlinearity.
Implications for practitioners
For practitioners, the study has important implications. The social media strategy is often still a separated and isolated chapter in the media strategy with its own objectives and KPIs. The results of the study plead for a more holistic approach in which the role and added value of each medium are defined and strategically integrated into the media plan. This would facilitate the use of offline media as a driver for the social media activities and could ultimately make these more efficient and effective.
When making decisions about which offline media to incorporate in a media plan, it is important to be aware that the influence of offline media on consumers’ online brand engagement is heterogeneous across the consumer journey or purchase funnel. The model does support a direct link between offline media expenditures and organic reach, and to a lesser extent viral reach of brand pages on Facebook. However, a direct link between offline media expenditures and likes could not be established. This implies that offline media might be most suitable to drive consumers into the consumer journey or purchase funnel, by driving them to search for brands on social media. It seems less likely that offline media can directly drive consumers to later stages of the purchase funnel, such as the stage in which consumers evaluate brands. Thus, whether advertising in offline media should be used a driver for consumers’ brand engagement on Facebook heavily depends on the specific campaign objectives.
If advertisers decide to use advertising in offline media to try to influence engagement with brands on Facebook, this study implies that they could best increase their spendings on television, newspapers, and out-of-home media. Furthermore, also spending a part of their budget on advertising on Facebook itself seems a good idea as paid advertising on Facebook has a direct influence across all types of reach and engagement. Given the existence of cross-over effects between offline and online media, the findings also emphasize the importance of consistency of message content across different advertising media (Voorveldand Valkenburg 2015). More specifically, a good coordination of the content on TV, in newspapers, out-of-home (the significant drivers of organic and viral reach) and Facebook might be of crucial importance to make optimal use of the advertising budget. This might not be surprising news for most advertisers; however, it is not evident that it is been practised.
Acknowledgment
The authors are grateful to DAN DNA, a data-driven agency powered by the Dentsu Aegis Network, for making the data available.
Disclosure statement
No potential conflict of interest was reported by the authors.
Additional information
Notes on contributors
Hilde A. M. Voorveld
Hilde A.M. Voorveld (PhD, University of Amsterdam) is an associate professor in Marketing Communication at the Amsterdam School of Communication Research ASCoR, Department of Communication Science, University of Amsterdam, the Netherlands. Her research interests include cross-media advertising, media multitasking, and new media uses and effects.
Theo Araujo
Theo Araujo (PhD, University of Amsterdam) is an assistant professor at the Amsterdam School of Communication Research (ASCoR), Department of Communication Science, University of Amsterdam, the Netherlands. His research interests include information diffusion on social media, the role of artificial intelligence and virtual agents online, and emerging methods in computational social sciences.
Stefan F. Bernritter
Stefan F. Bernritter (PhD, University of Amsterdam) is an assistant professor in Marketing Communication at the Amsterdam School of Communication Research (ASCoR), Department of Communication Science, University of Amsterdam, the Netherlands. His research interests lie in consumers’ brand-related social media use, consumer identity, and mobile marketing.
Edwin Rietberg
Edwin Rietberg (Drs. VU University Amsterdam) is a key account director as DAN DNA, a data-driven agency powered by the Dentsu Aegis Network. He is also chief editor of the Media Facts Booklet at AEGIS Media.
Rens Vliegenthart
Rens Vliegenthart is a full professor for Media and Society at the Amsterdam School of Communication Research (ASCoR), Department of Communication Science, University of Amsterdam, the Netherlands. His research interests include media–politics relations, media coverage of social movements and businesses, election campaigns, European integration, soccer hooliganism, and time-series analysis.
Notes
1. Whenever the data were not available for a given day because of missing values in the database, we averaged the previous and the following day. When either the previous or the following day was also missing, we set the day as a missing value.
2. The literature did not give any indications for the best time lag to be used in our models. We have tested models that also include additional lags for each of the independent variables that showed results largely aligning with the simpler, yet more robust models reported in the paper.
3. We would like to thank reviewer 3 for this excellent explanation.
4. Data on magazines and trade publications were aggregated on a weekly basis, because magazines are usually published on a weekly, rather than daily basis and can be read at any time after the date of publication (Olbrich and Schultz Citation2014). We also ran our model without the seven-day average lag to see whether the results changed, but the results seem to be robust.
References
- Alhabash, S., A.R. McAlister, C. Lou, and A. Hagerstrom. 2015. From clicks to behaviors: The mediating effect of intentions to like, share, and comment on the relationship between message evaluations and offline behavioral intentions. Journal of Interactive Advertising 15, no. 2: 82–96.
- Araujo, T., P.C. Neijens, and R. Vliegenthart. 2015. What motivates consumers to re-Tweet brand content? The impact of information, emotion, and traceability on pass-along behavior. Journal of Advertising Research 55, no. 3: 284–95.
- ARF. 2003. Making better media decisions. http://s3.amazonaws.com/thearf-org-aux-assets/downloads/research/2003_ARF_media_model.pdf ( accessed July 19, 2017).
- Assael, H. 2011. From silos to synergy. Journal of Advertising Research 51, no. 1: 42–58.
- Berger, J., and K. Milkman. 2010. Social transmission, emotion, and the virality of online content. Wharton Research Paper. http://opim.wharton.upenn.edu/∼kmilkman/Virality.pdf ( accessed July 19, 2017).
- Bergkvist, L., and T. Bech-Larsen. 2010. Two studies of consequences and actionable antecedents of brand love. Journal of Brand Management 17, no. 7: 504–18.
- Bernritter, S.F. 2016. Signaling warmth: How brand warmth and symbolism affect consumers’ online brand endorsements. In Vol. VI, Advances in advertising research, ed. P.W.J. Verlegh, H.A.M. Voorveld, and M. Eisend, 1–10. Wiesbaden: Springer Fachmedien.
- Bernritter, S.F., A.C. Loermans, P.W.J. Verlegh, and E.G. Smit. 2017. ‘We’ are more likely to endorse than ‘I’: The effects of self-construal and brand symbolism on consumers’ online brand endorsements. International Journal of Advertising 36, no. 1: 107–20.
- Bernritter, S.F., P.W.J. Verlegh, and E.G. Smit. 2016. Why nonprofits are easier to endorse on social media: The roles of warmth and brand symbolism. Journal of Interactive Marketing 33 (February): 27–42.
- Bickart, B., and R.M. Schindler. 2001. Internet forums as influential sources of consumer information. Journal of Interactive Marketing 15, no. 3: 31–40.
- Blackwell, R.D., P.W. Miniard, and J.F. Engel. 2001. Consumer behaviour. 9th ed. Mason, OH: South-Western Thomas Learning.
- Brettel, M., J.C. Reich, J.M. Gavilanes, and T.C. Flatten. 2015. What drives advertising success on Facebook? An advertising-effectiveness model. Journal of Advertising Research 55, no. 2: 162–75.
- Briggs, R., R. Krishnan, and N. Borin. 2005. Integrated multichannel communication strategies: Evaluating the return on marketing objectives – the case of the 2004 Ford F-150 launch. Journal of Interactive Marketing 19, no. 3: 81–90.
- Bronner, F., and P.C. Neijens. 2006. Audience experiences of media context and embedded advertising – a comparison of eight media. International Journal of Market Research 48, no. 1: 81–100.
- Bucher, T. 2012. Want to be on the top? Algorithmic power and the threat of invisibility on Facebook. New Media and Society 14, no. 7: 1164–80.
- Buschow, C., B. Schneider, and S. Ueberheide. 2014. Tweeting television: Exploring communication activities on Twitter while watching TV. Communications: The European Journal of Communication Research 39, no. 2: 129–49.
- Chang, Y., and E. Thorson. 2004. Television and web advertising synergies. Journal of Advertising 33, no. 2: 75–84.
- Chang, Y.-T., H. Yu, and H.P. Lu. 2015. Persuasive messages, popularity cohesion, and message diffusion in social media marketing. Journal of Business Research 68, no. 4: 777–82.
- Danaher, P.J., and J.R. Rossiter. 2011. Comparing perceptions of marketing communication channels. European Journal of Marketing 45, no. 1/2: 6–42.
- Davenport, S.W., S.M. Bergman, J.Z. Bergman, and M.E. Fearrington. 2014. Twitter versus Facebook: Exploring the role of narcissism in the motives and usage of different social media platforms. Computers in Human Behavior 32, no.1: 212–20.
- De Boef, S., and L. Keele. 2008. Taking time seriously. American Journal of Political Science 52, no. 1: 184–200.
- De Vries, L., S. Gensler, and P.S.H. Leeflang. 2012. Popularity of brand posts on brand fan pages: An investigation of the effects of social media marketing. Journal of Interactive Marketing 26, no. 2: 83–91.
- Dijkstra, M. 2002. An experimental investigation of synergy effects in multiple-media advertising campaigns. PhD diss., University of Tilburg, The Netherlands.
- Dijkstra, M., H.E. Buijtels, and W.F. van Raaij. 2005. Separate and joint effects of medium type on consumer responses: A comparison of television, print, and the internet. Journal of Business Research 58, no. 3: 377–86.
- Edell, J.A., and K.L. Keller. 1989. The information processing of coordinated media campaigns. Journal of Marketing Research 26: 149–63.
- Eisingerich, A.B., S. Auh, and O. Merlo. 2014. Acta non verba? The role of customer participation and word of mouth in the relationship between service firms’ customer satisfaction and sales performance. Journal of Service Research 17, no. 1: 40–53.
- Eisingerich, A.B., H.H. Chun, Y. Liu, H.M. Jia, and S.J. Bell. 2015. Why recommend a brand face-to-face but not on Facebook? How word-of-mouth on online social sites differs from traditional word-of-mouth. Journal of Consumer Psychology 25, no. 1: 120–8.
- Escalas, J.E., and J.R. Bettman. 2003. You are what they eat: The influence of reference groups on consumers’ connections to brands. Journal of Consumer Psychology 13, no. 3: 339–48.
- Facebook. 2017. Insights export. https://www.facebook.com/help/285625061456389?helpref=uf_permalink ( accessed July 19, 2017).
- Fulgoni, G., and A. Lipsman. 2014. Numbers, please: Digital game changers: How social media will help usher in the era of mobile and multi-platform campaign-effectiveness measurement. Journal of Advertising Research 54, no. 1: 11–6.
- Gibson, L.D. 1996. What can one TV exposure do? Journal of Advertising Research 36, no. 2: 9–18.
- Havlena, W., R. Cardarelli, and M. de Montigny. 2007. Quantifying the isolated and synergistic effects of exposure frequency for TV, print, and internet advertising. Journal of Advertising Research 47, no. 3: 215–21.
- Hollenbeck, C.R., and A.M. Kaikati. 2012. Consumers’ use of brands to reflect their actual and ideal selves on Facebook. International Journal of Research in Marketing 29, no. 4: 395–405.
- Hollebeek, L.D., M.S. Glynn, and R.J. Brodie. 2014. Consumer brand engagement in social media: Conceptualization, scale development and validation. Journal of Interactive Marketing 28, no. 2: 149–65.
- Joo, M., K.C. Wilbur, B. Cowgill, and Y. Zhu. 2014. Television advertising and online search. Management Science 60, no. 1: 56–73.
- Kanso, A.M., and R.A. Nelson. 2004. Internet and magazine advertising: Integrated partnerships or not? Journal of Advertising Research 44, no. 4: 317–26.
- Klapdor, S., E. Anderl, J.H. Schumann, and F. von Wangenheim. 2015. How to use multichannel behavior to predict online conversions. Journal of Advertising Research 55, no. 4: 433–42.
- Kollewe, J. 2017. Google and Facebook bring in one-fifth of global ad revenue. The Guardian. https://www.theguardian.com/media/2017/may/02/google-and-facebook-bring-in-one-fifth-of-global-ad-revenue ( accessed December 12, 2017).
- Krämer, N.C., S. Winter, B. Benninghoff, and C. Gallus. 2015. How “social” is Social TV? The influence of social motives and expected outcomes on the usage of Social TV applications. Computers in Human Behavior 51: 255–62.
- Kumar, V., J.-W.B. Choi, and M. Greene. 2017. Synergistic effects of social media and traditional marketing on brand sales: Capturing the time-varying effects. Journal of the Academy of Marketing Science 45, no. 2: 268–88.
- Lapinski M.K. and R.N. Rimal. 2005. An explication of social norms. Communication Theory 15, no. 2: 127–47.
- Laroche, M., I. Kiani, N. Economakis, and M.-O. Richard. 2013. Effects of multi-channel marketing on consumers' online search behavior. Journal of Advertising Research 53, no. 4: 431–43.
- MacKenzie, S.B. 1986. The role of attention in mediating the effect of advertising on attribute importance. Journal of Consumer Research 13: 174–95.
- Malthouse, E.C., B.J. Calder, and A. Tamhane. 2007. The effects of media context experiences on advertising effectiveness. Journal of Advertising 36, no. 3: 7–18.
- Muntinga, D.G., M. Moorman, and E.G. Smit. 2011. Introducing COBRAs: Exploring motivations for brand-related social media use. International Journal of Advertising 30, no. 1: 13–46.
- Nagy, J., and A. Midha. 2014. The value of earned audiences: How social interactions amplify TV impact: What programmers and advertisers can gain from earned social impressions. Journal of Advertising Research 54, no. 4: 448–53.
- Naik, P.A., and K. Peters. 2009. A hierarchical marketing communications model of online and offline media synergies. Journal of Interactive Marketing 23, no. 4: 288–99.
- Naik, P.A., and K. Raman. 2003. Understanding the impact of synergy in multimedia communications. Journal of Marketing Research 40, no. 4: 375–88.
- Neijens, P.C., and H.A.M. Voorveld. 2015. Cross-platform advertising: Current practices and issues for the future. Journal of Advertising Research 55, no. 4: 362–67.
- Newman J.W, and R. Staelin. 1973. Information sources for durable goods. Journal of Advertising Research 13, no. 2:19–29.
- Olbrich, R., and C.D. Schultz. 2014. Multichannel advertising: Does print advertising affect search engine advertising? European Journal of Marketing 48, no. 9/10: 1731–56.
- Peterson, P. 2014. Advertisers shake off Facebook's latest reach cuts. Adage. http://adage.com/article/digital/advertisers-shake-facebook-s-latest-reach-cuts/295913 ( accessed July 19, 2017).
- Pfeiffer, M., and M. Zinnbauer. 2010. Can old media enhance new media? Journal of Advertising Research 50, no. 1: 42–9.
- Romaniuk, J., V. Beal, and M. Uncles. 2013. Achieving reach in a multi-media environment: How a marketer's first step provides the direction for the second. Journal of Advertising Research 53, no. 2:221–30.
- Rossiter, J.R., and S. Bellman. 1999. A proposed model for explaining and measuring web ad effectiveness. Journal of Current Issues & Research in Advertising 21, no. 1: 13–31.
- Scheinbaum, A.C. 2016. Digital engagement: Opportunities and risks for sponsors. Journal of Advertising Research 56, no. 4: 341–45.
- Schumann, D.W., R.E. Petty, and D.S. Clemons. 1990. Predicting the effectiveness of different strategies of advertising variation: A test of the repetition-variation hypotheses. Journal of Consumer Research 17: 192–202.
- Segijn, C.M., H.A.M. Voorveld, and E.G. Smit. 2016. The underlying mechanisms of multiscreening effects. Journal of Advertising 45, no. 4: 391–402.
- Snyder, J., and M. Garcia-Garcia. 2016. Advertising across platforms: Conditions for multimedia campaigns: A method for determining optimal media investment and creative strategies across platforms. Journal of Advertising Research 56, no. 4:352–67.
- Spotts, H.E., S.C. Purvis, and S. Patnaik. 2014. How digital conversations reinforce Super Bowl advertising. Journal of Advertising Research 54, no. 4: 454–68.
- Srinivasan, S., O.J. Rutz, and K. Pauwels. 2016. Paths to and off purchase: Quantifying the impact of traditional marketing and online consumer activity. Journal of the Academy of Marketing Science 44, no. 4: 440–53.
- Stammerjohan, C., C.M. Wood, Y. Chang, and E. Thorson. 2005. An empirical investigation of the interaction between publicity, advertising, and previous brand attitudes and knowledge. Journal of Advertising 34, no. 4: 55–67.
- Steele, A., D. Jacobs, C. Siefert, R. Rule, B. Levine, and C.D. Marci. 2013. Leveraging synergy and emotion in a multi-platform world. Journal of Advertising Research 53, no. 4: 417–30.
- Stewart, K., and I. Cunningham. 2017. Examining consumers' multiplatform usage and its contribution to their trust in advertising. Journal of Advertising Research 57, no. 1: JAR–2017.
- Stokburger-Sauer, N., S. Ratneshwar, and S. Sen. 2012. Drivers of consumer–brand identification. International Journal of Research in Marketing 29, no. 4: 406–18.
- Tavassoli, N.T. 1998. Language in multimedia: Interaction of spoken and written information. Journal of Consumer Research 25, no. 1: 26–37.
- Taylor, J., R. Kennedy, C. McDonald, L. Larguinat, Y.E. Ouarzazi, and N. Haddad. 2013. Is the multi-platform whole more powerful than its separate parts? Journal of Advertising Research 53, no. 2: 200–11.
- Unnava, H.R., and R.E. Burnkrant. 1991. Effects of repeating varied ad executions or brand name memory. Journal of Marketing Research 28: 406–16.
- Vandeberg, L., J.M.J. Murre, H.A.M. Voorveld, and E.G. Smit. 2015. Dissociating explicit and implicit effects of cross-media advertising. International Journal of Advertising 34, no. 5: 744–64.
- Voorveld, H.A.M., P.C. Neijens, and E.G. Smit. 2011. Opening the black box: Understanding cross-media effects. Journal of Marketing Communications 17, no. 2: 69–85.
- Voorveld, H.A.M, and S.M.F. Valkenburg. 2015. The fit factor: The role of fit between ads in understanding cross-media synergy. Journal of Advertising 44, no. 3: 185–95.
- Voorveld, H.A.M, and M. van der Goot. 2013. Age differences in media multitasking: A diary study. Journal of Broadcasting & Electronic Media 57, no. 3: 392–408.
- Voorveld, H.A.M., and G. van Noort. 2014. Social media in advertising campaigns: Examining the effects on perceived persuasive intent, campaign and brand responses. Journal of Creative Communications 9, no. 3: 253–68.
- Voorveld, H.A.M., E.G. Smit, P.C. Neijens, and A.E. Bronner. 2016. Consumers' cross-channel use in online and offline purchases. Journal of Advertising Research 56, no. 4:385–400.
- Voorveld, H.A.M., G. van Noort, D.G. Muntinga, and F. Bronner. 2018. Engagement with social media and social media advertising: The differentiating role of platform type. Journal of Advertising. 47, no. 1: 38–54
- Vries, N.J.D., and J. Carlson. 2014. Examining the drivers and brand performance implications of customer engagement with brands in the social media environment. Journal of Brand Management 21, no. 6: 495–15.
- Wallace, E., I. Buil, L. de Chernatony, and M. Hogan. 2014. Who “likes” you…and why? A typology of Facebook fans: From “fan”-atics and self- expressives to utilitarians and authentics. Journal of Advertising Research 54, no. 1: 92–109
- Waterloo, S.F., S.E. Baumgartner, J. Peter, and P.M. Valkenburg. Forthcoming. Norms of online expressions of emotion: Comparing Facebook, Twitter, Instagram, and WhatsApp. New Media & Society.
- Wilson, S.E., and D.M. Butler. 2007. A lot more to do: The sensitivity of time-series cross-section analyses to simple alternative specifications. Political Analysis 15, no. 2:101–23.
- Yaveroglu, I., and N. Donthu. 2008. Advertising repetition and placement issues in on-line environments. Journal of Advertising 37, no. 2:31–44.
- Yeykelis, L., J.J. Cummings, and B. Reeves. 2014. Multitasking on a single device: Arousal and the frequency, anticipation, and prediction of switching between media content on a computer. Journal of Communication 64, no. 1: 167–92.
- Yuki, T. 2015. What makes brands’ social content shareable on Facebook? Journal of Advertising Research 55, no. 4: 458–70.
- Zhu, Y.Q., and H.G. Chen. 2015. Social media and human need satisfaction: Implications for social media marketing. Business Horizons 58, no. 3: 335–45.