ABSTRACT
Models live in a state of exception. Their versatility, the variety of their methods, the impossibility of their falsification and their epistemic authority allow mathematical models to escape, better than other cases of quantification, the lenses of sociology and other humanistic disciplines. This endows models with a pretence of neutrality that perpetuates the asymmetry between developers and users. Models are thus both underexplored and overinterpreted. While retaining a firm grip on policy, they reinforce the entrenched culture of transforming political issues into technical ones, possibly decreasing citizens’ agency and thus favouring anti-democratic policies. To combat this state of exception, one should question the reproducibility of models, foster complexity of interpretation rather than complexity of construction, and encourage forms of activism aimed at achieving a reciprocal domestication between models and society. To breach the solitude of modellers, more actors should engage in practices such as assumption hunting, modelling of the modelling process, and sensitivity analysis and auditing.
1. Introduction: why models live in a state of exception
The thesis of the present work is that mathematical models enjoy a state of exception that results from their versatility and epistemic authority, coupled with the infinite spectrum of methods available to mathematicians and the difficulty of proving a model wrong. The state of exception also results from the pretence of neutrality customarily associated with mathematics, and from the asymmetry between developers and users. Models have a considerable influence on policy, and this often means transforming political issues into technical ones. Models used for the analysis of risk are a typical instance of this process of displacement, a situation well noted by ecologists: a new technology is introduced, and models are deployed to show that it is safe for humans and their environment, thereby displacing the attention from the reasons – and desirability – of introducing the technology in the first place (Marris Citation2001; Winner Citation1989).
At times this may lead to surreal predictions, like trying to foresee the risk of leakage from an underground nuclear waste repository million years ahead (Pilkey and Pilkey-Jarvis Citation2009b)), a profusion of digits such as that generated by mathematical models during the last pandemic (Saltelli et al. Citation2020a), or the ‘Funny Numbers’ produced by econometricians to assess the risk of financial instruments (Porter Citation2012). Such errors are not always innocent of consequences. The ‘Funny Numbers’ of speculation on the American mortgages market created the crash of 2008/9 (Ravetz Citation2008). The rescue operation of ‘quantitative easing’ (zero or negative interest rates) had destabilising consequences that are still with us. And the models of a population explosion in China, retained long after they became inappropriate, led to restrictive policies that now make a catastrophic population implosion all too likely (Qi Citation2024).
To correct this state of exception, one should question the reproducibility of models, foster complexity of interpretation rather than the complexity of construction, and encourage forms of activism following the French statactivists, aimed at achieving a reciprocal domestication between models and society (Bruno, Didier, and Prévieux Citation2014). To breach the isolation of modellers, more actors should engage in practices such as assumption hunting, modelling of the modelling process, and sensitivity analysis and auditing.
We will start this analysis by illustrating our claim that models are special compared to other families of quantification. This is based on their exceptional epistemic authority, and on the fact that the sociology of quantification is apparently less active when it comes to these elements of the family of quantification.
1.1. Unparalleled palette of methods/invisible models
Models dispose of a unique repertoire of methods. This statement hardly needs justification. In brief, this view stems from mathematics, the highest ranked among scientific disciplines (Davies and Hersh Citation1986), considered by the fathers of the scientific revolution the language of God himself. This perspective still endures to this day, and the ongoing effort to integrate mathematics with human experience remains an unfinished project (Lakoff and Núñez Citation2001). The persistence of this faith has been explained by T.M. Porter (Citation1995) with the role of numbers and mathematics as the source of legitimacy and trust in an increasingly impersonal society.
Models operate without established standards and disciplinary oversight. Until very recently, these features of scientific practice were not recognised in the philosophy of science, and so their absence did not signal a serious problem in the scientific status of a field. The fragmentation of mathematical modelling has resulted in a lack of agreed standards. A book on modelling read by all disciplines would enhance the communication of good practices among different fields. Unfortunately, such a book does not exist. A useful work is that of Foster Morrison (Citation1991), though it is silent about verification and validation procedures. These topics are instead well treated in Santner, Williams, and Notz (Citation2003), and in Oberkampf and Roy (Citation2010). The work of Robert Rosen (Citation1991) contains deep insights from system biology, including discussions on causality and ‘modelling as a craft’. An interesting text on the philosophy of models is offered by Morgan and Morrison (Citation1999). A recent book by Scott Page (Citation2018) draws a broad canvas of different modelling types and strategies, while Saltelli and Di Fiore (Citation2023) tackle the politics of modelling. Still, the problem is that none of these works, regardless of their merit, can command the necessary epistemic authority in the absence of a disciplinary basis, both methodological and social, for modelling.
Padilla et al. (Citation2018), after conducting a survey involving 283 responding modellers, noted that the heterogeneous nature of the modelling and simulation community prevents the emergence of consolidated paradigms, leading to validation and verification procedures being more of a trial and error business. These authors advocate a ‘more structured, generalized and standardized approach to verification’. After reviewing several existing checklists and approaches for model quality, they note that poorly informed users risk remaining ‘unaware of limitations, uncertainties, omissions and subjective choices in models’, with consequent over-reliance on the quality of model-based inferences. Model developers do not fare much better; they may over-elaborate their findings, concealing the uncertainties in their results and recommendations. Jakeman et al. (Citation2006) propose a 10-point checklist for model development. Noticeably, the list starts with the definition of the model’s purpose and context, the conceptualization of the task, the choice of the family of models and uncertainty specification before moving to the nitty-gritty issues of estimation, calibration and verification. Eker et al. (Citation2018) observe the existence of different cultures in model validation – one empirical and data driven, and another qualitative and participatory – which they associate with ‘positivistic’ versus ‘relativistic’ positions. These authors register the prevalence of the former view while acknowledging the usefulness of the latter, especially at the stage of identifying scenarios.
1.2. Mathematical models escape the sociology of quantification
Statistics has a much deeper connection to sociology, and to sociology of quantification in particular (Desrosières Citation1998; Mennicken and Nelson Espeland Citation2019; Mennicken and Salais Citation2022), than mathematical modelling. The sociology of quantification is more concerned with algorithmic governance, metrics, statistical indicators, the quantified self, and governing by numbers, although impact assessment tools such as cost benefit analyses – models of a sort – are a classic theme in this discipline (Porter Citation1995). An exception is the excellent work on models by Morgan and Morrison (Citation1999), which we will discuss below in relation to ‘models as mediators’.
1.2.1. The epistemic authority of models
The greater epistemic authority of mathematical modelling derives from the widespread assumption that models map onto the underlying state of affairs and therefore decode the mathematical structure of the process of interest. Modellers are regarded as endowed with privileged access to the foundations of reality. Additionally, modellers make use of state-of-the-art computing facilities and thus are at the forefront of technology and science, and this enables them to gain epistemic authority by working on timely and pressing societal issues. Their recommendations are often taken as guidance for policy-making. The already mentioned epistemic authority accruing from the use of mathematics provides models with a better pretence to neutrality than other families of quantification.
1.2.2. Models cannot be falsified
Models do not meet classic (Popperian) criteria of scientificity. Oreskes (Citation2010) has observed that model-based predictions tend to be treated like logical inferences in a classic hypothetic-deductive model. According to this perspective, laws are tested by making predictions which are then verified by experiments. If the prediction is not confirmed, then the law is ‘falsified’ (proven false). For Oreskes, a major problem in our use of mathematical models lies in assimilating them to physical laws, and hence treating their predictions with the same dignity. This is wrong, because in order to be of value in theory testing, the model-based predictions ‘must be capable of refuting the theory that generated them’. Since models are a ‘complex amalgam of theoretical and phenomenological laws (and the governing equations and algorithms that represent them), input parameters and concepts, then when a model prediction fails what part of all this construct was falsified? The underlying theory? The calibration data? The system’s formalization or choice of boundaries? The algorithms used in the model?’
Oreskes’ points are known in philosophy as the Duhem-Quine critique (Duhem Citation1991; Quine Citation1951). To the extent that scientific theories themselves are ‘models’, i.e. amalgams of theoretical assumptions, holistic preconceptions, measurement devices, and physical equations, when a prediction is contradicted by data, it needs to be reformulated, but it isn’t always clear how. We cannot even be sure that the problem lies with the model itself, because no element in a theory or model can be tested in isolation. Thus, we may need to revise other parts of the scientific edifice upon which our model rests in order to accommodate our results.
A related problem is that of contrastive underdetermination: even if a model output matches the data,Footnote1 it does not follow that the model is truth-conducive: no finite amount of data can narrow down the range of possible models to just a single function or set of functions. The classic example of oranges and apples (If I have spent $10 in apples and oranges, and each apple costs $2 and each orange cost $1, how many oranges and apples have I bought?) illustrates the problem (Stanford Citation2023). Under these circumstances, pragmatic and/or non-rational criteria may step in to do the heavy lifting of picking up the ‘best’ model. These factors would include, for example, the charisma and influence of the developer, the availability of resources, adherence to the prevailing scientific paradigm, or the simplicity or parsimony of the model (Feyerabend Citation1988).
1.2.3. Models as the most effective mediators between theory and reality
Models are regarded as a meaningful simplification of a real system, a device for predicting the unknown behaviour of a system from the operation of its parts, and as a formalization of our knowledge about a system (Hall and Day Citation1977). Many authors have noted that models mediate between theory and reality, due to their independence from both. Thus, models would be instruments that act and describe things in ways that advance understanding thanks to the tacit craftsmanship of scientists (Morrison and Morgan Citation1999). For Marcel Boumans (Citation1999), models act as integrators of a broad array of ingredients, including theoretical notions, mathematical concepts and techniques, stylized facts, empirical data, policy views, analogies and metaphors. According to Jerome Ravetz (Citation2023), instead, models are like metaphors that help us understand how we see the world: they express ‘in an indirect form our presuppositions about the problem and its possible solutions’, and can thus assist an extended community of peers in deliberating about social or ecological problems.
2. Consequences arising from the state of exception
What are the consequences of being special? The mentioned versatility of models is crucial because it enables them to colonize all aspects of knowledge. Coupling versatility with the other aspects of state of exception just mentioned leads to important consequences.
2.1. Gross asymmetry between developers and users
Models operate in a context of asymmetry of knowledge between developers and users, a point that is thoroughly elaborated in Jakeman, Letcher, and Norton (Citation2006). One can contest this claim by referring to ‘black boxes’ used in other families of quantification, typically algorithms or statistics. We contend that this asymmetry is especially of concern for large mathematical models (Puy et al. Citation2022).
2.2. Ritual use
Models lend themselves to ritual use. An important analogy between statistical and mathematical modelling lies in the ‘ritual’ use of methods. The existence of rituals in statistics has been discussed extensively by Gigerenzer (Citation2018) and by Gigerenzer and Marewski (Citation2014). We similarly call ‘cargo-cult’ statistics the use of statistical terms and procedures as incantations, with scant understanding of the assumptions or relevance of the calculations they are based on (Stark and Saltelli Citation2018). As for rituals in modelling, the best anecdote is perhaps that offered by Kenneth Arrow. During the Second World War, he was a weather officer in the US Army Air Corps working on the production of month-ahead weather forecasts, and this is how he tells the story (Szenberg Citation1992):
The statisticians among us subjected these forecasts to verification and they differed in no way from chance. The forecasters themselves were convinced and requested that the forecasts be discontinued. The reply read approximately like this: ‘The commanding general is well aware that the forecasts are no good. However, he needs them for planning purposes’.
Social scientist Niklas Luhmann, as discussed in Moeller (Citation2006), uses the term ‘deparadoxification’ to indicate the use of scientific knowledge to give a claim a pretension of objectivity, so as to show that policy decisions are based on a publicly verifiable process, rather than on an expert’s whim: we all recall the ‘We follow the science’ declaration of politicians during the COVID-19 pandemic (Devlin and Boseley Citation2020).
2.3. Proclivity for trans-science
Models lend themselves to trans-science. Trans-science refers to scientific practices that appear to be formulated in the language of science, but that science cannot solve because of their sheer complexity or insufficient knowledge (Weinberg Citation1972).
Examples of trans-science are not difficult to find. Examples include:
How many people will sit in an autonomous cars by 2050?
How will the spread of malaria change if global temperature increases by 1.5°C?
What will be the cost of CO2 averaged over the next three centuries?
Ambitious modelling projects such as the ongoing plans to develop a digital twin of the Earth (Bauer, Stevens, and Hazeleger Citation2021) may also be seen under this light, where models aspire to become one-to-one maps of the empire as described in the short story Del Rigor en la Ciencia (On Exactitude in Science) by Jorge Luis Borges (Citation1946).
2.4. Grip on policy-making
Models have their own political economy. Like other means of quantification, models encourage strategies of economicism, solutionism, reductionism, and transformation of quality into quantity (Stirling Citation2023a, Citation2023b). These strategies have been called out in relation to the use of quantitative methods in impact assessment, as well as in contexts where New Public Management strategies are adopted (Muller Citation2018; O’Neil Citation2016; Salais Citation2022). The political economy of modelling is based on its strong epistemic authority and influence on decision-making, and the fact that models are rarely blamed for failure.
Statistical experiments can be registered, while the preregistration of modelling studies (Ioannidis Citation2022) is still a long way off. For Chalmers and Glasziou (Citation2009) the percentage of non-reproducible studies in the field of clinical medical research could reach 85%. Nobody can provide a similar figure for mathematical modelling.
Two additional policy features of models are their capacity of ‘navigating the political’ (adapting their inference to an ongoing policy process, thus becoming part of the process itself, as discussed by van Beek et al. (Citation2022), and their ability to act as chameleons (jumping from a context of investigation, i.e. ‘what if’, to one of prediction, and defending against criticism by reverting to ‘this is just a study model’, as seen in Pfleiderer (Citation2020).
The idea that a quantification should conform to both technical and normative dimensions of quality is not new; it goes back to Amartya Sen’s Informational Basis for Judgment of Justice (IBJJ) (Sen Citation1990). This principle suggests asking the question of whether a system of measurement or analysis provides a fair, informed judgement of an issue. In this framework (capability approach), fairness is related to the possibility for individuals to achieve their goal, once their individual conditions are taken into account. In this way, fairness does not equate to all having the same material means, but rather to all having an equal opportunity to fulfill their aspirations. Salais (Citation2022) resumes this concept and notes that the prevailing unfair quantification schemes originating from new public management approaches contribute to the creation of a state of a-democracy – a system where citizens are confronted with all the signs of a democratic system of governance, but none of the corresponding chances to express agency.
The ideas that regimes of numericization may be ultimately anti-democratic are not new either. This argument has been made in relation to artificial intelligence and the use of big data (McQuillan Citation2022; Supiot Citation2017; Zuboff Citation2019). The models used in operations research may also fit into this category (Muller Citation2018; O’Neil Citation2016), with extreme cases of workers exploitation and deprivation (Kantor et al. Citation2022; Teachout Citation2022).
2.5. Vulnerability to modelling hubris
The conjecture of O’Neill (Citation1971), see also Turner and Gardner (Citation2015), posits that too simple models may overlook important features of the system, and thus lead to systematic errors. Conversely, models that are too complex (burdened by an excessive number of estimated parameters) may lead to greater imprecision due to error propagation. In this second case, one might speak of modelling hubris, i.e. a situation where the pursuit of improvement leads modellers to over-reach (Saltelli et al. Citation2020a). In data science, a very similar trade-off is that between under- and over-fitting. For instance, in interpolation, one may use a polynomial whose order is too low, thus underfitting the existing or training points. Instead, if the order is too high, the existing points are fitted too well, often at the cost of performing poorly when new points are added to the set. An array of methods is available in data science to tackle this problem (James et al. Citation2017).
Other disciplines have different names for this trade-off. In system analysis, Zadeh calls this the principle of incompatibility, whereby as complexity increases ‘precision and significance (or relevance) become almost mutually exclusive characteristics’. Finding the right balance, the point of minimum error shown in , is easier in data analysis than in modelling. An old discussion of this conundrum between model parsimony and modelling hubris can be found in Hornberger and Spear (Citation1981), who note that models’ over-parametrization may be fiddled with ‘to produce virtually any desired behaviour, often with both plausible structure and parameter values’.
Figure 1. Increasing the complexity of a model reduces its systematic bias due to the omission of relevant features of the system being modelled. Since descriptive power comes at the cost of additional estimated parameters, increasing the complexity of the model also increases the error due to the propagation of parametric uncertainty to the output.
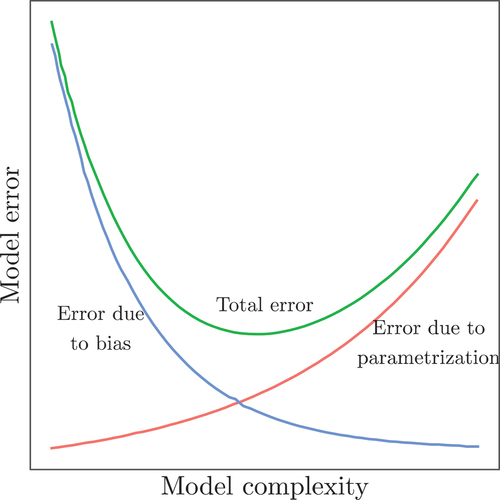
Note that in cases where models have access to validation data, there are statistical tests available for judging the parsimony of the model (Akaike Citation2011; Schwarz Citation1978). When instead the model projects into the future or operates outside the region in the input space where it was calibrated, the assessment of model bias may be challenging. To keep a proper balance between output uncertainty and complexity, modellers working in these scenarios should track the uncertainty build up at each stage of model refinement by calculating the model’s effective dimensions (i.e. the number of influential parameters and the order of the highest-order effect). This may help identify the threshold beyond which the addition of detail and its correspondent boost in uncertainty no longer makes the model fit-for-purpose (Puy et al. Citation2022).
3. Solutions to the state of exception
3.1. Thinking about the reproducibility of models
Preregistration of mathematical modelling studies is proposed by Ioannidis (Citation2022). Pre-registration, mostly advocated in relation to lab or field experiments and supported by some academic journals in the realms of psychology or human behaviour, is the practice of registering the study plan and objectives in a repository before a scientific project begins. The goal is to prevent malpractices such as HARK [Hypothesis After the Results are Known (Kerr Citation1998)], whereby different hypotheses are thrown around a set of data until one meets some test of significance. HARK violates the rules of hypothesis testing and, together with P-hacking, may be held responsible for the lack of reproducibility of scientific findings (Munafò et al. Citation2017; Saltelli and Funtowicz Citation2017). Whether pre-registration can be applied to mathematical modelling depends on many factors. For predictive models, for example, the time horizon of the prediction is important for the registration to be meaningful: a prediction concerning the performance of a nuclear waste repository over a million-year time span (Stothoff and Walter Citation2013) clearly falls beyond its scope. Ioannidis believes that preregistration may help build trust in modelling if accompanied by practices. These include specifying what constitutes a successful prediction, outlining the conditions for recalibration, agreeing on validation criteria, promoting team science whereby multiple teams and/or stakeholder participate in the analysis, see e.g. (Lane et al. Citation2011; Ravetz Citation2023), and thoroughly documenting present and past model records.
The common assumption is that the models, being mathematical, produce a unique outcome for each set of inputs. Based on the belief that computers only manipulate 0’s and 1’s, this assumption is a strong contributor to the state of exception of mathematical models. In practice, it is safest to treat each run of a model as an experiment. There is evidence that in quantitative analyses, the results can often display a wide range of outcomes depending on who performs the analysis. In Breznau et al. (Citation2022), for instance, researchers from 73 teams produced effects estimate ranging from positive to negative simply by making different modelling choices. It is also worth noting that what made this large experiment possible was the decision of the participating teams to state the objective of their analysis.
3.2. Sensitivity analysis and sensitivity auditing
But the real strength of the models, in my mind at least, were in sensitivity analysis (where one could examine the response of the model to parameters or structures that were not known with precision (i.e. sensitivity analysis), and in the examination of the behavior of the model components relative to that of the real system in question (i.e. validation). By undertaking sensitivity analysis and validation, a great deal can be learned about the real system, including what you do not know (Hall Citation2020).
For ecologist Charles A. S. Hall, not only is sensitivity analysis an important ingredient of model validation; it is one of the good things one can do with a model.
It is not in the scope of this paper to suggest that sensitivity analysis (SA) alone can lead to a thorough reformation of the field. Reforming modelling will involve both the adoption of methods in the practice of research and their incorporation into teaching syllabuses. It will also require the endorsement of hybrid quantitative/qualitative and transdisciplinary approaches – ‘Cinderella methods’, in the words of Andy Stirling (Stirling Citation2023b) – which shed light on the normative framworks, categories, value-ladenness and conditionalities of modelling. Sensitivity auditing (Saltelli et al. Citation2013), with its ‘modelling of the modelling process’ (Lo Piano et al. Citation2022, Citation2023), belongs to this class of transdisciplinary methods. If we accept sociologists’ view that every quantification needs to have both a technical and a normative justification of quality (Salais Citation2022), then we can say that sensitivity analysis and auditing fulfil these criteria (Saltelli and Puy Citation2022).
SA is especially useful to guide the modeller through the ‘garden of the forking paths’ (Gelman and Loken Citation2013), shedding light on the model output’s conditionality on the choices made during the modelling process. In an SA framework, all possible alternative modelling choices, from different boundary conditions to parametrizations and model structures, can be simultaneously activated. The modeller can therefore take both left and right at each bifurcation in the garden, like the Chinese author imagined by Borges (Citation1998), and observe how this multiplicity of options impacts the estimation in a modelling of the modelling process (Lo Piano et al. Citation2022). This may include stages of assumption hunting, where modellers or their interlocutors strive to retrace assumptions made in the construction of the model.
3.3. Following the example of Statactivism
When it comes to examining the quality of their quantifications, few communities have been as active as statisticians. The movement of French statactivists (Bruno, Didier, and Prévieux Citation2014; Bruno, Didier, and Vitale Citation2014), rooted in a strong national tradition of sociology of quantification (Bourdieu Citation1984; Desrosières Citation1998), has proven able to ‘fight a number with a number’ in domains of policy relevance such as poverty (Concialdi Citation2014) and consumer prices indices (Samuel Citation2022). One would very much like to imagine modellers taking the viewpoint of those ‘measured’ into the analysis as advocated by statactivists (Salais Citation2022), thereby making the invisible visible (Bruno, Didier, and Prévieux Citation2014), or fully internalizing the double nature – technical and normative – of the quality of a quantification (Mennicken and Salais Citation2022). Against this perspective, one should note that the statactivist approach comes more naturally in the domain of statistical indicators in socioeconomic systems. Can this be generalized to the many applications of mathematical modelling?
The success of statactivists is also due to the support of important institutional actors such as statistical offices – INSEE in the case of France. What would be the equivalent institution in the field of mathematical modelling? Regulatory authorities concerned with consumer or environmental protection? Or technology assessment offices akin to the now-defunct US institution (Chubin Citation2001)? We cannot answer these questions in the present work. When it comes to mathematical models, the distance from lay citizens is greater than in the case of socioeconomic statistics, which brings us back to the reciprocal domestication between models and society discussed in the responsible modelling manifesto (Saltelli et al. Citation2020a). Counter-modelizations similar to those presented by statactivists are not impossible – see e.g. Puy, Lo Piano, and Saltelli (Citation2020) – but at present they are more difficult to disseminate than the counter-statistics of statactivists.
3.4. Reciprocal domestication between models and society
The COVID pandemic of 2020 has dramatically increased the visibility of mathematical modelling, since models were increasingly needed when epidemiological evidence was scarce. Some models were praised for making a convincing case for intervention (Landler and Castle Citation2020). However, this was accompanied by considerable controversy, either due to the deficiencies of the model, or because of disagreement about the policies they informed (Pielke Citation2020; Rhodes and Lancaster Citation2020). See also Saltelli et al. (Citation2020a, Citation2020b). Interestingly, not only has mathematical modelling jargon entered the common language with expression such as ‘flattening the curve’, but politicians can now be heard discussing mathematical modelling with a notable level of detail (Seely Citation2022). One can only hope that this trend continues without leading to generalized scepticism toward the use of models for policy decision-making (Saltelli et al. Citation2023).
3.5. Defogging the mathematics of uncertainty
An important issue in mathematical modelling is the management of uncertainty. Uncertainty quantification should be at the heart of the scientific method, a fortiori when science is used for policymaking (Funtowicz and Ravetz Citation1990). In statistics, the P-test can be misused to overestimate the probability of having found a true effect. Likewise, in modelling studies certainty may be overestimated, thus producing numbers precise to the third decimal digit even in situations of pervasive uncertainty or ignorance, including important cases where science is needed to inform policy (Pilkey and Pilkey-Jarvis Citation2009a). Normative and cultural problems naturally compound the problem as for the case of statistics. It is an old refrain in mathematical modelling, first noted among hydro-geologists, that since models are often over-parametrized, they can be made to support any conclusion (Hornberger and Spear Citation1981).
Building on the notion of ‘Defogging the mathematics of uncertainty’, and more recent insights into the excess precision associated with impact assessment and risk analysis (Stirling Citation2023a), there is an evident need for greater clarity on how risk numbers are computed. It is essential to unveil the non-neutrality of techniques and of models (Saltelli et al. Citation2023; Saltelli et al. Citation2020) so as to allow each party in a conflicted policy issue to make best use of evidence based on numbers.
4. Conclusions
To statisticians (especially those concerned with social statistics) it may seem natural to consider that the quality of a quantification is both technical and normative (Salais Citation2022). This concept might be effectively transferred to modelling. Most modellers agree that while complex calculations are now being used to support important decisions, these decisions cannot be justified solely based on calculated results without an understanding of the associated uncertainties. At the same time, when confronted with the considerations collected in the present text, some elements of uneasiness do emerge among practitioners.
One is the deep-rooted resistance to ideas of non-neutrality of mathematical modelling. Here there is an important cultural and ritual element at play. The notion that a model can be an avenue of possibly instrumental ‘displacement’, in the sense of moving the attention from the system to its model, as discussed in Rayner (Citation2012), is still too radical for many practitioners to contemplate. Similarly, criticism against excessive or rhetorical complexity is often met with a defence of complex models on the basis of their ability to potentially ‘surprise’ the analyst. Overall, it is noted that modelling is too vast an enterprise to be boxed into a single quality assurance framework. The centrality of mathematical modelling and its insufficient quality control and governance arrangements would appear to offer the occasion to put quality at the core – or at least raise it as a priority theme – of a discussion about ethics of quantification (Di Fiore et al. Citation2022; Saltelli Citation2020). Still, we believe that there is sufficient material to give this process a good start (Oberkampf and Roy Citation2010; Santner, Williams, and Notz Citation2003).
A second source of discomfort is the sense that the methodologies advocated here may actually have excessive candour. Practitioners may be led to ignore SA and ‘adjust’ their level of uncertainty in the input upon discovering that the output results are too uncertain (Leamer Citation1985). Worse still, there is a possibility that SA might reveal that the source of a poor (i.e. diffuse) inference lies in an assumption that is hard to identify or to compress to a lower uncertainty. Along the same discomfort axis is the worry that SA may invalidate the use of models by showing the futility of an analysis (such as the cost-benefit modelling mentioned above), or by opening the door to relativism – whereby any framework can be upheld given some sort of evidence.
In the present work, we counter these doubts: we argue that it is better to deconstruct oneself systematically than to be deconstructed in the field. This consideration lies at the core of good scientific practice, as per Merton (Citation1973)’s principle of ‘Organized Scepticism’, whereby all ideas must be tested and subjected to rigorous, structured scrutiny within the community. Richard Feynman elaborated on this principle in his Cargo Cult lecture (Citation1985), emphasizing that a rigorous analyst ‘bends backward’ to offer to potential inquisitors all the elements to possibly deconstruct the analyst’s argument.
In econometrics, Mertonian concerns are known as the ‘honesty is the best policy’ approach (Leamer Citation2010), and this is the 10th law of applied econometrics as formulated by Peter Kennedy (Citation2008): ‘Thou shalt confess in the presence of sensitivity. Corollary: thou shalt anticipate criticism’. This reference to SA brings us back to the topic chosen as an illustration of statistics’ potential role in the rescue of mathematical modelling.
How needed is this rescue? Ravetz (Citation1971, 179) prophesized that entire research fields might become diseased, and noted: ‘reforming a diseased field, or arresting the incipient decline of a healthy one, is a task of great delicacy. It requires a sense of integrity, and a commitment to good work, among a significant section of the members of the field; and committed leaders with scientific ability and political skill’. While statistics has proven to possess the disciplinary arrangements and committed leaders to react to a crisis, mathematical modelling seems to lack both.
Declaration of Conflicting Interests
The Authors declare that there is no conflict of interest.
Acknowledgments
AS acknowledges the i4Driving project (Horizon 2020, Grant Agreement ID 101076165). AP was supported by funding from the UK Research and Innovation (UKRI) under the UK government’s Horizon Europe funding guarantee (project DAWN, PI Arnald Puy, EP/Y02463X/1).
Disclosure statement
No potential conflict of interest was reported by the author(s).
Notes
1. The relation between models and data is often more symbiotic than adversarial. In climate studies, this relation has been defined as ‘incestuous’, precisely to make the point that in modelling studies using data to prove a model wrong may not be straightforward (Edwards Citation1999).
References
- Akaike, Hirotugu. 2011. ‘Akaike’s Information Criterion’. In International Encyclopedia of Statistical Science, edited by M. Lovric, 25. Berlin: Springer. https://doi.org/10.1007/978-3-642-04898-2_110.
- Bauer, Peter, Bjorn Stevens, and Wilco Hazeleger. 2021. “A Digital Twin of Earth for the Green Transition.” Nature Climate Change 11 (2): 80–83. https://doi.org/10.1038/s41558-021-00986-y.
- Borges, Jorge Luis. 1946. ‘Del Rigor En La Ciencia (On Exactitude in Science)’. https://ciudadseva.com/texto/del-rigor-en-la-ciencia/.
- Borges, Jorge Luis. 1998. The Garden of Forking Paths. London: Penguin Books. https://www.penguin.co.uk/books/308559/the-garden-of-forking-paths-by-borges-jorge-luis/9780241339053.
- Boumans, Marcel. 1999. ‘Built-In Justification’. In Models As Mediators: Perspectives on Natural and Social Science, edited by Mary S. Morgan and Margaret Morrison, 66–96. Cambridge, New York: Cambridge University Press.
- Bourdieu, Pierre. 1984. Les héritiers. Paris: MINUIT.
- Brauneis, Robert, and Ellen P. Goodman. 2018. ‘Algorithmic Transparency for the Smart City’. Yale Journal of Law and Technology 20:103–176.
- Breznau, Nate, Eike Mark Rinke, Alexander Wuttke, Hung H. V. Nguyen, Muna Adem, Jule Adriaans, Amalia Alvarez-Benjumea, et al. 2022. “Observing Many Researchers Using the Same Data and Hypothesis Reveals a Hidden Universe of Uncertainty.” Proceedings of the National Academy of Sciences 119 (44): e2203150119. https://doi.org/10.1073/pnas.2203150119.
- Bruno, Isabelle, E. Didier, and J. Prévieux. 2014. Statactivisme. Comment Lutter Avec Des Nombres. Paris: Édition La Découverte.
- Bruno, Isabelle, Emmanuel Didier, and Tommaso Vitale. 2014. ‘Editorial: Statactivism: Forms of Action Between Disclosure and Affirmation’. The Open Journal of Sociopolitical Studies 2 (7): 198–220. https://doi.org/10.1285/i20356609v7i2p198.
- Chalmers, Iain, and Paul Glasziou. 2009. “Avoidable Waste in the Production and Reporting of Research Evidence.” The Lancet 374 (9683): 86–89. https://doi.org/10.1016/S0140-6736(09)60329-9.
- Chubin, Daryl E. 2001. ”Filling the Policy Vacuum Created by OTA’s Demise“. Issues in Science and Technology 17 (2).
- Concialdi, Pierre. 2014. ‘Le BIP40: Alerte Sur La Pauvreté’. In Statactivisme. Comment Lutter Avec Des Nombres, In edited by E. Didier Isabelle Bruno and J. Prévieux, 199–211. Paris: Édition La Découverte.
- Davies, Philip J., and Reuben Hersh. 1986. Descartes’ Dream: The World According to Mathematics (Dover Science Books) - Harvard Book Store. London: Penguin Books. https://www.harvard.com/book/descartes_dream_the_world_according_to_mathematics_dover_science_books/.
- Desrosières, Alain. 1998. The Politics of Large Numbers : A History of Statistical Reasoning. Paris: Harvard University Press.
- Devlin, Hannah, and Sarah Boseley. 2020. ‘Scientists Criticise UK Government’s “Following the Science” Claim’. The Guardian, April 2020. https://www.theguardian.com/world/2020/apr/23/scientists-criticise-uk-government-over-following-the-science.
- Di Fiore, Monica, Marta Kuc Czarnecka, Samuele Lo Piano, Arnald Puy, and Andrea Saltelli. 2022. ‘The Challenge of Quantification: An Interdisciplinary Reading’. Minerva 61 (1): 53–70. https://doi.org/10.1007/s11024-022-09481-w.
- Duhem, Pierre Maurice Marie. 1991. The Aim and Structure of Physical Theory. Princeton University Press. https://press.princeton.edu/books/paperback/9780691025247/the-aim-and-structure-of-physical-theory.
- Edwards, Paul N. 1999. “Global Climate Science, Uncertainty and Politics: Data‐Laden Models, Model‐Filtered Data.” Science as Culture 8 (4): 437–472. https://doi.org/10.1080/09505439909526558.
- Eker, Sibel, Elena Rovenskaya, Michael Obersteiner, and Simon Langan. 2018. “Practice and Perspectives in the Validation of Resource Management Models.” Nature Communications 9 (1): 5359. https://doi.org/10.1038/s41467-018-07811-9.
- Feyerabend, Paul. 1988. Farewell to Reason. Illustrated edition. London ; New York: Verso.
- Feynman, Richard P. 1985 Surely you’re joking, Mr. Feynman! (New York, USA: W.W. Norton and Company.) 400 978-0393355628
- Funtowicz, Silvio, and Jerome R. Ravetz. 1990. Uncertainty and Quality in Science for Policy. Dordrecht: Kluwer. https://doi.org/10.1007/978-94-009-0621-1_3.
- Gelman, Andrew, and Eric Loken. 2013. ‘The Garden of Forking Paths’. Working Paper Department of Statistics, Columbia University. http://www.stat.columbia.edu/~gelman/research/unpublished/p_hacking.pdf.
- Gigerenzer, Gerd. 2018. “Statistical Rituals: The Replication Delusion and How We Got There.” Advances in Methods and Practices in Psychological Science 1 (2): 198–218. https://doi.org/10.1177/2515245918771329.
- Gigerenzer, Gerd, and J. N. Marewski. 2014. ‘Surrogate Science: The Idol of a Universal Method for Scientific Inference’. Journal of Management, (2): 0149206314547522. https://doi.org/10.1177/0149206314547522.
- Hall, Charles A. S. 2020. ‘Systems Ecology and Limits to Growth: History, Models, and Present Status’. In Handbook of Systems Sciences, edited by Gary S. Metcalf, Kyoichi Kijima, and Hiroshi Deguchi, 1–38. Singapore: Springer. https://doi.org/10.1007/978-981-13-0370-8_77-1.
- Hall, Charles A. S., and John W. Day. 1977. Ecosystem Modeling in Theory and Practice: An Introduction with Case Histories. First Edition. New York: John Wiley & Sons.
- Hornberger, G. M., and R. C. Spear. 1981. ‘An Approach to the Preliminary Analysis of Environmental Systems’. Journal of Environmental Management 12 (1): 7–18.
- Ioannidis, John P. A. 2022. “Pre-Registration of Mathematical Models.” Mathematical Biosciences 345 (March): 108782. https://doi.org/10.1016/j.mbs.2022.108782.
- Jakeman, A. J., R. A. Letcher, and J. P. Norton. 2006. ‘Ten Iterative Steps in Development and Evaluation of Environmental Models. Environmental Modelling & Software 21 (5): 602–614. https://doi.org/10.1016/j.envsoft.2006.01.004.
- James, Gareth, Daniela Witten, Trevor Hastie, and Robert Tibshirani. 2017. An Introduction to Statistical Learning : With Applications in R. New York, USA: Springer.
- Kantor, Jodi, Arya Sundaram, Aliza Aufrichtig, and Rumsey Taylor. 2022. ‘The Rise of the Worker Productivity Score’. The New York Times, 15 August 2022. Business. https://www.nytimes.com/interactive/2022/08/14/business/worker-productivity-tracking.html.
- Kennedy, Peter. 2008. A Guide to Econometrics. 6th ed. Oxford, United Kingdom: Wiley-Blackwell.
- Kerr, Norbert L. 1998. “HARKing: Hypothesizing After the Results Are Known.” Personality and Social Psychology Review 2 (3): 196–217. https://doi.org/10.1207/s15327957pspr0203_4.
- Lakoff, George, and Rafael Núñez. 2001. Where Mathematics Come From: How the Embodied Mind Brings Mathematics into Being. Basic Books. https://www.goodreads.com/book/show/53337.Where_Mathematics_Come_From.
- Landler, Mark, and Stephen Castle. 2020. Behind the Virus Report That Jarred the U.S. and the U.K. to Action - The New York Times. The New York Times. https://www.nytimes.com/2020/03/17/world/europe/coronavirus-imperial-college-johnson.html.
- Lane, S. N., N. Odoni, C. Landström, S. J. Whatmore, N. Ward, and S. Bradley. 2011. “Doing Flood Risk Science Differently: An Experiment in Radical Scientific Method.” Transactions of the Institute of British Geographers 36 (1): 15–36. https://doi.org/10.1111/j.1475-5661.2010.00410.x.
- Leamer, Edward E. 1985. ‘Sensitivity Analyses Would Help’. The American Economic Review 75 (3): 308–313.
- Leamer, Edward E. 2010. “Tantalus on the Road to Asymptopia.” Journal of Economic Perspectives 24 (2): 31–46. https://doi.org/10.1257/jep.24.2.31.
- Lo Piano, Samuele, János Máté Lőrincz, Arnald Puy, Steve Pye, Andrea Saltelli, Stefán Thor Smith, and Jeroen P. van der Sluijs. 2023. ‘Unpacking Uncertainty in the Modelling Process for Energy Policy Making’. Risk Analysis, November. https://onlinelibrary.wiley.com/doi/epdf/10.1111/risa.14248.
- Lo Piano, Samuele, Razi Sheikholeslami, Arnald Puy, and Andrea Saltelli. 2022. ‘Unpacking the Modelling Process via Sensitivity Auditing’. Futures, (September). https://doi.org/10.1016/j.futures.2022.103041. 144 103041
- Marris, Claire 2001. ‘Final Report of the PABE Research Project Funded by the Commission of the European Communities, Contract Number: FAIR CT98-3844 (DG 12–SSMI)’. https://eur-lex.europa.eu/legal-content/EN/TXT/PDF/?uri=CELEX:52001DC0098.
- Martin, Felix. 2018. ‘Unelected Power: Banking’s Biggest Dilemma’. New Statemen, 13 June 2018. https://www.newstatesman.com/culture/2018/06/unelected-power-banking-s-biggest-dilemma.
- McQuillan, Dan. 2022. Resisting AI: An Anti-Fascist Approach to Artificial Intelligence. Bristol University Press. https://www.amazon.es/Resisting-AI-Anti-fascist-Artificial-Intelligence/dp/1529213495.
- Mennicken, Andrea, and Wendy Nelson Espeland. 2019. “What’s New with Numbers? Sociological Approaches to the Study of Quantification.” Annual Review of Sociology 45 (1): 223–245. https://doi.org/10.1146/annurev-soc-073117-041343.
- Mennicken, Andrea, and Robert Salais, eds. 2022. The New Politics of Numbers: Utopia, Evidence and Democracy. London, United Kingdom: Palgrave Macmillan.
- Merton, R. K. 1973. The Sociology of Science: Theoretical and Empirical Investigations. Edited by Chicago, Illinois, USA: University of Chicago Press.
- Moeller, H. G. 2006. Luhmann Explained. Chicago, Illinois, USA: Open Court Publishing Company.
- Morgan, Mary S., and Margaret Morrison, eds. 1999. Models As Mediators: Perspectives on Natural and Social Science. Cambridge, New York: Cambridge University Press.
- Morrison, Foster. 1991. The art of modeling dynamic systems: Forecasting for chaos, randomness, and determinism, 414. Mineola, New York, USA: Dover Publications.
- Morrison, Margaret, and May S. Morgan 1999. ‘Models As Mediating Instruments.’ In Models As Mediators: Perspectives on Natural and Social Science, edited by Mary S. Morgan and Margaret Morrison, 10–37. Cambridg New York: Cambridge University Press.
- Muller, Jerry Z. 2018. The Tyranny of Metrics. Princeton, New Jersey, USA: Princeton University Press.
- Munafò, M. R., B. A. Nosek, D. V. Bishop, K. S. Button, C. D. Chambers, N. Percie du Sert, U. Simonsohn, E. J. Wagenmakers, J. J. Ware, and J. P. A. Ioannidis. 2017. ‘A Manifesto for Reproducible Science’. Nature Human Behaviour 1 (1): 1–9. https://doi.org/10.1038/s41562-016-0021.
- Oberkampf, William L., and Christopher J. Roy. 2010. Verification and Validation in Scientific Computing. Cambridge, United Kingdom: Cambridge University Press.
- O’Neil, Cathy. 2016. Weapons of Math Destruction : How Big Data Increases Inequality and Threatens Democracy. New York, USA: Random House Publishing Group.
- O’Neill, R. V. 1971. ‘Error Analysis of Ecological Models’. In Radionuclides in Ecosystems, Proceedings of the Third National Symposium in Radioecology, edited by D. J. Nelson, 898–907. Oak Ridge - Tenn.
- Oreskes, Naomi. 2010. ‘Why Predict? Historical Perspectives on Prediction in Earth Sciences’. In Prediction, Science, Decision Making and the Future of Nature, edited by Daniel R. Sarewitz, Roger A. Pielke, and Radford Byerly, 23–40. Island Press. http://trove.nla.gov.au/work/6815576?selectedversion=NBD21322691.
- Padilla, Jose J, Saikou Y Diallo, Christopher J Lynch, and Ross Gore. 2018. “Observations on the Practice and Profession of Modeling and Simulation: A Survey Approach.” Simulation 94 (6): 493–506. https://doi.org/10.1177/0037549717737159.
- Page, Scott E. 2018. The Model Thinker: What You Need to Know to Make Data Work for You. 1st edition. New York: Basic Books.
- Pfleiderer, Paul. 2020. “Chameleons: The Misuse of Theoretical Models in Finance and Economics.” Economica 87 (345): 81–107. https://doi.org/10.1111/ecca.12295.
- Pielke, Roger, Jr. 2020. ‘The Mudfight Over ‘Wild-Ass’ Covid Numbers Is Pathological’. Wired, April. https://www.wired.com/story/the-mudfight-over-wild-ass-covid-numbers-is-pathological/.
- Pilkey, Orrin H., and L. Pilkey-Jarvis. 2009a. Useless Arithmetic: Why Environmental Scientists Can’t Predict the Future. New York, USA: Columbia University Press.
- Pilkey, Orrin H., and L. Pilkey-Jarvis. 2009b. ‘Yucca Mountain: A Million Years of Safety’. In Useless Arithmetic: Why Environmental Scientists Can’t Predict the Future, 45–65. New York, USA: Columbia University Press.
- ‘Planet Normal: Bob Seely MP on the “national Scandal” of “Hysterical” Covid Modelling’. 2022. Planet Normal, the Telegraph. https://www.youtube.com/watch?v=NyASSAfdM18.
- Porter, Theodore M. 1995. Trust in Numbers: The Pursuit of Objectivity in Science and Public Life. Princeton University Press. https://books.google.es/books?id=oK0QpgVfIN0C.
- Porter, Theodore M. 2012. ‘Funny Numbers’. Culture Unbound 4:585–598. (4) https://doi.org/10.3384/cu.2000.1525.124585.
- Puy, Arnald, Pierfrancesco Beneventano, Simon A. Levin, Samuele Lo Piano, Tommaso Portaluri, and Andrea Saltelli. 2022. ‘Models with Higher Effective Dimensions Tend to Produce More Uncertain Estimates’. Science Advances 8 (eabn9450). https://doi.org/10.1126/sciadv.abn9450.
- Puy, Arnald, Samuele Lo Piano, and Andrea Saltelli. 2020. “Current Models Underestimate Future Irrigated Areas.” Geophysical Research Letters 47 (8): e2020GL087360. https://doi.org/10.1029/2020GL087360.
- Qi, Liyan. 2024. ‘How China Miscalculated Its Way to a Baby Bust’. WSJ, 12 February 2024. World. https://www.wsj.com/world/china/china-population-births-economy-one-child-c5b95901.
- Quine, W. V. O. 1951. “Two Dogmas of Empiricism.” The Philosophical Review 60 (1951): 20–43. Reprinted in W.V.O. Quine, From a Logical Point of View (Harvard University Press, 1953; second, revised, edition 1961).
- Ravetz, Jerome R. 1971. Scientific Knowledge and Its Social Problems. Oxford, United Kingdom: Oxford University Press.
- Ravetz, Jerome R. 2008. ‘Faith and Reason in the Mathematics of the Credit Crunch’. The Oxford Magazine. Eight Week, Michaelmas Term 14–16. http://www.pantaneto.co.uk/issue35/ravetz.htm.
- Ravetz, Jerome R. 2023. ‘Models As Metaphors’. In The Politics of Modelling. Numbers Between Science and Policy, edited by Andrea Saltelli and Monica Di Fiore. Oxford, United Kingdom: Oxford University Press.
- Rayner, Steve. 2012. “Uncomfortable Knowledge: The Social Construction of Ignorance in Science and Environmental Policy Discourses.” Economy and Society 41 (1): 107–125. https://doi.org/10.1080/03085147.2011.637335.
- Rhodes, Tim, and Kari Lancaster. 2020. ‘Mathematical Models As Public Troubles in COVID-19 Infection Control: Following the Numbers’. Health Sociology Review, May, 177–194. https://doi.org/10.1080/14461242.2020.1764376.
- Rosen, R. 1991. Life Itself: A Comprehensive Inquiry into the Nature, Origin, and Fabrication of Life. In Complexity in Ecological Systems Series. New York, USA: Columbia University Press.
- Salais, Robert. 2022. ‘“La Donnée n’est Pas Un Donné”: Statistics, Quantification and Democratic Choice’. In The New Politics of Numbers: Utopia, Evidence and Democracy, edited by Andrea Mennicken and Rober Salais, 379–415. London, United Kingdom: Utopia, Evidence and Democracy. Palgrave Macmillan.
- Saltelli, Andrea. 2020. ‘Ethics of Quantification or Quantification of Ethics?’ Futures 116 (102509). https://doi.org/10.1016/j.futures.2019.102509.
- Saltelli, Andrea, Gabriele Bammer, Isabelle Bruno, Erica Charters, Monica Di Fiore, Emmanuel Didier, Wendy Nelson Espeland, et al. 2020a. ‘Five Ways to Ensure That Models Serve Society: A Manifesto’. Nature 582:482–484. 7813 https://doi.org/10.1038/d41586-020-01812-9.
- Saltelli, Andrea, Gabriele Bammer, Isabelle Bruno, Erica Charters, Monica Di Fiore, Emmanuel Didier, Wendy Nelson Espeland, et al. 2020b. ‘Five Ways to Make Models Serve Society: A Manifesto - Supplementary Online Material’. Nature 482–484. 582 7813 https://doi.org/10.1038/d41586-020-01812-9.
- Saltelli, Andrea, L. Benini, S. Funtowicz, M. Giampietro, M. Kaiser, E. Reinert, and P. van der Sluijs. Jeroen 2020. ‘The Technique Is Never Neutral. How Methodological Choices Condition the Generation of Narratives for Sustainability’. Environmental Science & Policy 106:87–98.https://doi.org/10.1016/j.envsci.2020.01.008.
- Saltelli, Andrea, and Monica Di Fiore, eds. 2023. The Politics of Modelling. Numbers Between Science and Policy. Oxford: Oxford University Press.
- Saltelli, Andrea, and Silvio Funtowicz. 2017. “What Is Science’s Crisis Really About?” Futures 91:5–11. https://doi.org/10.1016/j.futures.2017.05.010.
- Saltelli, Andrea, Ângela Guimaraes Pereira, Jeroen P. van der Sluijs, and Silvio Funtowicz. 2013. ‘What Do I Make of Your Latinorum” Sensitivity Auditing of Mathematical Modelling’. International Journal of Foresight and Innovation Policy 9 (2/3/4): 213–234. https://doi.org/10.1504/IJFIP.2013.058610.
- Saltelli, Andrea, Marta Kuc-Czarnecka, Samuele Lo Piano, János Máté Lőrincz, Magdalena Olczyk, Arnald Puy, Erik Reinert, Stefán Thor Smith, and Jeroen P. van der Sluijs. 2023. ‘Impact Assessment Culture in the European Union. Time for Something New?’ Environmental Science & Policy 142 (April): 99–111. https://doi.org/10.1016/j.envsci.2023.02.005.
- Saltelli, Andrea, and Arnald Puy. 2022. ‘What Can Mathematical Modelling Contribute to a Sociology of Quantification?’ SSRN Scholarly Paper. Rochester, NY. https://doi.org/10.2139/ssrn.4212453.
- Saltelli, Andrea, Joachim P. Sturmberg, Daniel Sarewitz, and John P. A. Ioannidis. 2023. “What Did COVID-19 Really Teach Us About Science, Evidence and Society?” Journal of Evaluation in Clinical Practice 29 (8): 1237–1239. https://doi.org/10.1111/jep.13876.
- Samuel, Boris. 2022. ‘The Shifting Legitimacies of Price Measurements:Official Statistics and the Quantification of Pwofitasyon in the 2009 Social Struggle in Guadeloupe’. In The New Politics of Numbers: Utopia, Evidence and Democracy, Andrea Mennicken and Robert Salais, 337–377. London, United Kingdom: Executive Policy and Governance. Palgrave Macmillan.
- Santner, Thomas J., Brian J. Williams, and William I. Notz. 2003. The Design and Analysis of Computer Experiments. New York, USA: Springer-Verlag.
- Schwarz, Gideon. 1978. ‘Estimating the Dimension of a Model’. Annals of Statistics 6 (2): 461–464. https://doi.org/10.1214/aos/1176344136.
- Sen, Amartya. 1990. ‘Justice: Means versus Freedoms’. Philosophy & Public Affairs 19 (2): 111–121.
- Stanford, Kyle. 2023. ‘Underdetermination of Scientific Theory’. In The Stanford Encyclopedia of Philosophy, edited by Edward N. Zalta and Uri Nodelman. Metaphysics Research Lab, Stanford University. https://plato.stanford.edu/archives/sum2023/entries/scientific-underdetermination/.
- Stark, Philip B., and Andrea Saltelli. 2018. “Cargo-Cult Statistics and Scientific Crisis.” Significance 15 (4): 40–43. https://doi.org/10.1111/j.1740-9713.2018.01174.x.
- Stirling, Andy. 2023a. “Against Misleading Technocratic Precision in Research Evaluation and Wider Policy – a Response to Franzoni and Stephan (2023), ‘Uncertainty and Risk-Taking in Science’”. Research Policy 52 (3): 104709. https://doi.org/10.1016/j.respol.2022.104709.
- Stirling, Andy. 2023b. ‘Mind the Unknown: Exploring the Politics of Ignorance in Mathematical Models’. In The Politics of Modelling. Numbers Between Science and Policy, edited by Andrea Saltelli, 99–118. Oxford, United Kingdom: and Monica Di Fiore. Oxford University Press.
- Stothoff, Stuart A., and Gary R. Walter. 2013. “Average Infiltration at Yucca Mountain Over the Next Million Years.” Water Resources Research 49 (11): 7528–7545. https://doi.org/10.1002/2013WR014122.
- Supiot, Alain. 2017. Governance by Numbers: The Making of a Legal Model of Allegiance. Oxford, United Kingdom: Hart Publishing.
- Szenberg, Michael. 1992. Eminent Economists: Their Life Philosophies. Cambridge University Press. http://admin.cambridge.org/gb/academic/subjects/economics/history-economic-thought-and-methodology/eminent-economists-their-life-philosophies#dkQwZVJ4RazyzwHC.97.
- Teachout, Zephyr. 2022. ‘The Boss Will See You Now | Zephyr Teachout’. New York Review of Books, 2022. https://www.nybooks.com/articles/2022/08/18/the-boss-will-see-you-now-zephyr-teachout/.
- Turner, Monica G., and Robert H. Gardner. 2015. ‘Introduction to Models’. In Landscape Ecology in Theory and Practice, 63–95. New York, NY: Springer New York. https://doi.org/10.1007/978-1-4939-2794-4_3.
- van Beek, Lisette, Jeroen Oomen, Maarten Hajer, Peter Pelzer, and Detlef van Vuuren. 2022. ‘Navigating the Political: An Analysis of Political Calibration of Integrated Assessment Modelling in Light of the 1.5 °C Goal’. Environmental Science & Policy 133 (July): 193–202. https://doi.org/10.1016/j.envsci.2022.03.024.
- Weinberg, A. M. 1972. “Science and Trans-Science.” Minerva 10 (2): 209–222. https://doi.org/10.1007/BF01682418.
- Winner, Langdon. 1989. The Whale and the Reactor : A Search for Limits in an Age of High Technology. Chicago, Illinois, USA: University of Chicago Press.
- Zuboff, Shoshana. 2019. The Age of Surveillance Capitalism : The Fight for a Human Future at the New Frontier of Power. New York, USA: PublicAffairs.