Abstract
Objectives
To develop and assess nonenhanced MRI-based radiomics model for the preoperative prediction of nonperfused volume (NPV) ratio of uterine leiomyomas after high-intensity focused ultrasound (HIFU) treatment.
Methods
Two hundred and five patients with uterine leiomyomas treated by HIFU were enrolled and allocated to training (N =164) and testing cohorts (N = 41). Pyradiomics was used to extract radiomics features from T2-weighted images and apparent diffusion coefficient (ADC) map generated from diffusion-weighted imaging (DWI). The clinico-radiological model, radiomics model, and radiomics-clinical model which combined the selected radiomics features and clinical parameters were used to predict technical outcomes determined by NPV ratios where three classification groups were created (NPV ratio ≤ 50%, 50–80% or ≥ 80%). The receiver operating characteristic (ROC) curve, area under the curve (AUC), and calibration and decision curve analyses were performed to illustrate the prediction performance and clinical usefulness of model in the training and testing cohorts.
Results
The multi-parametric MRI-based radiomics model outperformed T2-weighted imaging (T2WI)-based radiomics model, which achieved an average AUC of 0.769 (95% confidence interval [CI], 0.701–0.842), and showed satisfactory prediction performance for NPV ratio classification. The radiomics-clinical model demonstrated best prediction performance for HIFU treatment outcome, with an average AUC of 0.802 (95% CI, 0.796–0.850) and an accuracy of 0.762 (95% CI, 0.698–0.815) in the testing cohort, compared to the clinico-radiological and radiomics models. The decision curve also indicated favorable clinical usefulness of the radiomics-clinical model.
Conclusions
Nonenhanced MRI-based radiomics has potential in the preoperative prediction of NPV ratio for HIFU ablation of uterine leiomyomas.
Introduction
Uterine fibroids are the most common noncancerous growths of the uterus in reproductive women, which have a broad negative impact on the health and life quality of women [Citation1,Citation2]. When pharmacotherapy is noneffective in relieving symptoms caused by uterine fibroids, various intervention approaches, such as hysterectomy, laparoscopic myomectomy, uterine artery embolization, and high-intensity focused ultrasound (HIFU) ablation, have been established for eventual treatment [Citation2,Citation3]. Compared to surgical operation, minimally invasive treatment approaches have the advantages of lower risk, minor complications, no general anesthesia, and faster recovery [Citation4–7].
HIFU is an effective minimally invasive treatment option for symptomatic uterine leiomyomas in abundant clinical trials [Citation8,Citation9], especially applicable for patients with fertility desires. It uses high-intensity focused ultrasound to thermally ablate leiomyomas, which causes coagulative necrosis of the target lesions by focusing ultrasonic energy. The use of HIFU treatment in uterine leiomyomas leads to clinical improvement with few significant clinical complications and adverse events [Citation10]. However, preoperative evaluation of HIFU treatment is a crucial factor for guiding patient selection and ensuring the clinical success of HIFU treatment [Citation11,Citation12].
Magnetic resonance imaging (MRI) as noninvasive technique is commonly used to evaluate the technical and clinical outcomes of HIFU treatment of uterine fibroids [Citation13]. Some studies also focused on the investigation of the factors affecting clinical treatment success. T2 signal intensity is the primary radiological indicator for determining patient suitability for HIFU therapy. Several studies have investigated the correlation between the features of T2-weighted (T2W) images and the treatment outcome of HIFU ablation for uterine leiomyomas [Citation11,Citation14,Citation15]. The fibroids characterized by high T2 signal intensity (type III in Funaki classification) [Citation15] or heterogeneous and high T2 signal intensity relative to that of the myometrium are unsuitable for HIFU ablation [Citation16], while those with low signal intensity relative to that of the myometrium, lower signal intensity than that of skeletal muscle or isointensity on T2W images have a significantly higher nonperfused volume (NPV) after HIFU ablation [Citation12,Citation14]. Due to lacking of reflecting the effect of vascularity and perfusion, T2W image-based traditional classification alone not only produces inaccuracies in predicting therapeutic response but also exhibits varying treatment results [Citation11,Citation17]. Therefore, functional MRI technologies such as diffusion-weighted imaging (DWI) [Citation18], contrast-enhanced fat-saturated T1-weighted (CE-FS-T1W) imaging, dynamic contrast-enhanced MRI (DCE-MRI) have been used for investigating the factors related to the treatment outcome further. Sainio et al. [Citation19] investigated the use of apparent diffusion coefficient (ADC) in predicting the technical treatment outcome of symptomatic uterine fibroids and found that the ADC classification seemed to have potential for predicting the NPV ratio and may even outperform the Funaki classification. Mindjuk et al. [Citation11] manifested the fibroids with low signal intensity on CE-FS-T1W mages and had a significantly higher NPV. Moreover, a high Ktrans value at baseline DCE-MRI images was revealed to be a significant predictor of poor therapeutic response in HIFU ablation [Citation20]. Wei et al. [Citation21] reported higher Ktrans, blood flow, and blood volume values were correlated negatively with a successful clinical outcome. Keserci et al. [Citation22] studied multiple clinical predictors for predicting an NPV ratio of at least 90% using a generalized multivariate model. Although some influential clinical factors related to the successful HIFU treatment outcome of uterine fibroids were found, the causal explanation of a regularity, described by a clinical phenomenon, is somewhat different from that of individual medical diagnosis, and there is still lacking of the personalized prediction model to preoperatively guide clinical decision making and definitively known which patient will achieve better response to HIFU ablation.
At present, radiomics converting medical images into high-dimensional characterization data has been confirmed as a hopeful technology to quantify phenotypic characteristics of tumors for prognostic prediction in the multimodal medical images using high-throughput feature computation [Citation23–25]. By determining the best combination of feature selection and classifiers, machine learning algorithms are usually used to distinguish among patients with similar outcome conditions and to establish scientific and data-driven analysis individual prediction model for treatment outcome, which has potential in patient selection. Recent study has shown promising result in predicting the response to HIFU therapy in patients with adenomyosis using T2-weighted imaging (T2WI)-based radiomics machine learning model [Citation26]. Unfortunately, there remains lack of radiomics and machine learning–based studies to preoperatively predict the clinical therapeutic success for HIFU ablation of uterine leiomyomas using nonenhanced MR images.
This study aims to explore the use of radiomics and machine learning in nonenhanced images for preoperatively predicting immediate therapeutic response (NPV ratio prediction) of HIFU ablation of symptomatic uterine leiomyomas so as to reduce the risk of clinical unsuccessful outcome of uterine leiomyomas in HIFU therapy.
Materials and methods
Study population
This retrospective research was approved by the Institutional Review Board of our hospital, and the need to acquire patient consent was waived. From July 2013 to December 2020, 348 patients receiving HIFU ablation therapy for uterine leiomyomas were enrolled for this analysis. The inclusion criteria were as follows: premenopausal or perimenopausal women who (1) were above 18 years of age, (2) diagnosed with clinical symptomatic uterine leiomyomas with diameters ≥3 cm, (3) received MRI examinations before and after HIFU therapy, and (4) had no previous history of surgery or drug treatment. The exclusion criteria were as follows: patients (1) with intolerance to MRI examination or contrast agent injection, (2) with pelvic inflammatory disease or endometrial disease or uncontrolled systemic disease, (3) with suspected malignancy uterine tumor, and (4) in pregnancy and lactation. To eliminate the influence of texture and intensity differences caused by different b values on radiomics feature extraction, the DWI images with the uniform b values of 0 and 800 s/mm2 were used to generate ADC maps and the others were excluded, so a total of 205 patients with uterine leiomyomas were enrolled finally.
MRI scanning protocol
All patients with uterine leiomyomas were required to undergo MRI examination using 3.0 T system (Signa HDxt, GE Medical System) before and after HIFU treatment, respectively. Fast spoiled gradient echo T1-weighted imaging was acquired using the following parameters: TR/TE, 175/2 ms; slice thickness 5 mm; slice gap, 1.5 mm; matrix, 320 × 224; FOV, 400 × 280 mm; axial plane. Fat-suppressed fast-spin-echo T2-weighted imaging (T2WI) was acquired using the following parameters: TR/TE, 4380/106 ms; FA, 90°; slice thickness 5 mm; slice gap, 1.5 mm; matrix, 320 × 224; FOV, 280 × 224 mm; axial and sagittal plane. DWI was acquired using the following parameters: TR/TE, 4000/62.9 ms; slice thickness, 6 mm; slice gap, 1.5 mm; matrix, 128 × 130; FOV, 380 × 458 mm; b values, 0 and 800 s/mm2; axial plane. Dynamic contrast-enhanced (DCE) MRI using 3 D T1-weighted LAVA sequence was acquired using the following parameters: TR/TE, 4.2/1.9 ms; slice thickness 4 mm; slice gap, 0 mm; matrix, 320 × 224; FOV, 380 × 304 mm; axial plane.
HIFU system and treatment procedure
All HIFU therapy was performed using an ultrasound-guided HIFU tumor therapeutic system (JC200, Chongqing Haifu Medical Technology Co., Ltd., Chongqing, China). This system comprises a therapeutic focused ultrasound transducer and an ultrasound imaging equipment (MyLab 70, Esaote, Genova, Italy) situated in the center of the transducer which provides real-time imaging to monitor ablation process. The patients lay on the treatment bed in a prone position with the abdominal wall soaked in degassed water. HIFU ablation therapy was broken up when an obvious general or great grey-scale change was observed inside the leiomyoma lesion.
NPV calculation and classification grouping
Immediately after the treatment ablated tissue can be assessed from CE-FS-T1W images as non-enhancing regions also known as the NPV which can be used to calculate the NPV ratio, which is defined as nonperfused leiomyoma volume/total leiomyoma volume. The calculation of NPV ratio was implemented in the AW-server workstation (version 4.0, GE Healthcare) by outlining the target leiomyoma before treatment and the non-enhancing regions after treatment on the axial images layer by layer. A higher NPV ratio of more than 80%, that is, technical outcome, has been proven to be highly associated with a clinical treatment success [Citation11,Citation13]. Therefore, this study divided the samples into three subgroups in terms of the achieved NPV ratio ≤ 50%, 50–80% or ≥ 80%.
Image segmentation
All pretreatment MRI images were exported from PACS in DICOM format. Two blinded abdominal radiologists with 7 and 12 years of experience in pelvic radiological imaging independently interpreted all MR images and manually determined the regions of interest (ROI) by delineating the margin of leiomyoma using ITK-SNAP software (www. itksnap.org) in the axial plane, as shown in . The ROIs of DWI were delineated on corresponding apparent diffusion coefficient (ADC) maps. The principles of ROI sketching were as follows: (1) sketching layer by layer to form the 3 D ROIs of the lesion; (2) including the cystic and necrotic area of the lesion; (3) When the tumor boundary is blurred, sketching the maximum extent of the lesion as much as possible.
Radiomics feature extraction
Image preprocessing such as B-spline interpolation to isotropic voxel spacing and N4 bias correction was performed, then normalization was applied to the images to eliminate the influence of variation in gray-scale ranges. Radiomics features were extracted from each of T2W images and ADC maps, which comprise first-order statistics features, intensity- and shape-based features, and high-order textural features such as gray-level co-occurrence (GLC), run-length (GLRL), size zone (GLSZ), dependence (GLD) matrixes and neighborhood gray-tone difference matrix [Citation27]. Feature extraction procedure was performed based on the Image Biomarker Standardization Initiative (IBSI) [Citation28] using the PyRadiomics package (https://pyradiomics.readthedocs.io/) in Python (version 3.6), and a fixed bin width of 25 was used in the radiomics feature extraction [Citation29].
Feature selection and reproducibility evaluation
The z-score normalization was applied to the feature matrix by subtracting the mean and dividing by the standard deviation. To assess the reproducibility of radiomics features, we calculated the intraclass correlation coefficient (ICC) for each feature on two radiologists’ segmentations, and only those with ICC values more than 0.8 representing high stability entered further analysis [Citation30]. Then, differentiation and correlation analyses were employed to search redundant features for removal.
Clinico-radiological model, radiomics model, and radiomics-clinical model construction
The clinico-radiological model was constructed with clinical characteristics and radiological signs such as fibroid volume, fibroid type, fibroid location, T2 signal intensity, T2 signal homogeneity and uterine position, and the radiomics model was constructed with selected radiomics features from MR images. The different combinations of feature selection methods and machine learning classifiers were used to seek the best suitable radiomics model for the preoperative outcome prediction of HIFU treatment. Five machine learning classifiers such as k-nearest neighbor (KNN), logistic regression (LR), random forest (RF), CatBoost and support vector machine (SVM) were implemented using the scikit-learn (version 0.23.0) library in Python (version 3.6.2) environment. Then, the algorithms of recursive feature elimination (RFE), ReliefF and least absolute shrinkage and selection operator (LASSO) were utilized to select features. The publicly available implementations were readily available for these methods, which also increases their reusability. The classification performance of 15 radiomics-based machine learning models comprise of different combinations of feature selection and machining learning algorithms was compared and evaluated to determine the optimal classifier which then was used to construct the radiomics-clinical model. We also compared the performance of the optimal radiomics model constructed by radiomics features extracted from multi-parametric sequences and single-parametric sequence, respectively. The radiomics-clinical model was built by the combination of the MR-based radiomics features and clinico-radiological signs. The random numbers generated by computer were used to assign 70% of the data samples to the training cohort and the others to the testing cohort. In this study, a grid-search strategy with five-fold cross validation was applied on the training cohort to search the optimum hyper-parameters for all models [Citation31]. To avoid potential resampling biases and statistical anomalies, the data in 5-fold cross-validation were identical when training the different models [Citation32]. The process of the model construction is presented in .
Performance validation
The receiver operating characteristic (ROC) curve analysis was utilized to illustrate qualitatively the performance of classification model in the training and testing datasets, and the area under the ROC curve (AUC) was computed for quantitative evaluation. The maximum value of the Youden index determined by a cutoff value was calculated by means of standard performance metrics including accuracy, sensitivity and specificity. A nonparametric bootstrap method was used to calculate the 95% confidence interval (CI) by the repeated 1000 times sampling in the testing set. The comparison of AUCs was accomplished using the DeLong nonparametric approach [Citation33]. Decision curve analysis was performed by quantifying the net benefits for a range of threshold probabilities to evaluate the clinical usefulness of the radiomics model. Calibration curve along with the Hosmer–Lemeshow test was used to evaluate the similarity between the predicted and observed robabilities [Citation34]. The average predictive performance metrics were obtained, and the most efficient model with the highest AUC and accuracy was determined as predictive model used to evaluate the diagnostic efficacy in predicting outcome of uterine leiomyoma ablation.
Statistical analysis
Normally distributed continuous variables were presented as mean ± standard deviations (SD), whereas those variables with skewed distributions were presented as median with interquartile ranges [M (Q1, Q3)]. The independent-sample t-test or Mann–Whitney U-test was used between both groups, and analysis of variance or Kruskal–Wallis test was used among three groups. Categorical variables were expressed as frequency (proportion), and the chi-square test or Fisher's exact test was used for data comparison between groups. Statistical analysis was performed with R software (version 3.6.1). A two-sided p < 0.05 was considered to represent statistically significant.
Results
Clinical and radiological characteristics
The clinical and radiological characteristics of the patients were presented in . There were no significant differences of baseline characteristics between the training and testing cohorts. Among different NPV ratioing classification groups, there were also no significant differences in the train and testing cohorts in the volume, size, subtypes and location of leiomyomas (p > 0.05, ). T2 signal intensity was significantly different in the training cohort.
Table 1. The comparison of demographic information and radiological characteristics between the training and testing cohorts.
Table 2. The clinical and radiological characteristics among NPV ratio classification groups.
Performance comparison of radiomics based machine learning models
In this study, 1118 candidate radiomics features were extracted from T2W images and ADC maps, respectively. After reproducibility evaluation and redundant removal, totally 1537 radiomics features were filtered out. The 15 different radiomics models were constructed by the combinations of three feature selection methods and five machine learning classifiers, respectively. The ReliefF-SVM based radiomics model achieved best performance with an average AUC of 0.804 (95% CI, 0.757–0.849) and an accuracy of 0.769 (95% CI, 0.742–0.811), as shown in , based on the performance of 5-fold cross-validation in the training cohort. The RFE-CatBoost model showed the secondary predictive performance with an average AUC of 0.778 (95% CI, 0.734–0.822) and accuracy of 0.759 (95% CI, 0.714–0.806), followed by RFE-SVM model which yielded an average AUC of 0.764 (95% CI, 0.716–0.803) and accuracy of 0.745 (95% CI, 0.703–0.792) in the training cohort. Moreover, the comparison of performance between the incorporation of T2WI and ADC-based and alone T2WI-based ReliefF-SVM was performed, and the multi-parameter model was superior to single parameter model. DeLong test suggested that the prediction performance of the ReliefF-SVM model was significantly better than that of the other models (all p<0.05). The ReliefF-SVM model that achieved the best AUC and accuracy was chosen to construct radiomics-clinical model further.
Predictive validation of the clinico-radiological, radiomics, and radiomics-clinical models
The ROC analysis result of the clinico-radiological, radiomics, and radiomics-clinical models in the training and test cohorts was shown in . The clinico-radiological performed worst in predicting the NPV ratio after HIFU treatment, with an average AUC of 0.763 (95% CI, 0.561–0.882) in the training cohort and an average AUC of 0.715 (95% CI, 0.603–0.794) in the testing cohort. The radiomics model demonstrated a progressive performance to predict the outcome of HIFU treatment, with an average AUC of 0.804 (95% CI, 0.757–0.849) in the training cohort and an average AUC of 0.769 (95% CI, 0.701–0.842) in the testing cohort. The radiomics-clinical model exhibited the advanced capability for the prediction of clinical outcome in HIFU therapy, and achieved an average AUC of 0.857 (95% CI, 0.814–0.903) and 0.802 (95% CI, 0.796–0.850) in the training cohort and testing cohort, respectively, with a significant performance improvement (both p<0.05). Detailed information about the prediction performance of the models is shown in .
Figure 4. The average AUC shown by ROC curves for clinico-radiological model, radiomics model, and radiomics-clinical model in predicting NPV ratio classification for (a) the training and (b) testing cohorts.
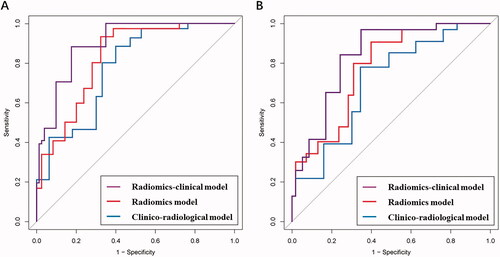
Table 3. The performance of clinico-radiological model, radiomics model, and radiomics-clinical model in predicting NPV ratio classification.
Clinical usefulness
The calibration curves and decision curves for the models that predict the NPV ratio great than or equal to 80% were shown in . A good agreement between the prediction and observation data in the testing cohort was confirmed for three models, and the optimal calibration curve demonstrated best agreement between the prediction of radiomics-clinical and actual outcome in the testing cohort. The result of Hosmer-Lemeshow test indicated no statistical significance (p = 0.325, 0.471 and 0.630, respectively) and suggested no departure from the favorable fitting. The decision curve analysis also demonstrated that the performance of the radiomics-clinical model () with a higher net benefit was superior to that of the clinico-radiological model and radiomics model. It showed that if the threshold probability is between 0.05 and 0.5, the application of the radiomics-clinical model in predicting the successful clinical outcome of HIFU treatment provides more net benefit than either the ‘treat-all-patients’ or the ‘treat-none’ method.
Figure 5. Further model performance evaluation by (a) calibration curve analysis in terms of the agreement between the predicted and actual probability and (b) decision curve analysis in terms of net benefit for the clinico-radiological model, radiomics model, and radiomics-clinical model in the testing cohort.
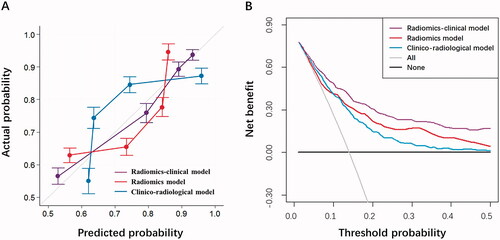
Discussion
HIFU ablation of uterine fibroids is being increasingly used globally owing to its outstanding therapeutic efficacy and promising clinical use. The NPV ratio has been proven to be the main factor associated with clinical success. Several studies reported with that NPV ratios ranged from 20% to 90% [Citation35–37]. Some studies showed that higher NPV ratios more than 80% could be associated with an excellent clinical result. Therefore, our study developed a nonenhanced MRI radiomics based machine leaning model to predict preoperatively the nonperfused volume ratio classification (≤ 50%, 50–80% or ≥ 80%) of uterine leiomyomas after HIFU treatment. This is also the first study that uses radiomics and machine learning analyses to predict NPV ratio classification and clinical success for HIFU treatment of uterine fibroids.
MRI is a noninvasive and accurate technique in the preoperative assessment of HIFU treatment of uterine fibroids, and allows differentiation of signal intensity between different tissues, because of its excellent contrast resolution. Previous studies suggest that some radiological and clinical characteristics such as the signal intensity of uterine fibroids relative to pelvis muscles or skeletal muscle on T2W images [Citation12], enhancement type on T1-weighted (T1WI) image, subcutaneous fat thickness, thickness of the rectus abdominis, distance from the anterior surface of the fibroid to the skin, and location of the uterus were the significant predictive indictors for NPV ratios [Citation15,Citation38,Citation39]. These qualitative MRI characteristics can be regarded as correlation with clinical success, while radiomics can effectively quantify those that are identified as radiological signs by using texture features, and therefore, radiomics analysis allows the new discovery of signatures in MR images not interpretable with the naked eye. This explains why the efficacy of the radiomics model is superior to the clinical model.
The study referring to MR imaging findings and histopathologic backgrounds confirms that low signal intensity on T2W images is caused by extensive hyalinization [Citation40], while high signal intensity is related to high vascularity, cellularity, rich tissues, or degeneration, which lead to poor tissue ablation because of low absorption of ultrasonic energy. Although the Funaki classification in terms of signal intensity on T2W images has been widely used as a predictor of the therapeutic efficacy of HIFU treatment [Citation15,Citation41,Citation42], some studies showed that the signal intensity of leiomyomas on T2W images cannot differentiate between vascularity and edema or degeneration. Therefore, it has been recognized that T2WI-based predictor may produce various or infeasible results for treatment outcome in conditions attributed to different tissue properties [Citation11,Citation17]. Our study showed there were more high-order textural features than first-order and shape features in the selected radiomics features for the outcome prediction of HIFU treatment. Although the T2WI signs visible to the naked eye cannot reflect vascularity and perfusion [Citation38], these tiny tissue differences caused by different histopathology in image intensity, shape, or texture can be quantified by means of radiomics, thus overcoming the subjective nature of image interpretation. This may be the reason why that T2WI based radiomics model can outperform the clinico-radiological model.
DWI is a commonly used functional MRI technique, and the postprocessing ADC map can use the motion of water molecules to generate the mapping of the diffusion process of those in biological tissues, which can provide information with respect to cellularity and microcirculation and reflect the perfusion on MR images [Citation19]. It has been recognized that the histopathologically poor HIFU treatment outcomes are often associated with high cellularity and increased vascularity of uterine leiomyomas, and the degenerative leiomyomas are easily treated by HIFU as they contain limited cellularity and decreased blood flow. Our study also showed that the multi-parametric MRI (T2WI and DWI)-based radiomics machine learning model outperformed T2WI-based one alone, because the radiomics features from both T2W images and ADC map could provide more information than those from T2W image alone. It is supported by previously published study [Citation19] that the ADC classification may even outperform the Funaki classification in predicting NPV ratio.
Moreover, the ability of quantitative DCE-MRI parameters to predict the ablation efficacy of HIFU treatment for uterine leiomyomas has been investigated [Citation17,Citation20,Citation43,Citation44]. They suggested that the higher Ktrans, blood flow, and blood volume derived from DCE-MRI are negatively associated with a successful treatment outcome. Ktrans map was also used to visualize the perfusion effect within the fibroids which might greatly impact the ablation efficacy. Mindjuk et al [Citation11] demonstrated that the NPV ratio could be effectively predicted on the basis of the signal intensity of fibroid on contrast-enhanced MR images. Yoon et al [Citation17] showed that fibroids with hyperintensity on T2W image exhibiting delayed enhancement on DCE-MRI images could receive successful ablation. Our study investigated the use of conventional noenhanced MRI images combined with radiomics and machine learning to predict the efficiency of HIFU ablation, so as to potentially minimize the need for contrast agent administration as well as avoid the contrast agent adverse events.
Our study showed that the combination of multi-parametric MRI based radiomics machine learning model with clinical parameters in the preoperative prediction of treatment outcome for HIFU ablation of leiomyomas achieved better performance than alone radiomics machine learning model. This is also in line with the previous study [Citation22], where clinical parameters such as the subcutaneous fat thickness and location of the uterus have been proven to be a significant impact on the HIFU treatment of uterine fibroids
Some limitations also exist in the present study. First, the single-center retrospective study design with small sample size is investigated, so further prospective multicenter studies are required for the model validation and improvement. Second, in our study, we only considered nonenhanced MRI parameters for preoperatively predicting NPV ratio of uterine leiomyomas after HIFU ablation, and future studies need combine the functional or perfusion parameters to predict HIFU efficacy. Third, more multiple-b values such as 200 s/mm2 and 400 s/mm2 in DWI should be considered to investigate vascularity in future. Fourth, some laboratory biochemical parameters such as luteinizing hormone, follicle-stimulating hormone and serum estradiol were not evaluated, and these indicators would be incorporated for evaluation of treatment efficacy in the future.
Abbreviations | ||
HIFU | = | High-intensity focused ultrasound |
T2WI | = | T2-weighted imaging |
DWI | = | diffusion-weighted imaging |
DCE | = | Dynamic contrast enhanced |
PACS | = | Picture archiving and communication system |
GLCM | = | Gray-level co-occurrence matrix |
GLRLM | = | Gray-level run-length matrix |
GLSZM | = | Gray-level size zone matrix |
NGTDM | = | Neighborhood gray-tone difference matrix |
GLDM | = | Gray-level dependence matrix |
ICC | = | Intraclass correlation coefficient |
NPV | = | Negative predictive value |
LASSO | = | Least absolute shrinkage and selection operator |
RFE | = | Recursive feature elimination |
KNN | = | K-nearest neighbors |
LR | = | Logistic regression |
RF | = | Random forest |
SVM | = | Support vector machine |
ROC | = | Receiver operating characteristics |
AUC | = | Area under the ROC curve |
Disclosure statement
No potential conflict of interest was reported by the author(s).
Data availability statement
The data used to support the findings of this study are available from the corresponding author upon request
Additional information
Funding
References
- Marshall LM, Spiegelman D, Barbieri RL, et al. Variation in the incidence of uterine leiomyoma among premenopausal women by age and race. Obstet Gynecol. 1997;90(6):967–973.,
- Ali M, Chaudhry ZT, Al-Hendy A. Successes and failures of uterine leiomyoma drug discovery. Expert Opin Drug Discov. 2018;13(2):169–177.
- Khan AT, Shehmar M, Gupta JK. Uterine fibroids: current perspectives. Int J Women's Health. 2014;6:95.
- Jun F, Yamin L, Xinli X, et al. Uterine artery embolization versus surgery for symptomatic uterine fibroids: a randomized controlled trial and a meta-analysis of the literature. Arch Gynecol Obstet. 2012;285(5):1407–1413.
- van der Kooij SM, Bipat S, Hehenkamp WJ, et al. Uterine artery embolization versus surgery in the treatment of symptomatic fibroids: a systematic review and metaanalysis. Am J Obstet Gynecol. 2011;205(4):317.e1–e318.
- R. Investigators. Uterine-artery embolization versus surgery for symptomatic uterine fibroids. New Eng J Med. 2007;356:360–370.
- Manyonda IT, Bratby M, Horst JS, et al. Uterine artery embolization versus myomectomy: impact on quality of life—results of the FUME (fibroids of the uterus: myomectomy versus embolization) trial. Cardiovasc Intervent Radiol. 2012;35(3):530–536.
- Cheung VY. High-intensity focused ultrasound therapy. Best Pract Res Clin Obstet Gynaecol. 2018;46:74–83.
- Chen J, Li Y, Wang Z, Committee of the Clinical Trial of HIFU versus Surgical Treatment for Fibroids, et al. C.o.t.C.T.o.H.v.S.T.f. Fibroids, evaluation of high‐intensity focused ultrasound ablation for uterine fibroids: an IDEAL prospective exploration study. BJOG: Int J Obstet Gy. 2018;125(3):354–364.,
- Ji Y, Hu K, Zhang Y, et al. High-intensity focused ultrasound (HIFU) treatment for uterine fibroids: a meta-analysis. Arch Gynecol Obstet. 2017;296(6):1181–1188.,
- Mindjuk I, Trumm CG, Herzog P, et al. MRI predictors of clinical success in MR-guided focused ultrasound (MRgFUS) treatments of uterine fibroids: results from a single centre. Eur Radiol. 2015;25(5):1317–1328.
- Kim Y-S, Lim HK, Park MJ, et al. Screening magnetic resonance imaging-based prediction model for assessing immediate therapeutic response to magnetic resonance imaging-guided high-intensity focused ultrasound ablation of uterine fibroids. Invest Radiol. 2016;51(1):15–24.,
- Andrews S, Yuan Q, Bailey A, et al. Multiparametric MRI characterization of funaki types of uterine fibroids considered for MR-guided high-intensity focused ultrasound (MR-HIFU) therapy. Acad Radiol. 2019;26(4):e9–e17.,
- Zhao W-P, Chen J-Y, Zhang L, et al. Feasibility of ultrasound-guided high intensity focused ultrasound ablating uterine fibroids with hyperintense on T2-weighted MR imaging. Eur J Radiol. 2013;82(1):e43–e49.,
- Funaki K, Fukunishi H, Funaki T, et al. Magnetic resonance-guided focused ultrasound surgery for uterine fibroids: relationship between the therapeutic effects and signal intensity of preexisting T2-weighted magnetic resonance images. Am J Obstet Gynecol. 2007;196(2):184. e181–184. e186.,
- Mikami K, Murakami T, Okada A, et al. Magnetic resonance imaging-guided focused ultrasound ablation of uterine fibroids: early clinical experience. Radiat Med. 2008;26(4):198–205.,
- Yoon S-W, Lee C, Kim KA, et al. Contrast-enhanced dynamic MR imaging of uterine fibroids as a potential predictor of patient eligibility for MR guided focused ultrasound (MRgFUS) treatment for symptomatic uterine fibroids. Obstet Gynecol Int. 2010;2010,
- Jha RC, Zanello PA, Ascher SM, et al. Diffusion-weighted imaging (DWI) of adenomyosis and fibroids of the uterus. Abdom Imaging. 2014;39(3):562–569.,
- Sainio T, Saunavaara J, Komar G, et al. Feasibility of apparent diffusion coefficient in predicting the technical outcome of MR-guided high-intensity focused ultrasound treatment of uterine fibroids–a comparison with the funaki classification. Int J Hyperthermia. 2021;38(1):85–94.
- Kim Y-S, Lim HK, Kim J-H, et al. Dynamic contrast-enhanced magnetic resonance imaging predicts immediate therapeutic response of magnetic resonance-guided high-intensity focused ultrasound ablation of symptomatic uterine fibroids. Invest Radiol. 2011;46:639–647.,
- Wei C, Fang X, Wang C-B, et al. The predictive value of quantitative DCE metrics for immediate therapeutic response of high-intensity focused ultrasound ablation (HIFU) of symptomatic uterine fibroids. Abdom Radiol. 2018;43(8):2169–2175.,
- Keserci B, Duc NM. Magnetic resonance imaging parameters in predicting the treatment outcome of high-intensity focused ultrasound ablation of uterine fibroids with an immediate nonperfused volume ratio of at least 90. Acad Radiol. 2018;25(10):1257–1269. %,
- Lambin P, Leijenaar RT, Deist TM, et al. Radiomics: the bridge between medical imaging and personalized medicine. Nat Rev Clin Oncol. 2017;14(12):749–762.,
- Huang Y-Q, Liang C-h, He L, et al. Development and validation of a radiomics nomogram for preoperative prediction of lymph node metastasis in colorectal cancer. J Clin Oncol. 2016;34(18):2157–2164.,
- Gillies RJ, Kinahan PE, Hricak H. Radiomics: images are more than pictures, they are data. Radiology. 2016;278(2):563–577.
- Li Z, Zhang J, Song Y, et al. Utilization of radiomics to predict long-term outcome of magnetic resonance-guided focused ultrasound ablation therapy in adenomyosis. Eur Radiol. 2021;31(1):392–402.,
- Aerts HJ, Velazquez ER, Leijenaar RT, et al. Decoding tumour phenotype by noninvasive imaging using a quantitative radiomics approach. Nat Commun. 2014;5(1):1–9.,
- Zwanenburg A, Vallières M, Abdalah MA, et al. The image biomarker standardization initiative: standardized quantitative radiomics for high-throughput image-based phenotyping. Radiology. 2020;295(2):328–338.
- Vallières M, Freeman CR, Skamene SR, et al. A radiomics model from joint FDG-PET and MRI texture features for the prediction of lung metastases in soft-tissue sarcomas of the extremities. Phys Med Biol. 2015;60(14):5471–5496.,
- Pavic M, Bogowicz M, Würms X, et al. Influence of inter-observer delineation variability on radiomics stability in different tumor sites. Acta Oncol. 2018;57(8):1070–1074.,
- Chang Y, Lafata K, Sun W, et al. An investigation of machine learning methods in Delta-radiomics feature analysis. PLoS One. 2019;14(12):e0226348.,
- Lafata K, Cai J, Wang C, et al. Spatial-temporal variability of radiomic features and its effect on the classification of lung cancer histology. Phys Med Biol. 2018;63(22):225003
- Pencina MJ, D' Agostino RB, D' Agostino RB, et al. Evaluating the added predictive ability of a new marker: from area under the ROC curve to reclassification and beyond. Statist Med. 2008;27(2):157–172.,
- Alba AC, Agoritsas T, Walsh M, et al. Discrimination and calibration of clinical prediction models: Users' Guides to the Medical Literature. JAMA. 2017;318(14):1377–1384.,
- Wang W, Wang Y, Wang T, et al. Safety and efficacy of US-guided high-intensity focused ultrasound for treatment of submucosal fibroids. Eur Radiol. 2012;22(11):2553–2558.,
- Leung JH, Yu SC, Cheung EC, et al. Safety and efficacy of sonographically guided high‐intensity focused ultrasound for symptomatic uterine fibroids: Preliminary study of a modified protocol. J Ultrasound Med. 2014;33(10):1811–1818.,
- Keserci B, Duc NM. Magnetic resonance imaging features influencing high-intensity focused ultrasound ablation of adenomyosis with a nonperfused volume ratio of≥ 90% as a measure of clinical treatment success: retrospective multivariate analysis. Int J Hyperthermia. 2018;35(1):626–636.
- Kim Y-S, Lee J-W, Choi CH, et al. Uterine fibroids: correlation of T2 signal intensity with semiquantitative perfusion MR parameters in patients screened for MR-guided high-intensity focused ultrasound ablation. Radiology. 2016;278(3):925–935.,
- Park H, Yoon SW, Sokolov A. Scaled signal intensity of uterine fibroids based on T2-weighted MR images: a potential objective method to determine the suitability for magnetic resonance-guided focused ultrasound surgery of uterine fibroids. Eur Radiol. 2015;25(12):3455–3458.
- Ueda H, Togashi K, Konishi I, et al. Unusual appearances of uterine leiomyomas: MR imaging findings and their histopathologic backgrounds. Radiographics. 1999;19 Spec No:S131–S145.
- Funaki K, Fukunishi H, Funaki T, et al. Mid-term outcome of magnetic resonance-guided focused ultrasound surgery for uterine myomas: from six to twelve months after volume reduction. J Minim Invasive Gynecol. 2007;14(5):616–621.
- Lénárd ZM, McDannold NJ, Fennessy FM, et al. Uterine leiomyomas: MR imaging-guided focused ultrasound surgery-imaging predictors of success. Radiology. 2008;249(1):187–194.,
- Liu J, Keserci B, Yang X, et al. Volume transfer constant (K(trans)) maps from dynamic contrast enhanced MRI as potential guidance for MR-guided high intensity focused ultrasound treatment of hypervascular uterine fibroids. Magn Reson Imaging. 2014;32(9):1156–1161.,
- Zhao WP, Chen JY, Chen WZ. Dynamic contrast-enhanced MRI serves as a predictor of HIFU treatment outcome for uterine fibroids with hyperintensity in T2-weighted images. Exp Ther Med. 2016;11(1):328–334.