ABSTRACT
Finnish students start academic or vocational track in upper secondary education at age 16 years. Track placement is based on grades, but family background and psychosocial resources may have influences. Using 2014 survey data of Grade 9 students in Helsinki Metropolitan area linked to the Joint Application Registry data as of 2017, we fitted two-level, sex-stratified, generalized structural equation models to determine how reserve capacity (academic self-efficacy and social support), family background, and the comprehensive schools predict track placement. Adjusting for the effect of grades, low reserve capacity and disadvantaged family background increased probabilities of vocational track and non-placement of students. Schools also affected track placement, suggesting differences among comprehensive schools in the area. Findings imply that building reserve capacities of adolescents, through enhancing academic self-efficacy and social support, particularly in those with disadvantaged backgrounds, could increase chances of academic track placement in upper secondary school.
Introduction
Selection of an educational path called tracking, takes place in adolescence. Within-school tracking includes ability grouping of students for specialized classes in the same school while between-school tracking includes placement of students into different school types, offering vocational or academic qualifications (Eccles & Roeser, Citation2011). In countries with selective school systems, between-school tracking typically occurs at an early age, for example, 10 to 12 years. In those with non-selective and comprehensive school systems like the Nordic countries, between-school tracking is applied at a later age, around 16 years (OECD, Citation2013). In Finland, this occurs upon graduation from comprehensive school in Grade 9 (15–16 years), although larger cities have various within-school tracking practices even during primary grades (Koivuhovi, Citation2021).
Tracking has been rationalized to improve learning (Ozer & Perc, Citation2020) and development outcomes of students (Ansalone, Citation2000). High performing students are generally placed in academic tracks and have more favourable educational outcomes compared to those in vocational tracks (Ansalone, Citation2000; Eccles & Roeser, Citation2011). In Finland, students placed in academic tracks were more likely to complete tertiary education and later have higher occupational status and earnings than those placed in vocational tracks. Those who were neither placed in academic nor vocational tracks were likely to be unemployed (Heiskala & Erola, Citation2019). Placement in non-academic tracks have been shown to be associated with negative emotions and attitudes as well as problem behaviours (Eccles & Roeser, Citation2011). Additionally, tracking affects the self-concept or confidence in academic abilities of students through social comparison, known as the Big Fish Little Pond Effect (BFLPE). Current evidence has shown that high performing students develop lower self-concept when placed in selective schools (where other students also have high achievement), unlike in regular schools, where they have higher self-concept as a big fish in a little pond (Fang et al., Citation2018; Koivuhovi, Citation2021). Since tracking differentiates students’ learning and other outcomes, we want to study which factors influence each type of track placement so that early interventions can be recommended to avert the negative effects of tracking.
Tracking may have indirectly led to the socioeconomic and racial segregation of students as those in academic tracks usually have advantaged backgrounds. They have higher economic, social, and cultural statuses and better educated parents (OECD, Citation2018). On the other hand, disadvantaged students disproportionately placed in vocational tracks generally have parents with lower education, jobs, and income, as well as immigrant background and residence in rural areas (OECD, Citation2018). Increased parental roles in track assignment also influenced such socioeconomic and racial segregation of students. In schools which tracked students early or based on economic capacity (e.g. to pay tuition fees), parents with higher education or more advantaged backgrounds placed their children in academic schools (Dustmann, Citation2004; OECD, Citation2013, Citation2018) or avoided schools with high minority student populations (Kruse, Citation2018). Recent Finnish research showed that these background-related differences also exist in within-school tracks of comprehensive school (Koivuhovi et al., Citation2019).
Sex differences in tracking have also been observed, either due to differences in abilities and academic performance (OECD, Citation2018) or differences in educational choices (Becker & Hecken, Citation2009; Pekkarinen, Citation2008). In European countries, there was a higher proportion of girls than boys found in academic tracks during secondary education as well as in academic tertiary education (Pekkarinen, Citation2008). In Finland, girls also select within-school tracks emphasizing certain subjects more often than boys (Koivuhovi et al., Citation2019).
Aside from family background and sex, psychosocial resources could influence tracking placement since cognitive, psychosocial, and emotional development occur during adolescence (Sanders, Citation2013). Following the reserve capacity framework of Gallo and Matthews (Citation2003), these psychosocial resources were proposed to be maintained by individuals as a bank of intrapersonal and interpersonal psychosocial resources, called reserve capacity, and kept in reserve for dealing with stressful situations. Specific examples of interpersonal resources are social support and social integration while intrapersonal characteristics include self-efficacy, mastery or a sense of perceived control (Matthews & Gallo, Citation2011). Initially, it was tested as an important cause of socioeconomic inequalities in health. Then, links with educational pathways were explored as studies showed that individuals with high reserve capacity gain the coping skills necessary to attain higher education while those with low reserve capacity may lack these skills and attain lower education and income (Matthews et al., Citation2010). Previous research showed that reserve capacity was significantly related to school achievement; it was also a direct pathway to educational level in adulthood (Acacio-Claro et al., Citation2019). Another psychosocial resource shown to affect educational outcomes was academic self-efficacy that indicates beliefs about one’s ability to succeed in school (Ryan & Patrick, Citation2001). It is predictive of educational expectations such that students with high academic self-efficacy were more likely to obtain higher degrees than those with low academic self-efficacy (Merritt & Buboltz, Citation2015). Such mechanisms raise the question of how reserve capacity might influence tracking placement since it affects educational achievements.
Much of the discussion about tracking dwells on its negative, long-term consequences particularly for disadvantaged students in non-academic tracks. Aside from the role of family background in tracking and its academic grounds (OECD, Citation2018; Ozer & Perc, Citation2020), there is limited evidence about its determinants which likely include reserve capacity. We aim to fill this research gap by assessing the role of reserve capacity in tracking during the transition phase from lower secondary to upper secondary education. We want to determine how its dimensions of social support and academic self-efficacy shape educational trajectories through the type of a student’s placement (academic vs. vocational) in upper secondary school. We will also assess if family background has an effect at this stage in the student’s life, when major influences are derived from one’s peer group (Viner et al., Citation2012). Since the school as an institution could impact students’ academic achievement, either due to presence of specialized classes (Koivuhovi, Citation2021) or area-based socioeconomic composition of the student population (Karvonen et al., Citation2018) as observed in urbanized areas of Finland, then the lower secondary schools these students attend may influence their placement. Considering this school effect and accounting for sex-differences, we aim to determine how reserve capacity, along with family background and school achievement, affect tracking of students in Finland which takes place at 16 years of age.
Materials and methods
Study design and sample
A longitudinal study design was constructed using survey data linked with register data. A total of 13,138 Grade 9 students (usually aged 15–16 years) in comprehensive schools located in the 14 municipalities of the Helsinki Metropolitan area in Southern Finland were recruited. Baseline data were obtained from school-based surveys collected in 2014 using two online questionnaires: Health Survey and Learning-to-Learn Assessment. 9,271 (70.6%) students completed at least one questionnaire. Out of this, 7344 (79.2%) comprised of 50.5% boys (n = 3711) and 49.5% girls (n = 3633) answered both questionnaires and were included in the study. Outcome data regarding type of track placement in upper secondary education were obtained from the National Board of Education’s register of applications to vocational and upper secondary general education (Joint Application Registry) covering the period 2014–2017.
The protocol was approved by the Ethics Committee of the National Institute for Health and Welfare. In line with the instructions of the Finnish National Board on Research Integrity (TENK) in 2009, no parental consent was required when the study was conducted as part of the students’ normal schoolwork. Parental consent was, however, obtained in two of the 14 municipalities where local authorities required it. Information letters were sent to parents in other municipalities. Participation was voluntary and the students were instructed that they can decline to answer any question or withdraw from the survey at any time.
Variables
Outcome
The type of placement in upper secondary school for Grade 9 students was obtained from the Joint Application Registry. Type of placement referred to the most recent placement of students in upper secondary school (vocational track, academic track, no placement) based on the registry as of 2017 application rounds.
Family background variables
Information related to parents’ work and education as well as immigrant background were reported by the students.
Parental work participation was based on combined answers to the question, ‘Is mother/father working?’ and categorized into no working parents, one parent working, both parents working and not applicable (no parents).
Parental education had the following categories: basic education only, vocational upper secondary education or vocational college, matriculation examination certificate, and bachelor’s degree or higher. The highest level of education of either mother or father was considered. If one parent had missing data, the available parent’s data was used. Those who indicated they had no mother/father had missing values for parental education. A bachelor’s degree or higher was classified as ‘high’ education and all other categories were recoded as ‘low or middle’ education.
An immigrant background was indicated by language spoken at home and categorized as either Finnish- or Swedish-speaking ‘native’ or immigrant who spoke other language.
Adolescent variables
Academic achievement was based on the student’s grade point average (GPA) in the school-leaving certificate obtained in the Joint Application Registry. The GPA of each student was calculated based on the student’s grade in mother tongue, foreign language, mathematics, and science (averaging grades in biology, geography, physics, and chemistry). Students’ GPA, ranging from 4 (fail) to 10 (excellent), was recoded to 3 categories: ‘high’ (9–10), ‘medium’ (7.0001–8.9999) and ‘low’ (less than or equal to 7).
Social support was used as one dimension of reserve capacity in this study. Several proxy indicators of social support from parents, peers, and teachers were used. Parental social support was based on ease of talking (easy, difficult, or very difficult) about troubling issues both to father and mother. Those who did not have a father (5.6%), or mother (1.8%) were included in the ‘very difficult’ category. Ranked responses of the students ranging from 1 (not true at all) to 7 (certainly true) to the statement ‘My relationship with my parents is very good’ were also used. Peer support was indicated by the student’s chosen option (not true, somewhat true, certainly true) regarding the statement, ‘I have one good friend or more.’ On the other hand, teacher support was based on the statement, ‘I usually get very well along with my teachers’, which students ranked from 1 (not true at all) to 7 (certainly true).
The variables talking to father and mother were previously shown to measure the underlying construct of reserve capacity (Acacio-Claro et al., Citation2019). When other measures indicating social support from peers and teachers were added, the overall construct of social support was estimated to have acceptable (0.60 Cronbach’s alpha) internal consistency or reliability (Ursachi et al., Citation2015).
Academic self-efficacy was another dimension of reserve capacity measured using three general statements with very good level of reliability (0.90 Cronbach’s alpha). These statements reflected action-control beliefs parallel to Bandura’s efficacy beliefs (Koivuhovi et al., Citation2019). These were: ‘I get good school grades when I want to;’ ‘I learn what I need to learn when I decide so;’ and, ‘I do well in my studies when I decide so,’ which students ranked from 1 (not true at all) to 7 (certainly true).
Ranked responses to the statements used as indicators for social support and academic self-efficacy were further grouped according to these categories: not true (ranks 1–3), somewhat true (rank 4) and certainly true (ranks 5–7).
Statistical analysis
We used a two-stage analytic approach. First, we fitted a series of LCA (latent class analysis) models to identify unobserved (latent) groups of students based on their responses to indicators of social support and academic self-efficacy. We started with a two-class model up until an additional class did not improve the model fit based on Bayesian and Akaike information criteria (BIC and AIC), and entropy index (). Lower BIC and AIC values indicate good fit while entropy greater than 0.80 indicates high separation between the classes (Nylund-Gibson & Choi, Citation2018). When the ideal number of classes was selected for each latent variable, the rho (ρ) parameters or item-response probabilities conditional on latent class membership were examined to determine meaningful interpretation of the latent class categories (). The models were again re-fitted, with measurement invariance imposed to ensure comparability of the latent classes between sexes (Lanza et al., Citation2007). Modal assignment of the respective latent class of social support and academic self-efficacy for each student was based on posterior probabilities (Lanza et al., Citation2015).
Table 1. Statistical fit indices for LCA models of social support and self-efficacy
Figure 1. Percent probability of positive response for each item in model A: three-class academic self-efficacy, and model B: four-class social support.
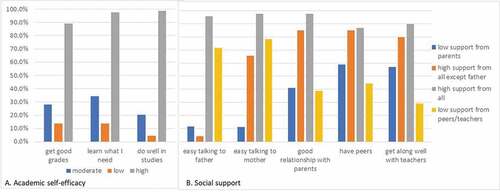
Second, we employed multilevel modelling to include random effects across groups or levels of the data as these may have unmeasured effects influencing the effects observed at the individual level (StataCorp, Citation2017). Our study used a two-level, generalized structural equation model (GSEM) for a multinomial logit response, where students (level 1) were nested within schools (level 2). The multilevel models were stratified by sex since statistically significant sex-differences were observed based on Chi-square (χ2) tests (). Using a backward elimination approach, variables included in multivariate analyses were only those statistically significant in bivariate analyses. Model fit was assessed based on BIC and AIC values. Final model parameters were presented as relative risk ratios (RRRs) with 95% confidence intervals (CIs). The marginal effects of the categorical predictors on the outcome were also calculated. These show how much the predicted probability of a particular outcome category changes as the category of each predictor also changes, controlling for all the other variables in the model using their means (Williams, Citation2012). Descriptive statistics were presented in tabular and graphical forms. All analyses were performed using STATA/SE version 15.1 and LCA Stata Plugin version 1.2 (The Methodology Center, Penn State, Citation2015).
Table 2. Distribution of participants according to background characteristics, reserve capacity and education-related factors
Results
Identifying latent classes of social support and self-efficacy
Results of latent class analyses showed that a 4-class solution of variables for social support and a 3-class solution for self-efficacy yielded lower BIC and AIC values. The respective entropy indices of the models also implied good separation among the identified classes ().
To interpret the latent classes, the conditional probabilities for the selected LCA models were assessed. In , the left side of the figure presents the percent probabilities of ‘certainly true’ responses for each item indicating self-efficacy: getting good grades, learning, and doing well in studies. The last class had high probability of endorsement across all items and was labelled as ‘high self-efficacy’. The other two were categorized as ‘low self-efficacy’ and ‘moderate self-efficacy’ classes based on percent probabilities of the item responses. Latent class modal assignment showed that 76.1% students had high self-efficacy, 15.9% had moderate and 8% had low self-efficacy.
The right side of shows the percent probability that a student selected the most positive category to each item for social support, that is, a student indicated it was easy talking to and having good relationship with parents as well as having friends and getting along well with teachers were certainly true. The first class showed low response probabilities for the parent-related variables but increased response probabilities for having friends and getting along well with teachers, thus, this class was labelled as ‘low support from parents’. The second class showed seemingly high response probabilities for social support from all sources, except for talking to father. We labelled this class as having ‘high support from all except father’ as the third class was clearly the group with ‘high support from all’. The last class had generally higher positive response probabilities in the parent-related variables but had low probabilities of positive responses for the other items; thus, this class was labelled as having ‘low support from peers/teachers’. Latent class modal assignment showed that 58.4% of students had high social support from all sources while 27.2% had high social support too, except from father. Less than a tenth of the students had low support from peers/teachers (8.1%) and from parents (6.3%). shows the distribution according to sex for both latent variables.
Background characteristics
The male and female students had comparable age distributions, with mean age of 16 ± 0.4 years. The oldest student among males was aged 21 years, while among females, 19 years. There were statistically significant sex-differences in terms of family background, academic grades, and reserve capacity characteristics (). On the average, females had higher GPAs than males. The proportion of female students who placed in academic track in upper secondary schools was also significantly greater than that of males (71.4% vs 58.4%, p < 0.001). presents the detailed distribution of these characteristics according to students’ sex.
Predictors of upper secondary school track placement
Results of multivariate analyses in already accounted for the school effect by including it as a higher level. In our study, an RRR > 1 indicates that the risk of either a vocational placement or none, relative to the risk of an academic one, increases with the category of the predictor as compared with the referent group. On the other hand, an RRR < 1 indicates that the risk of either a vocational placement or none is less likely than an academic placement.
Table 3. Multivariate associations of family background, academic achievement, and reserve capacity with type of placement of male and female students in upper secondary school (using academic placement as reference category)
Among male students, having an immigrant background was not associated with the type of placement in upper secondary school during bivariate analyses, hence, this was excluded in the multivariate model. shows that the relative probability of having a vocational rather than an academic placement for students whose parents had low or middle education is more than double the corresponding relative probability for male students whose parents had high education. Having lower academic achievement was associated with big increases in the relative probability of vocational over academic placement. In terms of reserve capacity, the expected risk of having a vocational placement for students was higher for those who had low social support from peers and teachers and lower levels of academic self-efficacy. On the other hand, having only one or no working parents, lower academic achievement and having low social support from peers and teachers increased the risk of non-placement relative to academic placement in upper secondary school.
Among female students, all family background, academic achievement, and reserve capacity variables were significantly associated with type of track placement in bivariate analyses. Thus, these were all included in the two-level multivariate model (). The multivariate model shows that the relative probability of having a vocational rather than an academic placement in female students with parents who were not degree holders nor working or with lone parent working was at least double the corresponding relative probability for those whose parents were highly educated and both working. Having lower academic achievement was associated with marked increases in the relative probability of vocational over academic placement. In terms of reserve capacity, the expected risk of having a vocational placement for female students was increased in those who had high support from all, except father and had low academic self-efficacy. On the other hand, having an immigrant family background, only one or no working parents, lower academic achievement, and having low self-efficacy increased the risk of female students’ non-placement relative to academic placement in upper secondary school.
School effect
The estimated variance of the random effect of school level among males was 0.3069 whose square root was equal to a standard deviation (sd) of 0.5540. Exponentiating this sd value was equal to 1.74. Thus, variability in the current school, such as a 1-standard-deviation change in the random effect, could lead to practical differences amounting to a 1.74 change in the RRR. This school effect in the type of placement of male students was statistically significant (p < 0.001).
The estimated variance of the random effect of school among girls was 0.2358, equal to a standard deviation of 0.4856. A 1-standard-deviation change in the random effect amounted to a 1.63 change in the RRR. This school effect in the tracking placement of female students was also statistically significant (p < 0.001).
Predicted probabilities of predictors on type of school placement among male students
show by how much a specific category of a predictor, compared to its referent group (RRR = 1.0 in ), increased (above zero point in the plot) or decreased (below zero point in the plot) the average predicted probability of each type of placement of the students in upper secondary school.
Figure 2. Average predicted probabilities of parental education and work, reserve capacity and grades of male students on type of track placement in upper secondary school.
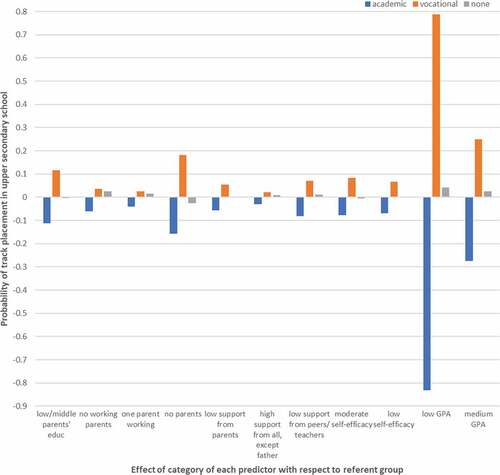
Figure 3. Average predicted probabilities of parental education and work, reserve capacity and grades of female students on type of track placement in upper secondary school.
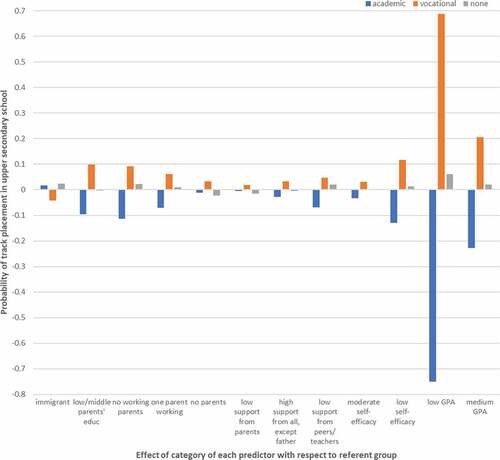
In , it is evident that school achievement based on grades was the strongest predictor of the type of placement of male students in upper secondary school. Low and medium GPAs significantly and markedly decreased (p < 0.001) the probability of academic track placement but greatly increased (p < 0.001) that of vocational track placement and slightly increased (p < 0.001) the probability of no placement. Other factors that significantly decreased the probability of academic placement of male students were having one working parent (p = 0.03) or parents with low or middle education (p < 0.001), having either low (p = 0.02) or moderate academic self-efficacy (p < 0.001), and having low social support from peers/teachers (p < 0.001). On the other hand, having parents without high education (p < 0.001), having low (p = 0.03) and moderate (p < 0.001) academic self-efficacy, and having low social support from peers/teachers (p < 0.001) significantly increased the probability of male students’ placement in vocational tracks. Having no parents also significantly increased (p < 0.001) the probability of not placing in any track in upper secondary school ().
Predicted probabilities of variables on type of school placement among female students
Having an immigrant background significantly decreased the probability of placement in vocational track (p = 0.03) but increased that of having no placement (p = 0.03) among female students. Like the results in males, school achievement strongly predicted placement of female students in upper secondary school. Less than high GPAs markedly decreased the probabilities of placement in academic tracks (p < 0.001) but increased those of vocational (p < 0.001) and no placement (p < 0.001). Female students whose parents had low or middle education (p < 0.001), and no work (p = 0.004) or only one working parent (p < 0.001), had significantly decreased probabilities of placement in academic tracks. Not obtaining social support from father (p = 0.034) and having low academic self-efficacy (p < 0.001) also decreased one’s probabilities of academic track placement. On the other hand, these factors significantly increased the probabilities of vocational track placement: having parents with low or middle education (p < 0.001), no working parents (p = 0.016) or only one parent working (p < 0.001), as well as having social support from all except father (p = 0.015) and having low academic self-efficacy (p < 0.001). See, .
Discussion
Summary and interpretation of main findings
Our findings showed that significantly more female students placed in academic tracks (71% vs. 58%) while significantly more males placed in vocational tracks (38% vs. 26%). Around 3% of all students did not have any placement. The strongest predictor of the type of track placement remained the students’ school achievement. This was expected because Finnish students apply to either academic or vocational tracks or both and selection is based on their grades and quota of the upper secondary schools (Heiskala & Erola, Citation2019). Adjusting for the effect of school achievement, reserve capacity dimensions of social support and academic self-efficacy significantly predicted track placement, although, there were minor sex differences in the effects of these factors. Likewise, family background, particularly parents’ education and work participation, still played a role in tracking placement of their adolescent children. In addition, the type of placement was also influenced by the school, suggesting that there were differences among the comprehensive schools in the region that affected the students’ track placement in upper secondary schools.
The relationship between reserve capacity and type of placement in upper secondary school observed in our study was logical since tracking occurs during adolescence and coincides with one’s cognitive, psychosocial, and emotional development (Sanders, Citation2013). Literature on youth development showed that individuals with psychosocial resources had positive emotional and psychosocial development, enabling them to perform well in academics (Kroenke, Citation2008). The link between academic achievement and psychosocial factors was also demonstrated in a study of adolescents during school transitions (ACT, Citation2007).
Our results showed that students’ academic self-efficacy was positively related with placement in academic track while negatively related with those of vocational track and non-placement in upper secondary school. These may be attributed to how higher levels of self-efficacy increases academic achievement as documented previously (Komarraju & Nadler, Citation2013; Uchida et al., Citation2018). Indeed, another research found that students in vocational tracks had lower levels of self-efficacy compared to those in regular or academic tracks (Chu et al., Citation2015). Our findings confirmed both theoretical and practical underpinnings of self-efficacy in schooling of adolescents as those with higher self-efficacy tend to ‘participate more readily, work harder, persist longer when they encounter difficulties, and achieve at higher levels’ compared to those with lower self-efficacy (Schunk & Meece, Citation2006).
Although we observed sex differences in the effect of social support on probability of track placement in our study, the general pattern seen was that students with low social support had decreased probabilities of academic placement. This was comparable with results of a previous study where students in vocational tracks had lower levels of social support from family, peers and others compared to students in academic tracks (Chu et al., Citation2015). We found that male students had low social support from teachers and peers which increased their probabilities of vocational track or non-placement. This was comparable to a previous study which observed that female students had higher social support from teachers and classmates than males (Demir & Leyendecker, Citation2018). On the other hand, female students who had increased probabilities of vocational track placement lacked paternal social support. While other studies did not distinguish between maternal and paternal support, a previous review found that adolescents with absentee fathers had poorer academic achievement than those with involved fathers (East et al., Citation2006).
The role of supportive relations with parents, teachers and peers on positive learning and educational outcomes has been widely documented. Social support from family improved academic performance and enhanced self-concept (Zhang, Citation2020), led to greater academic involvement (Eccles & Roeser, Citation2011; Vargas-Madriz & Konishi, Citation2021) and predicted enrolment in higher education (Brekke, Citation2015). Similarly, social support from teachers and friends increased academic efficacy, self-regulated learning and decreased disruptive behaviour (Ryan & Patrick, Citation2001). School-related support was also positively associated with sense of school belongingness and engagement (Demir & Leyendecker, Citation2018; Garcia-Reid et al., Citation2015; Vargas-Madriz & Konishi, Citation2021) and academic performance (Demir & Leyendecker, Citation2018). Thus, these may be the mechanisms through which social support influenced track placement.
Our results also showed that family background still play a role as parents’ education and employment exerted a statistically significant influence on probability of track placement of students with an average birth cohort in 1998. Those whose parents were not highly educated, unemployed or with only one working parent were less likely to be placed in academic tracks. This was consistent with findings in a 1975 birth cohort of Finns, where majority of students in vocational tracks came from parents with basic and secondary education and lower parental employment classes (Heiskala & Erola, Citation2019). Our study also showed that having an immigrant background increased the probability of female students not having any placement in upper secondary school. We surmise that this was compounded by the interrelationships among race, socioeconomic status (SES), and academic performance during secondary education (OECD, Citation2018; Rumberger & Palardy, Citation2005). Examining our data further revealed that this group of immigrant female students mostly had low grades.
The influence of family background should attenuate when tracking is applied at a later age, as the case in Finland. In countries that track students at a younger age such as in Germany, track types and school choices are based on parents’ preferences. Thus, parents with disadvantaged backgrounds may not be well informed to decide in the best interest of their children (Dustmann, Citation2004). As students get older, they are less dependent on their parents’ resources and are already capable of charting their own educational paths (OECD, Citation2013). In Finland, after compulsory schooling, students are entitled to a monthly stipend for a limited duration, which varies with parents’ income, age, and level of studies (Heiskala & Erola, Citation2019). Schools in the Nordic countries were characterized to ‘be homogenous and more inclusive, providing equal opportunities to students regardless of their geographical location, SES, gender and ethnicity’ (OECD, Citation2018). Our results, however, showed that even with this type of educational system, inequalities in track placement based on parents’ socioeconomic and immigrant backgrounds persisted. Indeed, a Finnish study has shown that schools differed by socioeconomic composition, possibly due to geographic segregation, and these differences were related to well-being and school achievement (Karvonen et al., Citation2018). In the 1990s, classes with a special emphasis were introduced in the Finnish basic education system, analogous to within-school tracking in other countries. Recent evidence showed that such classes were selective by nature and attended by students with higher grades and more advantaged backgrounds, compared to classes without a special emphasis (Koivuhovi, Citation2021). Thus, our findings of school-level effect on track placement further confirmed that there were inherent differences among the comprehensive schools, likely creating these inequalities.
The sex differences in the type of placement of students observed in our study were consistent with previous evidence which found that males were more likely to be in vocational tracks than in academic tracks (Pekkarinen, Citation2008). Pekkarinen (Citation2008) attributed this phenomenon to the postponement of tracking in Finland to age 16 years that occurred because of the educational reform in the 1970s. He explained that at this age, females can make critical educational decisions since they mature biologically and psychologically earlier than males. Our results showed that male students had generally lower grades compared to their female classmates; thus, they may be also less likely to apply for academic tracks. Other plausible explanations could be inherent gender differences in learning (Eccles & Roeser, Citation2011) or gender-based preferences for certain specialized classes or subjects within school (Koivuhovi et al., Citation2019) which affect school achievement.
Strengths and limitations of the study
Major strengths of our study included its large cohort of adolescent students during a critical educational transition and linkage with objective, register-based outcome measurements. Although family background and reserve capacity data collected were self-reported, multiple indicators were used and reliably measured latent constructs. The estimation of marginal effects also allowed for meaningful comparisons of the effects of each predictor on track placement.
We noted some limitations of our study. It was not known whether the low proportion of students with immigrant background in our study represented the actual proportion in the adolescent population or only reflected a low response rate among these students. This probably underestimated the effect of such family background on track placement. Class-level data were also not available; thus, while it was not the focus of this study to determine whether classroom differences exist, the inclusion of this class-level effect in our analyses could have been more informative.
Conclusions
The track choice is crucial as this determines the post-secondary educational pathways and income prospects of the students (Dustmann, Citation2004). Although it is widely recognized that the academic track is the preferred route for educational success, vocational students in Finland have entry pathways for tertiary education, too. Thus, the focus should be placed on the completion of upper secondary education that is a barrier for those students without placement. This leads to cumulative disadvantage, especially for students with low SES and immigrant backgrounds.
Our study showed that reserve capacity is one of the modifiable mechanisms which can influence track placement and likely ensure successful educational transitions. Building reserve capacities of adolescents, through increasing self-efficacy and supportive family and school relations, will likely improve their school performances and increase the chances of placement in upper secondary school.
Our results provide useful data for programme planners and policymakers. Programmes designed to reduce educational inequalities can be directed to addressing factors, which predict students’ type of track placement. Intervention measures at multiple levels (individual, family, school, society) could target particularly disadvantaged students and ensure they have a school placement, preferably in academic tracks. Thus, all students, regardless of background or abilities would be guaranteed better educational and other life outcomes in adulthood.
Acknowledgements
The authors thank Dr. Henrik Dobewall and Anna Myöhänen for assistance in data management and dataset preparation and the personnel of the Centre for Educational Assessment at the University of Helsinki for data collection.
Disclosure statement
No potential conflict of interest was reported by the authors.
Additional information
Funding
Notes on contributors
Paulyn Jean Acacio Claro
Paulyn Jean Acacio Claro is a visiting researcher at the Unit of Health Sciences, Faculty of Social Sciences, Tampere University, Finland, and a regular part-time faculty member at the Graduate School of Business, Ateneo de Manila University, Philippines.
Leena Koivusilta
Leena Koivusilta is University Lecturer and Adjunct Professor (in social policy) at the Department of Social Research, Faculty of Social Sciences, University of Turku, Finland.
Mari-Pauliina Vainikainen
Mari-Pauliina Vainikainen is a Professor of learning and instruction at the Faculty of Education and Culture, Tampere University. She leads the Research Group for Education, Assessment and Learning (REAL), which operates at the universities of Tampere, Helsinki and Jyväskylä in Finland.
Arja Rimpelä
Arja Rimpelä is Professor of Public Health at the Unit of Health Sciences, Faculty of Social Sciences, Tampere University, Finland. She leads the research group NEDIS (Research on Children’s and Adolescents’ Health Promotion).
References
- Acacio-Claro, P., Koivusilta, L. K., Doku, D. T., & Rimpelä, A. H. (2019). Timing of puberty and reserve capacity in adolescence as pathways to educational level in adulthood—a longitudinal study. Annals of Human Biology, 46(1), 35–45. https://doi.org/10.1080/03014460.2019.1596311
- ACT. (2007). Impact of cognitive, psychosocial, and career factors on educational and workplace success. Issues in college success. Retrieved September 30, 2021, from https://files.eric.ed.gov/fulltext/ED510542.pdf
- Ansalone, G. (2000). Keeping on track: A reassessment of tracking in the schools. Race, Gender & Class, 7(3), 108–132. Retrieved June 25, 2021, from: http://www.jstor.org/stable/41674950
- Becker, R., & Hecken, A. E. (2009). Higher education or vocational training? An empirical test of the rational action model of educational choices suggested by Breen and Goldthorpe and Esser. Acta Sociologica, 52(1), 25–45. https://doi.org/10.1177/0001699308100632
- Brekke, I. (2015). Health and educational success in adolescents: A longitudinal study. BMC Public Health, 15(1), 619. https://doi.org/10.1186/s12889-015-1966-0
- Chu, X., Li, Z., Yan, B., Han, J., & Fan, F. (2015). Comparative study of regular and vocational high school students on family socioeconomic status, social support, self-efficacy and well-being. Open Journal of Social Sciences, 3(8), 61–68. https://doi.org/10.4236/jss.2015.38006
- Demir, M., & Leyendecker, B. (2018). School-related social support is associated with school engagement, self-competence and health-related quality of life (HRQoL) in Turkish immigrant students. Frontiers in Education, 3, 83. Retrieved October 27, 2021, from: https://www.frontiersin.org/article/10.3389/feduc.2018.00083
- Dustmann, C. (2004). Parental background, secondary school track choice, and wages. Oxford Economic Papers, 56(2), 209–230. https://doi.org/10.1093/oep/gpf048
- East, L., Jackson, D., & O’Brien, L. (2006). Father absence and adolescent development: A review of the literature. Journal of Child Health Care, 10(4), 283–295. https://doi.org/10.1177/1367493506067869
- Eccles, J. S., & Roeser, R. W. (2011). School and community influences on human development. In M. H. Bornstein & M. E. Lamb (Eds.), Developmental science: An advanced textbook (pp. 571–643). Psychology Press.
- Fang, J., Huang, X., Zhang, M., Huang, F., Li, Z., & Yuan, Q. (2018). The big-fish-little-pond effect on academic self-concept: A meta-analysis. Frontiers in Psychology, 9, 1569. Retrieved September 27, 2021, from: https://www.frontiersin.org/article/10.3389/fpsyg.2018.01569
- Gallo, L. C., & Matthews, K. A. (2003). Understanding the association between socioeconomic status and physical health: Do negative emotions play a role? Psychological Bulletin, 129(1), 10–51. https://doi.org/10.1037/0033-2909.129.1.10
- Garcia-Reid, P., Peterson, C. H., & Reid, R. J. (2015). Parent and teacher support among Latino immigrant youth: Effects on school engagement and school trouble avoidance. Education and Urban Society, 47(3), 328–343. https://doi.org/10.1177/0013124513495278
- Heiskala, L., & Erola, J. (2019). Tracking and sorting in the Finnish educational system. (DIAL Working Paper Series Issue 12). NORFACE. https://dynamicsofinequality.org/wp-content/uploads/2019/07/LIFETRACK-country-report_FIN-1.pdf
- Karvonen, S., Tokola, K., & Rimpelä, A. (2018). Well-being and academic achievement: Differences between schools from 2002 to 2010 in the Helsinki metropolitan area. Journal of School Health, 88(11), 821–829. https://doi.org/10.1111/josh.12691
- Koivuhovi, S. (2021). Studying in a class with a special emphasis: Changes in children’s competence beliefs and mathematical thinking skills and the role of social comparisons [ Doctoral dissertation, University of Helsinki]. HELDA - Digital Repository of the University of Helsinki. http://hdl.handle.net/10138/330292
- Koivuhovi, S. E. K., Vainikainen, M.-P., Kalalahti, M. M. M., & Niemivirta, M. J. (2019). Changes in children’s agency beliefs and control expectancy in classes with and without a special emphasis in Finland from grade four to grade six. Scandinavian Journal of Educational Research, 63(3), 427–442. https://doi.org/10.1080/00313831.2017.1402364
- Komarraju, M., & Nadler, D. (2013). Self-efficacy and academic achievement: Why do implicit beliefs, goals, and effort regulation matter? Learning and Individual Differences, 25, 67–72. https://doi.org/10.1016/j.lindif.2013.01.005
- Kroenke, C. (2008). Socioeconomic status and health: Youth development and neomaterialist and psychosocial mechanisms. Social Science & Medicine, 66(1), 31–42. https://doi.org:/dx.doi.org/10.1016/j.socscimed.2007.07.018
- Kruse, H. (2018). Between-school ability tracking and ethnic segregation in secondary schooling. Social Forces, 98(1), 119–146. https://doi.org/10.1093/sf/soy099
- Lanza, S. T., Collins, L. M., Lemmon, D. R., & Schafer, J. L. (2007). PROC LCA: A SAS procedure for latent class analysis. Structural Equation Modeling: A Multidisciplinary Journal, 14(4), 671–694. https://doi.org/10.1080/10705510701575602
- Lanza, S. T., Dziak, J. J., Huang, L., Wagner, A. T., & Collins, L. M. (2015). LCA stata plugin users’ guide (version 1.2). University Park: The Methodology Center, Penn State. Retrieved August 21, 2020, from. methodology.psu.edu.
- Matthews, K. A., & Gallo, L. C. (2011). Psychological perspectives on pathways linking socioeconomic status and physical health. Annual Review of Psychology, 62(1), 501–530. https://doi.org/10.1146/annurev.psych.031809.130711
- Matthews, K. A., Gallo, L. C., & Taylor, S. E. (2010). Are psychosocial factors mediators of socioeconomic status and health connections? A progress report and blueprint for the future. Annals of the New York Academy of Sciences, 1186(1), 146–173. https://doi.org/10.1111/j.1749-6632.2009.05332.x
- Merritt, D. L., & Buboltz, W. (2015). Academic success in college: Socioeconomic status and parental influence as predictors of outcome. Open Journal of Social Sciences, 3(5), 127–135. https://doi.org/10.4236/jss.2015.35018
- The Methodology Center, Penn State. (2015). LCA stata plugin (version 1.2) [Computer software]. University Park: The Methodology Center, Penn State. https://scholarsphere.psu.edu/resources/feaaba7c-8d56-412c-b6a0-bfa4564506f0
- Nylund-Gibson, K., & Choi, A. Y. (2018). Ten frequently asked questions about latent class analysis. Translational Issues in Psychological Science, 4(4), 440–461. https://doi.org/10.1037/tps0000176
- OECD. (2013). PISA 2012 results: What makes schools successful (volume iv): Resources, policies and practices. https://doi.org/10.1787/9789264201156-en
- OECD. (2018). Equity in education: Breaking down barriers to social mobility. https://doi.org/10.1787/9789264073234-en
- Ozer, M., & Perc, M. (2020). Dreams and realities of school tracking and vocational education. Palgrave Communications, 6(1), 34. https://doi.org/10.1057/s41599-020-0409-4
- Pekkarinen, T. (2008). Gender differences in educational attainment: Evidence on the role of tracking from a Finnish quasi-experiment. The Scandinavian Journal of Economics, 110(4), 807–825. Retrieved June 17, 2021, from: http://www.jstor.org/stable/25195375
- Rumberger, R. W., & Palardy, G. J. (2005). Does segregation still matter? The impact of student composition on academic achievement in high school. Teachers College Record, 107(9), 1999–2045. https://doi.org/10.1177/016146810810700905
- Ryan, A. M., & Patrick, H. (2001). The classroom social environment and changes in adolescents’ motivation and engagement during middle school. American Educational Research Journal, 38(2), 437–460. https://doi.org/10.3102/00028312038002437
- Sanders, R. A. (2013). Adolescent psychosocial, social, and cognitive development. Pediatrics in Review, 34(8), 354–359. https://doi.org/10.1542/pir.34.8.354
- Schunk, D. H., & Meece, J. L. (2006). Self-Efficacy development in adolescence. In F. Pajares & T. Urdan (Eds.), Self-Efficacy beliefs of adolescents (pp. 71–96). Information Age Publishing.
- StataCorp. (2017). Stata structural equation modeling reference manual release 15. Stata Press.
- Uchida, A., Michael, R. B., & Mori, K. (2018). An induced successful performance enhances student self-efficacy and boosts academic achievement. AERA Open, 4(4), 2332858418806198. https://doi.org/10.1177/2332858418806198
- Ursachi, G., Horodnic, I. A., & Zait, A. (2015). How reliable are measurement scales? External factors with indirect influence on reliability estimators. Procedia Economics and Finance, 20, 679–686. https://doi.org/10.1016/S2212-5671(15)00123-9
- Vargas-Madriz, L., & Konishi, C. (2021). The relationship between social support and student academic involvement: The mediating role of school belonging. Canadian Journal of School Psychology, 36(4), 290–303. https://doi.org/10.1177/08295735211034713
- Viner, R. M., Ozer, E. M., Denny, S., Marmot, M., Resnick, M., Fatusi, A., & Currie, C. (2012). Adolescence and the social determinants of health. The Lancet, 379(9826), 1641–1652. https://doi.org/10.1016/S0140-6736(12)60149-4
- Williams, R. (2012). Using the margins command to estimate and interpret adjusted predictions and marginal effects. Stata Journal, 12(2), 308–331. Retrieved September 1, 2020, from: http://www.stata-journal.com/article.html?article=st0260.http://www.stata-journal.com/sjpdf.html?article=st0260
- Zhang, Y. (2020). Quality matters more than quantity: Parent–Child communication and adolescents’ academic performance. Frontiers in Psychology, 11, 1203. Retrieved October 27, 2021, from: https://www.frontiersin.org/article/10.3389/fpsyg.2020.01203