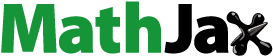
Abstract
Housing credit inflows are a substantial contributor to the economic opportunities and vitality of a community. However, these opportunities have not been dispersed equitably across diverse subpopulations and neighborhoods. Although various public and private initiatives have been launched to address inequitable access to mortgage capital, the role of localized and decentralized housing programs in achieving racially equitable outcomes remains understudied. As a case study, this study examines whether a decentralized and localized housing policy without explicit regulations for resource distribution across racial and ethnic groups promotes racial and neighborhood equity, focusing on its role in serving racial minority households and neighborhoods underserved by private mortgage markets. By incorporating various statistical methods, this study provides evidence that controlling for other factors, the program disproportionately supports racial minority households and neighborhoods that are underserved by private mortgage capital, although this positive role can vary across localities.
Introduction
Housing credit inflows are a substantial contributor to economic opportunity and vitality in a community. Mortgage capital inflows enable residents to purchase and own homes, the main source of personal wealth for the vast majority of American households (Krivo & Kaufman, Citation2004). However, these opportunities have not been dispersed equitably to various subpopulations in many cities. In the United States, neighborhoods whose residents predominantly belong to racial and ethnic (hereafter, racial) minority or low-income groups have witnessed low levels of capital inflow in line with a legacy of racially discriminatory policies (Massey & Denton, Citation1993). This geographically uneven structure of homeownership opportunities has been perpetuated by federal policies that regulated bank lending to neighborhoods with large concentrations of racial minorities in the past (Rothstein, Citation2017). Although progress has been made in reducing racial discrimination in homeownership and lending (Bhutta et al., Citation2022), the elements of this racially discriminatory structure have remained with racially-biased zoning practices, housing and urban policies, and tax incentives, which are designed to support White suburban neighborhoods. Whether the racial composition of neighborhoods still has a role in explaining distributions of federal and local housing funds remains an important equity question in this context.
Numerous studies have demonstrated that private financial institutions have maintained discriminatory practices against Black and Hispanic households (Boehm et al., Citation2006; Gotham, Citation1998; Kau et al., Citation2012; Massey et al., Citation2016; Nagase, Citation2018; Reid, Citation2021; Steil et al., Citation2018). Even after the Fair Housing Act of 1968 outlawed redlining, various implicit types of discriminatory lending behaviors maintained discriminatory patterns in the private mortgage market. Black or Hispanic mortgage applicants still tend to pay relatively high-interest rates compared to non-Hispanic White applicants (Boehm et al., Citation2006; Kau et al., Citation2012; Zou, Citation2014), and the rate of loan rejection tends to be higher for people of color than for Whites (Quillian et al., Citation2020), even after financial characteristics such as income and credit rating are controlled (Bayer et al., Citation2014). These discriminatory practices have exacerbated residential segregation in American metropolitan areas (Dawkins, Citation2004; Galster & Carr, Citation1991), although the racial gap in mortgage denials appears to have lessened in recent years (Bhutta et al., Citation2022).
Discrimination exhibits a spatial history, leads to spatial consequences, and yields spatial patterns (Liu et al., Citation2022; Talen & Anselin, Citation2022). In response to longstanding spatial disparities at the neighborhood level, the federal government issued a series of reforms to reduce lending disparities across neighborhoods with different racial profiles. For example, Congress enacted the 1977 Community Reinvestment Act ‘to end the banking practice known as redlining, by which banks would refuse to lend in certain neighborhoods, especially older and inner-city neighborhoods and neighborhoods whose residents were predominantly minority or low-income’ (Marsico, Citation2005, p. 3). However, accumulated evidence suggests that beyond individual racial and ethnic characteristics, neighborhood-level racial composition continues to be substantially associated with undervalued properties and restricted access to sound mortgage products (Broady et al., Citation2021; Perry et al., Citation2018).
Given that gaps and inequities vary across neighborhood scales in localities, the question of whether equity solutions should be similarly localized emerges. Governing close to the ground may enable more equitable decision-making through a greater understanding of local knowledge and realities (Abimbola et al., Citation2019). Providers of local assistance may tailor resources to the local needs and contexts; formulate appropriate rules for service delivery; change such rules flexibly in response to local circumstances; and have fewer providers in areas of their jurisdiction due to proximity, which may reduce the cost of monitoring and enforcing rules. However, theoretically, the opposite may be true, where decentralization strengthens local discriminatory practices. The literature points out that local discretion often leads local politicians to place fiscal objectives over equity objectives, which may be followed by exclusionary, not equitable, housing and land use policy outcomes (Been et al., Citation2019). Even when housing programs are decentralized from the national or state level to the local level, responsibility for service delivery remains in a limited set of agencies. In such cases, the commitment to equity depends on the decisions of existing power structures within local government bodies, and these powerful actors may overlook concerns of equity and neighborhood racial composition. In fact, decentralization may not be related to the achievement of equity at all or may lead to inequitable outcomes—i.e. frequently supporting White-dominant neighborhoods that presumably have been supported by private mortgage markets. Despite these widely varying possibilities, little research exists regarding whether decentralized and localized housing programs without explicit rules for racial equity promote racial and neighborhood equity and, if the programs are locally specified and implemented, how the role of the programs in addressing equity varies by location.
This case study investigates the role of one such program with decentralized and local administration, Florida’s State Housing Initiatives Partnership (SHIP) program, in addressing racial equity. This study defines ‘equity’ as a policy framework for redistributing resources and transforming historically marginalized subgroups and disinvested communities through reparative investment. Given the historical context of racial segregation enabling disinvestment in racial minority communities, access to homeownership has been restricted in racial minority neighborhoods. Thus, racial equity in distributing resources for housing assistance is operationalized as closing disparities in accessibility to homeownership. Regarding distributive justice standards, we follow Frankfurt’s (Citation1987) and Casal (Citation2007)’s line of thought, arguing that the chance to reach a certain level of adequate conditions should be considered. In their thoughts, equity is defined as every individual (or neighborhood) having access to a standard basic minimum, as opposed to the identical distribution of resources across different people and neighborhoods. The literature highlights the potentially promising consequences of homeownership, including residential and neighborhood stability,—although a few argue that there is a lack of robust empirical evidence supporting those potential impacts of homeownership (Dietz & Haurin, Citation2003; Haurin et al., Citation2002). In this context, we argue that different parts of the city relate to different levels of locational (dis)advantage and opportunity for homeownership, and it is important to pursue distributing resources, in this study SHIP funds, in a way to reduce existing disparities in accessibility to homeownership across different racial groups and neighborhoods.
Specifically, we seek answers to the following research questions: (1) Does the SHIP program promote equity by providing financial benefits to racial and ethnic minority households and neighborhoods? (2) Are the racial and ethnic features of a neighborhood significantly associated with the receipt of SHIP funds, even after controlling for other factors that might influence funding levels? and (3) Does the relationship between a neighborhood’s racial composition and the number of units supported by the SHIP program vary across localities?
To answer these questions, we first conduct descriptive analyses to examine the extent to which the SHIP program benefits racial minority households and neighborhoods—which are defined, in this study, as households represented by non-White applicants and the proportions of non-White households to all households, respectively—that are expected to be underfunded in private mortgage markets. Then, we estimate multivariate models to determine if racial composition is significant in explaining the distributions of SHIP funds and private mortgage capital after controlling for various neighborhood-level characteristics. Finally, we select 12 sample counties and estimate county-specific multivariate models to explore the variation in the relationships between a neighborhood’s racial composition and the number of units supported by the SHIP program. We anticipate the presence of such variation because although the SHIP program may contribute to improving racial and neighborhood equity overall, its role can substantially vary due to its localized nature in designing and implementing the program. This analysis can provide light on the (unintended) role of the SHIP program in promoting racial and neighborhood equity. Florida is diverse enough in terms of demographics and types of jurisdiction and is one of only two states that distribute the majority of state housing trust funds (HTFs) through local governments (Center for Community Change, Citation2016). This study provides unique evidence evaluating the argument that decentralized and localized housing programs can contribute to the promotion of racially equitable access to mortgage capital for underserved communities.
State Housing Initiatives Partnership (SHIP) program, decentralization, and equity
In 1991, Governor Lawton Chiles created a housing workgroup that ultimately recommended that Florida increase its documentary stamp taxFootnote1 on deeds and use additional revenues to fund affordable housing programs. This recommendation served as the basis of the Sadowski Act in 1992, which established a documentary stamp tax and the Local Government Housing Trust Fund (LGHTF). The LGHTF, in turn, funds the SHIP program, under which funds are distributed to local SHIP offices based on population size. The exact size of the trust fund is dependent on the documentary stamp tax collected and the portion of the trust fund allocated by the legislature to affordable housing. In recent years, the SHIP program has received hundreds of millions of dollars. In 2016–17, local governments reported spending $139 million on 7,552 households (Florida Housing Coalition, Citation2019). SHIP is administered in 119 units of jurisdiction, including 52 cities and 67 counties (a few of these jurisdictions pool resources and are administered by centralized agencies). The strategies and priorities through which funds are disbursed are typically established in Local Housing Assistance Plans (LHAPs). Local SHIP personnel then use funds to administer housing programs or SHIP Strategies as the need arises. This system enables broad discretion for local governments to establish their SHIP Strategies and for administrators to decide on the spending of SHIP dollars. Instead of setting the majority of strategies and requirements at the statewide level, which is the tendency of the majority of other state programs, this decentralized system enables local governments to self-assess their housing needs and priorities.
Rather than mandate specific strategies and programs, SHIP sets broad categories for local expenditures that prioritize assistance to low- and moderate-income households, homeownership, and construction. Specifically, SHIP requires 75% of funding to be used for new construction or rehabilitation; at least 30% of all revenue must be allocated to those making 50% area median income (AMI) or less, at least 60% for those making less than 80% AMI (with all funds being spent on households making 140% AMI or below), and 65% for homeownership activities, which includes but is not limited to new homeownership, emergency repairs, and rehabilitation of owner-occupied homes.Footnote2 These two types of requirements (and assistance) are intended to overlap; for example, individual expenditures such as constructing a new house for sale to an owner-occupant can satisfy both the construction/rehabilitation requirement and the AMI limit requirement.
Additionally, the program requires that 20% of the funds distributed to each jurisdiction be used to assist individuals with special needs as defined by Florida statute. Moreover, SHIP assistance funds are governed by price limits that are annually announced by Florida Housing Finance Corporation (FHFC). According to the SHIP Statute 420.9071, those price limits are set as 90% of average purchase price in a county and are applied to either the amounts on the executed sales contract in case of home purchase or appraisal values in case of rehabilitation or emergency repair of an existing unit. As an example, in 2018, the price limit of Miami-Dade County was $317,647. Apart from these basic set-aside strategies, the local government submits a new LHAP every three years, which enables funds to respond to the local conditions. The SHIP program can be used as a stand-alone program, but it is also designed to be leveraged with other sources of affordable housing subsidy, such as Community Development Block Grants and Weatherization assistance programs.
Research suggests that state-administered funds may be well-positioned for the task of promoting racial equity in the flow of mortgage capital. Scally (Citation2008) noted that state housing finance agencies balance financial risk aversion with the objective of addressing low- and moderate-income housing needs, different from profit-motivated private lenders. For example, state-funded mortgage products in Massachusetts outperformed their federal counterparts in terms of equity by providing lower monthly costs and additional financial benefits across the life span of the loan compared with insured mortgages of the Federal Housing Administration (Interrante & Schmiedl, Citation2019). Various studies that aim to compare HTFs across the country have examined the Florida Sadowski HTFs, which finance SHIP and the State Apartment Incentive Loan (Basolo & Scally, Citation2008; Flink et al., Citation2021; Larsen, Citation2004; Scally, Citation2012). However, the literature on state HTFs has focused on income targeting and housing tenure (assistance to renters vs. owners) as indicators of equity instead of race/ethnicity and neighborhoods. Previous studies have found that state trust funds tend to emphasize homeownership assistance for low- and moderate-income households over rental housing development and rent assistance for the poorest households (Larsen, Citation2009; Mueller & Schwartz, Citation2008).
Previous studies of the SHIP program have employed descriptive and qualitative approaches to examine program rules and outcomes (Larsen, Citation1998, Citation2007). For example, Larsen (Citation1998) analyzed the extent to which SHIP accurately targets those in need, the relationship between SHIP administrators and non-profits, the relationship between the LHAPs that govern the spending of SHIP funds, and the actual administration of such funds. However, the role of SHIP funds in advancing equitable access to capital for racial minority households and neighborhoods remains to be examined. Florida does not impose specific requirements for achieving racial equity within the SHIP program. Moreover, for (potential) SHIP-eligible low-income homebuyers, the program does not directly provide mortgage loans and is not intended to counter racial disparities in mortgage lending. Rather, most SHIP assistance to homebuyers is made up of down payment and closing cost assistance and rehabilitation funding.
Theoretically, the decentralization and localization of housing programs could be a powerful tool in promoting racial equity. Designating local officials in sub-state jurisdiction can increase the possibility that local knowledge and expertise will inform policies (Hayek, Citation1945; Musgrave, Citation1961). Decentralization and localization may enable residents to monitor and discipline local officials, which ensures that local public goods and services reflect local preferences (Ostrom et al., Citation1994). This so-called close-to-the-ground mechanism reflects how the close proximity of governance to the locality served (from the transfer of responsibilities to or the existence of responsibilities at local levels) enables the better use of local initiative, information, feedback, input, and control (Abimbola et al., Citation2019, p. 612). From the economic perspective, decentralization reduces potential information asymmetry in relationships between housing assistance providers and individuals with housing needs. This benefit potential, however, is dependent on the assumption that local officials understand local preferences and possess the incentive to respond to such preferences. Furthermore, it is dependent on the assumption that residents are informed about the quantity and quality of services to expect and which levels of public agencies are designated or required to provide such services (Hayek, Citation1945; Musgrave, Citation1961; Ostrom et al., Citation1994). As noted earlier, however, an opposing potential effect of decentralization could be to increase local discriminatory practices driven by a biased administrator or a biased group of influential local citizens. Thus, decentralization may not be related to the achievement of equity at all or may lead to inequitable outcomes—i.e. directing more resources to White-dominant neighborhoods that presumably have been supported by private mortgage markets. In summary, there are gaps in our knowledge about the role of SHIP funds in advancing equitable access to capital, specifically in addressing the housing needs of Black and Hispanic neighborhoods with presumably limited access to capital in private mortgage markets. This research intends to fill the gap in understanding the program’s role in promoting racial equity by supporting (potential) homeowners in predominantly minority neighborhoods.
Data and methods
This study investigates whether SHIP funds are distributed in a racially equitable manner that addresses the gap in housing capital in private markets. The focus of our analysis is to examine whether the relationships of SHIP allocations to race/ethnicity of households and racial composition of neighborhoods differs from those of private mortgage allocations. As a preliminary step in assessing the disparities between neighborhood-level distributions, we measure the number of owner-occupied units in a tract with SHIP support for homeowners (SHIP flows). In this study, we use the Census tract as a proxy for neighborhoods. To develop this measure, we rely on the data gathered by the FHFC, which manages and monitors all SHIP funds across the state, to compile specific information regarding individual SHIP activities. We focus on the period from 2016 to 2018, mainly due to the availability of data. The collected data that cover the period prior to 2016 lack accurate information on purposes of assistance. Specifically, we consider 11 types of support for (potential) homeowners, though downpayment assistance (with or without repairs) was the most prevalent strategy by far (see the details of the strategies for homeowners in footnote 2). After we minimize duplication in raw records and remove records with missing values and errors, all individual SHIP cases are geocoded and aggregated at the tract level.
In total, SHIP data contain 8,641 cases across the state of Florida, for a total amount of funding of roughly 195.5 million USD.Footnote3 On average, each census tract contains approximately two units supported by SHIP assistance for homeowners during the period. In 49% of the state’s 4,245 tracts, one to nine units received SHIP assistance, and in only about 3% of tracts, ten or more units received SHIP assistance. Conversely, nearly half of the tracts (2,024) did not receive any SHIP assistance—primarily due to a lack of needs and qualified applicants. Therefore, we consider this zero-inflated characteristic of the SHIP distribution when we conduct descriptive and multivariate analyses.
As a counterpart to SHIP assistance flows, we measure private mortgage capital flows at the neighborhood level. Specifically, we measure the number of private mortgages originated between 2014 and 2018 (private mortgage flows) by incorporating the Home Mortgage Disclosure Act (HMDA) data. We use the HMDA data for the particular period in order to make private mortgage capital flows appropriately aligned with other datasets used in this study and to increase the overall reliability of the measurement. Numerous studies exploring neighborhood-level mortgage lending patterns, such as high-cost lending before the financial crisis in 2007, have used HMDA data (e.g. Bayer et al., Citation2014; Reid et al., Citation2017; Zou, Citation2015). The data include information on all mortgage applications, such as the type, purpose, and amount of loan, action taken toward the application, the census tract of the property, and income, gender, and race or ethnicity. We restrict the HMDA data to all originated mortgage loans, including conventional and FHA-insured mortgages for home purchase and home improvement.Footnote4 Given the focus of our study on neighborhood-level capital flows, we exclude all refinance cases. In total, 1,291,351 loans were originated across the state. On average, approximately 23 housing units were financed by any mortgage product in each neighborhood. We include these two key variables indicating SHIP and private mortgage flows as dependent variables that reflect the relative degree of neighborhood-level private mortgage capital or SHIP assistance.
We use the 5-year American Community SurveyFootnote5 (ACS) data from 2014 to 2018 to measure various demographic and socioeconomic characteristics at the tract level. Given the limited sample size of the ACS survey and associated measurement errors, we did not consider smaller geographical units than a census tract. Excluding 137 census tracts with missing data on key variables such as owner-occupied housing units, population by race, and median housing, we conducted our analyses based on 4,108 tracts in total.
To examine whether SHIP assistance contributes to neighborhood equity by supporting more housing units in racial minority neighborhoods, we construct four variables to capture tract-level racial composition based on the ACS data: the shares of non-Hispanic Black, Hispanic, non-Hispanic Asian, and non-Hispanic Others.Footnote6 Additionally, we include a set of variables to control for various economic, housing market, sociodemographic, and geographical factors that may influence the distributions of private mortgage capital, SHIP assistance, or both. Since few studies have been conducted on the spatial distribution of SHIP assistance, we select factors based on local knowledge and informal interviews with staff of the Florida Housing Coalition and local SHIP offices.
To control economic conditions, we include a log-transformed median household income variable with the expectation that mortgage lending is less prevalent in tracts with lower median incomes because lenders perceive households to have a high risk of loan rejection and default. We include poverty rates based on the literature suggesting that private mortgage capital is less abundant in high-poverty neighborhoods because they are more subject to housing discrimination (Leven & Sykuta, Citation1994) and concentrated high-cost loans (Lanzerotti, Citation2006). As self-employed individuals may face high loan rejection rates (Munnell et al., Citation1996), we included the share of self-employed working adults with the expectation that private capital may be less available in neighborhoods with high levels of self-employment. In addition, we include the number of banks from Federal Deposit Insurance Corporation data as a proxy for the accessibility to private mortgages. We anticipate that private lending is more prevalent in neighborhoods with a greater number of banks because borrowers have more sources of information regarding mortgage availability (Ergungor, Citation2010; Hyra et al., Citation2013).
To account for housing market-related conditions, we incorporate the log-transformed median housing value as a proxy of collateral available to secure loans, expecting that private mortgage capital may be less prevalent in neighborhoods with low values (Baptiste, Citation1976). Moreover, we include the percentage change in median housing values between the 2009–2013 and 2014–2018 ACS periods, expecting that neighborhoods with recent price increases are likely to attract private mortgage capital. As proxies of market tightness and neighborhood-level stability, we include housing vacancy rates and the share of households living in the same unit as the previous year. To account for the potential size of the market for homeownership, we include the number of owner-occupied housing units.
Additionally, to control for local housing demand in neighboring areas, we include variables indicating the sizes of the following income groups at a county level based on the 2014–2018 Comprehensive Housing Affordability StrategyFootnote7 (CHAS) data: (1) households whose incomes are below 30% of generic HUD-adjusted Median Family Incomes (HAMFI) estimates (extremely low-income or ELI households), (2) households whose incomes are between 30% and 50% of HAMFI (very low-income or VLI households), and (3) households whose incomes are between 50% and 80% of HAMFI (low-income or LI households).Footnote8
To control for a neighborhood’s sociodemographic characteristics that may influence SHIP and private mortgage flows, we include the share of non-citizens. We anticipate that neighborhoods with a high proportion of immigrants tend to have lower homeownership rates because of their brief financial history and limited economic assets in the United States, as well as limited access to SHIP assistance due to their immigration status. We also include the proportion of households with limited English proficiency, expecting that high proportions of limited English households are negatively associated with both private mortgage and SHIP flows because insufficient English skills can be an impediment to homeownership (Borjas, Citation2002; Haurin & Rosenthal, Citation2009; Ratner, Citation1996). We include the median number of persons per household and the share of households with more than one person per room, expecting that large and overcrowded households may experience increased difficulty in securing sufficiently large units that still meet SHIP price limits. Additionally, because many census tracts cross municipal boundaries, residents in different portions of the tract may be eligible for SHIP assistance from multiple city/county SHIP offices. Therefore, a set of dummy variables is included to control for potential effects originating from the administrative/political position of a neighborhood.Footnote9 Finally, we include a set of county-specific dummy variables to account for potential county-specific implications on the SHIP and private mortgage flows. The descriptive statistics of key independent variables are presented in Appendix A of the Supplemental Material.
We examine the first research question, using descriptive analysis to determine whether the SHIP program frequently serves racial minority households that are anticipated to be underfunded in private mortgage markets. Given the SHIP program’s emphasis on allocating funds by household income level, we also compare the racial composition of SHIP recipients and private mortgage recipients within various income levels. The SHIP and private mortgage recipients are subdivided into ELI, VLI, and LI households, and we examine whether racial minority households are likely to receive more SHIP funds in each AMI-based group than their proportions in private mortgage flows. We collect the information regarding AMI-based groupings of SHIP recipients from FHFC data, whereas we uniquely conduct AMI-based grouping for private mortgage recipients by using location and household size information from HMDA data. As additional reference groups, we consider the racial composition of all low-income households in Florida and low-income homeowners in Florida, both of which work as reference groups and are derived from 2014–2018 CHAS data.
At a neighborhood level, to examine whether the SHIP program frequently supports racial minority neighborhoods that are anticipated to be underfunded in private mortgage markets, we descriptively analyze two measures: (1) the number of units supported by the SHIP program within a tract and (2) the proportion of SHIP-supported units to all owner-occupied units. We then compare the two SHIP variables with corresponding variables measuring the quantity and the proportion of private mortgages originating in each tract across neighborhoods classified by racial composition. We categorize neighborhoods into the following five groups: (1) predominantly White (population >80% non-Hispanic White), (2) mainly White (population 50–80% non-Hispanic White), (3) predominantly Black (population >50% Black), (4) predominantly Hispanic (population >50% Hispanic), and (5) multiethnic neighborhoods (population >50% racial and ethnic minority that are not predominantly Black and Hispanic neighborhoods). We compare the numbers and the proportions of units supported by the SHIP program and originated private mortgages across the five types of neighborhoods.
We examine the second research question, whether the variables indicating the racial composition of a neighborhood are significantly associated with SHIP flows even after controlling for other relevant conditions by incorporating two multivariate approaches. Given the zero-inflated nature of SHIP fund distribution, we conduct a series of tests for model specification (e.g. checking the presence of over-dispersion) and confirm that zero-inflated negative binomial (ZINB) models are the most suitable for the models that explain the number of SHIP-supported units. For the two parts of a ZINB model—which consists of a binary logit model to model the process of the zero values and a negative binomial model to model the counting process—we first use the proportion of units under SHIP price limits to model the process of zero outcomes, and we include all previously introduced independent variables to model the counting process. We estimate the share of housing units with values in the ACS below the SHIP price limits (Appendix B in the Supplemental Material presents the details of SHIP price limits). Since the ACS data only provide the number of housing units in certain price ranges; thus, we use linear interpolation to estimate the number of houses falling under precise SHIP price limits. To construct the models for private mortgage flows, we incorporate the negative binomial (NB) approach due to over-dispersion and include the set of independent variables introduced above. All ZINB and NB models are estimated with robust errors to make them robust to the heteroskedasticity of the errors.
To examine the third research question, we select 12 large counties and estimate county-specific multivariate models to explore the variability in the relationships between a neighborhood’s racial composition and the number of units supported by the SHIP program. We confirm that ZINB models are appropriate and include the same set of independent variables.
Results
To begin, we examine the racial compositions of SHIP and private mortgage flows at the application level for various low- and moderate-income groups (see ). First, generally speaking, the distribution of private mortgage flows among the various racial groups substantially differs from the racial composition of low-income households in Florida. Specifically, approximately 64–69% of private mortgage flows are for low-income White households, 11–13% of them are for low-income Black households, and 13–20% of them are for Hispanic households, whereas low-income Black and Hispanic households cover 16–22% and 24–28% of low-income groups, respectively. This discrepancy suggests that despite the size of low-income Black and Hispanic populations, a relatively small number of private mortgages originated with them.
Table 1. Racial Compositions of SHIP and Private Mortgage Flows across Different Low-Income Subgroups.
Second, probably more important, the SHIP program frequently benefits Black low-income households, especially ELI Black households, even more than White low-income households that make up the majority of the low-income population in Florida. Among ELI households, 47% of SHIP recipients are Black households, yet just 13% of originated private mortgages are for Black households. Given that Black households represent 22% and 13% of all low-income households and low-income homeowners in Florida, this tendency is noteworthy. Similarly, approximately 39% of LI households are Black households, while just 11% of originated private mortgages are for LI Black households. This substantially high share of Black households is evident in the income bracket between 80 and 120 AMI as well.
Third, in contrast to Black households, Hispanic households indicate substantially lower proportions of SHIP and private mortgage flows, especially ELI Hispanic households. Only 11% of ELI SHIP recipients are Hispanic, and 13% of private mortgages for ELI households are for Hispanic households. This is unexpected, considering that Hispanic households represent 28% and 21% of ELI households and homeowners in Florida. Moreover, despite the fact that these disparities tend to diminish among LI households, it is evident that Hispanic households receive less SHIP and private mortgage capital than other racial groups.
Fourth, not surprisingly, Asian and other racial minority groups make up a small part of the entire SHIP and private mortgage recipients. Asian households indicate small proportions of SHIP flows and large proportions of private mortgages relative to their share of low-income households. In contrast, other racial minority groups indicate large SHIP and private mortgage proportions relative to their share of low-income households.
Fifth, although the number of SHIP-supported households is far lower than the number of private mortgage recipients, the magnitude of the disparity is substantially narrower for lower-income racial minorities, especially Black households. For instance, the total number of SHIP-supported LI households (n = 3,893) represents only 1.83% of all LI private mortgage recipients (n = 213,240) and 0.54% of total LI homeowners (n = 722,945). However, among ELI Black households, the number of SHIP-supported households (n = 613) represents 52.21% of all private mortgage recipients (n = 1,174) and 1.27% of all homeowners (n = 48,240). This suggests that the number of SHIP-supported households is substantially small in comparison to the magnitude of private loans, but the program contributes to racial equity by providing various types of assistance for homeownership.Footnote10
The descriptive findings above suggest that SHIP funds flow variedly into different racial groups among low-income households at an applicant level. Given the study’s focus on the aggregated neighborhood-level flows of SHIP funds and private mortgage capital, we descriptively analyze the aggregated those flows categorized by a neighborhood’s racial composition. displays descriptive statistics of several key variables reflecting neighborhood-level patterns of SHIP and private mortgage. Generally, predominantly Black neighborhoods tend to receive more SHIP funds and receive fewer private mortgages than predominantly and mainly White neighborhoods. In contrast, Hispanic-dominant neighborhoods tend to receive fewer SHIP funds and private mortgages than mainly White neighborhoods. Furthermore, multiethnic neighborhoods—where more than half of their populations are a racial and ethnic minority, but neighborhoods are not categorized as predominantly Black or Hispanic neighborhoods—tend to receive more SHIP funds than predominantly and mainly White neighborhoods. Despite the inconsistent distribution of SHIP funds across neighborhoods, the number of SHIP-supported units at a neighborhood level is extremely low in comparison to the number of originated private mortgages; the ratio of the number of SHIP-supported units to the number of mortgages is only approximately 1–5%. Because nearly half of the neighborhoods did not receive any SHIP assistance during the study period, we examine neighborhood-level patterns based on neighborhoods with at least one unit supported by the SHIP program. As displays, the aforementioned findings hold constant when neighborhoods with no SHIP assistance are excluded. Finally, the descriptive analysis shows that while the presence of SHIP assistance varies across neighborhoods, the overall number of SHIP-supported units at a neighborhood level is deficient in comparison to the number of originated private mortgages. However, for particular subgroups, such as the lowest-income Black homebuyers, SHIP’s presence is more substantial.
Table 2. Descriptive Statistics of SHIP and Private Mortgage Flows across Neighborhoods with Different Characteristics of Racial Composition.
To examine whether these descriptive findings are consistent after controlling for various factors that might influence SHIP and private mortgage flows, we estimate a series of multivariate models and present the results in . Without any control variable, the proportions of Black and other racial minority households are positively associated with SHIP flows, whereas the proportions of Hispanic and Asian households are negatively associated with the flows, consistent with the descriptive findings above. However, after controlling for a set of factors, the sign of the estimated coefficient for the share of Hispanic households changes to positive. Considering that this positive relationship between SHIP flows and the Hispanic share is significant after controlling for all county-specific effects, it implies that neighborhoods with higher proportions of Hispanic households are likely to indicate a higher number of SHIP-supported units within a county. The estimated relationship between the proportion of Black households and SHIP flows becomes marginally stronger. In addition to racial composition, the model indicates that SHIP flows are likely to be high in neighborhoods with the following conditions: low median household incomes, low proportion of self-employed households, high number of owner-occupied units, low median housing value, high housing price changes, low proportion of non-citizens, and high median household size.
Table 3. The Results of Multivariate Models that Explain SHIP and Private Mortgage Flows.
In the case of private mortgage flows, both the proportions of Black and Hispanic households are negatively associated with private mortgage flows, which is consistent with previous studies highlighting low mortgage origination rates among Black and Hispanic households (Dymski, Citation2009; Munnell et al., Citation1996). In contrast, the proportions of Asian and other racial minority households are positively associated with the flows. After including a set of control variables, we find that the sign of the estimated coefficient for the share of Hispanic households changes to positive, suggesting that the number of units supported by private mortgage capital increases by 0.003 units for every percentage point increase in the Hispanic share. In addition to racial composition, the model indicates that private mortgage flows are likely to be high in neighborhoods with a high number of banks, a low proportion of self-employed households, low poverty rates, a high number of owner-occupied units, a high median housing value, a low degree of neighborhood-level stability, a high housing price change, a low proportion of overcrowded households, a high median household size, and a low share of households with limited English proficiency.
One limitation of estimating models separately for SHIP and private mortgage flows is that the results do not directly answer the question of whether SHIP flows go to neighborhoods underserved by private mortgage capital—though the results indirectly imply that this is the case. Because the two flows are not analyzed together, it is challenging to determine whether SHIP assistance fills the gap in underfunded neighborhoods in private mortgage markets. Thus, as a supplementary analysis, we incorporate the location quotient (LQ) approach and classify all neighborhoods into the following four groups: (1) neighborhoods where both private mortgage and SHIP flows are relatively higher than the average rates in the county and where the tract is located (high-mortgage and high-SHIP neighborhoods, N = 476), (2) neighborhoods where private mortgage flows are relatively higher, and SHIP flows are relatively lower than the average rates in the county (high-mortgage and low-SHIP neighborhoods, N = 1,187), (3) neighborhoods where private mortgage flows are relatively lower, and SHIP flows are relatively higher than the average rates in the county (low-mortgage and high-SHIP neighborhoods, N = 893), and (4) neighborhoods where both private mortgage and SHIP flows are relatively lower than the average rates in the county (low-mortgage and low-SHIP neighborhoods, N = 1,552). Appendix C in the Supplemental Material provides the classification method in detail. Based on these categories, we estimate multinomial logistic models to examine the relationship between a neighborhood’s racial composition and the likelihood of belonging to these four groups with the same set of control variables used in our previous analysis. We primarily focus on comparing the associations between racial composition and the possibility of being in low-mortgage and high-SHIP neighborhoods.
displays the outcomes of the multinomial logistic regression models. The results reveal that neighborhoods with high proportions of Black households tend to be low-mortgage and high-SHIP neighborhoods after controlling for a neighborhood’s economic and social characteristics. Especially among neighborhoods with relatively low private mortgage flows, reflecting being underserved by private mortgage capital, the probability of belonging to low-mortgage and high-SHIP neighborhoods increases as the share of Hispanic households increases. These results confirm that SHIP funds are frequently allocated to Black and Hispanic neighborhoods underserved in private mortgage markets.
Table 4. The Results of Multinomial Logistic Regression Models based on County-Based LQ Index Values.
Although the previous findings reveal the overall associations between racial composition and SHIP flows, given the localized nature of the SHIP program, the statewide estimates can mask considerable heterogeneity in the associations between a neighborhood’s racial composition and SHIP flows in different localities. Thus, we estimate a series of county-specific models to explore the presence of variation in the relationships between racial composition and SHIP flows. presents the estimated coefficients of racial composition variables for the largest 12 counties in Florida. In many counties, for example, Palm Beach (approximately 18.1% of the Black population) and Miami-Dade (16.1%) Counties, the proportion of Black households is strongly associated with SHIP flows. In contrast, a few counties, such as Brevard (9.6%), Pasco (5.1%), and Volusia (10.3%) Counties, indicate insignificant relationships between the share of Black households and SHIP flows. Interestingly, the proportion of Hispanic households is positively and significantly associated with SHIP flows in the majority of the selected counties, such as Pasco (approximately 14.5% of the Hispanic population) and Palm Beach (21.9%) Counties. However, in some counties, the proportion of Hispanic households is either insignificant, for example, in Pinellas (9.4%) County, or is negatively associated with SHIP flows, for example, in Brevard (10.0%) County. The results imply that in counties with low proportions of a particular racial group, the relationships between SHIP flows and shares of Black or Hispanic households are likely to be either insignificant or negative. Although this pattern should be further empirically examined, the role of the SHIP program in promoting neighborhood equity might differ across localities depending on the overall representation of a particular racial group within a county, which may contribute to formulating different local policy demands for SHIP funds. One possible explanation is that areas with higher proportions of racial minorities could be more likely to have racial minority representation in the Affordable Housing Advisory Committees (AHACs), which typically help advise LHAPs and have members representing different housing stakeholders/groups. This racial minority representation might lead to LHAPS having SHIP strategies or incentives that better address racial equity issues. It might also lead to local SHIP offices having more partnerships with minority-serving organizations within and outside AHACs. Between SHIP administrators working with more representative local organizations and having a more representative AHAC, SHIP funds might have a greater reach in advertising programs, generate more trust between residents and the SHIP office, and have more racially diverse applicants and beneficiaries, all of which may lead to more equitable outcomes.
Table 5. The Results of County-Specific Models that Explain SHIP Flows.
In order to explore this county-to-county variation in the associations between a neighborhood’s racial composition and SHIP flows further, we conducted a supplementary investigation into the characteristics of local SHIP offices in the ten largest counties. Although these findings are exploratory and should be further examined, we find that the administrative capacity of local SHIP offices and staff’s perceptions of racial segregation of their communities and racial equity, in general, may be crucial to achieving racially equitable outcomes through SHIP assistance (i.e. greater SHIP flows to racial minority neighborhoods). Specifically, we find that counties with high-capacity SHIP offices tend to exhibit intense and positive associations between SHIP flows and shares of Black or Hispanic households; in this case, ‘high capacity’ is indicated by a larger staff, a larger volume of applications per year, and multiple online and offline channels to access funding applications. On the other hand, counties with local SHIP offices that frequently alter their priorities in response to changing community needs do not indicate strong and significant relationships between SHIP flows and racial minority composition. In addition, other factors did not show any noticeable differences, including the presence of language services for non-English speakers and staff turnover rates. Lastly, we observed that perceptions of racial/economic segregation in the communities of local SHIP officers vary significantly, even between local SHIP offices within the same county.
Discussion and conclusions
Taking the SHIP program as a case study, we investigate whether a decentralized and localized housing policy can promote racial equity, focusing on its role in serving racial minority households and neighborhoods underserved by private mortgage markets. By incorporating various descriptive and multivariate modeling approaches, this study provides evidence that although the SHIP program does not have any explicit regulations for resource distribution across racial and ethnic groups, the program frequently supports racial and ethnic households and neighborhoods that are underserved by private mortgage capital. Our descriptive analyses corroborate that SHIP funds disproportionately support low-income racial minority households and neighborhoods. In addition, SHIP assistance is small enough in magnitude that it will have only modest overall effects on financial benefits to racial minority neighborhoods in general. However, the magnitude may be sufficient to lower the barriers to homeownership at an applicant’s level for a particular subgroup defined by income levels and race.
Our multivariate models that analyze the relationships between a neighborhood’s racial composition, SHIP flows, and private mortgage flows after controlling for other conditions confirm this contribution of the SHIP program to racial and neighborhood equity. These findings support that the policy design of providing more discretion to local governments, even without explicit regulations for resource distribution across racial and ethnic groups, can effectively achieve racially equitable distribution, at least in terms of the distribution of funds within a county.
Significantly, the county-level analysis reveals more nuanced results on whether the localized policy design of SHIP produces racially equitable outcomes. In many counties, SHIP functions to support Black and Hispanic neighborhoods. However, in a few counties, such positive relationships substantially vary in terms of magnitude and, in some instances, direction. These differences reflect the varying roles of the decentralized housing policy designs. Therefore, further investigation should be conducted on which local conditions of housing programs lead to racially equitable outcomes.
The study presents several important implications for housing policies. Although various policy efforts have been made to improve racial and neighborhood equity in private mortgage markets, mortgage capital flows remain restricted in racial minority neighborhoods, especially in neighborhoods with a high proportion of Black households. According to 2021 ACS data, nationally White homeownership rates indicate 73%, whereas Black homeownership rates remain at 44%. Our results consistently indicate that Black neighborhoods and Black low-income households are much less likely to receive mortgages, which reflects constrained access to capital. At a household level, the results indicate that the income limits of SHIP funds (i.e. targeting low and moderate-income households) play a significant role in achieving equitable outcomes, particularly for Black and Hispanic households, whose median incomes tend to be lower. One interesting finding is that such a linear relationship, which reflects the degree of the gap in housing capital, is not observed for Hispanic low-income households and neighborhoods. This finding may be due to the economic heterogeneity among predominantly Hispanic neighborhoods. As a supplementary analysis, we explore the patterns of median family income levels across neighborhoods categorized by racial composition (see Appendix D in the Supplemental Material). Unlike predominantly White neighborhoods, which have concentrations of moderate-income levels, and predominantly Black neighborhoods, which show concentrations of low-income levels, predominantly Hispanic neighborhoods demonstrate a bimodal distribution in which low- and moderate-income groups coexist. Moreover, the concentration of moderate-income households in multiethnic neighborhoods mirrors the nuanced relationships between private mortgage and SHIP flows in neighborhoods with high proportions of Hispanic households. These heterogeneous economic conditions of predominantly Hispanic neighborhoods suggest that a detailed understanding of local characteristics and contexts around a specific minority neighborhood is necessary.
Although this study does not provide detailed mechanisms, which is a major limitation, a possible explanation for the promising outcomes of SHIP assistance is the proximity of local SHIP offices to localities. As the literature points out, experts in local SHIP offices are knowledgeable about local contexts, especially disadvantages that may have been imposed on racial minority neighborhoods over time. Theoretically, proximity to localities may lead to various mechanisms to direct resources to underserved minority neighborhoods, such as tailoring resources to local needs, formulating appropriate local rules for service delivery, changing these rules flexibly in response to local circumstances, designating fewer providers over areas of jurisdiction, and reducing the cost of monitoring and enforcing rules. Another possible reason is that SHIP assistance lacks a profit motive and that the local SHIP offices may practice different cultures or atmospheres as not-for-profit-oriented agencies compared with private banks. Another explanation is that local SHIP offices may employ different strategies depending on the amount of available SHIP funds and their local contexts. The information obtained from local SHIP officers reveals that some communities with larger SHIP allocations (i.e. populous communities) tend to invest the funds in development-type initiatives that target specific areas. Smaller communities, in contrast, tend to concentrate on individualized downpayment and rehabilitation assistance. These different ways to use SHIP funds may result in increased or decreased SHIP expenditures for racial minority households and neighborhoods. Among these mechanisms, the one that is the most critical to achieving racially equitable outcomes should be further examined in the future using empirical data on the characteristics of local SHIP offices. Moreover, our supplementary investigation suggests that administrative capacity (e.g. the numbers of staff or applications), accessibility of processes to those with housing needs, frequency of updating priorities of SHIP funding, and perceived racial segregation in a community may work to support racial minority applicants and neighborhoods. These potential explanations need further empirical examination.
Future studies could identify detailed mechanisms if administrative capacities and governance characteristics are measured in a systematic way using interviews or surveys on local SHIP offices and if these factors are included in analytic models. Moreover, future studies could incorporate more in-depth qualitative information and comparison with other programs to identify administrative mechanisms that promote equity. Specifically, researchers could compare policy outcomes between SHIP and state-administered housing initiatives, such as Florida Housing Finance Corporation’s statewide first-time homebuyer assistance, to examine whether such racially equitable outcomes are due to the work culture of local SHIP offices. In this manner, studies could better identify whether factors such as proximity to local actors or the work culture of SHIP offices are critical for promoting the racially equitable consequences of SHIP. Similarly, this study focuses on neighborhoods in Florida, so the findings related to the positive role of SHIP may be limited in generalizability and provide limited implications to other regions with different contexts. Thus, investigation of other areas that use state-local hybrid programs, such as Iowa’s housing program, can provide a further understanding of localized housing programs and their outcomes.
Supplemental Material
Download PDF (564.1 KB)Disclosure statement
No potential conflict of interest was reported by the author(s).
Additional information
Funding
Notes
1 Documentary stamp tax is an excise tax levied on documents (e.g. deeds, mortgages, and promissory notes) executed, delivered, or recorded in Florida.
2 SHIP assistance for homeowners, for example, can include a wide variety of locally selected strategies: (1) purchase assistance with rehabilitation, (2) purchase assistance without rehabilitation, (3) owner-occupied rehabilitation, (4) demolition and reconstruction, (5) disaster assistance referring to providing funds for immediate activities during/after a disaster or emergency, (6) emergency repair, referring to providing funds for a specific list of items such as windows, roofing and so on, (7) foreclosure prevention, (8) impact fees, covering the fees that are required to be paid prior to purchase, (9) acquisition and rehabilitation, (10) new construction, and (11) special needs including activities not eligible under any other strategy.
3 Although we have information on detailed dollar amounts for each SHIP activity, we did not use the information due to potential statistical problems caused by putting too much weight on a few extremely large SHIP disbursements. In the raw SHIP data, the funding amount varies from $52 to $272,185, with average and median values of $18,215 and $10,000, respectively. Focusing on the number of supported units may be insufficient for accurately capturing the amount of capital that flows into a certain neighborhood. However, we assume that the needs of applicants determine the amount of SHIP funds for each application.
4 Some may argue that HMDA data may include high-cost mortgages (also called subprime mortgages or, recently, nonprime mortgages), usually granted to households with poor credit scores that are not qualified for conventional mortgages. Typically, these high-cost mortgages charge interest rates above the prime lending rate, and they may not be viewed as decent opportunities for homeownership. However, after the housing bubble burst in 2010, subprime mortgage products or predatory lending practices have been subject to regulation, it has been almost impossible for someone with a credit score below 600 to obtain a home loan. Thus, given historically low mortgage interest rates during the study period, we assume that most originated loans were provided to qualified applicants, and even if there were high-cost mortgages, their roles were marginal. Also, as many previous studies analyzing HMDA data pointed out (e.g. Ergungor, Citation2010; Lanzerotti, Citation2006; Zonta, Citation2012), HMDA data do not provide credit scores such as FICO scores, which is a critical factor that explains the possibility of mortgage originations at an individual application level as well as at a neighborhood level. Therefore, potential errors may exist in our estimated coefficients and they should be interpreted descriptively.
5 American Community Survey (ACS) is the largest nationwide survey conducted by U.S. Census Bureau. It collects various information, particularly related to demographic, social, economic, and housing characteristics.
6 Hereafter referred to as “Black,” “Hispanic,” “Asian,” and “Other” respectively.
7 Comprehensive Housing Affordability Strategy (CHAS) data provide special tabulations generated from the American Community Survey (ACS) and HUD-adjusted Median Family Incomes (HAMFI) that enable analysis of specific housing needs and housing affordability issues.
8 The HUD-adjusted Median Family Incomes (HAMFI) estimates are conceptually similar to HUD’s area median income (AMI) estimates to check program eligibility. The main difference between HAMFI and AMI estimates is that the AMI estimates are trended forward to the fiscal year in which they are effective, whereas HAMFIs are calculated retrospectively (Joice, Citation2014).
9 We categorize all census tracts in Florida into the following groups using GIS: (1) when all areas in a tract are included in a specific local municipality (completely within a city, N = 652), (2) when only a part of a tract is included in a specific municipality and the remaining part is in its surrounding county (intersect with a city, N = 1,480), (3) when a tract is located across two or more municipalities (intersect with more than one city, N = 848), and (4) when no part in the tract is in a specific municipality (intersect with no city, N = 1,128).
10 It is important to note that a ratio of the number of homeowners to mortgage recipients during our study period is substantially high for LI households, compared with the ratio for ELI households. Although there should be further examination on this disparity, we guess that most Black ELI homeowners are elderly people who already paid off their homes and are living off retirement or Social Security.
References
- Abimbola, S., Baatiema, L. & Bigdeli, M. (2019) The impacts of decentralization on health system equity, efficiency and resilience: A realist synthesis of the evidence, Health Policy and Planning, 34, pp. 605–617.
- Baptiste, K. E. (1976) Attacking the urban redlining problem, Boston University Law Review, 56, pp. 989–1019.
- Basolo, V. & Scally, C. P. (2008) State innovations in affordable housing policy: Lessons from California and New Jersey, Housing Policy Debate, 19, pp. 741–774.
- Bayer, P., Ross, S. L. & Bayer, P. (2014). Race, ethnicity and high-cost mortgage lending, National Bureau of Economic Research, No. w20762.
- Been, V., Ellen, I. G. & O’Regan, K. (2019) Supply skepticism: Housing supply and affordability, Housing Policy Debate, 29, pp. 25–40.
- Bhutta, N., Hizmo, A. & Ringo, D. (2022). How Much Does Racial Bias Affect Mortgage Lending? Evidence from Human and Algorithmic Credit Decisions. In FEDS Working Paper No. 2022-67. doi: https://doi.org/10.2139/ssrn.3887663
- Boehm, T. P., Thistle, P. D. & Schlottmann, A. (2006) Rates and race: An analysis of racial disparities in mortgage rates, Housing Policy Debate, 17, pp. 109–149.
- Borjas, G. J. (2002) Homeownership in the immigrant population, Journal of Urban Economics, 52, pp. 448–476.
- Broady, K., McComas, M. & Ouazard, A. (2021) An analysis of financial institutions in Black-majority communities: Black borrowers and depositors face considerable challenges in accessing banking services. https://www.brookings.edu/research/an-analysis-of-financial-institutions-in-black-majority-communities-black-borrowers-and-depositors-face-considerable-challenges-in-accessing-banking-services/.
- Casal, P. (2007) Why sufficiency is not enough, Ethics, 117(2), pp. 296–326. doi: https://doi.org/10.1086/510692
- Center for Community Change. (2016) Opening Doors to Homes for All: The 2016 Housing Trust Fund Survey Report.
- Dawkins, C. J. (2004) Recent evidence on the continuing causes of Black-White residential segregation, Journal of Urban Affairs, 26, pp. 379–400.
- Dietz, R. D. & Haurin, D. R. (2003) The social and private micro-level consequences of homeownership, Journal of Urban Economics, 54, pp. 401–450. https://doi.org/10.1016/S0094-1190(03)00080-9.
- Dymski, G. A. (2009) Afterword: Mortgage markets and the urban problematic in the global transition, International Journal of Urban and Regional Research, 33, pp. 427–442.
- Ergungor, O. E. (2010) Bank branch presence and access to credit in low- to moderate-income neighborhoods, Journal of Money, Credit and Banking, 42, pp. 1321–1349.
- Flink, C., Wlter, R. J. & Xu, X. (2021) Policy diffusion in a redistributive policy: Affordable housing and state housing trust funds, State and Local Government Review, 53(3), pp. 187–209. https://doi.org/10.1177/0160323X211049420.
- Florida Housing Coalition. (2019). Guidebook for SHIP Administrators (Florida: Florida Housing Coalition).
- Frankfurt, H. (1987) Equality as a moral ideal, Ethics, 98, pp. 21–43. http://www.jstor.org/stable/2381290
- Galster, G. & Carr, J. (1991) Housing discrimination and urban poverty of African-Americans, Journal of Housing Research, 2, pp. 87–123.
- Gotham, K. F. (1998) Race, mortgage lending and loan rejections in a U.S. city, Sociological Focus, 31, pp. 391–405.
- Haurin, D. R., Dietz, R. D. & Weinberg, B. A. (2002) The impact of neighborhood homeownership rates: A review of the theoretical and empirical literature, Journal of Housing Research, 13, pp. 119–151. http://www.jstor.org/stable/44944780.
- Haurin, D. R. & Rosenthal, S. S. (2009) Language, agglomeration and Hispanic homeownership, Real Estate Economics, 37, pp. 155–183.
- Hayek, F. A. von. (1945) The Use of Knowledge in Society (Menlo Park: Institute for Humane Studies).
- Hyra, D. S., Squires, G. D., Renner, R. N. & Kirk, D. S. (2013) Metropolitan segregation and the subprime lending crisis, Housing Policy Debate, 23, pp. 177–198.
- Interrante, J. & Schmiedl, E. (2019) Building wealth through homeownership: A comparative study of MHP’s ONE mortgage program and FHA. https://www.jchs.harvard.edu/research-areas/working-papers/building-wealth-through-homeownership-comparative-study-mhp's-one.
- Joice, P. (2014) Measuring housing affordability, Cityscape: A Journal of Policy Development and Research, 16, pp. 299–307.
- Kau, J. B., Keenan, D. C. & Munneke, H. J. (2012) Racial discrimination and mortgage lending, The Journal of Real Estate Finance and Economics, 45, pp. 289–304.
- Krivo, L. J. & Kaufman, R. L. (2004) housing and wealth inequality: Racial-ethnic differences in home equity in the United States, Demography, 41, pp. 585–605. http://proxy.lib.ohio-state.edu/login?url=http://search.ebscohost.com/login.aspx?direct=true&db=bth&AN=14421235&site=ehost-live
- Lanzerotti, L. (2006). Homeownership at High Cost: Foreclosure Risk and High Cost Loans in California (No. 2006–1; Issue July).
- Larsen, K. (1998) Revitalizing the parramore heritage renovation area: Florida’s state housing initiatives partnership program and orlando’s historic African-American community, Housing Policy Debate, 9, pp. 595–630.
- Larsen, K. (2007) Housing opportunities in Florida: The state housing trust fund, Journal of Land Use & Environmental Law, 23, pp. 161–176.
- Larsen, K. (2009) Reassessing state housing trust funds: Results of a Florida survey, Housing Studies, 24, pp. 173–201.
- Larsen, K. (2004) State housing trust funds in the U.S.: A comparative study, Adequate & Affordable Housing for All, pp. 1–10.
- Leven, C. L. & Sykuta, M. E. (1994) The importance of race in home mortgage loan approvals, Urban Affairs Quarterly, 29, pp. 479–489.
- Liu, B., Tian, Y., Guo, M., Tran, D., Alwah, A. A. Q. & Xu, D. (2022) Evaluating the disparity between supply and demand of park green space using a multi-dimensional spatial equity evaluation framework, Cities, 121, pp. 103484.
- Marsico, R. (2005) Democratizing Capital: The History, Law, and Reform of the Community Reinvestment Act. https://papers.ssrn.com/sol3/papers.cfm?abstract_id=651482.
- Massey, D. S. & Denton, N. A. (1993) American Apartheid: Segregation and the Making of the Underclass.
- Massey, D. S., Rugh, J. S., Steil, J. P. & Albright, L. (2016) Riding the stagecoach to hell: A qualitative analysis of racial discrimination in mortgage lending, City & Community, 15, pp. 118–136.
- Mueller, E. J. & Schwartz, A. (2008) Reversing the tide: Will state and local governments house the poor as federal direct subsidies decline?, Journal of the American Planning Association, 74, pp. 122–135.
- Munnell, A. H., Tootell, G. M. B., Browne, L. E. & McEneaney, J. (1996) Mortgage lending in Boston: Interpreting HMDA data, The American Economic Review, 86, pp. 25–53.
- Musgrave, R. A. (1961) The Theory of Public Finance: A Study in Public Economy (New York: McGraw-Hill).
- Nagase, D. (2018) Spatial Differences in Flows and Costs of Residential Mortgage Capital during Boom and Bust in Ohio (Columbus: The Ohio State University).
- Ostrom, E., Gardner, R. & Walker, J. (1994) Rules, Games, and Common-Pool Resources (Ann Arbor: University of Michigan Press).
- Perry, A. M., Rothwell, J. & Harshbarger, D. (2018) The devaluation of assets in Black neighborhoods. https://www.brookings.edu/research/devaluation-of-assets-in-black-neighborhoods/
- Quillian, L., Lee, J. J. & Honoré, B. (2020) Racial discrimination in the U.S. Housing and mortgage lending markets: A quantitative review of trends, 1976–2016, Race and Social Problems, 12, pp. 13–28.
- Ratner, M. S. (1996) Many routes to homeownership: A four-site ethnographic study of minority and immigrant experiences, Housing Policy Debate, 7, pp. 103–145.
- Reid, C. K. (2021). Crisis, Response, and Recovery : The Federal Government and the Black/White Homeownership Gap (Issue March).
- Reid, C. K., Bocian, D., Li, W. & Quercia, R. G. (2017) Revisiting the subprime crisis: The dual mortgage market and mortgage defaults by race and ethnicity, Journal of Urban Affairs, 39, pp. 469–487.
- Rothstein, R. (2017) The color of law: A forgotten history of how our Government Segregated America, in: The Color of Law: A Forgotten History of How Our Government Segregated America (New York: Liveright Publishing Corporation).
- Scally, C. P. (2008) Decoding Housing Finance Agencies (Montclair: Shelterforce).
- Scally, C. P. (2012) The past and future of housing policy innovation: The case of US state housing trust funds, Housing Studies, 27, pp. 127–150.
- Steil, J. P., Albright, L., Rugh, J. S. & Massey, D. S. (2018) The social structure of mortgage discrimination, Housing Studies, 33, pp. 759–776.
- Talen, E. & Anselin, L. (2022) Tracking sixty years of income diversity within neighborhoods: The case of chicago, 1950–2010, Cities, 121, pp. 103479.
- Zonta, M. M. (2012) Applying for home mortgages in immigrant communities: The case of Asian applicants in Los Angeles, Environment and Planning A, 44, pp. 89–110.
- Zou, Y. (2014) Analysis of spatial autocorrelation in higher-priced mortgages: Evidence from philadelphia and chicago, Cities, 40, pp. 1–10.
- Zou, Y. (2015) Re-examining the neighborhood distribution of higher priced mortgage lending: Global versus local methods, Growth and Change, 46, pp. 654–674.