ABSTRACT
Background: Images are essential materials for assessment and rehabilitation in aphasia. Psycholinguistic research has identified name agreement (the degree to which different people agree on a particular name for a particular image) to be a strong predictor of picture naming in healthy individuals in a wide variety of languages. Despite its significance in naming performance and its impact across linguistic families, studies investigating the effects of name agreement in neuropsychological populations are limited. Determining the impact of name agreement in neuropsychological populations can inform us about lexical processing, which in turn can aid in development of improved assessment and rehabilitation materials.
Aims: To compare the naming accuracy and error profile in naming high versus low name agreement (HighNA and LowNA) images in people with aphasia (PWA) and in healthy Adults (HA).
Methods & Procedures: Participants were 10 PWA and 21 age- and gender-matched HA. Stimuli were black-and-white line drawings of 50 HighNA images (e.g., acorn, bell) and 50 LowNA images (e.g., jacket, mitten). The image sets were closely matched on a range of image and lexical variables. Participants were instructed to name the drawings using single words. Responses were coded into exclusive categories: correct, hesitations, alternate names, visual errors, semantic errors and omissions.
Outcomes and Results: HighNA images were named more accurately than LowNA images; the HA group had higher accuracy than the PWA group; there was a significant interaction in which the name agreement effect was stronger in HA than in PWA. In individual analyses, 7 of 10 PWA participants showed the group pattern of higher accuracies for HighNA, whilst 3 PWA did not. HighNA and LowNA images gave rise to more alternate names in HA than in PWA. There were also fewer visual errors, and more omissions, in PWA than in HA, but only for LowNA items.
Conclusions: Name agreement produced measurable differences in naming accuracy for both HA and PWA. PWA shows a reduced effect of name agreement and exhibit a different pattern of errors, compared to healthy controls. We speculate that in picture naming tasks, lower name agreement increases competitive lexical selection, which is difficult for PWA to resolve. In preparation of clinical materials, we need to be mindful of image properties. Future research should replicate our findings in a larger population, and a broader range of pathologies, as well as determine the executive mechanisms underpinning name agreement effects.
Introduction
Images form essential materials for assessment and rehabilitation in aphasia. Despite the importance of understanding how image properties affect picture naming performance in neuropsychological populations, there are surprisingly few relevant studies. This deficiency is in contrast to the large body of psycho- and neuro-linguistic research investigating the effects of lexical properties on naming latency and accuracy in both healthy and impaired populations. Visual complexity, concept familiarity, word frequency, imageability, age-of-acquisition and word length are some of the well-documented variables affecting picture naming (e.g., Indefrey, Citation2011; Johnson, Paivio, & Clark, Citation1996). In contrast, name agreement (the extent to which different people agree with a particular name for an object, e.g., Alario et al., Citation2004) has received less attention. However, name agreement has been shown to be a robust predictor of naming latency in healthy participants (Alario et al., Citation2004; Bonin, Peereman, Malardier, Méot, & Chalard, Citation2003) and to have a distinctive event-related potential (ERP) signature (Cheng, Schafer, & Akyurek, Citation2010). Effects of name agreement on naming latency are independent of frequency or age-of-acquisition (Vitkovitch & Tyrrell, Citation1995); name agreement is the strongest predictor of naming latency amongst a range of lexical variables (e.g., Alario et al., Citation2004).
Name agreement has also been shown to be a significant predictor for picture naming latency in a variety of languages including British English (Ellis & Morrison, Citation1998), Welsh (Barry, Morrison, & Ellis, Citation1997), Dutch (Shao, Roelofs, & Meyer, Citation2014), Icelandic (Pind & Tryggvadóttir, Citation2002), French (Bonin et al., Citation2003), Spanish (Cuetos, Ellis, & Alvarez, Citation1999), Greek (Dimitropoulou, Duñabeitia, Blitsas, & Carreiras, Citation2009), Japanese (Nishimoto, Ueda, Miyawaki, Une, & Takahashi, Citation2012) and Persian (Bakhtiar, Nilipour, & Weekes, Citation2013). For this reason, researchers have not infrequently controlled for name agreement effects, typically by using only items with high name agreement (e.g., Bormann, Kulke, Wallesch, & Blanken, Citation2008; Fieder, Nickels, Biedermann, & Best, Citation2014). However, despite its significance in determining naming performance and its impact across linguistic families, studies investigating the specific effects of high versus low name agreement (HighNA and LowNA) in neuropsychological populations are limited (Laiacona, Luzzatti, Zonca, Guarnaschelli, & Capitani, Citation2001; Rodríguez-Ferreiro, Davies, González-Nosti, Barbón, & Cuetos, Citation2009). Differential (or indeed similar) processing of images which vary in name agreement in patient populations can inform us about lexical processing in them and in healthy adults (HA). Such information will in turn aid development of better assessment and rehabilitation materials. In this research, we measure both accuracy, and errors profiles, in naming performance for HighNA versus LowNA images, for a group of individuals with aphasia and for healthy control participants. This research fills a significant gap in the neuropsychological literature on name agreement effects.
Name agreement for a given image is usually measured by assessing the number of different names given to that image across a number of participants. The greater the number of different names which an image elicits, the lower its name agreement (e.g., an image of a bee could be named as “bee”, “wasp”, “insect”). Images which elicit only a single name across all participants have the highest possible name agreement (conventionally, 100%). Thus, name agreement is a property of an image, not of a word, although some basic level nouns (e.g., book) are considerably more likely to have images of HighNA than are other images. Images with higher name agreement are named more accurately and more quickly than those with LowNA (e.g., Barry et al., Citation1997; Vitkovitch & Tyrrell, Citation1995). Vitkovitch and Tyrrell (Citation1995) suggested different categories of name disagreement for low-agreement items as follows: (1) multiple names (e.g., “sweater” or “sweatshirt” named for jumper), (2) abbreviations or elaborations (e.g., “TV” named for television) or (3) incorrect names (e.g., “celery” named for marrow). It has been argued that different types of name disagreements originate at different levels of processing within the lexical processing system (O’Sullivan et al., Citation2012; Vitkovitch & Tyrrell, Citation1995). For example, naming delay due to incorrect names has been suggested to have its source at the stage of object recognition, whereas naming delay due to multiple names has its source at the lexical retrieval stage. Cheng et al. (Citation2010) reported ERP data to support this distinction between forms of name disagreements.
Alario et al. (Citation2004) argued that name agreement effects occur between the conceptual stage and the lexical stage: LowNA pictures evoke a greater number of candidates for the name of the depicted object than do HighNA pictures; therefore, compared with HighNA pictures, it takes longer to eliminate competitors and select one specific name for LowNA pictures. This is in line with Johnson’s (Citation1992) suggestion that name agreement effects arise after object identification (because LowNA increases naming time, whereas reaction times based on object identification times are unaffected by LowNA). Johnson, Paivio and Clark suggested that name agreement effects occur “[during] name retrieval, response generation, or both” (Johnson et al., Citation1996, p. 119). Bonin et al. (Citation2003) followed Vitkovitch and Tyrrell (Citation1995) in identifying the two main sources of name disagreement as uncertainty of pictures and alternative names of depicted objects. Bonin et al. argued that in the case of picture uncertainty, name agreement effects occur while accessing stored structural knowledge, whereas if a picture has more than one alternative name, name agreement effects occur after conceptual access. Difficulty in resolving the heightened competition during lexical access has been put forward as a possible reason for greater difficulty in naming LowNA images (e.g., Cameron-Jones & Wilshire, Citation2007). Kan and Thompson-Schill (Citation2004) had found more left inferior frontal gyrus activity when their healthy participants named low-agreement pictures than when they named high-agreement pictures. By studying brain-damaged populations, such as PWA, we can investigate whether and how they resolve the heightened cognitive competition involved in processing LowNA items; the pattern of their error responses in such tasks offers a window on mechanisms of lexical response generation.
Experimental name agreement research in neurological populations has focused mainly on people with dementia, Alzheimer’s disease (Harley & Grant, Citation2004; Rodríguez-Ferreiro et al., Citation2009) or Primary Progressive Aphasia (Kremin et al., Citation2001). The main finding has been that name agreement is a significant predictor of naming accuracy, with LowNA having a detrimental impact on picture-naming accuracy. Relevant to the present study, Rodriguez-Ferreiro et al. analysed the errors of AD participants during picture naming, arguing that AD participants were more likely to make semantic errors when name agreement was lower, as a consequence of degradation in the specificity of the semantic system. Such degradation was argued by Rodriguez-Ferreiro et al. to result in selection difficulties particularly where multiple candidate names were in competition.
In aphasia naming literature, there exist only two published abstracts which have included name agreement as an experimental manipulation. The abstract of Laiacona et al. (Citation2001) reports a multiple regression analysis of picture naming performance from 49 individuals with aphasia. Name agreement was the single most influential variable in predicting accuracy, followed by word frequency. Unfortunately, Laiacona et al.’s study omitted to report parameter estimates, or any error analysis; nor were any data from healthy control participants included. Comparison with control participants would have facilitated examination of whether effects shown by PWA are result of typical processing or an indication of impairment (see Coltheart, Citation2001). Cameron-Jones and Wilshire’s (Citation2007) abstract presents data from four individuals with aphasia in three experiments comparing picture naming in low versus high competitive conditions. One of these experiments tested picture naming with images of high (i.e., low-competitive condition) versus low (i.e., high-competitive condition) name agreement. Qualitatively, all their participants showed better naming in the HighNA condition, but in only one non-fluent participant was this difference statistically significant. Similar to Laiacona et al., Cameron-Jones and Wilshire do not report data from healthy participants, neither do they provide error analysis.
From the ongoing review, it is clear that name agreement has a robust effect on naming latency and accuracy for healthy participants. It remains to be established whether PWA will show a similar pattern of performance on name agreement variation in picture naming tasks. Moreover, in the case of aphasia, both differential effects of HighNA versus LowNA, and analysis of error patterns, provide opportunities to investigate the lexical processing system in aphasia. In terms of the role of name agreement effects in theories of lexical access, there are (at least) two distinct possibilities: (1) if brain damage introduces additional statistical noise into the lexical system (i.e., results in a system in which signals are subject to increased moment-by-moment random variation), we might expect to see spurious boosting of candidate semantic competitors above threshold. This would lead to error responses involving alternate names, or to semantically related responses. (2) Alternatively, if brain damage instead causes excessive competition in the lexical network – and hence additional inhibition between items – it is likely the response type would be omissions or other random responses of a type less systematic than alternate name or semantically related responses.
We compared the performance (naming accuracy and error profiles) between a group of PWA and age-matched HA speakers, in a picture naming task, using high and low naming agreement items. We controlled the stimuli carefully on 15 relevant lexical or image variables, including measures of neighbourhood densities. The two sets of pictures did not differ statistically on any of these variables. The naming responses were transcribed and coded using a detailed taxonomy of candidate error types. Our specific research questions were
Does HighNA versus LowNA images affect the naming accuracy for PWA? If there is an effect, is it similar or different when compared between PWA and HA?
Do PWA and HA produce similar error profiles in respect of the difference between HighNA and LowNA images?
Method
Participants
Two groups, consisting of 10 PWA (6 males, 4 females) and 21 age-, gender- and education-matched HA control participants (13 males, 8 females) participated. Inclusion criteria for PWA were a single left hemisphere cardiovascular accident as determined by neuroradiological and/neurological examinations; a diagnosis of aphasia on standardised clinical tests (Boston Diagnostic Aphasia Examination; Goodglass, Kaplan, & Barresi, Citation2001); at least 8 months post-stroke; monolingual English speaker; no history of other neurological illness, psychiatric disorders or substance abuse; and no other significant sensory and/or cognitive deficits that could interfere with the individual’s performance in the investigation. presents demographic information, aphasia type and severity for each of the PWA. Ages of the PWA individuals ranged from 52 to 85 years (M = 67.2, SD = 10.7), level of education ranged from 11 to 18 years (M = 13.7, SD = 2.1) and post-onset to stroke from 1.2 to 16 years (M = 6.8, SD = 4.9). All PWA were pre-morbidly right-handed individuals. The healthy control group age ranged from 41 to 79 years (M = 62.8, SD = 9.3), and level of education ranged from 11 to 19 years (M = 14.3, SD = 2.5) consisted of right-handed monolingual native English speaking individuals with no reported history of speech, language or hearing problems or any other neurological deficits. There was no significant difference between the groups with regard to age (p = 0.25) or level of education (p = 0.48). Ethical approval was obtained in advance from the University Research Ethics Committee and written informed consent obtained for all participants.
Table 1. Demographic details, aphasia type and severity and performance (% correct) on language tasks for people with aphasia (PWA).
Background test battery
PWA were administered an extensive test battery to profile their semantic and phonological processes at the single-word level. This battery measured overall picture naming abilities, output phonological abilities and conceptual and lexico-semantic processing. Our approach was to obtain a good all-round understanding of the processing abilities of PWA in relation to the main experimental task. Individual PWAs’ performances on the semantic and phonological battery are given in .
Picture naming
Because the principal task in this study was picture naming, we thought it important to characterise participants’ picture naming outside the main experimental manipulation. To capture a potentially wide range of relative severities of picture naming effects in our participants, we used the Philadelphia Naming Test (PNT, Roach, Schwartz, Martin, Grewal, & Brecher, Citation1996). This test has a large number of items, comprising 175 pictures, the names of which vary from 1 to 4 syllables and reflect a wide frequency range in general usage. Naming responses were recorded and transcribed for accuracy analysis.
Output phonology tasks
To get a better idea of output phonological skills, tasks administered included the Psycholinguistic Assessments of Language Processing in Aphasia (PALPA) subtests of Word repetition: Imageability and Frequency (PALPA 9) and Nonword Repetition (PALPA 8, Kay, Lesser, & Coltheart, Citation1992). The Word Repetition (PALPA 9) investigated the effects of imageability and frequency (and their interaction) in auditory repetition and used 80 words divided equally among the following categories: high imageability/high frequency (e.g., mother), high imageability/low frequency (e.g., drum), low imageability/high frequency (e.g., idea) and low imageability/low frequency (e.g., bonus). The 30-item Nonword Repetition (PALPA 8) examined participants’ ability to repeat unfamiliar yet word-like sound forms in which length of the utterance was varied systematically from one to three syllables (10 items in each syllable length). Although syllable length was manipulated, phoneme length was constant across the items. This task probed the integrity of the sub-lexical acoustic-phonological conversion route.
Conceptual and lexico-semantic processing tasks
Measures of semantic processing included the following tasks: the 3-picture version of the Pyramids and Palm Trees Test (PPT, Howard & Patterson, Citation1992), PALPA 47 (Spoken word-to-picture matching), PALPA 49 (Auditory synonym judgment) and Synonymy Judgements with Nouns and Verbs (Saffran, Schwartz, Linebarger, Martin, & Bochetto, Citation1987). The 3-picture version of the PPT tested nonverbal conceptual semantics, by which participants were shown three pictures and was required to judge which of the bottom two pictures (e.g., palm tree and pine tree) was associated with the top (pyramid). In spoken word-to-picture matching (PALPA 47), participants matched a target word presented verbally by the examiner (e.g., carrot) with its matching picture, amongst an array of distractor pictures related semantically (e.g., cabbage or lemon), visually (e.g., saw) or not related at all (e.g., chisel). The 60-item auditory synonym judgment (PALPA 49) tested participants’ ability to judge whether two spoken words were close in meaning (e.g., story-tale vs. tool-crowd) meaning. This task also assessed performance on high- and low-imageability words, which were matched for frequency. The Synonymy Judgements with Nouns and Verbs (Saffran et al., Citation1987) test included 30 triplets of words: 15 noun triplets (e.g., violin, fiddle, clarinet) and 15 verb triplets (e.g., to repair, to design, to fix). The participant viewed three written words that were spoken aloud by the examiner and decides which two were most similar in meaning. No information was provided about the meaning of the words.
In summary, our group of PWA showed several types of aphasia (three Broca’s, two Anomic, three Conduction, one Transcortical motor and one Mixed nonfluent). Based on BDAE aphasia severity rating of 1–5, the aphasia severity ranged from 1 to 4 (M = 2.5, SD = 1.2), with 1 indicating most severe. The participants had a wide range of picture naming abilities as demonstrated by performance on PNT (ranged from 33.7% to 91.4%, M = 74%, SD = 17.1). As a group, they showed relatively better repetition of words (M = 92%, SD = 11) compared to nonwords (M = 65.3%, SD = 26). Their conceptual and lexical semantics were better preserved (as indicated by performance on PPT, PALPA 47 and 49), notwithstanding some difficulties, in Synonym judgment task with nouns and verbs. Individual variability was noted amongst the participants on the semantic and phonological tasks. In sum, PWA constituted a group with heterogeneity, typical of the aphasia population, in respect of aphasia severity, naming difficulties and performance on semantic and phonological batteries.
Stimuli and materials
The experimental stimuli include 50 HighNA images (HighNA, e.g., acorn, bell, snail), and 50 LowNA images (LowNA, e.g., jacket, mitten, bear). Images were digitally scanned bitmap images of black-and-white line drawings from Snodgrass and Vanderwart’s (Citation1980) standardised bank of pictures and represented nouns from a variety of semantic categories. The stimulus set had been developed and used by Cheng et al. (Citation2010). These stimuli were presented along with 8 practice items and 30 fillers. Name agreement estimates were drawn from norms used by Morrison, Chappell and Ellis (Citation1997), who defined name agreement as the percentage of their participants who used the target word to name a particular picture. Based on Morrison et al. norms, our HighNA and LowNA items were significantly different only on name agreement (HighNA M = 100%, range = 0, SD = 0; LowNA M = 76%, range = 50–87%, SD = 10.2%, p = 0.001). Cheng et al. (Citation2010) had matched the two-word sets on several lexical and images variables, namely visual complexity, picture-name agreement, objective AoA, rated AoA, frequency, familiarity, number of phonemes and number of syllables. For the current research, we further examined six other lexical variables to ensure that our HighNA and LowLA items did not differ in ways other than name agreement. We tested the two sets of words on number of phonological neighbours and number of orthographic neighbours (Marian, Bartolotti, Chabal, Shook, & White, Citation2012); concreteness (Morrison et al., Citation1997); imageability (Wilson, Citation1988); Colorado Meaningfulness (the extent to which a given word is related to other words, Toglia & Battig, Citation1978) and a corpus estimate of how many other words are found in the same context as the target, that is, an estimate of semantic neighbourhood density (Durda & Buchanan, Citation2006). None of these differences was significant. Furthermore, HighNA and LowNA images did not reliably differ from each other in terms of low-level picture attributes (i.e., proportion of black pixels, complexity of each image or visual overlap of pictures with each other; Cheng et al., Citation2010). The stimulus list, values across these 15 variables and results of comparisons between HighNA and LowNA across these variables can be found in . The authors actively invite researchers to use the stimulus and explore the data (please visit http://www.psychology.reading.ac.uk/aphasia).
Procedure and apparatus
Participants were tested individually in a quiet room either at the university clinic or in their homes. The task was picture naming and participants were instructed to name the depicted objects using single word names, as quickly and accurately as possible. Pictures were presented one-at-a-time on a laptop computer screen. On each picture-naming trial, a fixation cross was presented for 1000 ms, following which a target picture appeared with a brief auditory beep. The picture remained on the screen for 4000 ms, which was then followed by a blank screen for 1000 ms. Vocal responses were recorded from the onset of the image to the end of the trial, giving a trial length of 6 s; participants had 5 s per trial to respond. The experimenter then pressed a button to launch the next trial. During the experiment, no corrective feedback was provided for either group. For PWA, occasional nonspecific encouragement was given if participants appeared to become frustrated. Each participant was presented with one of two randomised sequences containing 8 practice trails, 100 stimuli (50 HighNA, 50 LowNA) and 30 fillers. To minimise the risk of fatigue, two breaks were scheduled in the experiment and participants were encouraged to take additional breaks as required. Sessions were recorded using high-quality digital audio-recordings. All responses were transcribed by a trained research assistant with expertise in phonetic transcription.
Scoring, error patterns and reliability
Transcribed responses for each trial were coded using the standard practice of using the first complete non-fragmented naming attempt before the 5-s deadline (Roach et al., Citation1996). The target name correctly pronounced following appearance of the picture was scored correct. When a participant was unable to name an item correctly, it was classified according to the response taxonomy developed for this study (see ). Note, this taxonomy not only coded the error responses into different categories but coded correct responses in detail (e.g., when the correct name was produced following a false start or hesitation, or when the correct name was produced with an article). The number of errors of each response type made to each item was computed. Error coding was performed by a trained research assistant and the first author performed reliability checks on 40% of the data. The point-by-point inter-rater agreement was 95%; disagreements were resolved by reviewing the scoring definitions and the transcripts.
Table 2. The taxonomy for classifying naming responses.
Statistical analysis
Practice items and fillers were removed prior to the statistical analysis. Analyses were conducted on groups of participants, and on items. In both cases, accuracy formed our initial measure. In addition, the distribution of error types was computed by participant for the two different types of stimuli (HighNA, LowNA). In common with many studies in aphasia, our PWA group represents wide range of performance on background and experimental testing. In the report of the statistical analysis, we initially present group data and supplement this with individual participant data for the PWA participants. Following Crawford and colleagues’ statistical method of comparing a single neuropsychological client’s performance with a group performance, we performed a Revised Standardized Difference Test (RSDT, Crawford & Garthwaite, Citation2002; Crawford, Garthwaite, & Porter, Citation2010) for every PWA. The RSDT establishes whether the difference between an individual’s scores on two conditions/tasks (X and Y, in this case HighNA and LowNA) is significantly different from the differences observed in a control sample. A significant difference between an individual PWA’s performance and the control would imply that that specific PWA is not showing the difference in performance between HighNA and LowNA, that is exhibited by the HA. This analysis allowed us to report if every PWA performed similarly to the group patterns or whether there were significant individual differences amongst them.
Naming accuracy
The dependent variable was the mean percentage of items correct in the naming task. Separate repeated measure ANOVAs were carried out on the accuracy rates using either the means per subject or means per item as dependent variables, yielding F1 and F2 statistics, respectively. In the subject analysis (F1), Group (PWA, HA) was a between-subject factor, and Type (HighNA, LowNA) was a within-subject factor. In the item analysis (F2), Type (HighNA, LowNA) was a between-subject factor, and Group (PWA, HA) was a within-subject factor. Post-hoc analyses were performed for a significant interaction in the subject analysis, using the derived “Name Agreement Effect” – that is, the difference between high and LowNA items.
Error pattern
The proportion of errors made by each participant across the five error types – Hesitations, Alternate names, Semantic, Visual and Omissions – was calculated separately for HighNA and LowNA items. These are presented in . We analysed these data in three ways: (1) Because we have specific a-priori reason to think that PWA may produce fewer alternate names than the HA group, we directly compared the proportion of these error type between groups. (2) In a more general analysis, we compared error profiles for HighNA versus LowNA for each group (i.e., separately for HA and PWA). (3) We compared error profiles for HA versus PWA for each stimulus type (i.e., separately for HighNA and LowNA). In all cases, we used nonparametric methods. For these exploratory analyses (2 and 3 above), we applied a correction to the alpha-level criterion to adjust for Type I error due to multiple comparisons. Given the explorative nature of this study, we present exact p-values for all the comparisons.
Table 3. Mean and standard deviations for the naming accuracy for high and low name agreement items (HighNA and LowNA) for people with aphasia (PWA) and healthy adults (HA) averaged across participants, as well as individual participant data for each PWA.
Results
presents the mean and standard deviations for the naming accuracy for HighNA and LowNA, and the name agreement effect (i.e., magnitude of the difference in accuracy between HighNA and LowNA) for the PWA and HA averaged across participants, as well as individual participant data from the PWA along with the results of the RSDT. also presents the data for error pattern for each of the PWA participants and the group averages. shows the naming accuracy performance of the two groups. Results of the statistical analysis for error profile are presented in .
Table 4. Comparisons of error types across High versus Low Name Agreement (HighNA vs. LowNA) for people with aphasia (PWA) and Healthy Adults (HA).
Figure 1. Mean (% correct) naming accuracy on high vs. low name agreement items (HighNA and LowNA) for healthy adults and people with aphasia. Error bars represent standard error of the mean.
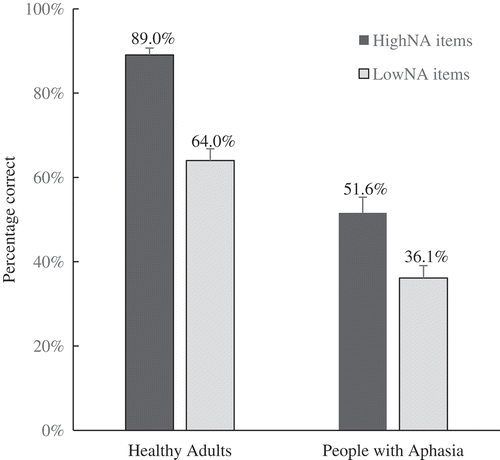
Naming accuracy
Overall, PWA produced fewer correct words than HA (PWA mean accuracy = 43.8%, SD = 19%; HA mean accuracy = 76.5%, SD = 11%). The HighNA condition resulted in a greater number of accurate responses (HighNA mean accuracy = 77%, SD = 23%; LowNA mean accuracy = 55%, SD = 19%); both PWA and HA showed a name agreement effect albeit to a different degree (see and ). The subject analysis (F1) showed a significant main effect of Group [F(1, 29) = 40.6, p < 0.001, ], Type [F(1, 29) = 138.3, p < 0.001,
] and a significant Group X Type interaction [F(1, 29) = 7.5, p = 0.01,
]. The interaction was investigated using a Mann–Whitney independent samples test on the magnitude of the naming agreement effect (HighNA–LowNA) for each group. This comparison showed a significant difference [U (21, 10) = 49.5, p = 0.019], which can be seen in : In terms of accuracy, the PWA group was much less able than the HA group to take advantage of HighNA items. Individual PWA analysis based on the RSDT showed that three PWA (i.e., PWA8, PWA9, PWA10) did not show the group pattern of better naming for HighNA than LowNA; instead showing equivalent performance for HighNA and LowNA. We looked for systematic differences between these two emergent aphasia subgroups (i.e., PWA who showed the name agreement effect and PWA who did not). However, aphasia types, PNT naming severity and/or performance on the semantic or phonological tasks did not reveal any robust differences between these two groups. The item analysis (F2) confirmed the results of the subject analysis. There were significant main effects of Type (HighNA vs. LowNA) [F(1, 98) = 32.8,
] and Group [F(1, 98) = 287,
], and a significant Type X Group interaction [F(1, 98) = 5.98, p = 0.016].
Error pattern
We calculated error distributions by dividing the number of each error type made by each participant for each image set (HighNA or LowNA), by the total number of errors made by the participant to that image set (see ). First, we used a Mann–Whitney test to examine whether HA were more likely to produce a larger proportion of Alternate names than PWA. This was strongly the case, both for HighNA and LowNA items [U(12,3) = 0, and U(21, 8) = 4, respectively, p = 0.004 and <0.0001] (see ). Second, we compared all other error types for HighNA versus LowNA items, for each group separately (upper half of ). Applying a Bonferroni correction for eight simultaneous comparisons, the critical value of alpha becomes 0.006, and the only robust difference between HighNA and LowNA items is for Alternate names, in the HA group, where (unsurprisingly, but in confirmation of the method), reliably more Alternate names are seen in the LowNA than the HighNA condition (43% vs. 25%). A somewhat different and more interesting picture emerged in our third analysis, in which we compared error profiles for PWA vs. HA separately for HighNA and LowNA (lower half of ). HA and PWA showed robust differences in the number of errors generated in the form of Alternate names, in which the proportion of Alternate name errors were much lower for PWA (see ). Furthermore, Visual and Omission error proportions discriminated between HA and PWA, but only for LowNA: PWA made relatively fewer Visual (7% vs. 27%), and more Omissions errors (32% vs. 8%), than the HA (see ).
Discussion
We set out to examine the influence of images’ name agreement – high versus low – on picture naming accuracy and error profile in the context of aphasia. We had several motivations. First, we simply wished to establish if name agreement effects are observable in PWA, and if so, if they are in any way distinct from such effects in HAs. Second, we wanted to test if PWA would have particular difficulty when generating the alternative names which are a natural outcome of trying to name a LowNA image. Third, we wished to describe the patterns of errors observed in HighNA and LowNA contexts both aphasia and HAs.
Do we observe name agreement effects in aphasia and HAs?
We considered it important to control our stimuli carefully. Our two word sets (HighNA vs. LowNA) did not differ statistically on 15 relevant lexical or image variables, including measures of neighbourhood densities.
Name agreement effects were clearly observable, producing strong main effects in our ANOVAs, and there was an unsurprisingly higher naming accuracy for HighNA items in both groups (see ). This corroborates the literature on name agreement effects on reaction time data from HAs (e.g., Alario et al., Citation2004; Bonin et al., Citation2003; Ellis & Morrison, Citation1998; Vitkovitch & Tyrrell, Citation1995) and accuracy data from neuropsychological populations (e.g., Laiacona et al., Citation2001; Rodríguez-Ferreiro et al., Citation2009). The possible mechanism for the name agreement effect in object naming can be explained in the context of the semantic and lexical levels of processing within word production models (Dell, Schwartz, Martin, Saffran, & Gagnon, Citation1997; Indefrey, Citation2011). During picture naming, a semantic cohort is automatically activated, and a selection process chooses among competing alternatives. Specifically, picture stimuli automatically trigger activation of nodes at the semantic level which flows to the lexical level, where specific nodes corresponding to individual lemmas are activated; eventually one of these activated nodes is selected. Accordingly, while activation at the semantic level will be approximately equivalent in the HighNA and LowNA conditions, at the lexical level, semantic activation will be experienced by more lemmas in the LowNA condition than in the HighNA condition (because there are more words that correspond to the given semantic concept). Therefore, selection demands are higher when multiple names apply to a single picture than when a single name applies reliably. For example, consider naming book compared to naming couch. Most likely, the picture of a book will evoke a single reliable response (i.e., HighNA). In contrast, names such as settee, sofa and couch may all come to mind for the picture of a couch (i.e., LowNA). Thus, when a person names a picture, demands for selection are lower when naming a picture of a book than when naming a picture of a couch. This competitive situation implies a controlled selection at the lexical level for LowNA items to deliver the best lemma (Cameron-Jones & Wilshire, Citation2007; Ellis & Morrison, Citation1998; Kan & Thompson-Schill, Citation2004; Vitkovitch & Tyrrell, Citation1995). However, if the target lemma does not exceed the critical threshold required for selection, an error will result. Our error analysis provides insight into how this competition may occur in PWA.
As expected, a main effect of Group was observed with HA performing significantly better than PWA. Further, there was an interaction, of medium–large effect size (0.46), for participant group and stimuli type. Specifically, the reduction in accuracy between HighNA and LowNA pictures was lower for the PWA group (15.6%) than for the HA group (25%). Prima facie, then, the name agreement of an image has a particularly strong effect on accuracy of picture naming in HA, and less of an effect in PWA. How might aphasia reduce sensitivity to name agreement effects? We return to this issue in our analysis of error patterns (see below), which we believe may shed light on this question.
Individual analysis of our PWA showed several interesting patterns: First, as expected PWA showed high variability in performance, with the magnitude of the name agreement effect ranging from 0% to 30%. This suggests that some participants were more sensitive than others to our name agreement manipulation. Second, comparison of the name agreement effect for individual PWA participants with the HA group data using RSDT revealed that three of our PWA (i.e., PWA8, PWA9, PWA10) did not show the group pattern of higher accuracies for HighNA. Although all PWA found naming LowNA difficult, those who could name HighNA better showed a name agreement effect of comparable effect to that in the healthy controls. Somewhat paradoxically, and with the caveat that our numbers are small, it appears that in our sample what really distinguishes PWA from HA is the difference in their ability to capitalise on the benefits of HighNA images. This in turn suggests that perhaps aphasia results in high levels of lexical competition. This is of course a preliminary conclusion but one that might be further tested in future.
Previous studies that have investigated name agreement in stroke or progressive aphasia have reported similar results to ours, that is, an overall performance of higher accuracy for images with HighNA, with considerable individual variation (Cameron-Jones & Wilshire, Citation2007; Kremin et al., Citation2001; Laiacona et al., Citation2001). For example, Laiacona et al.’s study of 49 PWA found name agreement to be the best predictor of naming accuracy for the largest proportion of their participants (27/49, 55%); and Kermin et al. (Citation2001) reported a significant name agreement effect in 5 of their 8 participants with Primary Progressive Aphasia. Similarly, Cameron-Jones and Wilshire (Citation2007) found their four participants with aphasia performed better for HighNA images than for LowNA images, with a mean difference of 11% between the two conditions; however, in only one participant (DBU with Broca’s aphasia) was this difference statistically significant. In the present study, we found a mean difference of 15.6% between the HighNA and LowNA for our PWA, which is comparable to the magnitude difference noted by Cameron-Jones and Wilshire (Citation2007). None of the three previously mentioned studies included a control group of participants. This restricts our ability to comment on whether PWA in these studies appeared to show higher or lower sensitivity to name agreement manipulation than controls.
Accuracy data from HA controls often suffer from ceiling effects. However, our HA made sufficient errors to permit a statistical analysis on their accuracy. The spread of performance in our HA data is probably a combination of use of a carefully controlled stimulus set sensitive enough to capture name agreement effects, and/or use of a detailed response coding protocol which allowed coding of word-finding difficulties. Our protocol captured hesitations, whilst including responses which were produced quickly and accurately as correct. Our results suggest that our image set is effective for the manipulation of name agreement, and further that this variable is a robust image property which results in differential performance even in accuracy in HA.
What does error pattern difference between the two groups reveal about the word production?
The types of errors made by the two groups are distinctive (see ). Our a-priori prediction was that PWA would generate fewer Alternate names than HA did; it was confirmed. PWA generated fewer alternate names for the stimuli than did the HA participants, for all items, but particularly for the LowNA ones (see for raw means, for statistical analysis). This finding is in agreement with the literature: errors made by unimpaired participants frequently involve stimuli with close semantic links to the target and/or are in the target’s semantic neighbourhood (Kemmerer & Tranel, Citation2000; Vitkovitch & Tyrrell, Citation1995). As expected, PWA were particularly challenged when there were competing candidate names for an image. This result is consistent with other reports (Cameron-Jones & Wilshire, Citation2007; Scott & Wilshire, Citation2010).
Further, exploratory analyses suggest some other robust findings (inasmuch as they survived alpha correction for eight comparisons). PWA and HA subjects were different and distinct in their generation of errors, but only to the LowNA items. Specifically, PWA showed a higher number of Omissions than did HA, for LowNA. This result supports the idea that heightened competition in LowNA increases the chance of word retrieval failures. It seems plausible that LowNA images induce a highly competitive situation such that all potential competitors inhibit one another and response fails completely. Similar arguments have been put forward to explain possible reason for omission errors in naming in high-competition conditions (e.g., Dell, Lawler, Harris, & Gordon, Citation2004; Robinson, Blair, & Cipolloti, Citation1998; Schnur, Schwartz, Brecher, & Hodgson, Citation2006; Scott & Wilshire, Citation2010; Spalek, Damian, & Bölte, Citation2013).
Conclusions, limitations and future directions
Images and pictures are essential materials for assessment and rehabilitation in aphasia. Reports propose that name agreement (the degree to which different people agree with a particular name for an object) is a strong and robust predictor of picture naming in healthy individuals in a wide variety of languages. We examined the influence of images’ name agreement – high versus low – on picture naming accuracy and error profile in context of aphasia. Name agreement, as a lexical variable, produced measurable differences in naming accuracy for both HAs and PWA. PWA were distinguished from HAs by showing a reduced effect of name agreement, and by exhibiting a quantitatively different pattern of errors. We propose that lower name agreement induces a high degree of competitive lexical selection during naming, and that PWA find this competition particularly difficult to resolve.
Future research should address several of the limitations of the current study. Limited sample size and heterogeneity of aphasia is a perennial problem in any aphasia research. We attempt to circumvent these issues by using a large number (100) of items, and by providing group and individual level analyses. Future research should preferably replicate our findings in a larger and varied aphasia population, potentially linking lesion site to behaviour. For example, it has been suggested that lexical selection competition is more prominent in nonfluent than fluent aphasia (Cameron-Jones & Wilshire, Citation2007), possibly due to damage in left inferior frontal gyrus.
Our analysis showed name agreement to be a reliable experimental variable not just in HAs (as reported in previous literature), but also in aphasia, at least for the group we studied. The distinct error pattern in the LowNA condition between healthy controls and aphasia provides us opportunity to investigate the possible mechanism for this difference. We speculate that heightened lexical competition could be a source of this finding; investigation into detailed executive control processes that underpin lexical selection in healthy and impaired population would be a productive avenue for research. Another point of theoretical interest would be to explore how different types of name disagreement affect impaired populations. There is more than one source of name disagreements between images, and these are associated with differing loci of origin within the lexical system for HAs (Cheng et al., Citation2010; O’Sullivan et al., Citation2012; Vitkovitch & Tyrrell, Citation1995); currently, experimental data are lacking for impaired populations.
Our findings further underscore the need for researchers and clinicians to be mindful in preparation of their assessment and therapy materials (e.g., Fieder et al., Citation2014). Failure to do so may have important consequences in studies involving naming. Further exploration of the name agreement variable in response to (re)learning of words in therapeutic context would have important implications. Despite the limitations of the current study, we believe the present study provides useful data and motivates several lines of future research with theoretical and clinical implications.
Acknowledgements
We are indebted to all our participants for their enthusiasm and time for participation in this research. We thank Emma Thomas, Natasha Wilkinson and Anne-Marie Greenway for assistance in data collection. We acknowledge the support of British Academy Small Grant Scheme (SG120324), which allowed funding for collection of data on the background tests.
Disclosure statement
No potential conflict of interest was reported by the authors.
Additional information
Funding
References
- Alario, F. X., Ferrand, L., Laganaro, M., New, B., Frauenfelder, U. H., & Segui, J. (2004). Predictors of picture naming speed. Behavior Research Methods, Instruments, & Computers, 36, 140–155. doi:10.3758/BF03195559
- Bakhtiar, M., Nilipour, R., & Weekes, B. S. (2013). Predictors of timed picture naming in Persian. Behavior Research Methods, 45, 834–841. doi:10.3758/s13428-012-0298-6
- Barry, C., Morrison, C. M., & Ellis, A. W. (1997). Naming the Snodgrass and Vanderwart pictures: Effects of age of acquisition, frequency, and name agreement. The Quarterly Journal of Experimental Psychology Section A, 50, 560–585. doi:10.1080/783663595
- Bonin, P., Peereman, R., Malardier, N., Méot, A., & Chalard, M. (2003). A new set of 299 pictures for psycholinguistic studies: French norms for name agreement, image agreement, conceptual familiarity, visual complexity, image variability, age of acquisition, and naming latencies. Behavior Research Methods, Instruments, & Computers, 35, 158–167. doi:10.3758/BF03195507
- Bormann, T., Kulke, F., Wallesch, C.-W., & Blanken, G. (2008). Omissions and semantic errors in aphasic naming: Is there a link? Brain & Language, 104, 24–32. doi:10.1016/j.bandl.2007.02.004
- Cameron-Jones, C. M., & Wilshire, C. E. (2007). Lexical competition effects in two cases of non-fluent aphasia. Brain & Language, 103, 136–137. doi:10.1016/j.bandl.2007.07.083
- Cheng, X., Schafer, G., & Akyürek, E. G. (2010). Name agreement in picture naming: An ERP study. International Journal of Psychophysiology, 76, 130–141. doi:10.1016/j.ijpsycho.2010.03.003
- Coltheart, M. (2001). Assumptions and methods in cognitive neuropsychology. In B. Rapp (Ed.), The handbook of cognitive neuropsychology: What deficits reveal about the human mind. Philadelphia, PA: Psychology Press.
- Crawford, J. R., & Garthwaite, P. H. (2002). Investigation of the single case in neuropsychology: Confidence limits on the abnormality of test scores and test score differences. Neuropsychologia, 40, 1196–1208. doi:10.1016/S0028-3932(01)00224-X
- Crawford, J. R., Garthwaite, P. H., & Porter, S. (2010). Point and interval estimates of effect sizes for the case-controls design in neuropsychology: Rationale, methods, implementations, and proposed reporting standards. Cognitive Neuropsychology, 27, 245–260. doi:10.1080/02643294.2010.513967
- Cuetos, F., Ellis, A. W., & Alvarez, B. (1999). Naming times for the Snodgrass and Vanderwart pictures in Spanish. Behavior Research Methods, Instruments, & Computers, 31, 650–658. doi:10.3758/BF03200741
- Dell, G., Schwartz, M., Martin, N., Saffran, E., & Gagnon, D. (1997). Lexical access in aphasic and nonaphasic speakers. Psychological Review, 104, 801–838. doi:10.1037/0033-295X.104.4.801
- Dell, G. S., Lawler, E. N., Harris, H. D., & Gordon, J. K. (2004). Models of errors of omission in aphasic naming. Cognitive Neuropsychology, 21, 125–145. doi:10.1080/02643290342000320
- Dimitropoulou, M., Duñabeitia, J. A., Blitsas, P., & Carreiras, M. (2009). A standardized set of 260 pictures for Modern Greek: Norms for name agreement, age of acquisition, and visual complexity. Behavior Research Methods, 41, 584–589. doi:10.3758/BRM.41.2.584
- Durda, K., & Buchanan, L. (2006). WordMine2 [Online]. Retrieved from http://web2.uwindsor.ca/wordmine
- Ellis, A. W., & Morrison, C. M. (1998). Real age-of-acquisition effects in lexical retrieval. Journal of Experimental Psychology: Learning, Memory, & Cognition, 24, 515–523.
- Fieder, N., Nickels, L., Biedermann, B., & Best, W. (2014). From “some butter”to “a butter”: An investigation of mass and count representation and processing. Cognitive Neuropsychology, 31, 313–349. doi:10.1080/02643294.2014.903914
- Goodglass, H., Kaplan, E., & Barresi, B. (2001). Boston diagnostic aphasia examination (3rd ed.). Baltimore, MD: Lippincott, Williams & Wilkins.
- Harley, T. A., & Grant, F. (2004). The role of functional and perceptual attributes: Evidence from picture naming in dementia. Brain & Language, 91, 223–234. doi:10.1016/j.bandl.2004.02.008
- Howard, D., & Patterson, K. (1992). Pyramids and palm trees test. Bury. St Edmunds: Thames Valley Test.
- Indefrey, P. (2011). The spatial and temporal signatures of word production components: A critical update. Frontiers in Psychology, 2, 1–16. doi:10.3389/fpsyg.2011.00255
- Johnson, C. J. (1992). Cognitive components of naming in children: Effects of referential uncertainty and stimulus realism. Journal of Experimental Child Psychology, 53, 24–44. doi:10.1016/S0022-0965(05)80003-7
- Johnson, C. J., Paivio, A., & Clark, J. M. (1996). Cognitive components of picture naming. Psychological Bulletin, 120, 113–139. doi:10.1037/0033-2909.120.1.113
- Kan, I. P., & Thompson-Schill, S. L. (2004). Effect of name agreement on prefrontal activity during overt and covert picture naming. Cognitive, Affective, & Behavioral Neuroscience, 4, 43–57. doi:10.3758/CABN.4.1.43
- Kay, J., Lesser, R., & Coltheart, M. (1992). Psycholinguistic assessments of language processing in aphasia. London: Psychology Press.
- Kemmerer, D., & Tranel, D. (2000). Verb retrieval in brain-damaged subjects: 1. Analysis of stimulus, lexical, and conceptual factors. Brain & Language, 73, 347–392. doi:10.1006/brln.2000.2311
- Kremin, H., Perrier, D., De Wilde, M. D., Dordain, M., Le Bayon, A. L., Gatignol, P., … Arabia, C. (2001). Factors predicting success in picture naming in Alzheimer’s disease and primary progressive aphasia. Brain & Cognition, 46, 180–183. doi:10.1006/brcg.2000.1270
- Laiacona, M., Luzzatti, C., Zonca, G., Guarnaschelli, C., & Capitani, E. (2001). Lexical and semantic factors influencing picture naming in aphasia. Brain & Cognition, 46, 184–187. doi:10.1016/S0278-2626(01)80061-0
- Marian, V., Bartolotti, J., Chabal, S., Shook, A., & White, S. A. (2012). CLEARPOND: Cross-linguistic easy-access resource for phonological and orthographic neighborhood densities. PLoS ONE, 7, e43230. doi:10.1371/journal.pone.0043230
- Morrison, C. M., Chappell, T. D., & Ellis, A. W. (1997). Age of acquisition norms for a large set of object names and their relation to adult estimates and other variables. The Quarterly Journal of Experimental Psychology Section A, 50, 528–559. doi:10.1080/027249897392017
- Nishimoto, T., Ueda, T., Miyawaki, K., Une, Y., & Takahashi, M. (2012). The role of imagery-related properties in picture naming: A newly standardized set of 360 pictures for Japanese. Behavior Research Methods, 44, 934–945. doi:10.3758/s13428-011-0176-7
- O’Sullivan, M., Lepage, M., Bouras, M., Montreuil, T., Brodeur, M. B., & Paterson, K. (2012). North-American norms for name disagreement: Pictorial stimuli naming discrepancies. Plos One, 7, e47802. doi:10.1371/journal.pone.0047802
- Pind, J., & Tryggvadottir, H. B. (2002). Determinants of picture naming times in Icelandic. Scandinavian Journal of Psychology, 43, 221–226. doi:10.1111/sjop.2002.43.issue-3
- Roach, A., Schwartz, M. F., Martin, N., Grewal, R. S., & Brecher, A. (1996). The Philadelphia naming test: Scoring and rationale. Clinical Aphasiology, 24, 121–134.
- Robinson, G., Blair, J., & Cipolotti, L. (1998). Dynamic aphasia: An inability to select between competing verbal responses? Brain, 121, 77–89. doi:10.1093/brain/121.1.77
- Rodríguez-Ferreiro, J., Davies, R., González-Nosti, M., Barbón, A., & Cuetos, F. (2009). Name agreement, frequency and age of acquisition, but not grammatical class, affect object and action naming in Spanish speaking participants with Alzheimer’s disease. Journal of Neurolinguistics, 22, 37–54. doi:10.1016/j.jneuroling.2008.05.003
- Saffran, E. M., Schwartz, M. F., Linebarger, M. C., Martin, N., & Bochetto, P. (1987). The Philadelphia comprehension battery. Unpublished test battery. Philadelphia, PA.
- Schnur, T. T., Schwartz, M. F., Brecher, A., & Hodgson, C. (2006). Semantic interference during blocked-cyclic naming: Evidence from aphasia. Journal of Memory and Language, 54, 199–227. doi:10.1016/j.jml.2005.10.002
- Scott, R., & Wilshire, C. (2010). Lexical competition for production in a case of nonfluent aphasia: Converging evidence from four different tasks. Cognitive Neuropsychology, 27, 505–538. doi:10.1080/02643294.2011.598853
- Shao, Z., Roelofs, A., & Meyer, A. S. (2014). Predicting naming latencies for action pictures: Dutch norms. Behavior Research Methods, 46, 274–283. doi:10.3758/s13428-013-0358-6
- Snodgrass, J. G., & Vanderwart, M. (1980). A standardized set of 260 pictures: Norms for name agreement, image agreement, familiarity, and visual complexity. Journal of Experimental Psychology: Human Learning and Memory, 6, 174–215.
- Spalek, K., Damian, M. F., & Bölte, J. (2013). Is lexical selection in spoken word production competitive? Introduction to the special issue on lexical competition in language production. Language and Cognitive Processes, 28, 597–614. doi:10.1080/01690965.2012.718088
- Toglia, M. P., & Battig, W. F. (1978). Handbook of semantic word norms. Hillsdale, NJ: Lawrence Erlbaum Associates.
- Vitkovitch, M., & Tyrrell, L. (1995). Sources of disagreement in object naming. The Quarterly Journal of Experimental Psychology Section A, 48, 822–848. doi:10.1080/14640749508401419
- Wilson, M. D. (1988). MRC psycholinguistic database: machine-usable dictionary, version 2.00. Behavior Research Methods, Instruments, & Computers, 20, 6–10. doi:10.3758/BF03202594
Appendix
The section provides the stimulus list of the High Name Agreement (HighNA) and Low Name Agreement (LowNA) words used in this study. We provide the values of lexical and image variables that were controlled for the two sets of words. Cheng et al. (Citation2010) had controlled for eight variables, namely visual complexity, picture-name agreement, two estimates of Age-of-Acquisition (AoA) (objective AoA and rated AoA), frequency, familiarity, number of phonemes, and number of syllables. Values for these eight variables, along with name agreement values, were drawn from Morrison et al. (Citation1997). For this present paper, we further checked for imageability, concreteness, Colorado meaningfulness, an estimate of semantic neighbours, number of phonological and number of orthographic neighbours. The values for imageability ratings were drawn from Morrison et al. (Citation1997); concreteness ratings and Colorado meaningfulness norms from MRC Psycholinguistic Database (Wilson, Citation1988); semantic neighbours from Wordmine2 (Durda & Buchanan, Citation2006); number of phonological and orthographic neighbours from CLEARPOND (Marian et al., Citation2012).