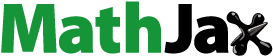
Abstract
Objective
To achieve a result of a large tumor ablation volume with minimal thermal damage to the surrounding blood vessels by designing a few clinically-adjustable operating parameters in radiofrequency ablation (RFA) for liver tumors abutting complex vascular structures.
Methods
Response surface method (RSM) was employed to correlate the ablated tumor volume () and thermal damage to blood vessels (
) based on RFA operating parameters: ablation time, electrode position, and insertion angle. A coupled electric-thermal-fluid RFA computer model was created as the testbed for RSM to simulate RFA process. Then, an optimal RFA protocol for the two conflicting goals, namely (1) large tumor ablation and (2) small thermal damage to the surrounding blood vessels, has been achieved under a specific ablation environment.
Results
Linear regression analysis confirmed that the RFA protocol significantly affected and
(the adjusted coefficient of determination
= 93.61% and 95.03%, respectively). For a proposed liver tumor scenario (liver tumor with a dimension of 4
3
2.9 cm3 abutting a complex vascular structure), an optimized RFA protocol was found based on the regression results in RSM. Compared with a reference RFA protocol, in which the electrode was centered in the tumor with a 12-min ablation time, the optimized RFA protocol has increased
from 98.1% to 99.6% and decreased
from 4.1% to 0.4%, achieving nearly the complete ablation of proposed liver tumor and ignorable thermal damages to vessels.
Conclusion
This work showed that it is possible to design a few clinically-adjustable operating parameters of RFA for achieving a large tumor ablation volume while minimizing thermal damage to the surrounding blood vessels.
1. Introduction
Radiofrequency ablation (RFA) is one of the energy-based minimally invasive tumor ablation modalities [Citation1,Citation2]. RFA has been widely used in the treatment of various tumors with favorable clinical outcomes [Citation3–8]. However, when treatment is applied to tumors abutting to large blood vessels, the heat generated by the electrode is dissipated by those large vessels (‘heat-sink’ effect), leading to incomplete tumor ablation [Citation9–11]. The incomplete ablation usually leads to the treatment failure and local recurrence [Citation12,Citation13]. Currently, there are no effective, safe, and easy ways available clinically to eliminate or minimize the ‘heat-sink’ effect of large vessels when RFA is used. It is still a challenge for RFA to achieve the complete ablation of liver tumors abutting large vessels and minimal thermal damages to surrounding vessels. Some methods attempted clinically include a temporary interruption of the blood flow (i.e., Intermittent Pringle maneuver [Citation14] or Balloon occlusion [Citation15]) and the development of new ablation electrodes [Citation16–19]. However, due to cooling protection of blood fluid, the method of vessel occlusion could usually cause thermal damages to vessels, leading to severe post-ablation complications, such as hemorrhage. Mathematical modeling and simulation have been applied widely in both the design and development of novel clinical protocols and the optimization and improvement of existing clinical protocols [Citation20,Citation21]. A comprehensive and validated mathematical model can provide reliable information not only on the treatment performance, but the spatiotemporal temperature and electric field distributions in the treatment area, which are difficult to attain in experiments [Citation19,Citation22]. Shao et al. [Citation19,Citation23] used mathematical models to investigate the thermal ablation of para-macrovascular tumors and the influence of the ‘heat-sink’ effect on the ablation zone. They designed a novel ablation electrode and a specific ablation protocol to reduce the ‘heat-sink’ effect of large blood vessels. They concluded that the distance between the RFA electrode and the blood vessel plays a major role in the incomplete ablation during the heating process and a new bipolar electrode matrix system can achieve a larger tissue ablation zone while arriving at a lower peak temperature outcome. Huang et al. [Citation24] established a finite difference method to calculate the ablation zone formation via RFA and investigated the influence of the location, diameter, and orientation of the blood vessel to the electrode on the ablation zone formation. They found that a vessel that is orthogonal to the RF electrode has less cooling impact in the ablation zone than a parallel vessel. González-Suárez et al. [Citation25] established a computer model to simulate in vivo RFA experiments in which the liver surface was heated by an internally cooled electrode placed as close as possible to a vessel. The computational findings verified that the heat-sink effect could protect the portal vein wall.
It is worth mentioning that the computer models currently used in literature only considered a simplified vascular system within homogenous liver tissues [Citation19,Citation21,Citation24,Citation25]. However, a growing body of evidence suggests that a liver tumor with different thermal and electrical properties should be included in the computer model. Furthermore, given that the liver is very vascular, the model should include a liver tumor abutting to a major vascular structure. Moreover, the size of the tumor and the distance between the tumor and the large blood vessels are also required to be considered in the model as they can affect the complete ablation of tumors and the thermal damage to the vessels. A model incorporating all these elements would be more representative of the treatment planning of RFA.
This paper aims to combine an engineering optimization method, response surface method (RSM) with computer modeling to enhance RFA treatments for a liver tumor scenario mentioned-above. Further, RFA treatment protocols with the goal of completely ablating the liver tumor while minimizing the thermal damage to the vessels were determined by optimizing only operating parameters that can be adjustable by radiologists or clinician during treatment in clinic. These parameters included the ablation time, the electrode position, and the angle of RF electrode. By considering the real liver anatomy, a 3 D RFA liver tumor model was built and placed in proximity to a complex vascular system including the hepatic vein, portal vein, and hepatic artery. In the such tumor position, the heat-sink effect of vessels has a significant effect on treatment outcome. The heat dissipation was modeled by using the Navier-Stokes equation combined with the Pennes bioheat transfer equation. The proposed model was then verified by comparing the tissue impedance change, ablation zone, and tissue temperature between the current model and ex vivo experiments in literature.
2. Materials and methods
2.1. Geometrical modeling
As shown in , a 3 D liver tumor with a complex vascular system was created in a cubic liver tissue with a dimension of 120120
130 mm3. The dimension of the liver tissue was determined by a convergence test with a criterion of less 0.1% change in the ablated volume between two contiguously increasing dimensions of liver tissue domain. An ellipsoidal liver tumor with dimensions of 40, 30, and 29 mm along the X-, Y- and Z-axis, respectively was generated within the liver tissue. Three tubular structures (i.e., hepatic vein, portal vein, and hepatic artery) in an anatomical structure were also modeled around the liver tumor. A commercial cool-tip RFA electrode (Cool-tipTM RF Ablation System E Series, Medtronic, Minneapolis, MN, USA) with a diameter of 15 mm and the active tip of 30 mm was inserted into the liver tumor. The inside of the electrode was cooled with chilled saline at the temperature of 10
The relative distribution of the elements in the 3 D model is shown in .
Figure 1. The geometric model of RFA for a liver tumor abutting a vascular structure: (a) a 3 D view and (b) X-Z cross-sectional view (with the variables () of electrode placement; dimension in mm and out of scale).
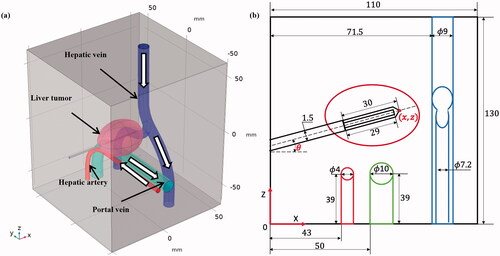
The diameters of the main hepatic vein and branch veins were taken as 9 and 7.2 mm, respectively, consistent with measurements from human liver anatomy [Citation26] (as illustrated in ). The velocity of blood flow in the hepatic vein was set as 39.5 cm/s. Similarly, the diameter of the portal vein and hepatic artery were 10 and 4 mm, respectively, and the flow velocities of the portal vein and hepatic artery were 19.8 and 44.3 cm/s, respectively. The thickness of all vessel walls was assumed set at 0.5 mm [Citation27–29]. The direction of blood flow in the three vascular structures are shown in the . Note that the flow directions of the portal vein and the hepatic artery are opposite.
Table 1. The velocity and diameter of three vessels in the hepatic vascular system [Citation27].
2.2. Coupled electric-thermal-fluid modeling of RFA
A commercial finite element software (COMSOL Multiphysics 5.5, COMSOL inc., Burlington, MA, USA) was used to simulate RFA procedure of the coupled multi-physics. During ablation process, RF power was applied to the electrode, and the Laplace equation was used to describe the electric field generated in the tissue of interest:
(1)
(1)
where
is the temperature-dependent tissue electrical conductivity and
is the applied voltage. Electric field intensity (
) and current density (
) can be calculated by
(2)
(2)
(3)
(3)
The local power density that causes the tissue heating is the product of the current density and the electric field strength as follows
(4)
(4)
The Pennes bioheat transfer Equation [Citation29] was used to govern the heat transfer in the tissues, as shown in Equation (5). Meanwhile, for the blood vessel, the fluid dynamic equation and the heat transfer equation were combined to simulate the ‘heat-sink’ effect of vessels. Given that, the Pennes equation was modified by adding a convection term to the right side of the equation, as given by Equation (6).
(5)
(5)
(6)
(6)
where
is the density of the tissue,
is the specific heat,
is the temperature,
is the thermal conductivity.
is the blood density,
is the specific heat of blood,
is the blood perfusion rate,
is the temperature of blood entering the tissue,
is the heat generated in the process of tissue metabolism, which is usually negligible due to its small magnitude compared with other items, and
is an external heat power generated by RF energy and it can be solved in Equation (4). In the convection term,
is the blood flow velocity field in the blood vessel, which is derived by the incompressible Navier–Stokes equation [Citation30], which consists of the momentum conservation equation (i.e., Equation. (7)) and mass conservation equation (i.e., EquationEquation. (8)
(8)
(8) )
(7)
(7)
(8)
(8)
where
is the pressure,
is the viscosity of the blood 0.0021(kg/m·s), and
is the volume force, which was not considered owing to its relatively small magnitude in this model.
In this study, the water vaporization of liver tissues was also considered as temperature-dependent [Citation30]
(9)
(9)
where
and
are the density and specific heat of the tissue that change due to the phase change, that is, at a temperature below 100 °C (
=
for liquid tissue phase) and a temperature above 100 °C (
=
for gaseous tissue phase),
is the latent heat, that is, the product of the latent heat of vaporization of water and the water density at 100 °C (2.162 × 109 J/m3), and
is the water ratio inside the liver tissue (68%). The thermal conductivity and electrical conductivity of liver tissues and liver tumor were also considered as temperature dependent in the study [Citation30,Citation31]
(10)
(10)
(11)
(11)
where
and
are the reference thermal conductivity and electrical conductivity, which are measured at a reference temperature of 37 °C, respectively. The blood perfusion of liver tissue was given by EquationEquation (12)
(12)
(12)
(12)
(12)
To evaluate the ablation zone, the Arrhenius model was used to determine the damaged tissue, which was given by EquationEquation (13)(13)
(13) [Citation19]
(13)
(13)
where
is the degree of tissue death,
is the frequency factor,
is the activation energy for the irreversible damage reaction,
is the universal gas constant (8.314 J mol−1 K−1), and
is the tissue temperature inside the computational domain at a specified time. For the liver tissue and liver tumor,
= 7.390 × 1039s−1 and 3.247 × 1043s−1, respectively, and
= 2.577 × 105 J mol−1and 2.814 × 105 J mol−1, respectively [Citation32]. Regarding to tissue damage,
corresponds to a probability of 63% cell death and
corresponds to a probability of 99% cell death. In the study, we defined the
as the threshold of tissue ablation for a higher probability of tumor tissue death and
as the threshold of thermal damage to blood vessels for less thermal damage [Citation24].
Except for the electrical conductivity, thermal conductivity, and blood perfusion rate, other parameters of the tissues pertaining to the model were assumed to be constants, summarized in .
Table 2. Characteristics of the materials used in the theoretical model [Citation32–34].
2.3. Mesh and boundary conditions
A mesh convergence test was performed in the study with the criterion of the difference in the temperature of two points between two contiguous meshes smaller than 0.1%. The two points were chosen at 5 mm away perpendicular to the center of the active tip and 5 mm away along the active tip when electrode is placed at the central position (i.e., = 65 mm,
= 58.5,
= 0
). Eventually, the free tetrahedral elements in a total number of 214091 were used in the model.
The initial temperature of the finite element model of RFA was assumed to be uniform and the same as the internal temperature of the human body
(14)
(14)
Adopting the power delivery method from Ben-David et al. [Citation35], a time-dependent power was applied on the active tip of the electrode in the study
(15)
(15)
where
is the applied power and has an initial value of 1.5 W. The transient variation in
is governed by an impedance-controlled algorithm.
The electrical boundary between the external healthy liver tissue and the electrode cavity was electrically insulated
(16)
(16)
All other boundaries were assumed to be of continuity
(17)
(17)
The thermal boundary condition across the active part of the electrode was
(18)
(18)
where
is the convection heat transfer coefficient and
is the temperature of the internally chilled saline. EquationEquation (18)
(18)
(18) describes the convective heat transfer between the internally chilled saline and the surrounding tissue. In the present study, the flow rate of the internally chilled saline was chosen to be 45 mL min−1, which results in a value of
of 4416.3 W m−2K−1 [34]. The temperature of the internally chilled saline was chosen to be 10 °C. The selected flow rate and temperature combination was sufficient to maintain the temperature along the surface of the active tip around 10 − 15
during RFA. All the other thermal boundary conditions were considered as continuity
(19)
(19)
Then, all outer surfaces of the liver tissue (i.e., the external boundaries) were set as a Dirichlet boundary condition () and a ground terminal (V = 0). Meanwhile, the inlet and outlet boundaries of blood flow were also defined as blood average velocities in the hepatic vascular system, as shown in , and zero pressure boundary (
Pa), respectively.
2.4. Impedance-controlled ablation protocol
The RFA protocol adopted in this study was the optimized impedance-controlled ablation protocol proposed by Ben-David et al. [Citation35], which was introduced as a way of avoiding high impedance of the tissues surrounding the electrode as a result of complete water vaporization of the tissue surrounding the RF electrode (i.e., ‘roll-off’). To maximize the efficiency of energy deposition, the generator algorithm first endeavored to achieve a pre-set power based upon the active tip length over 30 s, and then pulsed the power in response to changes in tissue impedance, as shown in . While the impedance was stable, power was increased in an increment of 5 W every 30 s. When the impedance increased over a pre-set trigger point (i.e., 120 ), the input power was halted for at least 15 s before it was resumed. When impedance rose so rapidly that a power could not be maintained for at least 10 s, the input power was then reduced by 5 W when energy deposition resumed.
2.5. Thermal damage to vessels
The closest edge of thermal damage (i.e., ) to inner vessel walls was always in the cross-section (
) perpendicular to the blood vessel and passing through the electrode. The thermal damage of the vessel wall could be evaluated by
which was the ratio of damaged wall area (
) and the intact wall area (
) in the
as shown in .
(20)
(20)
2.6. Treatment protocol optimization
In this study, RSM was used to do RFA protocol optimization, which was divided into three main steps: (1) to determine optimizing variables, (2) to design experiments, and (3) to build a statistical model for finding optimal results. As mentioned in Introduction, the purpose of this study is to achieve a larger tumor ablation volume for complete ablation of liver tumors and minimal thermal damages to vessels simultaneously by optimizing a few clinically adjustable RFA operating parameters. Therefore, two targets of treatment optimization could be defined: (1) the ablated ratio (), which is the ratio of the ablated tumor volume (
) to the whole tumor volume (
); (2) the thermal damage to vessels
in EquationEquation (20)
(20)
(20) .
(21)
(21)
As shown in , four operating parameters taken as variables were chosen to be optimized, including the ablation time (), insertion position of electrode along X-axis (
), Z-axis (
), and the angle (
) [Citation23–25]. The adjustable range of the ablation time (i.e., 660–780 s) was determined by considering a 12-min RFA protocol for a live tumor of 3 cm diameter in clinic [Citation35]. Therefore, the insertion of RF electrode was determined by the three parameters (i.e.,
and
as shown in ). Typically, the electrode was centered in the tumor for a best match between the predicated ablation zone and tumor, i.e.,
= 65 mm,
= 58.5 mm, and
= 0° in the study [Citation36]. Therefore, we chose this central position of electrode and the clinical ablation time (i.e., 12 min) as a reference protocol. Based on this central position, the range of the
and
for optimization were adopted as 60 − 70 mm, 53.5 − 63.5 mm, and −30° − 30°, respectively. In general, this multi-objective treatment optimization problem can be defined as
(22)
(22)
2.7. Statistical analysis
A commercial statistical software of Minitab (Version 20, Minitab Inc., PA, USA) was used in the study to perform the statistical modeling of RSM, of which Box-behnken method in RSM was chosen to design the experiment matrix for simulations. Based on the simulation results, a statistical predictive model was built by using linear regression. The adjusted coefficient of determination () was used to evaluate the accuracy of model, and each variable in the model were considered as statistical significance at p < 0.05.
3. Results
3.1. Validation of the RFA model
The validation of the RFA model was performed by comparing the temperature results of the simulation and an ex vivo experiment in literature [Citation27]. The geometry and initial conditions of RFA model was adjusted to be consistent with the conditions in the ex vivo experiment. The coolant volume flow rate inside the electrode was set at 25 ml/min and the blood perfusion was ignored in the liver. A good agreement in the temperature at the end of RFA treatment (i.e., 720 s) between the simulation and experiment results was recognized at 5, 10, 15, and 20 mm from the center of RF electrode, as shown in and . Meanwhile, similar ablation diameters also can be found with a minor difference of 2.83% (4.47 mm in the simulation vs. 4.6 ± 0.2 mm in the experiment). A similar acceptable accuracy of the proposed model was also validated by comparing the temperatures at three locations (10, 15, and 30 mm from the center of RF electrode) between the computer model and an
experiment study under a constant power delivery method (i.e., 50 V) for 200 s [Citation37], as illustrated in . All these comparisons support the accuracy of the proposed RFA model as a reasonable testbed for treatment optimization.
Figure 4. (a) the temperature change at 5, 10, 15, and 20 mm from the center of RF electrode, and (b) the temperature distribution at the ending of RFA treatment.
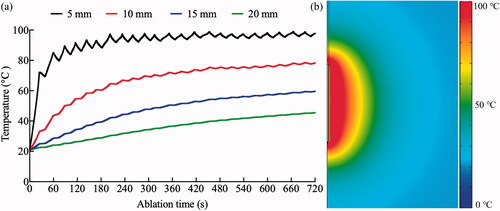
Table 3. Comparison of the temperature between the proposed RFA computer model and the ex vivo experiments.
3.2. RSM results
demonstrates the designed simulation matrix including 25 cases corresponding with the responses (i.e., and
) for four variables (i.e.,
and
), and extra data on the simulation results were provided in Table A1 of Appendix. Meanwhile, based on the simulated results, shows the linear regression results using a full quadratic polynomial model presented in Equation (23).
(23)
(23)
where
represents the responses (i.e.,
or
),
and
are rescaled variables of
and
using min-max normalization method. Generally, two regression results showed a significant influence of four variables on both two responses (both p < 0.001). The influence of linear, square and two-way interaction were considered for each variable in the regression model. The ablation time (
) did not present a significant effect on both
and
in the scope of 660–780 s. Otherwise, other variables has showed the significance on the response of
or
Especially, the significances of
and
were presented in the square part of the regression models for
(p = 0.003 and < 0.001, respectively) and
(p = 0.009 and < 0.001 respectively). Meanwhile, only
and
has a significant effect in the way of two-way interaction (p < 0.001 for
and p = 0.043 for
). In the linear part, the significant effects of
and
are only presented in the response of
with p < 0.001, 0.039, and < 0.001, respectively.
Table 4. Results of ablation and thermal damage for each designed simulation.
Table 5. The value of coefficient in RSM regression model.
3.3. Multi-objective optimization
As shown in , multi-objective optimization was performed (i.e., maximal and minimal
) and the change tendency of
and
along with the variables (i.e.,
and
) were found. A monotonical increase relationship can be observed in the two pairs of
and
and however, a non-monotonic change was found in other pairs. For achieving a maximal
and minimal
an optimal set for all variables was predicted and set as
= 780 s,
= 62 mm,
= 57.8 mm, and
= 3.6°. The predictive optimal results of
and
are 99.0% with a 95% Confidence Interval (CI) of 93.4 − 100% and 0.1% with a 95% CI of 0 − 3.4%, respectively. Correspondingly, the computer model results showed in agreement with the predictive results on
and
using the optimal set, which are 99.6% and 0.4%, respectively, as shown in . The results of the reference protocol are illustrated in with
= 98.1% and
= 4.1%. The demonstrations of the computer model results in other views were also provided in Figures A1 and A2 of Appendix.
Figure 5. The multi-objective optimization results of RSM (the red solid line and blue dashed line represent the optimal solutions and predictive results of the regression models, respectively).
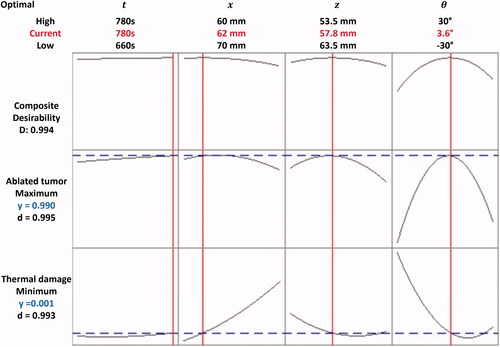
Figure 6. The RFA treatment results in X-Z cross-sectional view: (a–c) ablation, thermal damage, and temperature distribution, respectively for the optimized protocol; (d–f) ablation, thermal damage, and temperature distribution, respectively for the reference protocol. (The black line is the contour line of tumor).
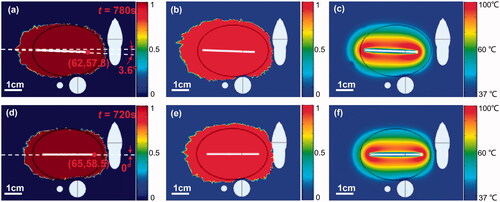
4. Discussion
Using a coupled electric-thermal-fluid RFA model, we demonstrated the feasibility of optimizing a few clinically adjustable RFA operating parameters for a liver tumor in an anatomically sensitive location (tumor abutting a complex vascular structure). As shown in , although a similar change tendency of along with four variables could be observed for
it is difficult to find a consistent variable set for both
and
simultaneously. A possible explanation is the nonuniform distribution of blood vessels in liver compared to the tumor zone, which caused the following two issues for RFA treatment. First, the thermal distribution during RFA treatment was distorted by the heat-sink effect of blood vessels, as shown the temperature distribution in . Thus, it is difficult RFA treatment to generate a uniform ablation zone that matches the tumor shape (i.e., ellipsoid in this study). Second, avoiding thermal damages to the blood vessels is another constraint. Thus, it is difficult for radiologists to find an appropriate RFA treatment protocol, which could ablate the tumor completely and avoid thermal damages to blood vessels for the tumor scenario presented in this study. Instead, what is needed is an optimized protocol plan before RFA treatment. Such a personalized plan is necessary to achieve a more efficient and safe treatment rather than using a constant protocol (such as, the reference protocol).
We believe that the calculation of an optimized protocol could be pursued for each patient, especially for those tumors abutting to major vascular structure. In this study, maximizing the completeness of tumor ablation () while minimizing thermal damage to vessels (
) have been simultaneously achieved by optimizing four clinically adjustable RFA operating parameters, including the ablation time (i.e.,
) and placement of RF electrode (i.e.,
and
) using an engineering optimization method (i.e., RSM). Compared with the reference protocol, the optimized protocol achieved a better simulation result, of which
increased from 98.1% to 99.6% and
decreased from 4.1% to 0.4%. As shown in Figure A3 of Appendix, the optimized protocol successfully avoided the thermal damage penetrating the hepatic vein wall while increasing the ablated tumor volume (See Figure A1 of Appendix). Thus, it is possible for such a liver tumor abutting complex blood vessel structure to achieve a complete tumor ablation without thermal damages to blood vessels by the proposed RFA protocol optimization method, i.e., RSM. The benefit to the patient is a minimally invasive procedure that yields a lower risk of recurrence, while minimizing complications.
In addition, a regression model has been established to predict the relationship between variables and their responses. The optimized protocol agreed nicely with the predicted simulation results. In this study, three conclusions can be made from the RSM regression model ( and ). They are that 1) ablation time () in such range is not a significant variable affecting
and
Two possible reasons are that the thermal equilibrium was reached at the end of RFA treatment [Citation32], and the 2-min range of
chosen in the study is not long enough to introduce a significant influence on ablation results; 2) between the electrode and blood vessel, two distances (
and
) were considered of which
represents the distance along the electrode direction and
represents the distance perpendicular to electrode. A more significant effect for the treatment outcome could be observed in
rather than
In other words, RFA treatment is more sensitive to the blood vessel on the side of the electrode compared with it at the bottom of electrode; and 3) both
and
are very sensitive to insertion angle (
) by which it dominates the direction of ablation zone. It is worth mentioning that the insertion of electrode along with the long axis of tumor is not always the best choice, especially for tumors surrounding the blood vessels, like the one in this study.
However, there are still two limitations with this study needed to be overcome in the future. First, the vascular structure in the study was built based on data from the literature. An image-based 3 D model should provide a more accurate and patient-specific vascular structure. Second, only simulation was performed to validate the efficiency of engineering optimization on enhancing RFA treatment outcome. Future studies of in vivo experiments are warranted for the application of engineering optimization in clinical RFA treatment.
5. Conclusion
The combination of computer modeling and engineering optimization technique can determine a few operating parameters in the process of the ablation of liver tumors abutting complex blood vessel structures. A large tumor ablation with minimal thermal damages to surrounding blood vessels can be achieved simultaneously by using the optimized RFA treatment protocol. The proposed method and finding can contribute to the application of RFA in the treatment of liver tumors in critical anatomical locations.
Supplemental Material
Download PDF (1.7 MB)Disclosure statement
No potential conflict of interest was reported by the author(s).
Additional information
Funding
References
- Lee MW, Kang D, Lim HK, et al. Updated 10-year outcomes of percutaneous radiofrequency ablation as first-line therapy for single hepatocellular carcinoma < 3 cm: emphasis on association of local tumor progression and overall survival. Eur Radiol. 2020;30:733–2400.
- Zhang B, Moser MA, Luo Y, et al. Evaluation of the current radiofrequency ablation systems using axiomatic design theory. Proc Inst Mech Eng H. 2014;228(4):397–408.
- Nour-Eldin N-EA, Exner S, Al-Subhi M, et al. Ablation therapy of non-colorectal cancer lung metastases: retrospective analysis of tumour response post-laser-induced interstitial thermotherapy (LITT), radiofrequency ablation (RFA) and microwave ablation (MWA). Int J Hyperthermia. 2017;33(7):820–829.
- Arrigoni F, Barile A, Zugaro L, et al. CT-guided radiofrequency ablation of spinal osteoblastoma: treatment and long-term follow-up. Int J Hyperthermia. 2018;34(3):321–327.
- Mauri G, Gennaro N, Lee MK, et al. Laser and radiofrequency ablations for benign and malignant thyroid tumors. Int J Hyperthermia. 2019;36(2):13–20.
- Zhang B, Moser MAJ, Zhang EM, et al. Numerical analysis of the relationship between the area of target tissue necrosis and the size of target tissue in liver tumours with pulsed radiofrequency ablation. International Journal of Hyperthermia. 2015;31(7):715–725.
- Xu A, Zhang L, Yuan J, et al. TLR9 agonist enhances radiofrequency ablation-induced CTL responses, leading to the potent inhibition of primary tumor growth and lung metastasis. Cell Mol Immunol. 2019;16(10):820–832.
- Fang Z, Moser MAJ, Zhang EM, et al. A novel method to increase tumor ablation zones with RFA by injecting the cationic polymer solution to tissues: in vivo and computational studies. IEEE Trans. Biomed. Eng. 2020;67(6):1787–1796.
- Lu DSK, Raman SS, Limanond P, et al. Influence of large peritumoral vessels on outcome of radiofrequency ablation of liver tumors. Journal of Vascular and Interventional Radiology. 2003;14(10):1267–1274.
- Poch FGM, Rieder C, Ballhausen H, et al. The vascular cooling effect in hepatic multipolar radiofrequency ablation leads to incomplete ablation ex vivo. Int J Hyperthermia. 2016;32(7):749–756.
- Fang Z, Zhang B, Zhang W. Current solutions for the Heat-Sink effect of blood vessels with radiofrequency ablation: a review and future work. Advanced computational methods in life system modeling and simulation. Singapore: Springer Singapore; 2017. 113–122.
- Zhang B, Moser M, Zhang E, et al. Radiofrequency ablation technique in the treatment of liver tumours: review and future issues. J Med Eng Technol. 2013;37(2):150–159.
- Vaidya N, Baragona M, Lavezzo V, et al. Simulation study of the cooling effect of blood vessels and blood coagulation in hepatic radio-frequency ablation. Int J Hyperthermia. 2021;38(1):95–104.
- Poch FGM, Neizert CA, Gemeinhardt O, et al. Intermittent pringle maneuver may be beneficial for radiofrequency ablations in situations with tumor-vessel proximity. Innovative Surg Sci. 2018;3(4):245–251.
- Kariya Z, Yamakado K, Nakatuka A, et al. Radiofrequency ablation with and without balloon occlusion of the renal artery: an experimental study in porcine kidneys. J Vasc Intervent Radiol. 2003;14(2):241–245.
- Fang Z, Moser MAJ, Zhang E, et al. Design of a novel electrode of radiofrequency ablation for large tumors: in vitro validation and evaluation. Trans ASME, J Biomech Eng. 2019;141(3):031007.
- Fang Z, Zhang B, Moser M, et al. Design of a novel electrode of radiofrequency ablation for large tumors: a finite element study. J Eng Sci Med Diagn Ther. 2018;1(1):011001.
- Haemmerich D, Wright AW, Mahvi DM, et al. Hepatic bipolar radiofrequency ablation creates coagulation zones close to blood vessels: a finite element study. Med Biol Eng Comput. 2003;41(3):317–323.
- Shao YL, Leo HL, Chua KJ. Studying the thermal performance of a bipolar radiofrequency ablation with an improved electrode matrix system: in vitro experiments and modelling. Appl Therm Eng. 2017;116:623–635.
- Zhang B, Moser MAJ, Zhang EM, et al. A review of radiofrequency ablation: Large target tissue necrosis and mathematical modelling. Physica Med. 2016;32(8):961–971.
- Haemmerich D, Schutt DJ. RF ablation at low frequencies for targeted tumor heating: in vitro and computational modeling results. IEEE Trans Biomed Eng. 2011;58(2):404–410.
- Andreozzi A, Brunese L, Iasiello M, et al. Modeling heat transfer in tumors: a review of thermal therapies. Ann Biomed Eng. 2019;47(3):676–693.
- Shao YL, Arjun B, Leo HL, et al. A computational theoretical model for radiofrequency ablation of tumor with complex vascularization. Comput Biol Med. 2017;89:282–292.
- Huang H-W. Influence of blood vessel on the thermal lesion formation during radiofrequency ablation for liver tumors. Med Phys. 2013;40(7):073303.
- González-Suárez A, Trujillo M, Burdío F, et al. Could the heat sink effect of blood flow inside large vessels protect the vessel wall from thermal damage during RF-assisted surgical resection? Med Phys. 2014;41(8):083301.
- Afif AM, Chang JP-E, Wang YY, et al. A sonographic doppler study of the hepatic vein, portal vein and hepatic artery in liver cirrhosis: Correlation of hepatic hemodynamics with clinical child pugh score in Singapore. Ultrasound. 2017;25(4):213–221.
- Goldberg SN, Stein MC, Gazelle GS, et al. Percutaneous radiofrequency tissue ablation: Optimization of Pulsed-Radiofrequency technique to increase coagulation necrosis. J Vasc Intervent Radiol. 1999;10(7):907–916.
- Lindström SB, Uhlin F, Bjarnegård N, et al. Computer-Aided evaluation of blood vessel geometry from acoustic images. J Ultrasound Med. 2018;37(4):1025–1031.
- Pennes HH. Analysis of tissue and arterial blood temperatures in the resting human forearm. J Appl Physiol. 1948;1(2):93–122.
- González-Suárez A, Pérez JJ, Berjano E. Should fluid dynamics be included in computer models of RF cardiac ablation by irrigated-tip electrodes? Biomed Eng Online. 2018;17(1):43.
- Singh S, Melnik R. Thermal ablation of biological tissues in disease treatment: a review of computational models and future directions. Electromagn Biol Med. 2020;39(2):49–88.
- Zhang B, Moser MAJ, Zhang EM, et al. A new approach to feedback control of radiofrequency ablation systems for large coagulation zones. Inter J Hyperthermia. 2017;33(4):367–377.
- Trujillo M, Bon J, José Rivera M, et al. Computer modelling of an impedance-controlled pulsing protocol for RF tumour ablation with a cooled electrode. Int J Hyperthermia. 2016;32(8):931–939.
- Zhang B, Moser MAJ, Zhang EM, et al. Study of the relationship between the target tissue necrosis volume and the target tissue size in liver tumours using two-compartment finite element RFA modelling. Int J Hyperthermia. 2014;30(8):593–602.
- Ben-David E, Nissenbaum I, Gurevich S, et al. Optimization and characterization of a novel internally-cooled radiofrequency ablation system with optimized pulsing algorithm in an ex-vivo bovine liver. Int J Hyperthermia. 2019;36(2):81–88.
- Ewertowska E, Quesada-Diez R, Radosevic A, et al. Computational modeling of radiofrequency ablation with an internally cooled wet electrode. In: Eskola H, Väisänen O, Viik J, editors. Embec & nbc 2017. Singapore: Springer; 2018. p. 1069–1072.
- Haemmerich D. Mathematical modeling of impedance controlled radiofrequency tumor ablation and ex-vivo validation In 2010 Annual International Conference of the IEEE Engineering in Medicine and Biology. IEEE, 2010. p. 1605–1608.