Abstract
There is no doubt of the contributions made by mobile phones and mobile network operators in increasing access to communications in rural areas of developing countries. Yet how affordable is this ubiquitous access in such an ICT ecosystem? Using data from two stratified random surveys conducted in a South African rural community, this paper provides a unique in-depth picture of the expenditure and communication patterns of its dwellers. Results show a high access ratio of people using mobile phone services weekly and a high proportion of disposable income dedicated to a very constrained set of mobile phone services. Factors such as mobile phone charging and the extra charges added by airtime resellers contribute to increase the communication costs. This data and its analysis can be used by the following: regulators and government agencies to better design their policy implementations to provide universal service and access; competing industry players to understand the dynamics within rural communities to better target their products; civil society organizations to use it as a case in their efforts to make affordable communications a constitutional right.
1. Introduction
Providing telecommunication access to rural areas is neither easy nor profitable. As a result of these disincentives, traditional telecommunication companies have had little interest to provide telecom infrastructure in the past (Navas-Sabater, Dymond, & Juntunen, Citation2002). This lack of private sector action has pushed national governments to devise policy strategies to ensure that parts of their population are not left behind in the digital revolution. Initially, universal access and service plans took different forms in different countries with very uneven success rates (Gillwald, Citation2005). In most cases, these plans proved to be inadequate to meet the substantial scale of the access problem (Ó Siochrú & Girard, Citation2005). Due to the inefficiency of their former plans, some governments included universal service and access obligations to mobile network operators when liberalizing the telecommunications market (Westerveld, Citation2012). In combination with the overwhelming and unexpected uptake of mobile phones by poor people (Aker & Mbiti, Citation2010), this initiative has mitigated the lack of access in most places, and now mobile phone use and the opportunity to exercise their right to communicate extend to those living below the international poverty lines (Elder, Samarajiva, Gillwald, & Galperin, Citation2013).
Despite the contribution made by mobile network operators in increasing access to communications in rural areas of developing countries, the development outcomes that increased connectivity promises to bring to underprivileged areas remain unmet (Westerveld, Citation2012). Digital communication remains unaffordable to most, and both voice (Bidwell et al., Citation2011) and data communications (Johnson & Roux, Citation2008; Mathee, Mweemba, Pais, Van Stam, & Rijken, Citation2007) are more expensive to rural dwellers when compared to their urban counterparts.
In South Africa, very little is known about the communication expenditure and patterns of use by rural dwellers. Considerable work has been dedicated to understanding mobile phone usage among the youth, specifically students, in urban areas (Donner & Walton, Citation2013; Kreutzer, Citation2009). Using the methodology from Kreutzer (Citation2009), Gunzo and Dalvit (Citation2012) presented a study on the ICT usage between school students (grade 2–12) from rural and urban areas in the Eastern Cape. The research reports usage among students is mainly for text messaging, playing games and listening to radio, and a higher uptake of multimedia services by township students. In Bidwell et al., (Citation2011), a more comprehensive age group (ages from 14 to 54) is considered, but the mobile phone usage was primarily focused on investigating the Callback service.
In terms of affordability, whereas in South Africa the national average monthly individual income is R2587 (US$230.3Footnote1), the average monthly income in rural traditional areas is around R800 (US$71.21)Footnote2, i.e. less than a third of the national average. Thus, for a rural dweller to afford the Organisation for Economic Co-operation and Development's (OECD) lower user mobile basket from the incumbent operator in the area (R90 (US$8.01) for 40 calls/monthFootnote3), they would need to use 11.25% of their monthly income on mobile phone services. Very little is known about the telecommunication expenditure of traditional rural dwellers. In other words, it is uncertain if rural residents spend R90 (US$8.01) on telecommunications a month. Only Bidwell et al. (Citation2011) provide some data and report a weekly expenditure of R13 (US$1.16) in 2010. The authors also report that rural dwellers in these areas incur additional expenses to communicate, such as paying for charging the mobile phone battery and additional mark-up added by the local airtime resellers. Similarly, there is little data on Internet expenditure for rural dwellers. One study argues that 50% of respondents are not using the Internet because they consider it too expensive (Gillwald, Moyo, & Stork, Citation2012).
This paper bridges this research gap by providing a unique in-depth study of the communication expenditure and patterns of a rural community in South Africa. This research can be used by regulators and government agencies to better design policy implementations to provide universal service and access, by competing industry players to understand the dynamics within rural communities to better target their products and by civil society organizations to use it as a case study in efforts to make affordable communications a constitutional right (Right2know, Citation2013).
In order to provide a more systemic look at understanding communication expenditure and patterns of usage, this paper uses the concept of the ICT ecosystem (Fransman, Citation2007). The paper is organized as follows: in Section 2, the concept of ICT ecosystem is introduced along with the different actors who play a role in rural areas. Section 3 describes the methods followed to answer questions about expenditure and communication patterns in a specific rural community. The results are presented in Section 4. In Section 5, the results are discussed, and Section 6 concludes the paper.
2. The ICT ecosystem
The use of ecological metaphors to describe complex systems has grown over the past two decades, and this terminology has been linked to Moore's suggestion that businesses operate in symbiotic relationships with one another, with their customers and other economic actors (Moore, Citation1993, p. 76).
If this proposition is appropriate for ICT, then as with any ecosystem we might expect that the ICT ecosystem would comprise many mutually interacting parts, heterogeneous in their arrangement and characterized by interdependence (Bertalanffy, Citation1968). These components are arranged in sub-systems, each with their own networks and dynamics (Saaty & Kearns, Citation1985).
In this paper, the model presented by Fransman (Citation2007), reproduced in , is used. Apart from the four groups of players included in (network element providers; network operators; content and application providers; and final consumers), Fransman also includes institutions and organizations in the environment where those players interact. Institutions described are those who “shape the rules of the game according to which the players interact and influence their behaviour” and include standardization and regulation bodies and financial markets. Organizations are described as those who “have the power to change institutions although they themselves are influenced by the institutions they are changing” (Fransman, Citation2007, p. 2).
Figure 1. The new ICT ecosystem (Fransman, Citation2007).
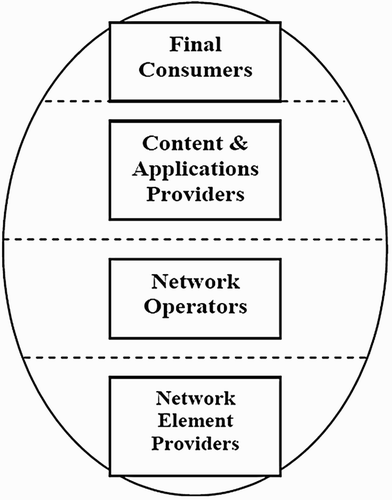
Although other groups of players may also play a role in the ICT ecosystem of rural areas in South Africa, this paper focuses on the two groups of players we believe to be most important for the discussion: the network operators – in particular the mobile network operators (MNOs), which are the predominant access providers used in rural areas – and the final consumers. Similarly, with the institutions and organizations, we only discuss the role played by the regulatory bodies. For the final consumers, we focus on the community where the study has taken place to provide the background for the results presented later in the paper.
2.1. The mobile network operators
In South Africa, there are four mobile network operators (MTN, Vodacom, Cell C and Telkom Mobile) and one virtual operator (Virgin Mobile). However, two operators, MTN and Vodacom, jointly command a market share of more than 80%.
The reduction of the mobile termination rates (MTR) (see below) has allowed smaller operators to reduce their prices in an attempt to gain some market share. Cell C has managed to use it to win a considerable market share previously belonging to MTN (McLeod, Citation2014), forcing it to bring the prices down in response. Vodacom has managed to maintain its dominant position by using “innovative new ideas” (McLeod, Citation2015) without having to bring the price of its products down.
Using an international benchmark for price, namely the OECD prepaid low-usage mobile basket (40 calls per month), Research ICT Africa (RIA) has been compiling data about the cheapest low-usage basket offered by each MNO.Footnote4 In its data, the reduction by MTN (from R122.00 (US$10.86) to R90.00(US$8.01)) can be observed clearly. Still, there are no data about the uptake of the different products offered by an operator, and other products such as those implementing “dynamic pricing discounts (where prices are determined on the basis of real-time traffic assessment within a particular cell)” (Gillwald et al. Citation2012) may have been more popular for the users. In such products, it is difficult to calculate the price per minute. For instance, with MTN Zone the price per minute, both off-net and on-net, is R2.50 (US$0.22), but users get a random discount up to 100%.Footnote5 Making some assumptions, RIA established that when using this product users pay R167.00 (US$14.86) for their low-usage basket.Footnote6
2.2. The regulatory environment
In a study from 2009, the South African Department of Communications (DoC) compared the cost, usage, access and quality of voice and data services in South Africa with those of five countries with similar economic indicators (Brazil, Chile, Korea, Malaysia and India). Their comparative study concluded that “South Africans talk less on their mobiles than any of the other peer countries” despite having the highest mobile penetration in the group (DoC, Citation2009). The same study pointed at the high MTR that mobile phone companies were charging others to terminate calls in their networks. These conclusions forced the Independent Communications Agency of South Africa (ICASA) – the national regulatory body – to take action and regulate the MTR, agreeing on a glide path that would reduce the MTR to R0.40 (US$0.036) per call by March 2013.
Although the reduction of the MTR has enabled smaller operators to provide off-net prices more cheaply than the on-net prices of the incumbents, forcing at least one of the latter to bring their prices down to retain customers, prices have remained high when compared to other African countries (Gillwald & Calandro, Citation2014). It is argued that the effects expected did not happen due to the MTR in South Africa being high compared to their real costs. Additional reductions in the MTR are considered to be required to create the competitive environment that will eventually bring the prices down (Gillwald & Calandro, Citation2014). Following this recommendation, ICASA designed a new glide path to cut down the MTR to R0.10 (US$0.01) by March 2016. Since March 2014, the MTR for calls from the smaller players (Cell C and Telkom Mobile) to the incumbent providers (Vodacom and MTN) has decreased to R0.20 (US$0.02). Smaller players benefit from an asymmetric rate in order to foster competition, and terminating the calls in their networks cost R0.44 (US$0.04) for incumbents, with a five-year glide path towards symmetry (Gillwald & Calandro, Citation2014).
2.3. The final consumers
Mankosi is a traditional rural community in the Eastern Cape province of South Africa. It comprises 564 households in 12 villages spread across 30 km2 of very hilly land. Families are composed of up to five adults and seven children, with a reported household average size of 6.02 people. Thus, Mankosi has an estimated local population of 3395 people, out of which 2070 (60.9%) are 15 or older. These data correlate with the data from StatsSA for the Nyandeni local municipality, where 59.6% of the population are 15 or older. The distribution of the age in the respondents greatly resembles the one observed by the local municipality.Footnote7
Most of the population (70.6%) in Mankosi is female. This contrasts with the 56.8%Footnote8 female distribution in the local municipality statistics (StatsSA, Citation2012a, p. 68). The higher concentration of men who stay and work in the urban areas of the local municipality explains this discrepancy.
Families live in homesteads: clusters of thatched, mud-brick rondavels, an occasional tin-roofed 2-room dwelling, an animal corral and a garden for subsistence crops. At the household level, it is only since 2013 that the government has built pit latrines in the yards of all the households. Most affluent people use their flat-roofed housing to collect rain water, although access to water for many is mainly via water points in communal land, and it is very common to see women carrying buckets in their heads (95.4% of the households use this method). With cooking, most families use open fires outside, with wood collected from the nearby indigenous forests, as they can only afford gas or paraffin from time to time. Eighty-four percent in Mankosi do not have electrical lighting infrastructure. Only 2.1% are connected to the grid, and only 13.5% have a generator, solar or gas infrastructure of some sort. This is similar to what has been observed by the authors in other rural communities in the Eastern Cape.
The reported household income per month is R1831 (US$163), coming mainly from government grants and payments from family members who temporarily migrate for work. Thus, the reported individual's monthly income in Mankosi is R388; that is, people in Mankosi live on roughly US$2 a day.Footnote9 This income is mainly used to cover the credit accumulated during the month in the local shop, which receives the outstanding debt the moment the government grant is received. Thus, very few households have savings and see no reason to open a bank account (58.6% of the households do not have one). This income contrasts with the R2587 (US$230.3)Footnote10 of monthly individual income, which is the average of the country (StatsSA, Citation2012b). Due to the high levels of inequality in South AfricaFootnote11 and the average disposable income at local municipality levels (StatsSA, Citation2012a), many other rural communities are expected to show disposable income levels similar to those of Mankosi.
Regarding formal education, only 13% of the population has completed matriculation (grade 12) or a higher level of education. The reported capacity to read and write isiXhosa is considerably higher (above 70% of respondents report doing so easily) when compared to the ability to read and write English (above 32% of respondents report not ability at all in both), with older people reporting the lowest levels.Footnote12
In Mankosi, as in 7218 communities in the country (of which 1192 are in the Eastern Cape) inhabitants are governed by a Tribal Authority (ICRPOB, Citation2011), which in Mankosi consists of the Headman, 12 Subheadmen (one per village) and messengers. The Headman and Subheadmen's homesteads are sites for local administration (Bidwell et al., Citation2013).
Recently, a non-for profit locally owned co-operative, Zenzeleni Networks Ltd., has been established in the community. Its main goal is to reduce the cost of communications of the community members. Since September 2014, the cooperative is a legal Internet Service Provider (ISP) (Rey-Moreno, Tucker, Cull, & Blom, Citation2015b) and is offering access to telephony services at a fraction of the price offered by MNOs from 12 public phones installed in the community. In August 2012, adapting the idea introduced in the community by Bidwell et al. (Citation2013), 8 of these public phones started operating as charging stations where community members can charge their phone batteries cheaper than in the options available before then. The records starting in July 2013 show that more than 3300 phones have been charged there since then (Rey-Moreno, Sabiescu, Siya, & Tucker, Citation2015a).
3. Methodology
The data presented in this paper (including the context information presented above) come from using a stratified sampling survey of the households in the 12 villages that comprise Mankosi. The total number of households per village was obtained by asking the subheadmen about “pieces of land”Footnote13 that they had allocated in their villages, as they keep a register.
The sample size was determined by setting the confidence level to 95% with a margin of error of 5%. Given a population size of 564 households, a sample of 224 was calculated. For a possible 10% non-response rate, the sample was increased to 250. The sample size within each strata (village) was calculated to be proportionally similar to ensure representativeness within the community surveyed.
The data presented in this paper come from two different surveys conducted in the community:
The first survey was conducted between July 2012 and January 2013. It was collected in paper format adapting the Household Survey from RIA (Stork & Stork, Citation2008). It consisted of the Household Roster being answered by the head of the household and an individual part being answered by a randomly selected individual. The data were collected by six different Local Researchers in three different periods. A total of 247 questionnaires were administered.
The second survey took place between December 2013 and January 2014. It was collected by a team of five Local Researchers using Open Data Kit (ODK). The questionnaire consisted of a reduced version of the first questionnaire, adapted based on communication patterns learned via participant observation and ethnography. A total of 255 questionnaires were administered.
Within each village, each household was selected randomly, choosing the first household found along a footpath and skipping the next. Within the household, the individual interviewed was also randomly selected. Two different techniques were used in the two surveys:
In the first one, the selection of the participants within the household followed the same procedure used by RIA, asking the date of birth of all the household members older than 15 years old and selecting the individual with the next birthday from the day the questionnaire was being administered.
In the second one, a random number generated by ODK was used. Every person 15 years old or older present at that moment in the household was given a number, and the person given the number shown by ODK was asked to give consent. If accepted, the questionnaire was administered. If not, the process was repeated again, excluding that person.
Due to inconsistencies discovered during the data-cleaning process of the first questionnaire, in-depth interviews were conducted with the surveyors. Although the randomization of the households was done properly, cultural factors were overlooked. This prevented the proper randomization of individualsFootnote14 inside each household and so the individual data are not representative of the population in Mankosi. Thus, only information from the Household Roster of the first questionnaire is used in this paper.
Additionally, the paper builds on ethnographic data collected by the principal author, who has lived in Mankosi for more than 15 months. During this period, the author has additionally visited nearby communities, and engaged in countless formal and informal conversations with their members about mobile phone ownership, usage and expenditure. This includes structured interviews to understand the sense of ownership of the local cooperative described in Section 2 (Rey-Moreno et al., Citation2015a) and the co-design of a billing system for the voice services provided by the cooperative (Rey-Moreno, Ufitamahoro, Venter, & Tucker, Citation2014).
4. Results
4.1. ICT ownership
In terms of ICT ownership per household, 97.7% of the households owned at least one mobile phone, with an average of 2.93 mobile phones per household. This contrasts with the ownership of a computer and a landline, where none of the households owned one.
At the individual level, 81.2% of the people surveyed owned a phone. Of them, 73.4% bought it and 26.6% received it from someone else. On average, people have had a phone for 4.09 years, but with a very high uptake in recent years, as observed in .Footnote15
Years owning a phone correlates positively with age, with older people owning phones for longer (ρ = 0.152 (n = 202), p < .05). Additionally, there is a difference in the age (U = 2997, p = .003) of the people who have bought their phones (Md = 27) and those who were given phones (Md = 21). Due to access to more employment opportunities, older people are more likely to have the economic resources to buy mobile phones than younger ones.
In relation to preferences about features in their mobile phones, many reported a preference for longer battery life (preferred by 58.0% of individuals). This is followed by the phone providing Internet capabilities (18.3%) and the possibility to play music (15%). As shown in , older people prefer longer battery life, whereas younger people are more interested in bigger storage capacity, music reproduction and Internet capabilities.
Table 1. Median comparison [Kruskal–Wallis] among categories in variable “Main feature you wish your phone had” and “Age.”
With regard to SIM card ownership, 83.9% of the respondents owned one, in contrast to the 81.2% of people who owned a phone. From those, 93.9% of SIM cards belonged to MTN, 5.6% to Vodacom and 0.5% (1 respondent) to Cell C.
4.2. ICT expenditure
Those owning a mobile phone were asked about the amount of money spent on charging it in the last month. People reported an average expenditure of R10.36 (US$0.92) a month. However, there is a large dispersion in the responses, as seen in . Forty-nine percent of the respondents reported R0 of expenditure. This is explained by the different levels of access to a place where phones can be charged for free. Some households are connected to the main power grid or have solar systems specifically installed for charging mobile phones, where not only household members, but relatives and friends also charge phones. Additionally, some locals work in the cottages owned by affluent fishermen from the cities, the main backpackers or other places (including the school and the high school) where one can charge at no cost.
Those who reported owning a SIM card were asked how much airtime they purchased in the last week, and how much they paid for it. On average they spent R17.49 (US$1.55) for a total of R15.28 (US$1.36) airtime, reflecting the mark-up reported previously by Bidwell et al. (Citation2011). This mark-up increased on average 17.19% the cost of communication for Mankosi dwellers. This mark-up is common in rural areas and has been observed in many other communities visited, and it is related to the increase on the prices that other products (like milk) suffer in rural areas to compensate for the cost of transport.
It is worth noting that 93.2% of the individuals reported topping-up R40 (US$3.56) or less, including 18% of those who reported not having topped-up their phone in the past week. Thus, on average mobile phone users in Mankosi received only R0.58 (US$0.05) in promotionsFootnote16 during the previous month. The money received in promotions correlates positively with the airtime topped-up (ρ = 0.196 (206), p < .01). However, due to the low amount topped-up, very few people (all of them with MTN SIM cards) received money in promotions (10.7% of those with a SIM card). It is worth noting that the MTN product observed to be used by the majority of people is MTN Zone. With this product, discounts come as a percentage of discount over the normal rates. In the main author's experience – observing the discounts received by others – they are regularly 20%. Sometimes they receive 100% discount of the total cost of the call; when this happens, they tell their friends as it seems to be related with the base station covering the area, and it lasts only a few minutes.
There is a correlation between the number of years owning a mobile phone and the money spent on mobile phone services, both having it charged and topped-up, as shown in . Furthermore, both expenditures correlate with age. Given the big uptake of mobile phones in the community in recent years and the young age of the population, this data suggest that expenditure in mobile phone services in Mankosi will continue increasing in the coming years.
Table 2. Spearman correlations between “Age,” “Years owning a mobile phone,” “Money spent on charging last month” and “Airtime topped-up last week” (n shown in brackets).
The data in also suggests that people spending on one mobile phone service (for example, charging) are willing to spend more on another (for example, airtime). This is further corroborated by the difference in expenditure between those who bought their phones, and those who were given phones as shown in . People buying their phones clearly spend more on mobile phone services.
Table 3. Median comparisons [Mann–Whitney] between those who bought their phones and those whose phone was given.
Additionally, people not only topped-up their own mobile phone, but they also used someone else's mobile phone during the week previous to the questionnaire. Sixteen percent of all respondents reported to have used someone else's phone in the prior week. This group included people not owning either a phone or a SIM card. Thus, 86.66% of the population used mobile phone services during the week prior. Out of those using someone else's phone, 56% (9% of the total) reported topping-up that phone with airtime for their own use, spending on average R6.65 (US$0.59) that week. It is unclear whether this amount was considered in the total amount reported as having been spent on airtime in the previous week. In this study it is considered as if it were.
People not only bought their own airtime, but they were also transferred airtime from wealthier relatives working outside the community. From those owning a SIM card, 54.7% reported having received airtime in the last week, for an average value of R4.87 (US$0.43).
Respondents were asked about the reasons for buying airtime. Most people (79.5%) used the airtime to make social calls only. As this was an open question, it is worth noting that some of the people reported these social calls to be made in order to be in contact with the people they care about, who are living outside the communities (including wives/husbands, children and other relatives). As observed in , older people used it for social calling and official calling, whereas younger people used it additionally for SMS, social Internet and music downloads.
Table 4. Median comparison [Kruskal–Wallis] among categories in variable “Reasons for buying airtime” and “Age”.
The respondents who owned a phone, a SIM card or who topped-up someone else's phone were also asked whether they sacrificed on other things to buy airtime and 41.2% reported affirmatively. From those, 34.5% reported sacrificing on essential food items, 16.7% for essential items for cooking and lighting and an additional 2.4% on both. As observed in , older people are more likely to sacrifice essential food and cooking and lighting, whereas younger people are more likely to sacrifice more luxurious items.
Table 5. Median comparison [Kruskal–Wallis] among categories in variable “Items sacrificed for airtime” and “Age.”
Considering the money spent for buying airtime in a week and on charging the mobile phone in a month, and extrapolating the money spent on a week to a monthly expenditure,Footnote17 the average monthly expenditure in mobile phone services was R85.21 (US$7.59). With the people older than 15 years using a mobile on a weekly basis showed above, a rural community like Mankosi spends around R153,000 (US$13,620) monthly using their mobile phones. Only 76.76% of this estimated individual monthly amount is for actual airtime used for MNO services.Footnote18
When comparing this expenditure with the average monthly income reported above, people in Mankosi spend 21.97% of their income on mobile phone use. If the disposable income does not increase, this percentage is likely to be higher with time due to the correlations with age and years owning a phone.
4.3. Mobile phone use
With regard to mobile phone use, the first variable studied was how many times people charged their phones during the last week. People in Mankosi charged their phones at least once a week, with 35.8% charging their mobile phones three or more times a week. The second variable took into consideration the number of days without a charged battery a month; 41.1% of the respondents reported not having had their phone without battery a single day in the last month. Still, the average was 1.95 of days without battery a month.
Then, respondents with phones were asked about the number of days they did not have airtime on their SIM cards last week. It is worth noting that on average people reported being without airtime 2.78 of days a week, i.e. people in Mankosi reported to be on average 39.71% of their time without airtime. So, even though people have their phone charged most of the time, they were unable to make calls or send SMS for a large portion of their time.
The days without battery in the last month correlates negatively with the airtime top-up last week (−.148, p < .01, n = 202) and the days without airtime in the last week (−.199, p < .01, n = 199). This suggests that the more the phone was charged, the more they spent, and the quicker they did so.
For obtaining the number of calls made during the last week and the length of these calls, interviewers obtained the readings directly from the person's mobile phone. Since not all participants carried their phone with them or had battery in the moment of the interview, only 195 responses for the variable “number of calls made last week” were collected. For the length of the calls, the number was even smaller as some of the local researchers added up the total number rendering values that did not make sense (e.g. 39 hours … ); these numbers were modified to compute as missing. Thus, a total of 141 answers were used to calculate this variable, as well as the average length of each call. According to the data, people in Mankosi made an average of 4.35 calls a week. The average total length of the calls reported was 17.95 minutes, with an average length per call of 3 minutes 20 seconds.Footnote19 A large proportion of people made shorter calls as shown in , even though they were made for social calling as reported earlier. The longest calls are associated with users who reported having received big discounts (90–100%) when using MTN Zone, and as it can be observed they represent the minority of the calls.
presents the strong positive correlations between the amount topped-up in the last week and the number of calls made, total minutes called, average call length, SMS sent last week and money spent on bundles in the last month. The same trend (and correlation) is shown by the other variables related to increased airtime (airtime transferred by others, airtime topped-up in others’ phones and airtime received in promotions). These results, although apparently obvious (the more people are willing to expend in communications the more they can communicate) show the important role played by the cost of the airtime (including the mark-up), especially for those who cannot afford it.
Table 6. Spearman correlations for “Days without airtime last week” and “Airtime topped-up last week” with communications-related variables (n shown in brackets).
This trend is further corroborated by the days without airtime as shown in . The more the number of days without airtime, the less people communicated. It is worth highlighting that in the absence of airtime, people still used PleaseCallMe.Footnote20
Additionally, as shown in , there is a strong positive correlation between communication indicators, suggesting that communication methods reinforce each other. This is relevant to the notion of an ICT ecosystem in which the components (i.e. the communication methods shown in ) form symbiotic relationships (i.e. reinforce each other).
Table 7. Spearman correlations among communications-related variables (n is shown in brackets).
Then, people were asked about the number of calls they made to people in Mankosi, the number of SMS and PleaseCallMe sent last week, whether they had had problems with the operators signal in the last week, and whether they had used Internet on their phones in the last month. These questions were only asked to people having a phone, a SIM card or someone who reported having used someone else's phone last week. Thus, a total of 221 answers were considered. The number of valid answers for the number of PleaseCallMe was later reduced to 217, as there were 4 respondents who reported values over the maximum limit allowed by their mobile phone company.Footnote21
People reported to call mainly to someone outside Mankosi, with only 26.7% reporting that “Most” or “All” their calls were made to people locally, thus corroborating that they mostly communicate with their relatives outside the community. With regard to the number of PleaseCallMe, 67% of the people reported using the service, with an average of 9.98 sent in the last week. This contrasts with the number of SMS sent last week, where 41.7% reported to have not sent any in the last week, and the average was 1.75. In both cases, older people use these services less (see for more details), which is associated to the lower capacity to read and write IsiXhosa and English reported earlier.Footnote22
Table 8. Median comparison [Kruskal–Wallis] among categories in variable “Age” and services used.
Thirty-nine percent of the respondents reported having problems with the signal of the operator during the last week when making or receiving calls (something the researcher has experienced personally on several occasions).
With regard to Internet use, 22.2% of the respondents reported having used it in the last month. From those, 42.9% reported to have bought data bundles in the last month. Among them, the average expenditure on data bundles in the previous month was R12.15 (US$1.08). There is a strong correlation among the money spent on bundles in the last month with the average call length (.609, p < .05, n = 19) and the number of SMS sent in the last week (.534, p < .05, n = 20). This suggests again, as presented in , that the more people communicate, the more they are inclined to use all means available to do so. Those reporting to have used Internet on the phone in the month previous to the questionnaire were significantly younger (Md = 21.0), than those who have not (Md = 28.0).
As in the case of the PleaseCallMe and SMS, a Chi-square test for independence (with Yates Continuity Correction) indicated significant association between the use of Internet and the ability to read and write English and IsiXhosa, as shown in . All cross-tabulation between those who used the Internet last month, who answered “Easily” in any of the four variables regarding ability (read IsiXhosa, write IsiXhosa, read English and write English), showed values over 71%.
Table 9. Chi-square test for independence between the “use of Internet on the phone last month” and the ability to read and write English and isiXhosa.
Finally, people were asked about whether they thought there was something preventing them from communicating more, and if so, to mention the factors. A total of 44.3% of the respondents answered positively. The combination of lack of battery, bad signal and lack of airtime represented 92.3% of the answers, with lack of airtime alone being the factor preventing communications to 46.2% of the respondents.
5. Discussion
This section discusses the nuances of networks and dynamics existing within the consumers' component of the ICT ecosystem. Then, it provides a detailed discussion of the interaction between the three players considered within the ICT ecosystem in rural areas of South Africa: MNO, regulator and consumers.
5.1. Networks and dynamics within the consumer sub-system
Access to mobile services in rural South Africa is very high, with almost all households (97.7%) of Mankosi owning a mobile phone. Individual ownership among people is 81.2%, which is a considerable increase from the 66.6% reported in Bidwell et al. (Citation2011) three years earlier. An additional 5.66% access mobile phones either from using a SIM card on someone else's phone or by directly topping up someone else's SIM card, for a total of 86.6% of people older than 15 accessing mobile phone services on a weekly basis. Shared used of devices is consistent with other findings in rural areas of South Africa (Bidwell et al., Citation2011; Gunzo & Dalvit, Citation2012). Access to mobile phones contrasts with the low, or non-existent penetration of other devices, such as landlines and computers.
People in Mankosi report spending disproportionate amounts of their disposable income (21.97%) in communications compared with the national average (2%) (StatsSA, Citation2012b). As acknowledged by the respondents, this forces them, especially older people, to sacrifice on basic food and household items to use mobile phone services. Other studies concerning poor people in South Africa (Duncan, Citation2013) and elsewhere in Africa (Diga, Citation2008; Gillwald & Stork, Citation2008) have shown similar results.
A significant percentage of the total expenditure is used to keep the mobile phone charged. Considering the data reported in Bidwell et al. (Citation2011), people's expenditure on charging has decreased over the past 3 years. In December 2010, they were expending R5.50 (US$0.49) a week on average (R23.57 (US$2.1) a month), while in December 2013 they reported to be expending R10.36 (US$0.92) a month. This 56% reduction can be explained by a combination of two factors: (1) the installation by Zenzeleni Networks Ltd and previous initiatives (Bidwell et al., Citation2013) of cheaper and closer charging stations (R3 (US$0.27) per charge instead of R5 (US$0.45) (Bidwell et al., Citation2011)) and (2) more community members installing their own solar energy solutions to charge phones. Although there is no data to prove this as yet, qualitative findings suggest that the charging stations from Zenzeleni Networks Ltd have also contributed to reduce the number of days without battery per month. Before the installation of the charging stations, a mobile phone could go a week without being charged (Bidwell et al., Citation2013). The importance for people to have their mobile phones charged should not be disregarded, as shown by the number of days a month people reported not having battery. Mobile phones are not only used to make calls but they are also used as a torch at night, for listening to the radio and music, and, provided they had long periods of time without airtime, receiving urgent information from their relatives (news, job offers, etc). Then, it is no surprise that the main feature people wished to have on their mobile phones was a longer battery life. This again supports the idea of an ICT ecosystem, in which access to battery charging may determine usage and the capacity to communicate and to build knowledge, and how local people are creating ways for this to happen.
Expenditure on airtime has increased by 33.05% when compared to the data collected by Bidwell et al. (Citation2011) three years prior to the results presented in this paper. This corroborates the results obtained with regard to the correlation between years owning a phone and expenditure in mobile phone services, a trend which is expected to continue in the future due to the even higher uptake in recent years. Provided there is a negative correlation between the number of days without battery a month and the airtime expenditure in a week, the installation of the charging stations (which has reduced the number of days without battery) may have contributed to the increased airtime expenditure.
Airtime, as other products resold in rural areas (Dicks & Simons, Citation2013), includes a mark-up. This mark-up, which was previously identified by Bidwell et al. (Citation2011), has been quantified in this research and increases communication costs by 17.19%. The mark-up and the payment required to charge a mobile phone are not incurred in urban areas and account for 23.24% of the total expenditure in mobile phone services in Mankosi.
The high proportion of disposable income dedicated to communications (which does not even include the airtime transferred to individuals by others) is not correlated with high usage. On the contrary, with this expenditure people were on average able to send 7.49 SMS and make 18.6 calls a month. Similarly, the monthly expenditure in Internet services (R12.50 (US$1.11)) provides very constrained use: 30MB with Vodacom,Footnote23 and 25MB with MTN.Footnote24 In this regard, cost appears as the main factor limiting people to communicate, similar to the results reported in other studies (Elder et al., Citation2013), followed by lack of battery and bad signal. This constrained use of communications may serve to explain the high number of PleaseCallMe messages sent, which do not require having airtime, and can be personalized (Bidwell et al., Citation2011). However, its use is further limited by levels of literacy, which results in older people not benefiting from these services. A similar occurrence happens with the Internet services, where mostly young people use them due to the increased level of literacy required.
5.2. Inefficient interactions of players within the ICT ecosystem
To understand the high percentage of income (21.97%) dedicated to mobile phone use, the huge income inequality existing in the country and the high prices of mobile services need to be considered (Gillwald & Calandro, Citation2014). This value is considerably higher than the 5% that the government is targeting (RSA, Citation2010). Additionally, usage numbers are considerably below both the low-usage basket created by the OECD, which considers 40 calls a month (Gillwald & Calandro, Citation2014), and the total length of these calls that the government targets people to speak with the 5% of their disposable income, which considers 90 minutes a month (RSA, Citation2010). Our results revealed an average duration of only 76.82 minutes per month.
Efforts to reduce these costs are not reaching the people in Mankosi. For instance, all people reported using pay-as-you-go services due to the informal economy, and so they are not able to benefit from the discounts available when using contracts (Elder et al., Citation2013). Additionally, they top-up small quantities that do not qualify for the free airtime that MNOs randomly give when topping-up bigger amounts. Finally, the small number of big discounts might also be explained by the fact that most of the calls are made during peak-timeFootnote25 when it is not profitable for the operators to provide discounts. Products using dynamic pricing, like MTN Zone, with high uptake in rural areas, could provide some relief, but still the percentages of discount observed are not in line with the existent inequality.
Due to the high cost of communications in South Africa, the regulator introduced a reduction of the MTR (this reduction was announced after the data presented in this paper were collected). Although this initiative has already had an effect on cheaper packages available nationwide (Gillwald & Calandro, Citation2014) with smaller operators offering bigger discounts, the effect of these changes in rural areas is still to be seen; and several factors may hinder this endeavor. The lack of price transparency from incumbent MNOs reported elsewhere (Right2know, Citation2013) has been corroborated by initial data collected in Mankosi and shows that very few community members are aware of the price they pay per minute (Rey-Moreno et al., Citation2014). This, together with the low levels of numeracy and literacy reported in other research conducted in Mankosi (Dicks & Simons, Citation2013), affects consumer's perceptions of price, which may explain not only why 93.9% ownership of SIM cards from the most expensive provider in the country at the moment this study took place (Gillwald & Calandro, Citation2014), but also why they prefer one of their most expensive products, namely MTN Zone.Footnote26 Additionally, it has been reported that low-income consumers in Mankosi do not base their purchase purely on price. Habit and perception of quality are deeply entrenched with consumer's choices (Dicks & Simons, Citation2013). Finally, the possibility to access these discounted prices by alternative providers is advertised via channels accessible to very few.Footnote27 In the event of someone being aware of them, it requires him/her to go to the closest city to change to a different provider. Given that people do not go to the city very often, this may prevent them from benefiting from these discounts. Due to the high proportion of users from the same company, airtime resellers mainly sell airtime from MTN, and people with SIM cards from the other companies struggle to find airtime locally.
The lack of airtime from a given provider and the mark-up problems could be solved simultaneously if people used Internet banking, as most people do in urban areas. It has no mark-up associated, and allows topping up a number from any MNO. However this method has several requirements attached: (a) being aware of the service; (b) having a bank account and (c) having access to the Internet. Data about awareness have not been collected, but the low number of households with bank accounts (41.4%, presumably owned by older people) and the low uptake of Internet services (22.2%, mostly young people) limit this possibility.
Similarly, the literature has reported using PleaseCallMe (Bidwell et al., Citation2011) or using missed calls (Donner, Citation2007) to avoid costs associated with communications. One would expect that the same might happen with the use of mobile data. In South Africa, airtime can be used to purchase data bundles that, depending on their size, can be up to 720% cheaper.Footnote28 However, only 42.9% of the people using Internet use this mechanism.
6. Conclusions
This paper has presented an in-depth study of the ownership of mobile phone devices and the expenditure and usage patterns of the members of a rural community in South Africa. Results show a high access ratio (86.6% of people using mobile phone services weekly) and a high proportion of the disposable income (21.97%) dedicated to a very constrained set of mobile phone services (1.75 SMS and 4.35 calls, with a total length of 17.95 minutes, a week). Regarding data, only 22.2% reported having used mobile data in the last month, with a very constrained use of the services (25–30 MB a month).
The internal dynamics within the consumer sub-system, such as the mark-up added to the price of the airtime and the challenges for changing to a cheaper provider, may circumvent the efforts from the regulator to reduce communication costs. In the meantime incumbent MNOs are benefiting extensively from the need of illiterate consumers to communicate. In addition, components of the ICT ecosystem, perhaps taken as given in the urban context, may become binding constraints in the rural context. Access to battery charging is a noteworthy example in this study. Although the time spent without a charge appears minimal, it is likely that consumers manage their usage of ICT in order to minimize the risk of being without access.
Communities of consumers such as Mankosi, where the majority of people live on incomes below national and international poverty levels, are willing to spend more than R150,000 (US$13,6200) monthly on a constrained set of communication services. In it therefore heartwarming that Mankosi is starting to follow other examples in rural areas worldwide (Heimerl, Hasan, Ali, Brewer, & Parikh, Citation2013) and become producers of their own networks. In this context, community-owned efforts such as Zenzeleni Networks Ltd are worth exploring, and the data presented in this paper provide a solid baseline to measure its impact.
Acknowledgements
We would like to thank Jay, Thulani, Patheka, Phiwe, Nomphelo, Vhiwe, Sibongeseni, Patrick, Mapru and Sintle for assisting with the data collection. We also thank Nic, David, Steve, Christoph and Isabella for the hints during the design of the questionnaire and the discussion around it.
Disclosure statement
No potential conflict of interest was reported by the authors.
Notes on contributors
Carlos Rey-Moreno is a Post-Doctoral fellow in the Computer Science Department at the University of the Western Cape, South Africa. His research interest is focused on multidisplinary approaches to allow disadvantaged communities to reduce their cost for digital communications.
Rénette Blignaut is a professor in Statistics at the University of the Western Cape, South Africa. Her research interests include HIV and AIDS prevention, biostatistics, data mining and science education.
William D. Tucker, also known as Bill Tucker, is an Associate Professor of Computer Science at the University of Western Cape (UWC) in South Africa. His research group is called BANG, Bridging Application and Network Gaps, and conducts applied multi-disciplinary research into information and communication technology for development (ICT4D).
Julian May is the Director of the NRF-DST Centre of Excellence in Food Security at the University of the Western Cape. He has worked on options for poverty reduction including land reform, social grants, information technology and urban agriculture in Africa and in the Indian Ocean Islands. His current research focuses on food security, childhood deprivation and malnutrition.
Additional information
Funding
Notes
1. The exchange rate used throughout the article is R1.00 = US$0.089; that of the 8 November 2014 http://zar.fxexchangerate.com/usd/388-currency-rates.html.
2. Own calculations based on the data from StatsSA (Citation2012a).
3. Cheapest prepaid product for OECD basket by operator in South Africa in local currency (ZAR). http://www.researchictafrica.net/prices/country.php?c=20. Last accessed 6 August 2015.
4. Cheapest prepaid product for OECD basket by operator in South Africa in local currency ZAR): http://www.researchictafrica.net/prices/country.php?c=20. Last accessed 6 August 2015.
5. Price Plans: https://shop.mtn.co.za/crs/siteInformation/staticPage.jsp?breadcrumb=Pre-Paid%20Plans&siteInformation=PrePaidPlans. Last accessed 6 August 2015.
6. Since MTN does not release ARPU and MoU data, for comparative purposes Research ICT Africa has calculated MTN Zone price based on assumed dynamic discounts and the resulting price is more expensive than other price plans. See OECD low-usage basket price of MTN prepaid products at http://www.researchictafrica.net/prices/operator.php?o=65.
7. Considering the data available per age group in (StatsSA, Citation2012a, page 68) and in the data collected.
8. Number generated by the author considering the data available on gender per age group in StatsSA (Citation2012a).
9. This does not take account of Purchasing Power Parity which adjusts for the cost of living, and the figure would be closer to US$75 http://zar.fxexchangerate.com/usd/388-currency-rates.html; http://salaryconverter.nigelb.me/).
10. Number generated by the author considering the average annual income household and the average household size (StatsSA, Citation2012b)
11. GINI Index, World Bank, http://data.worldbank.org/indicator/SI.POV.GINI. Last accessed 8 November 2014.
12. A Kruskall–Wallis analysis shows significant difference between the three capacity levels and age. For “Not at all” medians are above 42 years, and “Easily” below 23 years for the 4 variables studied.
13. Piece of land is the term used among Xhosa people to refer to the space in the community that has been allocated by the Tribal Authority to a given family to build its household.
14. Cultural norms in the community include addressing questions to the head of the household, in this case the eldest woman.
15. For this variable, three values were declared as missing, as the interviewee should have entered the natural year when the phone was obtained, and the value in the cell was 0 instead.
16. Bonus airtime received, many times randomly, after topping-up prepaid airtime.
17. A month is considered to have 30 days, i.e. 4.28 weeks.
18. This amount can be higher if the airtime topped-up on someone else's SIM card was not considered as part of the airtime purchased last week.
19. The average total call-length does not equate the number of calls times the average call-length per call, due to the disparities on the sample size.
20. It allows sending a message through USSD containing the caller's cell-phone number and a 10-character text intended to identify the caller. Users can personalize this text once per day (Bidwell et al., Citation2011).
21. In the moment, the data were collected the maximum number of PleaseCallMe per day allowed from MTN in a day are 5 and 10 from Vodacom. http://mypleasecallmeback.blogspot.com/2013/02/call-me-back-cell-cvodacommtnvirgin.html Last accessed 8 November 2014.
22. A Kruskall–Wallis analysis shows significant difference in the number of SMS and PleaseCallMe sent among the three ability levels in the four variables regarding reading and writing. People with reported ability “not at all” present in all tests the lowest value.
23. http://www.vodacom.co.za/mobile/main/datapromotion Last accessed 22 August 2014
24. https://www.mtninternet.co.za/getit/Pages/InternetBundles.aspx Last accessed 22 August 2014
25. Most rural activities take place during day light.
26. OECD low-usage basket pricing of different MTN prepaid products http://www.researchictafrica.net/prices/operator.php?o=65 Last Accessed 5 September 2015.
27. Cell-C offered the possibility to call for 50 cents to any network, but it was only advertized via their website. http://www.cellc.co.za/explore/newsroom/cell-c-launches-50c-per-minute-prepaid-call-rate. Last accessed, 8 November 2014.
28. Out of bundle costs are R2(US$0.18) per MB. http://www.vodacom.co.za/vodacom/shopping/plans/1gb-30-day-once-off-prepaid-data-bundle.
References
- Aker, J. C., & Mbiti, I. M. (2010). Mobile phones and economic development in Africa. Journal of Economic Perspectives, 24(3), 207–232. doi: 10.1257/jep.24.3.207
- Bertalanffy, L. (1968). General systems theory. New York, NY: George Braziller.
- Bidwell, N. J., Lalmas, M., Marsden, G., Dlutu, B., Ntlangano, S., Tucker, W. D., & Elizabeth, P. (2011). Please call ME.N.U.4EVER: Designing for ‘Callback' in rural Africa. In Donald Day (Ed.), Proceedings of the 10th international workshop on internationalisation of products and systems (pp. 117–125). Kuching, Malaysia: Product & Systems Internationalisation.
- Bidwell, N. J., Siya, M., Marsden, G., Tucker, W. D., Tshemese, M., Gaven, N., … Eglinton, K. A. (2013). Walking and the social life of solar charging in Rural Africa. ACM Transactions on Computer–Human Interaction, 20(4), 1–3. doi: 10.1145/2509404.2493524
- Dicks, E., & Simons, S. (2013). Value perceptions of fast moving consumer goods: Study of low-income rural consumers in South Africa. (unpublished Honors dissertation). University of Cape Town, Cape Town, South Africa.
- Diga, K. (2008). Workshop on the role of mobile technologies in fostering social development. Sao Paolo, Brazil: World Wide Web Consortium.
- DoC. (2009). International peer benchmarking study on South Africa's ICT sector. Retrieved September 14, 2015, from https://pmg.org.za/files/docs/091020telecoms.pdf
- Donner, J. (2007). The rules of beeping: Exchanging messages via intentional “missed calls” on mobile phones. Journal of Computer-Mediated Communication, 13, 1–22. doi: 10.1111/j.1083-6101.2007.00383.x
- Donner, J., & Walton, M. (2013). Your phone has internet-why are you at a library PC? Re-imagining public access in the mobile internet era. In Proceedings of the Human–Computer Interaction–INTERACT 2013, 347–364. Berlin: Springer.
- Duncan, J. (2013). Mobile network society? Affordability and mobile phone usage in Grahamstown East. Communicatio: South African Journal for Communication Theory and Research,, 39(1), 35–52. doi: 10.1080/02500167.2013.766224
- Elder, L., Samarajiva, R., Gillwald, A., & Galperin, H. (2013). Information lives of the poor. Fighting poverty with technology. Ottawa, Canada: IDRC.
- Fransman, M. (2007). Innovation in the new ICT ecosystem. Communication & Strategies, 68 (4), 89–110.
- Gillwald, A. (2005). A closing window of opportunity: Under-serviced area licensing in South Africa. Information Technologies and International Development, 2(4), 1–19. doi: 10.1162/154475205775249364
- Gillwald, A., & Calandro, E. (2014). SA prepaid mobile prices plateau. RIA Policy Brief Feb 2014(1) South Africa.
- Gillwald, A., Moyo, M., & Stork, C. (2012). Understanding what is happening in ICT in South Africa. A supply – and demand-side analysis of the ICT Sector. Evidence for ICT Policy Action Policy Paper 7.
- Gillwald, A., & Stork, C. (2008). Towards evidence-based policy and regulation: ICT access and usage in Africa. Research ICT Africa, 1(2), 45.
- Gunzo, F., & Dalvit, L. (2012, 28–29 February). A survey of cell phone and computer access and use in marginalised schools in South Africa. Proceedings of the M4D 2012, 232–246, New Dheli, India.
- Heimerl, K., Hasan, S., Ali, K., Brewer, E., & Parikh, T. (2013, 7–10 December). Local, sustainable, small-scale cellular networks. Paper presented at the 6th ACM international conference on information and communications technologies and development: Full Papers, 1, 2–12, Cape Town, South Africa.
- ICRPOB. (2011). Annual Report 2011.
- Johnson, D., & Roux, K. (2008). Building rural wireless networks: Lessons learnt and future directions. Proceedings of the WiNS-DR'08, San Francisco, USA, pp 17–22.
- Kreutzer, T. (2009). Generation mobile: Online and digital media usage on cellphones among low-income urban youth in South Africa. University of Cape Town. Retrieved March 7, 2016, from http://tinokreutzer.org/mobile/MobileOnlineMedia-SurveyResults-2009.pdf
- Mathee, K., Mweemba, G., Pais, A., Van Stam, G., & Rijken, M. (2007). Bringing internet connectivity to rural Zambia using a collaborative approach. Paper presented at the 2nd IEEE/ACM international conference on ICTD, 1–12, Bangalore, India.
- McLeod, D. (2014). MTN haemorrhaging market share. 19 February 2014, TechCentral. Retrieved September 2, 2015, from http://www.techcentral.co.za/mtn-haemorrhaging-marketshare/46537/
- McLeod, D. (2015). Cell C enjoying ‘massive’ growth. 19 August 2015, TechnCentral. Retrieved September 2, 2015, from http://www.techcentral.co.za/cell-c-enjoying-massive-growth/59102/
- Moore, J. F. (1993). Predators and prey: A new ecology of competition. Harvard Business Review, 71(3), 75–83.
- Navas-Sabater, J., Dymond, A., & Juntunen, N. (2002). Telecommunications and information services for the poor. (World Bank Discussion Paper No. 432), 1–118.
- Ó Siochrú, S., & Girard, B. (2005). Community-based networks and innovative technologies: New models to serve and empower the poor. In Making ICT work for the poor. United Nations Development Program. Retrieved from http://p-ced.com/reference/community-based_nets.pdf
- Republic of South Africa. (2010). Electronic communications act: Determination: Universal access to and universal provision of electronic communications services and electronic communications network services. Government Gazette, 85(32939), 3–6.
- Rey-Moreno, C., Sabiescu, A. G., Siya, M. J., & Tucker, W. D. (2015a). Local ownership, exercise of ownership and moving from passive to active entitlement: A practice-led inquiry on a rural community network. The Journal of Community Informatics, 11(2). Retrieved from http://ci-journal.net/index.php/ciej/issue/view/54
- Rey-Moreno, C., Tucker, W. D., Cull, D., & Blom, R. (2015b, May 15–18). Making a community network legal within the South African regulatory framework. Proceedings of the 7th international conference on information and communication technologies and development, Singapore, 57.
- Rey-Moreno, C., Ufitamahoro, M. J., Venter, I., & Tucker, W. D. (2014). Co-designing a billing system for voice services in Rural South Africa: Lessons learned. Proceedings of the 5th conference of computing for development (ACM Dev-5). San Jose, CA, USA. 5–6 December.
- Right2know. (2013). The right 2 communicate. Retrieved October 10, 2014, from http://www.r2k.org.za/wp-content/uploads/right2communic8_WEB.pdf
- Saaty, T. L., & Kearns, K. P. (1985). Analytical planning: The organisaton of systems, international series in modern applied mathematics and computer science, 7. Oxford: Pergamon Press.
- StastsSA. (2012a). Census 2011 municipal report: Eastern Cape”. Report No. 03-01-50. Statistics South Africa. ISBN 978-0-621-41460-8.
- StastsSA. (2012b). Income and expenditure of households, 2010/2011. Statistical Release No. P0100. Statistics South Africa.
- Stork, C., & Stork, M. (2008). ICT household survey methodology & fieldwork. Volume ONE. Policy Paper 1. Research ICT Africa. 2008.
- Westerveld, R. (2012). Inverse telecommunications: The future for rural areas in developing countries? In T.M. Egyedi, & D.C. Mehos (Eds.), Inverse infrastructures: Disrupting networks from below (pp. 187–207). Cheltenham, UK: Edward Elgar Publishing Ltd.