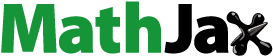
ABSTRACT
Speech intelligibility is an essential though complex construct in speech pathology. In this paper, we investigated the interrater reliability and validity of two types of intelligibility measures: a rating-based measure, through Visual Analogue Scales (VAS), and a transcription-based measure called Accuracy of Words (AcW), through two forms of orthographic transcriptions, one containing only existing words (EWTrans) and one allowing all sorts of words, including both existing words and pseudowords (AWTrans). Both VAS and AcW scores were collected from five expert raters. We selected speakers with various severity levels of dysarthria (SevL) and employed two types of speech materials, i.e. meaningful sentences and word lists. To measure reliability, we applied Generalizability Theory, which is relatively unknown in the field of pathological speech and language research but enables more comprehensive analyses than traditional methods, e.g., the intraclass correlation coefficient. The results convincingly indicate that five expert raters were sufficient to provide reliable rating-based (VAS) and transcription-based (AcW) measures, and that reliability increased as the number of raters or utterances increased. Generalizability Theory has proved effective in systematically dealing with reliability issues in our experimental design. We also investigated construct and concurrent validity. Construct validity was addressed by exploring the correlations between VAS and AcW within and across speech materials. Concurrent validity was addressed by exploring the correlations between our measures, i.e. VAS and AcW, and two external measures, i.e. phoneme intelligibility and SevL. The correlations corroborate the validity of VAS and AcW to assess speech intelligibility, both in sentences and word lists.
Introduction
Dysarthria is a motor speech disorder caused by neurological injury, e.g., Parkinson’s disease and stroke. It can result in losing control of tongue, larynx, vocal folds and surrounding muscles, thus leading to reduced speech intelligibility and possibly to consequent loss of social participation (Hustad, Citation2008). Patients with dysarthria may receive intensive speech therapy, e.g., Lee Silverman Voice Treatment (LSVT), to improve their intelligibility (Cannito et al., Citation2012; Levy et al., Citation2020; Nakayama et al., Citation2020; Yuan et al., Citation2020). For diagnosis and therapy effectiveness, it is necessary to have a clear definition of speech intelligibility. Over the years different definitions and related measurement methods of speech intelligibility have been advanced (for an overview, see Dos Barreto & Ortiz, Citation2008; Miller, Citation2013). In our research, we have adopted the definition proposed by Hustad (Citation2008): “how well a speaker’s acoustic signal can be accurately recovered by a listener” (p. 1).
According to this definition, an acceptable measurement method of speech intelligibility could be an orthographic transcription that represents the number of correctly perceived words by a listener. In collecting these transcriptions, subjects are normally instructed to use only existing words. Accordingly, word lists consisting of only existing, meaningful words are commonly used, as well as sentences, which are semantically either predictable (meaningful) or unpredictable (Abur et al., Citation2019; Beijer et al., Citation2012; Carvalho et al., Citation2021; Dos Barreto & Ortiz, Citation2008, Citation2016; Ganzeboom et al., Citation2016; Hodge & Gotzke, Citation2014; Hustad, Citation2006, Citation2007, Citation2008; Hustad & Cahill, Citation2003; Ishikawa et al., Citation2021; Liss et al., Citation2002; Middag, Citation2012; Miller, Citation2013; Stipancic et al., Citation2016; Sussman & Tjaden, Citation2012; Tjaden et al., Citation2014; Tjaden & Liss, Citation1995a, Citation1995b; Tjaden & Wilding, Citation2010; Xue et al., Citation2020, Citation2021; Yorkston & Beukelman, Citation1978, Citation1981; Yorkston et al., Citation1996).
However, such transcriptions force raters to align a sequence of phonemes with an existing word and thus, more specific information on speech deviations is likely to be omitted. Therefore, less restricted instructions about the transcriptions may be needed to capture this information. For example, Xue et al. (Citation2021) found a very low interrater reliability (0.47) for a word-level measure of intelligibility, namely word accuracy, obtained from orthographic transcriptions based on existing words only. This reliability was much lower than that (0.93) for the measure of intelligibility obtained with Visual Analogue Scales (VAS). The low reliability of word accuracy scores was caused by the poor variability of scores since many of the utterances received scores of 100, indicating perfect intelligibility. On the other hand, these utterances did receive VAS scores lower than 100, indicating imperfect and lower intelligibility. Also, the VAS scores had a wider range of variability. These results suggested that although raters may have perceived speech deviations, e.g., a distortion, deletion, or substitution of a phoneme in a word, as indicated by the imperfect intelligibility and the wide range of variability in the VAS scores, they still had to transcribe the same existing, meaningful words to follow the instructions requiring only existing words. Alternatively, instructions that allow also pseudowords would seem to provide raters with more flexibility in transcribing words and so help report speech deviations at segmental level.
Because orthographic transcriptions are laborious and time-consuming, researchers have adopted more efficient methods that are based on raters’ perception or estimates of speech intelligibility (Abur et al., Citation2019; Ganzeboom et al., Citation2016; Ishikawa et al., Citation2021; Sussman & Tjaden, Citation2012; Xue et al., Citation2020; Yorkston & Beukelman, Citation1978, Citation1981; Yorkston et al., Citation1996). In such methods, raters are asked to indicate how intelligible a speech utterance is by assigning a numeric value on a scale such as a VAS (Abur et al., Citation2019; Ganzeboom et al., Citation2016; Stipancic et al., Citation2016; Sussman & Tjaden, Citation2012; Xue et al., Citation2020; Yorkston & Beukelman, Citation1978; Yorkston et al., Citation1996), or an x-point scale with equal appearing intervals, as in Likert scales (EAI; Ganzeboom et al., Citation2016; Miller, Citation2013), or by estimating the percentage of understandable words, which is called percentage estimates (Yorkston & Beukelman, Citation1978). Studies using these methods have found different results. Some researchers reported that transcription-based measures showed higher intelligibility scores for the same speech samples than rating-based ones using EAI and VAS (Ganzeboom et al., Citation2016), or percentage estimates (Hustad, Citation2006). Other studies found transcription-based measures were comparable to percentage estimates (Yorkston & Beukelman, Citation1978) or were highly correlated to VAS scores (Abur et al., Citation2019; Ishikawa et al., Citation2021; Schiavetti, Citation1992; Stipancic et al., Citation2016).
A broad consensus is that orthographic transcriptions yield reliable and valid measures (Bunton et al., Citation2001; Miller, Citation2013; Tjaden & Wilding, Citation2010), since they rely on the amount of information listeners accurately perceived. In contrast, rating tasks have been questioned, since they rely on the listeners’ impression of intelligibility. Rating tasks have been found to yield measures with reliability values too low for research purposes (Miller, Citation2013; Schiavetti, Citation1992). Nevertheless, rating-based measures through VAS have shown promise for measuring intelligibility (Kent & Kim, Citation2011; Van Nuffelen et al., Citation2010), with an interrater reliability comparable to that of orthographic transcriptions.
A point of concern here is that the interrater reliability of these measures has been evaluated by different statistical analyses. Some studies (e.g., Hustad, Citation2007; Van Nuffelen et al., Citation2008) reported percentage agreement between raters without dealing with chance agreement. Others (e.g., Hustad, Citation2006, Citation2008) used Pearson correlations taking only raters as a source of variance. The Intraclass Correlation Coefficient (ICC; Fisher, Citation1992) has become the standard measure of interrater reliability in pathological speech research (Rietveld, Citation2020). However, ICC can only handle two factors, i.e. speaker and rater, in a crossed design where all raters assess all speakers. This approach to reliability has been expanded into an overarching type of analysis, called Generalizability Theory (G Theory; Brennan, Citation2001), which is based on the ICC but can take more than two sources of variance (utterances, speakers, and raters in our case) into account. G Theory can handle not only crossed designs but also nested designs, in which different raters assess different utterance samples of one or more speakers. In addition, G Theory allows calculating the optimal number of raters and utterance samples required to obtain reliable measures by conducting a decision study. In fact, a growing number of studies have conducted reliability analyses through G Theory and showed effectiveness in defining optimal measurement procedures in different disciplines. For example, Ford and Johnson (Citation2021) explored a multidimensional understanding of reliability through G Theory and gained insights into optimal measurement procedures for examining the language of preschool educators interacting with children with an autism spectrum disorder. O’Brian et al. (Citation2003) applied G Theory for assessing the reliability of ratings from 15 raters through the 9-point speech naturalness scale for adults’ speech collected before and after treatment for stuttering. They successfully distinguished various sources of measurement error and used these to estimate the minimum number of raters and ratings per rater for a reliable result. Hollo et al. (Citation2020) applied G Theory to optimize the analysis of spontaneous teacher talk in elementary classrooms with teacher and sample duration as two factors. They assessed the minimum number and duration of samples needed for a reliable result. They found that a large proportion of variance was attributable to individuals rather than the sampling duration. To the best of our knowledge, G Theory has not been used for investigating experimental designs of speech intelligibility assessment of pathological speech. Rietveld (Citation2020) explains the relevance of the G Theory approach in speech and language pathological research when multiple sources of variance are involved, including raters.
Another point of concern is that, up to now, relatively few studies have addressed the validity of speech intelligibility measures (Ellis & Fucci, Citation1991; Hustad, Citation2007; Stipancic et al., Citation2016; Van Nuffelen et al., Citation2008). Validity indicates the extent to which the scores measure what they intend to measure. This is a key question in research and it is therefore important to investigate validity. Studies addressing the validity of intelligibility measures were normally conducted with hearing-impaired subjects or children. Dos Barreto and Ortiz (Citation2016) investigated the criterion validity of a transcription-based measure using two types of materials, i.e. sentences and word lists. Validity was studied in relation to speaker types, i.e. control and dysarthric speakers. Word lists appeared to have significantly greater discriminatory power than sentences. Hodge and Gotzke (Citation2014) evaluated the construct-related validity of the Test of Children’s Speech (TOCS), which uses transcription-based measures for children with and without a speech disorder. Results supported the usage of TOCS as a valid tool for measuring the intelligibility of children.
Many factors such as speech materials, severity levels of dysarthria, and raters’ experience and familiarity (Miller, Citation2013) have been shown to affect intelligibility measures. Firstly, intelligibility measures perform differently on different lengths of speech materials but this difference does not seem to be consistent across different speakers’ severity levels. Specifically, intelligibility scores for sentences have been found higher than those for words due to additional contextual cues when speech is mildly and moderately dysarthric (Hustad, Citation2007; Yorkston & Beukelman, Citation1978, Citation1981). However, when speech is more severely dysarthric, the intelligibility measures at sentence level could be higher than, equal to (Dongilli, Citation1994; Dos Barreto & Ortiz, Citation2008; Middag et al., Citation2009a; Yorkston & Beukelman, Citation1978), or lower (Yorkston & Beukelman, Citation1981) than those at word level. One possible reason may be that speakers with more severe dysarthria might have so many difficulties in producing sentences that listeners are no longer able to benefit from the contextual cues present in sentences. Secondly, raters’ experience could also influence intelligibility assessment. For instance, Carvalho et al. (Citation2021) reported significant differences in speech intelligibility ratings of speakers with Parkinson’s disease assigned by healthcare professionals, referring to experienced (i.e. ‘expert’) raters, and inexperienced (i.e. ‘lay’ or ‘naive’) raters. Similarly, Monsen (Citation1983)found that experienced and inexperienced raters significantly differed in the evaluation of intelligibility in adolescents with hearing impairment. In contrast, other researchers reported no differences (Ellis & Fucci, Citation1991; Maruthy & Raj, Citation2014). For instance, Maruthy and Raj (Citation2014) investigated the performance of 10 naïve and 10 expert raters in evaluating speakers with hypokinetic dysarthria. They found no effect of listener experience on speech intelligibility computed as the percentage of correctly transcribed words, although they did find a significant effect on listener effort ratings. Nevertheless, as pointed out by Mencke et al. (Citation1983), measures collected from inexperienced raters tend to show larger variation than those collected from well-trained expert raters such as speech-language therapists. Therefore, expert raters such as speech-language therapists were preferred over naïve listeners in the current study. In addition, raters’ familiarity with either speakers or speech materials has been reported to increase intelligibility scores (Hustad & Cahill, Citation2003; Liss et al., Citation2002; Tjaden & Liss, Citation1995a, Citation1995b) and thus, listening times of utterances to be assessed should be limited to reduce the impact of familiarity.
In order to better understand the performance of different speech intelligibility measures, as well as their interrater reliability and validity, we conducted a study that addressed (a) two types of intelligibility measures, i.e. one rating-based measure through VAS and one transcription-based measure through orthographic transcriptions, (b) two types of speech materials, i.e. meaningful sentences selected from a phonetically-balanced narrative and word lists consisting of unconnected, monosyllable pseudowords and existing words, and (c) speakers with different severity levels of dysarthria. Moreover, for transcription-based measures, we adopted two forms of transcription. One, called Existing-Word Transcription (EWTrans), allows only existing, meaningful words and has been commonly applied in previous studies. The other one, called All-Word Transcription (AWTrans), allows all sorts of words, including pseudowords. One of the reasons for applying AWTrans was that AWTrans was the only reasonable choice for one of our speech materials (word lists) due to the pseudowords it contained. In this way, we were able to compare intelligibility measures between the two types of speech materials. Another reason was that as AWTrans has not been investigated on meaningful sentences, we applied it together with EWTrans to investigate (1) whether AWTrans can generate reliable measures for meaningful sentences, and (2) whether the newly-proposed form (AWTrans) differs from the commonly-applied form (EWTrans). In addition, for assessing interrater reliability, we applied G Theory to (1) analyse the effects of utterances, raters and speakers in one overall analysis, and to (2) evaluate the number of raters and utterances needed to obtain reliable measures. By doing so, we focused on providing interesting insights and guidance for reliability analyses in research of speech and language pathology. Our study addressed the following three research questions:
To what extent are intelligibility measures reliable?
How many raters and utterance samples per speaker are needed to obtain reliable intelligibility measures?
To what extent are intelligibility measures valid?
Method
In this study, we investigated two types of speech intelligibility measures, a rating-based measure through VAS and a transcription-based measure through two forms of orthographic transcriptions. Two separate listening experiments, the Sentence Experiment and the Word Experiment, were designed with each involving one specific type of speech material, meaningful sentences selected from a narrative and word lists containing existing words and pseudowords. The speech materials and the speakers were selected from the Corpus of Pathological and Normal Speech (COPAS) databaseFootnote1 (Middag, Citation2012). This database contains recordings from a large number of speakers of Belgian Dutch (the variety of Dutch spoken in Flanders, the northern part of Belgium) with and without speech disorders, with reading materials (isolated words, isolated sentences, short passages) and spontaneous speech. The two listening experiments were conducted within the research project Developing valid measurement procedure of pathological speech intelligibility (application 2019–3197) that has been approved by the Ethics Assessment Committee Humanities of the Faculty of Arts and the Faculty of Philosophy, Theology and Religious Studies at the Radboud University with reference number Let/MvB19U.514400.
Speech material
For the Sentence Experiment, we selected four meaningful sentences from the Dutch commonly-used phonetically balanced narrative ‘Papa en Marloes’ (PM, ‘Papa and Marloes’ in English; Van de Weijer & Slis, Citation1991):
‘Papa en Marloes staan op het station. ’ (PM1, in English ‘Papa and Marloes are at the station.’),
‘Marloes kijkt naar links.’ (PM2, in English ‘Marloes looks to the left.’),
‘In de verte ziet ze de trein al aankomen. ’ (PM3, in English ‘In the distance she can see the train coming.’),
‘Het is al vijf over drie dus het duurt nog vier minuten.’ (PM4, in English ‘It is already five past three so it will take another four minutes.’).
These sentences vary in length and contain the corner vowels, i.e. /a:/, /u/, and /i/, which may be relevant for future acoustical analyses. Accordingly, for each speaker, four recordings were made, each of which being a reading of one of the sentences.
For the Word Experiment, we selected word lists from those constructed in the Dutch Intelligibility Assessment (DIA) task (De Bodt et al., Citation2006), which was designed to assess intelligibility at the phoneme level called Phoneme Intelligibility (PhonI). Unlike the Sentence Experiment, in which speakers read the same four sentences, speakers in the Word Experiment received three word lists, each of which was a variant of those for each of three subsets, i.e. A, B and C, constructed in the DIA task. These three subsets are designed to assess initial consonants, final consonants and medial vowels of Consonant-Vowel-Consonant (CVC) words, including both existing words and pseudowords, respectively. Accordingly, for each speaker, three recordings were made, each of which being a reading of a word list (a variant of a subset).
Speakers
The COPAS database contains recordings from 197 dysarthric speakers and 122 healthy speakers. The recordings covered different speech materials. However, since we were interested in the four meaningful sentences and word lists of the DIA task, as described above, we focused on speakers (49 dysarthric and 83 healthy speakers) whose recordings of these two speech materials were available. In order to ensure the diversity of speaker data, we carefully selected 26 dysarthric speakers based on their identical proportions among 49 dysarthric speakers in terms of dysarthria type, severity levels of dysarthria (mild-moderate-severe), PhonI scores obtained through the original DIA task, age and gender. Based on the same selection principle, we selected 10 healthy speakers out of 83 healthy speakers as a non-dysarthric group. The number of non-dysarthric speakers was smaller than that of dysarthric speakers because we focused on dysarthric speakers, but we also maintained the possibility of comparing dysarthric and non-dysarthric speakers. In total, we selected 36 speakers for the Sentence Experiment, and half of them for the Word Experiment (the reason is described in “Experimental procedure”). presents the information about the selected 36 speakers regarding dysarthria type, etiology, the severity level of dysarthria, PhonI scores, which was extracted from the COPAS dataset1, and whether a speaker was involved in the Word Experiment. We set the severity levels of dysarthria (SevL) at four levels (non-mild-moderate-severe). shows the distribution of the speakers over the four different levels of SevL.
Table 1. Detailed information for 36 speakers including Speaker ID, gender, age, etiology, dysarthria type, PhonI score (%), severity level of dysarthria (SevL; non-mild-moderate-severe), and whether the speakers were selected for the Word Experiment.
Figure 1. Distribution of speakers over four severity levels of dysarthria (SevL) in our two experiments.
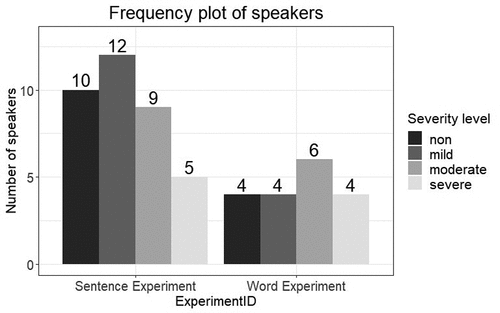
Both the SevL and PhonI had been assigned by experienced speech-language pathologists at the time the COPAS database was compiled. PhonI, calculated as the percentage of correctly transcribed target phonemes over all three word lists (each is a variant of a subset) for each speaker, is a highly reliable measure, with an inter-rater correlation of 0.91 and an intra-rater correlation of 0.93 using ICC (De Bodt et al., Citation2006; Middag et al., Citation2009a; Van Nuffelen et al., Citation2008). SevL has also been used in many international publications (e.g., Middag, Citation2012; Van Nuffelen et al., Citation2009; Yilmaz et al., Citation2016) as a basis for selecting speech recordings for experiments. Therefore, both of them can be used for selecting speakers and for evaluating our measures in the current study (see details in “Data analysis”).
Expert raters
As mentioned earlier, measures collected from inexperienced listeners tend to show larger variation than those collected from well-trained expert raters (Mencke et al., Citation1983). We selected speech-language therapists as raters rather than naïve listeners. A previous study (Van Nuffelen et al., Citation2010) reported reliable measures with three experts. To ensure reliability, we recruited five Belgian Dutch-speaking speech-language pathologists (one male and four females) from the University Antwerp Hospital. They were working at the ear, nose and throat (ENT) revalidation center for communication disorders, and were all familiar with evaluating and testing dysarthric patients through the intelligibility tasks used in our two experiments.
Experimental procedure
All recordings included in our listening experiments were made in a quiet clinical setting without a sound-attenuated box, as described in the COPAS manual. Originally, two microphones were used, one on the table with a mouth-microphone distance of about 30 cm, and one headset. Recordings of the selected speakers were made through the microphone on the table, with the exception of one speaker (in the Sentence Experiment only), for whom the used microphone was not known. We evaluated this speaker’s recordings and found the sound quality to be similar to those of the other recordings.
Both experiments were set up through the online survey tool Qualtrics and were conducted on the same day, the Sentence Experiment in the morning and the Word Experiment in the afternoon, with two resting hours in between. Before starting, the raters received consent forms and descriptions of both experiments on the Qualtrics website. They gave their explicit consent by clicking on the ‘agree’ button. In each of the two experiments, they first received instructions in Belgian Dutch. Following this, they received three and two practice examples to familiarize themselves with the procedure in the Sentence Experiment and the Word Experiment, respectively. For each experiment, the same two anchor items selected from healthy and severely dysarthric speakers in the COPAS dataset were repeated after every ten utterances in a pop-up format to remind the raters of what high and low intelligibility sound like. We ensured the recordings used as examples and as anchor items were not from the speakers involved in the two actual experiments. Moreover, the utterance samples (recordings) were randomized to prevent any systematic order effect. We prevented every six consecutive samples from being selected from the same speakers and every two consecutive samples being about the same sentences or subsets. All the raters received the same randomized order of samples.
Specifically, for the Sentence Experiment, the raters assessed each of the 144 utterances (recordings), consisting of the same set of four sentences read by 36 speakers, by performing two kinds of tasks, i.e. making orthographic transcriptions and filling in a VAS scale ranging from 0 (not intelligible) to 100 (intelligible). In detail, the VAS contained tick marks with numbers for every ten scores shown (e.g., 10, 20, 30, etc), no scale endpoints’ labels, and was oriented horizontally with written instructions ‘Wat voor score zou u toekennen aan de spraakverstaanbaarheid?’ (in English ‘what score would you assign for speech intelligibility?’). Regarding the orthographic transcription task, two forms of transcriptions, i.e. EWTrans and AWTrans, were made by the raters. The new form of transcription, AWTrans, can provide the raters with more flexibility in their transcriptions, thus helping report speech deviations at segmental level. In addition, each utterance together with both tasks was presented on the same page in the order of AWTrans, VAS and EWTrans, as illustrated in . To prevent the raters from adapting to the speakers and the speech materials, the listening time of each utterance was limited. They were allowed to listen to each utterance twice since they had to complete two forms of transcriptions. Further, it was up to the raters to decide which form of transcription to complete first and when (after or between completing the two forms of transcriptions) to assign a score on VAS. The total time required for completing the Sentence Experiment was around one hour, and the raters were encouraged to take a break after half an hour to prevent them from being fatigued.
For the Word Experiment, the raters assessed each of 54 utterances (recordings), consisting of three word lists (three variants of the three DIA subsets) read by 18 speakers, by making AWTrans and filling in a VAS scale. The three word lists (variants) of the subsets for each speaker in the experiment were presented in three recordings, in each of which three seconds of silence had been inserted manually between successive words to ensure that the raters had enough time to transcribe each word. Unlike the original DIA procedure (Middag et al., Citation2009b), in which the listeners were asked to transcribe the missing target phonemes while the remaining phonemes of a word were presented (e.g., transcribing target phoneme ‘n’ in ‘nit’, which was presented as ‘.it’), the raters in our experiment had to transcribe the whole words in the word lists without any phonemes being presented. The three utterances were assessed separately for each speaker. Moreover, only one form of transcription, i.e. AWTrans, was applied. This was because pseudowords were contained in the word lists, so it was not reasonable to transcribe using only existing words, as in EWTrans. Accordingly, all the raters were allowed to listen only once to each utterance to complete the AWTrans first and then assign a score on VAS. Also, considering that the required time for completing the two tasks for each utterance was much longer than that of the Sentence Experiment, and to ensure that this experiment could also be completed within one hour, only half of the speakers of the Sentence Experiment were involved in the Word Experiment.
Intelligibility measures
For each utterance, we obtained scores from the VAS and the orthographic transcription tasks. For the orthographic transcriptions, we calculated the Accuracy of Words (AcW) as followsFootnote2
where denotes the total number of words in the reference transcriptions,
denotes the number of matched words between the orthographic and the reference transcriptions, and
denotes the number of insertions in the orthographic transcriptions. Note that we removed punctuation and symbols indicating missing words to obtain cleaned transcriptions for the calculation. We also removed pseudowords in EWTrans before calculating AcW. In addition, no errors, such as misspellings, homophones or incorrect tense markers, were permitted. This was because the raters recruited in the present study were experienced, well-trained experts, and thus, we believed that they transcribed what they thought they had heard.
Data analysis
To address the first two research questions regarding the interrater reliability of the VAS and AcW scores, we applied G Theory by using the gtheory package (Moore, Citation2016) in RStudio (RStudio Team, Citation2020) with R version 4.0.1 (R Core Team, Citation2014). The advantage of applying G Theory is that all sources of variance relevant in the experiments can be dealt with simultaneously, e.g., raters, speakers, and utterances. In G Theory, the reliability coefficient is defined as the proportion of score variance attributable to the different sources in relation to the total variance. This model of analysis produces two reliability coefficients, i.e. the Generalizability coefficient (G-coefficient) and the Phi coefficient (D-coefficient). A G-coefficient should be calculated when one is interested in making decisions about an individual’s performance relative to that of his or her peers. The more demanding or strict D-coefficient should be calculated when one is interested in an individual’s performance irrespective of that of his or her peers and is therefore most likely to be used when making criterion-referenced screening or progress monitoring decisions. We chose the D-coefficient as reliability coefficient and to evaluate the number of raters and utterances needed to achieve an acceptable reliability level. The model designs for the two experiments were different because of the utterance samples. In the Sentence Experiment, the model was fully crossed since all five raters assessed all four utterances from all 36 speakers: Rater×Utterance×Speaker. However, in the Word Experiment, each speaker received a random variant of each subset A, B and C, resulting in three utterances per speaker. We considered the subsets to be replications of sets of CVC words. The actual word list (Utterance) was nested under Speaker. Such a design can be summarized as: (Utterance:Speaker)×Rater,Footnote3 meaning that all combinations of subsets, i.e. utterances and speakers were rated by all raters. In addition, Utterance, Rater, and Speaker were defined as random factors in both experiments since they are all potential samples from their universe. Also, to gain more insight into the reliability of AcW and VAS in the Sentence Experiment, we computed the reliability of the measures on the four utterances separately. Since each utterance was rated by all raters, giving two sources of variance, ICC could be used as a reliability measure. We applied the ICC by using the R psych package (Revelle, Citation2019).
Moreover, G Theory allows calculating the consequences of modifying the size of a factor, such as the number of raters, in measuring reliability. Consequently, it is possible to calculate the minimum size of a factor required to obtain reliable data (Li et al., Citation2015; Shavelson & Webb, Citation2006). By taking this strength of G Theory, we were able to address the second research question regarding the optimal numbers of raters and utterances per speaker. Specifically, following the common practice (Brennan, Citation2001; Briesch et al., Citation2014; Hollo et al., Citation2020; O’Brian et al., Citation2003; Webb et al., Citation2006), we first calculated sources of measurement error based on the collected data and then used these to estimate the reliability (D-coefficient) for different numbers of raters and utterances per speaker. According to the estimations, we can infer the minimum number of raters and the minimum number of utterances per speaker required for a reliable measure. A detailed explanation of G Theory can be found in Brennan (Citation2001); Briesch et al. (Citation2014) is a practical guide in implementing the analyses.
To address the third research question regarding validity, we investigated construct validity and concurrent validity for VAS and AcW, averaging the scores of each speaker. Construct validity investigates whether the test measures the concept it intends to measure and was analysed through Pearson correlations between VAS and AcW within each experiment and between experiments for each measure (VAS/AcW). Concurrent validity is a type of criterion validity and measures how well a test compares to other criteria. We correlated our measures to the two external measures that were available: SevL and PhonI. We applied multinomial regression to investigate the correlations of SevL, as this variable defines four severity groups, without the claim of being a continuous variable. Then based on the predicted labels (severity levels of dysarthria) generated by multinomial regression analysis, we calculated the percentage of speakers correctly classified in these four groups. To interpret the validity results, we used the guidelines from Evans (Citation1996, p. 146): a correlation between 0.80 and 1.0 is ‘very strong’, between 0.60 and 0.79 is ‘strong’, between 0.40 and 0.59 is ‘moderate’, between 0.20 and 0.39 is ‘weak’, and that even lower is ‘very weak’. We used the stats (R Core Team, Citation2014), nnet (Venables & Ripley, Citation2002), and DescTools (Signorell et al., Citation2021) packages in R version 4.0.1 for the implementation and ggplot2 package (Wickham, Citation2016) for making plots.
Results
General results of the intelligibility measures
Means and standard deviations of the VAS and AcW scores in both experiments are shown in . For the Sentence Experiment, higher mean values were observed for EWTrans than for AWTrans. Compared with the Sentence Experiment, the Word Experiment showed lower scores, especially on AcW. This might be due to the absence of contextual cues in the word lists compared to the meaningful sentences.
Table 2. Means (standard deviations) for the two types of intelligibility measures, VAS and AcW, in our two experiments. The results for AcW in the Sentence Experiment are denoted by All-Word Transcription (AWTrans) on the left and Existing-Word Transcription (EWTrans) on the right.
Interrater reliability of the intelligibility measures
We computed the interrater reliability of the VAS and AcW scores based on the D-coefficient. shows that the reliability values were high (above 0.90) for VAS in both experiments and for AcW in the Word Experiment. The values for AcW were slightly lower in the Sentence Experiment with the one of EWTrans being the lowest.
Table 3. Interrater reliability of the intelligibility measures in our two experiments based on the D-coefficient. The results for AcW in the sentence experiment are denoted by All-Word Transcription (AWTrans) on the left and Existing-Word Transcription (EWTrans) on the right.
Interrater reliability of the intelligibility measures per utterance in the sentence experiment
As shown in , low reliability values were observed for the PM2 sentence, which is ‘Marloes kijkt naar links’, especially for EWTrans. After analysing the transcriptions, we noticed four problems with this sentence. Firstly, the first word in this sentence is not a very common proper name. This name could be modified in various ways which caused the increase in the number of incorrectly transcribed words. Secondly, the second and third words are ‘kijkt’ and ‘naar’. Dutch native speakers realize only one release burst in the cluster ‘kt’ and even no release at all when the nasal ‘n’ follows. This reduces the distinction with ‘keek naar’ (past tense), taking into account the regional variation in pronouncing diphthongs and tensed vowels in Dutch (cf. Adank et al., Citation2007). Thirdly, the third word is ‘naar’. Many Dutch native speakers do not distinguish spatial ‘naar’ (i.e. ‘to’ as in English) and temporal ‘na’ (i.e. ‘after’ as in English) in their spontaneous speech. Finally, the fourth word is ‘links’, which is often pronounced without a plosive burst related to the ‘k’ (‘lings’ in AWTrans). The cumulation of four pronunciation variants made the raters’ transcriptions of this sentence less reliable than the other three, particularly in EWTrans. This should be avoided when constructing sentences to calculate the accuracy of transcribed words for intelligibility measures.
Table 4. Interrater reliability of the intelligibility measures in the Sentence Experiment based on the Intraclass Correlation Coefficient for the four sentences, i.e. PM1, PM2, PM3 and PM4. The results for AcW in the Sentence Experiment are denoted by All-Word Transcription (AWTrans) on the left and Existing-Word Transcription (EWTrans) on the right.
Reliability as a function of the number of raters and the number of utterance samples
shows that in both experiments, the D-coefficient increased when the number of raters or the number of utterance samples increased. When the number of utterance samples was fixed, the reliability increased rapidly at first and then this increase began to plateau. As suggested by Wells and Wollack (Citation2003), professionally developed high-stake tests should have a reliability of at least 0.90. We can observe that VAS reached this reliability level with three raters and three samples in the Sentence Experiment, and with four raters and three samples in the Word Experiment. The results for VAS were comparable, but those for AcW were not. Specifically, for AcW in the Sentence Experiment, the scores using AWTrans reached the reliability level with seven raters and four utterances, but those using EWTrans were the lowest and remained below this level for all cases of raters and utterances. This might also be due to the problematic sentence PM2. For AcW in the Word Experiment, the reliability level can be reached with only two raters and two utterances.
Construct validity
shows that all correlations between VAS and AcW in the same experiment were very strong (above 0.94). In contrast, the correlations of VAS and AcW between the two experiments were slightly weaker, but were still strong, with 0.88 for VAS and 0.81 for AcW.
Table 5. Pearson correlations between VAS and AcW in the two experiments, with two forms of transcriptions in the Sentence Experiment.
Concurrent validity
We investigated the concurrent validity of our measures with two external measures, i.e. SevL and PhonI. We first computed the correlations between these two external measures. Specifically, the multinomial regression with SevL as criterion and PhonI as predictor gave a Nagelkerke R2 (as the correlation) of 0.296 in the Sentence Experiment and 0.299 in the Word Experiment. The percentages of correctly classified speakers in the four levels of SevL were 44.4% in the Sentence Experiment and 50.0% in the Word Experiment. These outcomes suggest that SevL and PhonI reflect different constructs of intelligibility.
The correlations between PhonI and VAS/AcW were strong, as shown in . The correlations of AcW using AWTrans were slightly stronger than those of VAS for both experiments. shows five scattergrams, including the regression lines and their confidence intervals (95%) with distinguished SevL levels of the speakers. In the Sentence Experiment, the points were concentrated at the top-right corner, but in the Word Experiment, they were scattered across the scale. This might be due to the differences in speech material. These scattergrams also show that intelligibility measured at the phoneme level, i.e. PhonI, was different from intelligibility at higher levels, i.e. VAS at the utterance level and AcW at the word level.
Table 6. Pearson correlations between the external measure PhonI and our two intelligibility measures, i.e. VAS and AcW. The results for AcW in the Sentence Experiment are denoted by All-Word Transcription (AWTrans) on the left and Existing-Word Transcription (EWTrans) on the right.
Figure 4. Scattergrams, including the regression lines and their confidence intervals (95%) with distinguished SevL of the speakers, between the external measure PhonI and our two intelligibility measures, VAS and AcW (with two forms of transcriptions).
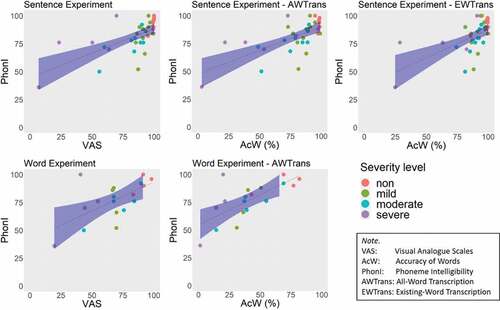
shows the Nagelkerke R2s and the percentage of correctly classified speakers by using multinomial regression with SevL as criterion and VAS/AcW as predictor. The results showed that most of the time VAS was better than AcW. Compared with EWTrans, AWTrans provided better results for AcW.
Table 7. Correlation (Nagelkerke R2s) and SevL classification (percentage of correctly classified speakers) for the external measure SevL as the criterion and one of our two intelligibility measures, VAS and AcW, as a predictor. The results for AcW in the Sentence Experiment are denoted by All-Word Transcription (AWTrans) on the left and Existing-Word Transcription (EWTrans) on the right.
shows that VAS and AcW overlapped between levels of SevL, but the tendency was that the more severe levels corresponded to lower VAS/AcW scores, whereas the less severe levels corresponded to higher VAS/AcW scores. We can also see, in line with the results in , that VAS better discriminated the levels of SevL than AcW does, with AWTrans being better than EWTrans.
Discussion
In this study, we investigated the interrater reliability and validity of two types of speech intelligibility measures, one rating-based measure through Visual Analogue Scales (VAS) and one transcription-based measure, i.e. Accuracy of Words (AcW), through orthographic transcriptions, by conducting two listening experiments, one targeting meaningful sentences (Sentence Experiment) and one targeting word lists (Word Experiment). For AcW in the Sentence Experiment, we studied two forms of transcriptions, i.e. Existing-Word Transcription (EWTrans) allowing meaningful, existing words, and All-Word Transcription (AWTrans) allowing all sorts of words, including both existing words and pseudowords. The mean values of VAS and AcW were generally higher for the meaningful sentences than for the word lists with comparable standard deviations. This is in line with previous findings (Dos Barreto & Ortiz, Citation2008; Hustad, Citation2007; Miller, Citation2013; Yorkston & Beukelman, Citation1978, Citation1981) that intelligibility measures result in higher values for meaningful sentences than for word lists due to the presence of contextual information. However, this difference was not found for severe dysarthria in our VAS scores. In addition, we observed higher mean values for VAS than for AcW in AWTrans. This seems to be in conflict with the finding in Stipancic et al. (Citation2016), which showed that the percent correct scores obtained from orthographic transcriptions were higher than the VAS scores. However, the same result can actually be observed in our study when AcW was derived from EWTrans, as was done in Stipancic et al. (Citation2016). This suggests that EWTrans and AWTrans can provide different results and thus, raters should be clearly instructed when using one or the other form. In the remainder of this section, we firstly discuss the results of the present study in relation to the specific research questions we addressed. Following this, we describe the limitations of the present study. After that, we stress our recommendations in designing listening experiments and the focus of future work.
RQ1: to what extent are intelligibility measures reliable?
For VAS, very high interrater reliability values (above 0.90) were observed for both speech materials. However, for AcW, the reliability was higher for the word lists (0.95) than for the meaningful sentences (below 0.90), especially when using EWTrans (0.83). The relatively lower reliability for EWTrans may be explained by the fact that one of the specific sentences we used (PM2) contained an uncommon name and an unintended cumulation of pronunciation variability. These problems should be avoided when selecting sentences to calculate accuracy of words for intelligibility measures through orthographic transcriptions. In general, the results suggest that VAS is more reliable than AcW. This finding is consistent with results of previous studies (Ganzeboom et al., Citation2016; Stipancic et al., Citation2016; Tjaden et al., Citation2014), in which high interrater reliability values (above 0.90) were also reported for VAS. Reliability was also high for AcW, which is in line with the broad consensus that transcription yields good interrater reliability (Bunton et al., Citation2001; Miller, Citation2013; Tjaden et al., Citation2014; Tjaden & Wilding, Citation2010).
Note that we did not measure intra-rater reliability due to several reasons. Firstly, intra-rater reliability has the disadvantage of requiring repeated measurements. Secondly, it does not generalize to a measure representing a group of raters, e.g., experts or human listeners overall. Moreover, high intra-rater reliability does not imply high inter-rater reliability.
RQ2: how many raters and utterance samples per speaker are needed to obtain reliable intelligibility measures?
The interrater reliability analyses showed that the number of raters and utterance samples per speaker was positively related to the reliability of VAS and AcW. For VAS, regardless of speech materials, at least three samples per speaker in combination with four raters were needed to obtain reliable results, i.e. passing the criterion of 0.90 for professionally developed high-stake tests (Wells & Wollack, Citation2003). However, for AcW, different materials yielded different results. Specifically, for the word lists, at least two samples per speaker in combination with two raters were needed, while for the meaningful sentences many more raters with all the samples involved were needed. In detail, at least seven raters were needed when using AWTrans, while more than ten raters were needed for EWTrans. In this case, if all individual utterances meet the criteria of a good test item, four raters in combination with four utterances may be also sufficient. Note that our study used expert raters. Recruiting naïve listeners as raters may lead to different results and the number of raters needed for high reliability may be much larger than four (Ganzeboom et al., Citation2016).
RQ3: to what extent are the intelligibility measures valid?
Regarding construct validity, very strong correlations were found between VAS and AcW within the same speech material. This is in line with the finding by Abur et al. (Citation2019), who also found strong, positive relationships (0.886; p < .001) between scores derived from the orthographic transcription and VAS tasks. This suggests that for the same material, VAS and AcW are related to the same construct of intelligibility, even when different transcription forms are used for AcW. These results indicate that both VAS and AcW are valid to a great extent when they are collected for the same material. The correlations of the same measure, i.e. VAS or AcW, between different speech materials were below 0.90, indicating perhaps that different constructs of intelligibility are measured in different speech materials. Specifically, the correlations were 0.88 for VAS and 0.81 for AcW. This suggests that VAS and AcW remain valid to a substantial extent across materials and that VAS might be a more stable or robust indicator of intelligibility.
Regarding concurrent validity, the weak correlations between the two external measures, i.e. severity level of dysarthria (SevL) and phoneme intelligibility (PhonI), indicate that they reflect different constructs of intelligibility. VAS was much more strongly correlated to SevL than to PhonI, and showed better discriminations of the levels of SevL than AcW. Differently, the correlations between AcW and the two external measures (SevL/PhonI) were comparably strong. These results seem to suggest that AcW is related to a construct of intelligibility that is similar to those of SevL/PhonI. For both VAS and AcW, we found that more than half of the speakers were classified in the correct levels of SevL. These results applied to both sentences and word lists.
Limitations
The present study investigated the interrater reliability and validity of VAS and AcW on two types of speech materials, i.e. meaningful sentences from a narrative and word lists containing pseudowords. The meaningful sentences were employed to obtain more ecologically valid intelligibility scores since these sentences are closer to those used in daily conversation in comparison to the word lists. Previous studies criticized these measures arguing that listeners could rely on more contextual information to understand the message in sentences than in words (Dongilli, Citation1994; Hustad, Citation2007; Yorkston & Beukelman, Citation1978, Citation1981). Thus, to limit the contextual information in sentences, some studies (e.g., Ellis & Fucci, Citation1991; Ganzeboom et al., Citation2016) have used Semantically Unpredictable Sentences (SUS) in an attempt to avoid listeners ‘guessing’ of the content. Therefore, the absence of SUS in the present study could be seen as one of the limitations. However, using contextual information to understand a message is what listeners actually do in normal life. Thus, our employment of speech material with contextual information may be very useful to understand how patients would fare under more realistic conditions. Another limitation might be that only expert raters were involved in our study and thus, we cannot compare their performance to that of naïve listeners as reported in many studies (Abur et al., Citation2019; Ganzeboom et al., Citation2016; Ishikawa et al., Citation2021; Stipancic et al., Citation2016; Sussman & Tjaden, Citation2012). Moreover, we did not permit any errors in the transcriptions, including misspellings and homophones, based on the assumption that the raters transcribed what they thought they had heard as they were well-trained. However, such an assumption may be too ideal and the subsequent processing of transcriptions may be rigorous because in practice people can make such errors in transcriptions. Therefore, although the measures showed very high reliability values, more research is necessary to refine and further elaborate our novel findings.
In addition, some settings of the experiments in our study were different from those used in cited studies, and thus, might influence the results. For example, the VAS in the current study was presented with an anchor every 10 points, which differs from the typical presentations, with only a beginning (0) and end (100 or 1) anchor (Abur et al., Citation2019; Ganzeboom et al., Citation2016; Stipancic et al., Citation2016). Another example is the different diagnoses and severity levels of dysarthria of speakers involved in the intelligibility assessment. Also, no errors were permitted in calculating AcW since the raters in the present study were experienced, well-trained experts. This is stricter than studies in which naïve listeners were recruited as raters (Ishikawa et al., Citation2018; Stipancic et al., Citation2016).
Furthermore, as we used the existing dataset to design the experiments, our setting options were limited by the restrictions of this dataset. For instance, the number of raters for SevL and PhonI measures in the dataset was not clear although the reliability of these measures seems to be sufficient for selecting speakers and evaluating our intelligibility measures. Also, for some of the selected speakers, the etiology of dysarthria was indicated, but not the dysarthria type. Another example is that to collect the measures in meaningful sentences, we were only able to use the exact same four sentences for all the speakers. Although we randomized the sentences and speakers to avoid raters’ adaptation to them, repeating the sentences may still impact the results. Thus, future studies may use comparable but different sentences for different speakers rather than the same sentences to further elaborate our findings.
Recommendations
The results presented in this study show that VAS is as reliable and valid as AcW. This indicates that VAS could be a good alternative for research and clinical practice, as also suggested by Ishikawa et al. (Citation2021), especially if we consider that VAS also appears to be more robust due to the small difference in reliability between different speech materials. However, the rating task might not provide enough information for in-depth diagnosis and detailed analysis in research and clinical practice. In turn, this suggests that deciding which measure to apply depends directly on the specific goals of research and clinical practice.
The analyses of the two forms of transcriptions suggest that AWTrans seems to provide more reliable and valid AcW scores than EWTrans, anyway in the case that the raters are familiar with the speech materials. This shows that the contextual information in the meaningful sentences might be limited to a certain extent by using AWTrans. Notice that raters should be clearly instructed when using AWTrans.
The analyses of each utterance in the Sentence Experiment indicate, as we have already discussed above, that an uncommon name and an unintended cumulation of pronunciation variability should be avoided when selecting sentences to calculate AcW for intelligibility through orthographic transcription tasks.
Last but not least, it is important that we were able to systematically handle the issue of reliability in terms of raters and utterances by using Generalizability Theory. In this way, the optimal number of utterance samples per speaker and raters can be determined. This is very helpful for researchers and clinicians in designing listening experiments on speech intelligibility. Having four expert raters in combination with three samples per speaker is sufficient for obtaining reliable VAS scores regardless of speech materials. Having two expert raters in combination with two samples per speaker is sufficient for obtaining reliable AcW scores on the word lists, while many more raters and samples are required for meaningful sentences.
Future work
The finding that EWTrans and AWTrans, as the two forms of transcriptions, led to different reliability measures is a good reason for further investigation at a more fine-grained granularity level, i.e. subword level, also because many studies (De Bodt et al., Citation2006; Hustad, Citation2006; Kent et al., Citation1989; Xue et al., Citation2020) focusing on the subword level have shown its potential value for both research and clinical practice. In addition, since it was required to prevent the raters from being fatigued, we evaluated fewer speakers in one experiment than the other. Future work can address such restrictions by using more complex designs. For example, speakers can be split into multiple groups, and each of the groups can be evaluated by a different group of raters (Hubers et al., Citation2019). This takes advantage of one of the strengths of G Theory to handle diverse designs including both crossed and nested factors.
Conclusions
The present study investigated the interrater reliability and validity of two types of speech intelligibility measures, one rating-based measure, VAS, and one transcription-based measure, AcW, for two different speech materials. With five expert raters, VAS is as reliable and valid as the commonly-used AcW regardless of speech materials and forms of transcriptions.
Our reliability analysis of intelligibility measures by five expert raters on speech from speakers with different severity levels of dysarthria with respect to two different speech materials leads us to recommend that future studies on intelligibility measures use the D-coefficient, which is part of Generalizability Theory, as a measure of reliability. The D-coefficient can be used for all kinds of experimental designs, and it is allowed to be generalized across raters and/or samples. This metric also allows assessing the minimum numbers of raters and samples per speaker that are required to obtain reliable data.
Acknowledgments
We would like to thank the raters who participated in our experiments and our colleague Viviana Mendoza Ramos of the Department of Otorhinolaryngology, Head and Neck Surgery and Communication Disorders of the University Hospital of Antwerp for helping in conducting the experiments during the internship of the first author at the University Hospital of Antwerp. We are particularly grateful to two anonymous reviewers for their constructive comments which led to considerable improvements in the manuscript. The authors report no conflict of interest.
Disclosure statement
No potential conflict of interest was reported by the author(s).
Additional information
Funding
Notes
1 More information and the manual of COPAS can be found on https://taalmaterialen.ivdnt.org/download/tstc-corpus-pathologische-en-normale-spraak-copas/.
2 We used the asr-evaluation python module provided in https://github.com/belambert/asr-evaluation. The calculation was done fully automatically.:
3 We also explored the model including the subsets of DIA as a fourth factor which led to the model design being (Utterance:(Speaker×Subset))×Rater. However, we did not find a main effect of Subset on the reliability of the measures. Therefore, we simplified the model design as it is now in the current study, which is (Utterance:Speaker)×Rater.
References
- Abur, D., Enos, N. M., & Stepp, C. E. (2019). Visual analog scale ratings and orthographic transcription measures of sentence intelligibility in Parkinson’s disease with variable listener exposure. American Journal of Speech-Language Pathology, 28(3), 1222–1232. https://doi.org/10.1044/2019_AJSLP-18-0275
- Adank, P., Van Hout, R., & Van de Velde, H. (2007). An acoustic description of the vowels of Northern and Southern Standard Dutch II: Regional varieties. Journal of the Acoustical Society of America, 121(2), 1130–1141. https://doi.org/10.1121/1.2409492
- Beijer, L. J., Clapham, R. P., & Rietveld, A. C. M. (2012). Evaluating the suitability of orthographic transcription and intelligibility scale rating of semantically unpredictable sentences (SUS) for speech training efficacy research in dysarthric speakers with Parkinson’s disease. Journal of Medical Speech-Language Pathology, 20(2), 17–35. https://doi.org/10.1089/tmj.2009.0183
- Brennan, R. L. (2001). Generalizability theory. Springer.
- Briesch, A. M., Swaminathan, H., Welsh, M., & Chafouleas, S. M. (2014). Generalizability theory: A practical guide to study design, implementation, and interpretation. Journal of School Psychology, 52(1), 13–35. https://doi.org/10.1016/j.jsp.2013.11.008
- Bunton, K., Kent, R. D., Kent, J. F., & Duffy, J. R. (2001). The effects of flattening fundamental frequency contours on sentence intelligibility in speakers with dysarthria. Clinical Linguistics & Phonetics, 15(3), 181–193. https://doi.org/10.1080/02699200010003378
- Cannito, M. P., Suiter, D. M., Beverly, D., Chorna, L., Wolf, T., & Pfeiffer, R. M. (2012). Sentence intelligibility before and after voice treatment in speakers with idiopathic Parkinson’s disease. Journal of Voice, 26(2), 214–219. https://doi.org/10.1016/j.jvoice.2011.08.014
- Carvalho, J., Cardoso, R., Guimarães, I., & Ferreira, J. J. (2021). Speech intelligibility of Parkinson’s disease patients evaluated by different groups of healthcare professionals and naïve listeners. Logopedics Phoniatrics Vocology, 46(3), 1–7. https://doi.org/10.1080/14015439.2020.1785546
- De Bodt, M., Guns, C., & Van Nuffelen, G. (2006). NSVO: Nederlandstalig Spraakverstaanbaarheidsonderzoek. Vlaamse Vereniging voor Logopedisten.
- Dongilli, P. (1994). Semantic context and speech intelligibility. In J. Till, K. Yorkston, & D. Beukelman (Eds.), Motor speech disorders: Advances in assessment and treatment (pp. 175–191). Paul H. Brookes.
- Dos Barreto, S., & Ortiz, K. (2008). Intelligibility measurements in speech disorders: A critical review of the literature. Pró-Fono Revista de Atualização Científica, 20(3), 201–206. https://doi.org/10.1590/s0104-56872008000300011
- Dos Barreto, S., & Ortiz, K. (2016). Protocol for the evaluation of speech intelligibility in dysarthrias: Evidence of reliability and validity. Folia Phoniatrica Et Logopaedica, 67(4), 212–218. https://doi.org/10.1159/000441929
- Ellis, L. W., & Fucci, D. J. (1991). Magnitude-estimation scaling of speech intelligibility: Effects of listeners’ experience and semantic-syntactic context. Perceptual and Motor Skills, 73(1), 295–305. https://doi.org/10.2466/pms.1991.73.1.295
- Evans, J. D. (1996). Straightforward statistics for the behavioral sciences. Brooks/Cole Publishing.
- Fisher, R. A. (1992). Statistical methods for research workers. In S. Kotz & N. L. Johnson (Eds.), Breakthroughs in statistics (pp. 66–70). Springer.
- Ford, A. L., & Johnson, L. D. (2021). The use of generalizability theory to inform sampling of educator language used with preschoolers with Autism Spectrum Disorder. Journal of Speech, Language, and Hearing Research, 64(5), 1748–1757. https://doi.org/10.1044/2021_JSLHR-20-00586
- Ganzeboom, M., Bakker, M., Cucchiarini, C., & Strik, H. (2016). Intelligibility of disordered speech: Global and detailed scores. In Proceedings of Interspeech 2016 (pp. 2503–2507). San Francisco, USA.
- Hodge, M. M., & Gotzke, C. L. (2014). Construct-related validity of the TOCS measures: Comparison of intelligibility and speaking rate scores in children with and without speech disorders. Journal of Communication Disorders, 51, 51–63. https://doi.org/10.1016/j.jcomdis.2014.06.007
- Hollo, A., Staubitz, J. L., & Chow, J. C. (2020). Applying generalizability theory to optimize analysis of spontaneous teacher talk in elementary classrooms. Journal of Speech, Language, and Hearing Research, 63(6), 1947–1957. https://doi.org/10.1044/2020_JSLHR-19-00118
- Hubers, F., Cucchiarini, C., Strik, H., & Dijkstra, T. (2019). Normative data of Dutch idiomatic expressions: Subjective judgments you can bank on. Frontiers in Psychology, 10, 1075. https://doi.org/10.3389/fpsyg.2019.01075
- Hustad, K. C., & Cahill, M. A. (2003). Effects of presentation mode and repeated familiarization on intelligibility of dysarthric speech. American Journal of Speech-Language Pathology, 12(2), 198–208. https://doi.org/10.1044/1058-0360(2003/066)
- Hustad, K. C. (2006). Estimating the intelligibility of speakers with dysarthria. Folia Phoniatrica Et Logopaedica, 58(3), 217–228. https://doi.org/10.1159/000091735
- Hustad, K. C. (2007). Effects of speech stimuli and dysarthria severity on intelligibility scores and listener confidence ratings for speakers with Cerebral Palsy. Folia Phoniatrica Et Logopaedica, 59(6), 306–317. https://doi.org/10.1159/000108337
- Hustad, K. C. (2008). The relationship between listener comprehension and intelligibility scores for speakers with dysarthria. Journal of Speech Language and Hearing Research, 51(3), 562. https://doi.org/10.1044/1092-4388(2008/040)
- Ishikawa, K., de Alarcon, A., Khosla, S., Kelchner, L., Silbert, N., & Boyce, S. (2018). Predicting intelligibility deficit in dysphonic speech with cepstral peak prominence. Annals of Otology, Rhinology & Laryngology, 127(2), 69–78. https://doi.org/10.1177/0003489417743518
- Ishikawa, K., Webster, J., & Ketring, C. (2021). Agreement between transcription- and rating-based intelligibility measurements for evaluation of dysphonic speech in noise. Clinical Linguistics & Phonetics, 35(10) , 1–13. https://doi.org/10.1080/02699206.2020.1852602
- Kent, R. D., & Kim, Y. (2011). The assessment of intelligibility in motor speech disorders. In A. Lowit & R. D. Kent (Eds.), Assessment of motor speech disorders (pp. 21–37). Plural.
- Kent, R. D., Weismer, G., Kent, J. F., & Rosenbek, J. C. (1989). Toward phonetic intelligibility testing in dysarthria. Journal of Speech and Hearing Disorders, 54(4), 482. https://doi.org/10.1044/jshd.5404.482
- Levy, E. S., Moya-Galé, G., Chang, Y. H. M., Freeman, K., Forrest, K., Brin, M. F., & Ramig, L. A. (2020). The effects of intensive speech treatment on intelligibility in Parkinson’s disease: A randomised controlled trial. EClinicalMedicine, 24, Article 100429. https://doi.org/10.1016/j.eclinm.2020.100429
- Li, M., Shavelson, R. J., Yin, Y., Wiley, E., Li, M., & Shavelson, R. J. (2015). Generalizability theory. In R. L. Cautin, S. O. Lilienfeld, et al. (Eds.), The encyclopedia of clinical psychology (pp. 1–19). John Wiley & Sons. Inc.
- Liss, J. M., Spitzer, S. M., Caviness, J. N., & Adler, C. (2002). The effects of familiarization on intelligibility and lexical segmentation in hypokinetic and ataxic dysarthria. Journal of Acoustical Society of America, 112(6), 10. https://doi.org/10.1121/1.1515793
- Maruthy, S., & Raj, N. (2014). Relationship between speech intelligibility and listener effort in Malayalam-speaking individuals with hypokinetic dysarthria. Speech, Language and Hearing, 17(4), 237–245. https://doi.org/10.1179/2050571X14Z.00000000057
- Mencke, E. O., Ochsner, G. J., & Testut, E. W. (1983). Listener judges and the speech intelligibility of deaf children. Journal of Communication Disorders, 16(3), 175–180. https://doi.org/10.1016/0021-9924(83)90031-X
- Middag, C., Martens, J. P., Van Nuffelen, G., & De Bodt, M. (2009b). DIA: A tool for objective intelligibility assessment of pathological speech. In 6th International workshop on Models and Analysis of Vocal Emissions for Biomedical Applications (pp. 165–167).
- Middag, C., Martens, J., Van Nuffelen, G., & De Bodt, M. (2009a). Automated intelligibility assessment of pathological speech using phonological features. EURASIP Journal on Advances in Signal Processing 2009, 2009, Article 629030. https://doi.org/10.1155/2009/629030
- Middag, C. (2012). Automatic analysis of pathological speech. Ghent University, Department of Electronics and information systems.
- Miller, N. (2013). Measuring up to speech intelligibility. International Journal of Language & Communication Disorders, 48(6), 601–612. https://doi.org/10.1111/1460-6984.12061
- Monsen R. B. (1983). The Oral Speech Intelligibility of Hearing-Impaired Talkers. Journal of Speech & Hearing Disorders, 48(3), 286–296. https://doi.org/10.1044/jshd.4803.286
- Moore, C. T. (2016). gtheory: Apply generalizability theory with R. [Computer software]. Retrieved from https://CRAN.R-project.org/package=gtheory
- Nakayama, K., Yamamoto, T., Oda, C., Sato, M., Murakami, T., & Horiguchi, S. (2020). Effectiveness of Lee Silverman Voice Treatment® LOUD on Japanese-speaking patients with Parkinson’s disease. Rehabilitation research and practice, 2020, Article 6585264. https://doi.org/10.1155/2020/6585264
- O’Brian, S., Packman, A., Onslow, M., & O’Brian, N. (2003). Generalizability Theory II: Application to perceptual scaling of speech naturalness in adults who stutter. Journal of Speech, Language & Hearing Research, 46(3), 718–723. https://doi.org/10.1044/1092-4388(2003/057)
- R Core Team. (2014). R: A language and environment for statistical computing [Computer software]. http://www.R-project.org/
- Revelle, W. (2019) psych: Procedures for psychological, psychometric, and personality research [Computer software]. Northwestern University. https://CRAN.R-project.org/package=psych
- Rietveld, T. (2020). Human measurement techniques in speech and language pathology: Methods for research and clinical practice. Routledge.
- RStudio Team. (2020). RStudio: Integrated development for R [Computer software]. http://www.rstudio.com/
- Schiavetti, N. (1992). Scaling procedures for the measurement of speech intelligibility. In R. D. Kent (Ed.), Intelligibility in speech disorders: Theory, measurement and management (pp. 11–34). John Benjamins.
- Shavelson, R. J., & Webb, N. M. (2006). Generalizability theory. In J. L. Green, G. Camilli, & P. B. Elmore (Eds.), Handbook of complementary methods in education research (pp. 309–322). Lawrence Erlbaum Associates.
- Signorell, A., et al. (2021). DescTools: Tools for descriptive statistics (R package version 0.99.44) [Computer software]. https://cran.r-project.org/package=DescTools
- Stipancic, K. L., Tjaden, K., & Wilding, G. (2016). Comparison of intelligibility measures for adults with Parkinson’s disease, adults with multiple sclerosis, and healthy controls. Journal of Speech Language and Hearing Research, 59(2), 230. https://doi.org/10.1044/2015_JSLHR-S-15-0271
- Sussman, J. E., & Tjaden, K. (2012). Perceptual measures of speech from individuals with Parkinson’s disease and multiple sclerosis: Intelligibility and beyond. Journal of Speech, Language, and Hearing Research, 55(4), 1208–1219. https://doi.org/10.1044/1092-4388(2011/11-0048)
- Tjaden, K. K., & Liss, J. M. (1995a). The role of listener familiarity in the perception of dysarthric speech. Clinical Linguistics & Phonetics, 9(2), 139–154. https://doi.org/10.3109/02699209508985329
- Tjaden, K., Kain, A., & Lam, J. (2014). Hybridizing conversational and clear speech to investigate the source of increased intelligibility in speakers with Parkinson’s disease. Journal of Speech, Language, and Hearing Research, 57(4), 1191–1205. https://doi.org/10.1044/2014_JSLHR-S-13-0086
- Tjaden, K., & Liss, J. M. (1995b). The influence of familiarity on judgments of treated speech. American Journal of Speech-Language Pathology, 4(1), 39–48. https://doi.org/10.1044/1058-0360.0401.39
- Tjaden, K., & Wilding, G. (2010). Effects of speaking task on intelligibility in Parkinson’s disease. Clinical Linguistics & Phonetics, 25(2), 155–168. https://doi.org/10.3109/02699206.2010.520185
- Van de Weijer, J., & Slis, I. (1991). Nasaliteitsmeting met de Nasometer Logopedie en Foniatrie, 63, 97–101.
- Van Nuffelen, G., De Bodt, M., Guns, C., Wuyts, F., & Van de Heyning, P. (2008). Reliability and clinical relevance of segmental analysis based on intelligibility assessment. Folia Phoniatrica et Logopaedica, 60(5), 264–268. https://doi.org/10.1159/000153433
- Van Nuffelen, G., De Bodt, M., Vanderwegen, J., Van de Heyning, P., & Wuyts, F. (2010). Effect of rate control on speech production and intelligibility in dysarthria. Folia Phoniatrica Et Logopaedica, 62(3), 110–119. https://doi.org/10.1159/000287209
- Van Nuffelen, G., Middag, C., De Bodt, M., & Martens, J. P. (2009). Speech technology-based assessment of phoneme intelligibility in dysarthria. International Journal of Language & Communication Disorders, 44(5), 716–730. https://doi.org/10.1080/13682820802342062
- Venables, W. N., & Ripley, B. D. (2002). Modern applied statistics with S (4th ed.). Springer. https://www.stats.ox.ac.uk/pub/MASS4/
- Webb, N. M., Shavelson, R. J., & Haertel, E. H. (2006). Reliability coefficients and generalizability theory. Handbook of Statistics, 26, 81–124. https://doi.org/10.1016/S0169-7161(06)26004-8
- Wells, C. S., & Wollack, J. A. (2003). An instructor’s guide to understanding test reliability. Testing & Evaluation Services, University of Wisconsin.
- Wickham, H. (2016). ggplot2: Elegant graphics for data analysis. New York: Springer-Verlag. https://ggplot2.tidyverse.org
- Xue, W., Ramos, V. M., Harmsen, W., Cucchiarini, C., van Hout, R. W. N. M., & Strik, H. (2020). Towards a comprehensive assessment of speech intelligibility for pathological speech. In Proceedings of interspeech 2020 (pp. 3146–3150). Shanghai, China.
- Xue, W., van Hout, R., Boogmans, F., Ganzeboom, M., Cucchiarini, C., & Strik, H. (2021). Speech intelligibility of dysarthric speech: Human scores and acoustic-phonetic features. In Proceedings of interspeech 2021 (pp. 2911–2915). Brno, Czech Republic.
- Yilmaz, E., Ganzeboom, M. S., Cucchiarini, C., & Strik, H. (2016). Combining non-pathological data of different language varieties to improve DNN-HMM performance on pathological speech. In Proceedings of interspeech 2016 (pp. 218–222). San Francisco, USA.
- Yorkston, K. M., & Beukelman, D. R. (1978). A comparison of techniques for measuring intelligibility of dysarthric speech. Journal of Communication Disorders, 11(6), 499–512. https://doi.org/10.1016/0021-9924(78)90024-2
- Yorkston, K. M., & Beukelman, D. R. (1981). Communication efficiency of dysarthric speakers as measured by sentence intelligibility and speaking rate. Journal of Speech and Hearing Disorders, 46(3), 296–301. https://doi.org/10.1044/jshd.4603.296
- Yorkston, K., Beukelman, D., & Tice, R. (1996). Sentence intelligibility test [Computer software]. Tice Technologies.
- Yuan, F., Guo, X., Wei, X., Xie, F., Zheng, J., Huang, Y., Huang, Z., Chang, Z., Li, H., Guo, Y., Chen, J., Guo, J., Tang, B., Deng, B., & Wang, Q. (2020). Lee Silverman Voice Treatment for dysarthria in patients with Parkinson’s disease: A systematic review and meta-analysis. European Journal of Neurology, 27(10), 1957–1970. https://doi.org/10.1111/ene.14399