ABSTRACT
Speakers with motor speech disorders (MSD) present challenges in speech production, one of them being the difficulty to adapt their speech to different modes. However, it is unclear whether different types of MSD are similarly affected when it comes to adapting their speech to various communication contexts. This study investigates the encoding of speech modes in individuals with AoS following focal brain damage and in individuals with hypokinetic dysarthria (HD) secondary to Parkinson’s disease. Participants with mild-to-moderate MSD and their age-matched controls performed a delayed production task of pseudo-words in two speech modes: normal and whispered speech. While overall accuracy did not differ significantly across speech modes, participants with AoS exhibited longer response latencies for whispered speech, reflecting difficulties in the initiation of utterances requiring an unvoiced production. In contrast, participants with HD showed faster response latencies for whispered speech, indicating that this speech mode is easier to encode/control for this population. Acoustic durations followed these same trends, with participants with AoS showing greater lengthening for whispered speech as compared to controls and to participants with HD, while participants with HD exhibited milder lengthening. Contrary to the predictions of speech production models, suggesting that speech mode changes might be particularly difficult in dysarthria, the present results suggest that speech mode adaptation rather seems particularly costly for participants with AoS.
Introduction
Speech production is a complex sensory-motor skill that requires coordination of respiratory, phonatory and resonance systems for precise, fast, and adaptable communication. Indeed, the speech environment is never completely noise-free and we sometimes need to speak more or less loudly, clearly, or quickly to convey a message, constantly adapting the speaking mode. These speech adaptations are generally called ‘speech modulations’, ‘speech modes’ or even ‘uttering conditions’, all used here as synonyms. It is widely recognised that modulating speech can be particularly challenging in some motor speech disorders (MSD) and speech modes are often used by speech and language therapists (SLT) for their treatment (Palmer & Enderby, Citation2007). However, it is unknown how changes in speech modes are affected in different pathologies. In the present study, we focus on two prominent MSD: Apraxia of Speech (AoS) and dysarthria, both affecting speech production through distinct underlying mechanisms, as, detailed below. In the next sections, we will first examine the clinical features of these two MSD. Subsequently, we will explore previous studies on speech modes in MSD. Finally, we will describe speech motor control models, their incorporation of speech modes and how those last ones are expected to be impaired in different MSD.
Apraxia of speech (AoS) and dysarthria
Within MSD, AoS and dysarthria present both distinctive and common signs of impaired speech production. On one hand, AoS is characterised by laborious (articulatory efforts) and non-fluent speech, syllable segregation, variability over time, dysprosody, phonetic distortions and phonemic errors, and frequent false starts and restarts (Allison et al., Citation2020; Ziegler et al., Citation2012). A broad consensus in the literature indicates that AoS reflects impaired ability to retrieve and/or assemble the different elements of the phonetic plans (e.g. Blumstein, Citation1990; Varley & Whiteside, Citation2001). On the other hand, dysarthria affects speech in terms of the strength, speed, range, steadiness, tone, or accuracy of movements required for breathing, phonation, resonance, articulation, or prosody (Duffy, Citation2019). Disturbances in speech control or execution are due to sensorimotor abnormalities such as weakness, spasticity, lack of coordination, involuntary movements, or variable muscle tension. While AoS and dysarthria differ in their underlying neuropathology and characteristics, they also share some clinical signs depending on the type of dysarthria, including phonetic distortions, reduced speech rate and impaired prosody, with the degree of overlap varying according to severity and subtype of dysarthria. Consequently, distinguishing between AoS and dysarthria can be difficult both via perceptive (Pernon et al., Citation2022) and automatic/acoustic classifications (Fougeron et al., Citation2022). Additionally, only a few studies have compared directly AoS and dysarthria using the same methods of analysis for both groups. To the best of our knowledge, a direct comparison between AoS and dysarthria has only been reported on vowel formants in a small group (Melle & Gallego, Citation2012), on a DDK production task (Lancheros et al., Citation2023; Ziegler, Citation2002), on coarticulation (D’Alessandro et al., Citation2019), on rate modulation abilities (Utianski et al., Citation2023) and on automatic discrimination (Kodrasi et al., Citation2020). As AoS and dysarthria share both unique features and common traits, investigating speech modes within the context of MSD can provide valuable insights into their underlying mechanisms.
Speech modes in AoS and dysarthria
To our knowledge, speech modes have received minimal investigation in the AoS, probably due to the implicit assumption that their capacity to modulate speech remains unaffected (see the subsequent section on ‘speech modes in speech production models’). Only one study has focused on rate modulation (i.e. faster speech) in AoS and found that patients with prosodic AoS demonstrated a reduced ability to accelerate speech rate (Utianski et al., Citation2021). By contrast, speech modes have been investigated in dysarthria, often showing difficulties in modulating speech, especially in hypokinetic dysarthria (HD, Duffy, Citation2019; Tjaden & Wilding, Citation2004). For instance, patients with HD secondary to Parkinson’s disease (PD) have been shown to struggle with speaking loudly (Fox et al., Citation2012) or controlling speech rate (Skodda, Citation2011). Similarly, patients with ataxic dysarthria have been found to have difficulties in controlling vocal intensity and fundamental frequency (Enderby, Citation2013). Moreover, speech modes and in particular loud speech are often used for rehabilitation by speech and languages therapists for dysarthric speech (e.g. the Lee Silverman Voice Treatment, LSVT, Ramig et al., Citation1995). Dysarthria’s speech modes production difficulties not only highlight challenges in adapting speech but also suggest impairment of a specific motor speech encoding process. Despite its clinical significance, a comprehensive understanding of the precise encoding mechanisms that govern speech modes and their impact in dysarthria remains elusive.
Speech modes in speech production models
Among the different speech production models currently available (see Parrell et al., Citation2019 for a review), the Four-level Framework (FLF) proposed by Van der Merwe (Citation1997, Citation2021) and the neuro-network Laryngeal Directions Into Velocities of Articulators (LaDIVA) model (Weerathunge et al., Citation2022) are the primary models shedding light on the underlying processes of speech modes.
The FLF is based on MSD and specifically addresses and defines the encoding of speech modes. The fundamental premise of the FLF is that ‘motor speech planning’ and ‘motor speech programming’ are two differentiable pre-execution processes. In this model, following linguistic (phonological) encoding, the motor speech planning processing stage encodes the place and mode of articulation for each phoneme/segment, and inter- and co-articulatory control of the segmental features of speech. This processing stage is thought to be impaired in AoS. During motor speech programming, the muscle tone, movement direction, velocity, force, range, and mechanical stiffness of the articulatory, laryngeal, and respiratory muscles are defined and parametrised (Brooks, Citation1986; Schultz & Romo, Citation1992). The specification of spatiotemporal parameters, force, and metrical structure can adapt to various circumstances, such as emotional content, speech rate, and prosody. According to the FLF, speech modes are therefore implemented at the motor speech programming level, the processing stage impaired in dysarthria (along with execution depending on the subtype of dysarthria).
The LaDIVA (laryngeal DIVA) extends the ‘Directions Into Velocities of Articulators’ (DIVA) model by Guenther et al. (Citation1998). DIVA is a computational model built on behavioural and neuroimaging findings on contextual variability, motor equivalence, coarticulation and speech rate effects (Guenther, Citation2016). Based on a linguistic (phonological) input, speech encoding activates motor targets in the ‘speech sound map’, leading to the reading of a learned set of articulatory gestures. This process is thought to be affected in AoS (Miller & Guenther, Citation2021). The feedforward controller then compares the motor target to the system’s current motor state, generating motor feedforward commands via initiation and articulation circuits, which are added to auditory and somatosensory feedback controllers to complete motor commands. In this framework, ‘pitch, loudness, and duration are controlled via feedforward and feedback control mechanisms’ (Guenther, Citation2016, p. 267). Despite describing the mechanisms underlying certain speech mode parameters (e.g. loudness), the encoding of speech mode is not further developed in DIVA. Indeed, DIVA lacks a function to convert motor movements into laryngeal articulator velocities. In LaDIVA (Weerathunge et al., Citation2022), a physiologically relevant vocal fold model is incorporated to simulate different modes of laryngeal motor control, including simulating pathological speech behaviours such as atypical vocal fold vibration. This vocal fold model receives information from the feedforward controller and translates vocal folds mobility space trajectories into changes in auditory and somatosensory task spaces. These laryngeal mobility space trajectories are then converted into sensory task space trajectories consisting of two auditory dimensions: vocal fundamental frequency, and sound pressure level. With the inclusion of this vocal fold model, both laryngeal and vocal tract articulatory components of the speech sound are controlled via the feedforward-feedback control architecture. Auditory feedback gain and feedforward learning rate in LaDIVA are adjustable model parameters that can simulate MSD. For instance, HD can be modelled by increasing the auditory feedback gain and decreasing the feedforward learning rate, as this MSD is thought to be represented by damage to the cortico-basal ganglia-thalamo-cortical motor loop, which is a critical component of the feedforward control system (Guenther, Citation2016, p. 282). From the DIVA model and its LaDIVA extension, the exact specification of speech mode encoding remains implicit, yet our interpretation is that it involves a combination of feedforward and feedback control mechanisms.
The presented speech control models make assumptions about the processing levels underpinning speech mode encoding (motor speech programming in FLF and feedforward/feedback control in LaDIVA) and the underlying impairments of AoS and dysarthria (motor speech planning in FLF and speech sound map in DIVA for AoS; motor speech programming in FLF and feedforward and feedback control in LaDIVA for dysarthria; specifically initiation circuit for HD). Consequently, producing different speech modes is presumed to be affected only by dysarthria, which explains why speech modes have not been investigated in AoS, as highlighted earlier. However, these assumptions about speech modes in the models rely on presumed neuronal structures and observations in pathology (speech modes production difficulties in dysarthria but not in AoS) without empirical confirmation. Investigating different speech modes in participants with different MSDs would add empirical background to the assumptions made by the speech production models.
In this study, our attention is directed towards examining the production of speech modes in individuals diagnosed with two distinct MSD, AoS and HD. Our objective is to better understand at which processing stage speech modes are encoded. An experimental paradigm was used to elicit meaningless speech sequences of different syllabic complexity produced in standard and whispered speech by speakers with AoS and dysarthria, and their matched controls. Whispered speech was chosen because of previous studies demonstrating difficulties in producing loud speech among individuals with HD (i.e. Fox et al., Citation2012; Sapir et al., Citation2007). Moreover, whispered speech is the most divergent speech mode from normal speech both behaviourally and in terms of phonatory pattern (Kelly & Hansen, Citation2018), as it involves laryngeal movement without periodic vocal fold vibration, but with the emission of a noisy laryngeal source. Finally, this speech mode was chosen to avoid a training bias in certain patients, as this whispered speech is typically not targeted in therapy. HD participants were selected because this type of dysarthria most closely represents an impairment located at the motor speech programming process. Performance in different speech modes is quantified with different measures: accuracy, initialisation time and acoustic duration. Besides speech modes, the articulatory complexity of the speech sequences was also manipulated as it is expected to elicit specific encoding/production difficulties in participants with AoS (Aichert & Ziegler, Citation2004).
If speech modes (here whispering) are encoded during the motor speech programming stage according to the FLF (corresponding to feedback and feedforward control mechanisms in LaDIVA), then we expect differences in accuracy and response latencies between normal and modulated speech only in participants with suggested motor programming impairments, namely dysarthria. Participants with AoS, characterised by an underlying motor speech planning impairment, should not display additional difficulties for whispered speech relative to normal speech. By contrast, they should be particularly impaired in encoding and producing complex syllables independent of the speech mode, while this should not apply to participants with dysarthria.
Method
Participants
A total of 40 participants were enrolled in the study, divided in four groups of 10 each: 2 clinical groups (AoS and HD) and their age-matched control groups (CNTRL-AoS and CNTRL-HD). The clinical groups included speakers with mild to moderate MSD presenting with AoS following a focal lesion (stroke or tumour) in the left hemisphere (AoS group) and with HD associated with PD (HD group).
All participants gave their consent, which was approved by the Swiss cantonal ethic research committee (2021–01597).
Clinical groups
The inclusion criteria for clinical group participants were: (1) French native speakers (2) with mild or moderate acquired speech difficulties diagnosed by a SLT at the recruiting hospitals (Geneva University Hospital, Switzerland or University Hospital of Bordeaux, France) and (3) no or only very mild language impairment. Participants with diagnosed dementia or psychiatric disorders and with history of developmental speech and language disorders or hearing impairment were excluded. The language abilities of the patients were assessed using the Geneva Bedside Aphasia Scale (Ge-BAS), a computerized battery for screening language disorders, allowing for the quantification of the severity of disorders on a 100-point scale (Chicherio et al., Citation2016). This battery consists of 12 subtests evaluating both oral and written production and comprehension abilities, oral calculation, orientation, as well as orofacial and ideomotor gestures. Only AoS participants with minimally impaired language (Ge_BAS >70) were included. Cognitive abilities in patients with HD were assessed using the Mini Mental State Examination (MMSE, Folstein, Folstein & McHugh, Citation1975). Only participants with unimpaired MMSE (>28) were included.
Patient speech was evaluated perceptually by five SLT using the BECD’s (‘Batterie d’Evaluation Clinique de la Dysarthrie’, a French clinical tool for evaluating motor speech disorders developed by (Auzou & Rolland-Monnoury, Citation2019) Composite Perceptive (CP) score. Such score was calculated by combining perceptual ratings across five dimensions: voice quality, phonetic realisation, prosody, intelligibility, and naturalness of speech. Ratings for each dimension were summed, with a maximum possible score of 20. Higher scores indicate more pronounced abnormalities in speech. Each dimension was evaluated based on an approximately 2-minute recording of the patient reading a sample text. The severity on the CP score was comparable between the two MSD groups (p = 0.99).
The AoS group consisted of 10 patients aged from 29 to 70 years old (mean age = 49.4 y.o., standard deviation (sd) = 12.19, 5 men) with AoS secondary to a left hemisphere stroke (N = 9) or brain tumour (N = 1). The diagnosis of AoS was determined by a SLT, who also rated the Apraxia of Speech Rating Scale (ASRS 1.0; Strand et al., Citation2014). This scale quantitatively assesses 16 speech characteristics linked to AoS, assigning scores ranging from 0 (normal speech) to 64. Among the participants with AoS, the average ASRS score was 21.6, with a range spanning from 8 to 40. The criteria for diagnosing AoS included laborious and non-fluent speech, syllabification, variability over time, dysprosody, phonetic and phonemic errors, as well as frequent false starts (Ziegler et al., Citation2012; Allison et al., Citation2020
The HD group included 10 patients presenting PD. They were aged from 52 to 84 years old (mean age = 70.3 y.o., sd = 11.11, 8 men). 9 participants were on classical medication for PD, and one of them (P15) was implanted with DBS. The diagnosis of HD was also determined by a SLT. Participants with HD were required to exhibit PD accompanied by speech symptoms such as articulatory imprecision, hypophonia, dysprosody, or variable speech rate (Duffy, Citation2019).
Detailed demographic and clinical information for the patients, including severity, are presented in . The two groups of participants were not age-matched (p < 0.001).
Table 1. Participants’ demographic information and severity.
Control groups
Neurotypical control groups were recruited in Geneva using public advertisements. The CNTRL-AoS group included 10 neurotypical speakers (mean age = 44.9 y.o., sd = 17.27, 4 men) matched on age to the AoS clinical group, and the CNTRL-HD group comprised 10 neurotypical speakers (mean age = 72.2 y.o., sd = 8.26, 3 men) matched on age to the HD clinical group. A key criterion for recruitment was being a native French speaker without foreign accent. Moreover, they had no history of voice, speech, or language disorders.
Materials
The stimuli were 54 disyllabic pseudo-words composed of a first syllable corresponding to the three following syllabic structures: 18 legal CV syllables, 18 legal CCV syllables and 18 illegal CCV syllables. These syllables were chosen from the set of phonotactically legal CCV syllables in French starting with voiced plosive consonants/b/,/d/and/g/(mean syllabic frequency = 644.25, SD = 1683.96, Lexique 3.8, (New et al., Citation2004). From these syllables, the set of CV syllables (mean syllabic = 699.97, SD = 1617.71) was obtained by removing the second consonant. The third set of illegal CCV syllables (syllabic frequency = 0) was created by replacing the second consonant of the legal CCV syllables in order to create illegal clusters (changing/l/or/R/to/d/,/n/or/v/). To form disyllabic pseudo-words, those three sets of syllables were associated to four different -CV syllables:/si/,/mi/,/ʁe/and/mɛ/. The stimuli were thus constructed based on matched sets of three stimuli (CV.CV, leal CCV.CV and illegal CCV.CV, hereafter called ‘triplets’), with increasing articulatory complexity from CV to illegal CCV. In addition to the 54 target stimuli (examples in ), 12 similarly-structured stimuli were included as training items and fillers. A complete list of all stimuli is provided in Appendix A.
Table 2. Examples of three matched triplets of pseudo-words.
Procedure
Participants were situated in a quiet room, positioned at a distance of 70 cm from a computer screen. The E-prime software (version 2.0, Schneider et al., (Citation2002)) was used for the stimuli presentation and audio data collection.
Prior to the main task, participants underwent a familiarisation phase where they read aloud all the stimuli in a random order in the presence of the experimenter, who provided corrections if necessary.
The primary task employed a standard delayed production paradigm targeting motor speech encoding processes (e.g. Chang et al., Citation2009; Kawamoto et al., Citation2008; Lancheros et al., Citation2020). In such a task, participants produce the targeted pseudo-word upon the presentation of a response cue after a variable delay. During such a delay, participants complete linguistic encoding processes (see Laganaro, Citation2019 for more details).
Guided training preceded each experimental block. More specifically, during the training phase, the experimenter explained the task, calibrated speech modes, and provided four example productions (normal and whispered). Then, participants were asked to produce five pseudo-words in the specific speech mode. If participants did not whisper correctly, the experimenter first explained the expected production (e.g. ‘no voicing’) and provided further training on additional items. Only when the two uttering conditions (i.e. normal and whispered speech) were correctly produced, the experimental phase started.
The experimental phase consisted of separate, counterbalanced blocks for normal and whispered speech. Each trial began with a 100 ms blank screen interval, followed by a ‘+’ in the middle of the screen for 500 ms. Then, a written pseudo-word was visually presented for 1200 ms, followed by a random delay of 1300, 1500, 1700, or 1900 ms, during which participants waited. A variable delay was introduced so that participants could not anticipate the presentation of the response cue (Laganaro & Alario, Citation2006). After the delay, a response-cue (see ) accompanied by a sound signal prompted participants to speak. The response cue remained until the experimenter moved to the next stimulus. Participants were asked to produce the target pseudo-word as fast and accurately as possible, either normally or whispering, according to the condition. Audio recording started at the response cue and continued until the examiner proceeded to the next stimulus. For the normal speech condition, the instructions were: ‘As soon as the image that tells you to speak appears on the screen, you must say aloud the sequence of syllables previously presented as quickly and accurately as possible. WARNING: in this condition you will have to produce the sequence of syllables normally’. For the whispered speech task, only the last part of the instruction varied: ‘(…) WARNING: in this condition you will have to produce the sequences of syllables by whispering’.
Figure 1. Experimental design of the delayed production task (either in the normal or whispered condition).
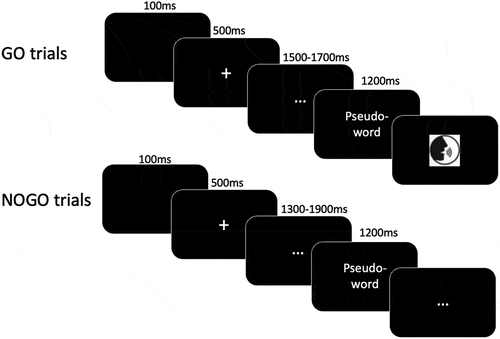
A ‘no-go’ condition, indicated by ‘…’ instead of the production cue, prompted participants to wait for the next stimulus. This condition was associated with fillers items (12 additional pseudo-words) that were not analysed but aimed to maintain participant attention and prevent anticipatory responses. The shortest and longest delays (1300 and 1900 respectively) were associated with this ‘no-go’ condition.
Items were pseudo-randomised such that the same type of syllables, the same initial phoneme, and the same delay were not presented in more than three consecutive trials. The order of the two blocks/conditions (normal or whispered) was counterbalanced across participants.
Pre-analyses
Digitized responses were analysed with Praat (Boersma & Weenink, Citation2018) to identify their accuracy. Only the first (target) syllable was coded. Phonemic substitutions on the first syllable were considered production errors. Perceived phonetic transformations, auto-corrections, and voicing errors (in the normal speech condition) were not scored as errors. No responses (N = 26, 1.20%), or responses in the wrong speech mode (N = 4, 0.19%), were removed from the dataset. Two independent raters coded accuracy.
Production latencies (hereafter response times -RTs-) were measured on Praat (Boersma & Weenink, Citation2018) from the presentation of the response cue until the vocal onset. To maintain consistent alignment between the normal and whispered conditions despite the voiced onset consonant, RTs were synchronised with the onset of the first vowel in the pseudowords. Thus, for both normal and whispered speech conditions, the analysis involved spectrograms, where the presence of a vowel was indicated by the attendance of formants in the mid-frequency region (F1/F2 region) (Ridouane & Fougeron, Citation2011). The vowel onset corresponded to the end of the preceding consonant (Meynadier & Gaydina, Citation2013).
Finally, the speech sequence duration, starting from the onset of the first vowel (V.CV duration), was measured as for the RTs analysis. Examples of pseudo-word segmentations are provided in Appendix B.
Analyses
For data cleaning purposes on the RTs and durational data, errors and outliers (RTs <200 ms or >1500 ms; durations <200 ms or >1600 ms) were excluded. RTs, accuracy and duration were fitted with mixed models (Baayen et al., Citation2008) in the R-software (R-project, R-development core team 2005) to asses performance among each MSD group and their matched control group as well as between the two MSD groups. Three models were thus performed comparing: AoS versus CNTRL-AoS, HD versus CNTRL-HD, and AoS versus HD. Statistical analyses were computed with the packages lmerTest (Kuznetsova et al., Citation2017) and Lme4 (Bates et al., Citation2015). The model included accuracy, RT or acoustic durations as dependent variables. Speech mode (Mode, normal versus whispered speech), type of syllable (Typesyll), group (Group, patients versus controls, or AoS versus HD) and order of the stimuli (Order – absolute order) were the fixed factors. Participants and triplets were entered as random factors, but slopes could not be added to the random structure because of convergence issues. Interactions between Mode, Typesyll and Group and were also entered in the initial models and removed when non-significant. Post-hoc analyses were performed using Tukey tests from the emmeans package (Lenth et al., Citation2018).
Results
Accuracy
Inter-rater agreement for accuracy was substantial, with a value of .79 for the patient groups and .69 for the control groups (Kappa statistics, Landis & Koch, Citation1977)). Mean accuracy for MSD patients and their controls for each speech mode and per syllable type are displayed in .
Clinical versus control groups
The generalised mixed models for binomial distribution on accuracy revealed in the two models no significant effect of Mode; a main effect of Typesyll with better performance for CV stimuli than for both legal and illegal CCVs (p < 0.001), and better performance for legal CCVs compared to illegal syllables (p < 0.001); and a main effect of Group with better performances for controls compared to patients. A main effect of Order (p = 0.04, indicating performance improvement) was observed only in the HD versus CNTRL-HD model. Finally, an interaction effect between Typesyll and Group (p = 0.03) was present only for the AoS versus CNTRL-AoS indicating lower performance only for CCV stimuli (both legal and illegal) in the AoS patients relative to their matched controls. See detailed results in .
Table 3. Results from the generalised mixed models on accuracy.
AoS versus HD
The model showed no significant main effect neither of Mode nor of Group. However, a main effect of Typesyll was found, with better performance for CV than for both legal and illegal CCV stimuli (p < 0.001) and better performance for legal compared to illegal CCV syllables (p < 0.001); as well as a significant effect of Order (p = 0.001) showing increase of performance throughout the task; and a significant interaction between Group and Typesyll (p < 0.001) showing better performances for the HD group only on illegal CCV stimuli. See detailed results in .
Given the low performance of the illegal CCV stimuli, especially in patient groups, they were removed from the RTs and duration analysis leading to 36 disyllabic pseudo-words per speech mode. Typesyll was no longer analysed as the acoustic analyses were aligned to the first vowel (see rationale in the Method section).
Response latencies
The results on the RTs aligned to the first vowel per speech mode (Mode) and per Group are presented in .
Clinical versus control groups
The linear mixed model on RTs comparing the AoS and the CNTRL-AoS groups revealed a main effect of Mode (p = 0.002) with longer RTs for whispered than for normal speech; a main effect of Group (p = 0.001) with significantly longer RTs for the AoS patients compared to the CNTRL-AoS; a main effect of Order (p < 0.001) with a decrease of RTs throughout the task; and a significant interaction effect between Mode and Group (p = 0.003) which shows a 57-ms-longer-initialisation time for whispered speech relative to normal speech only in AoS patients.
Comparing the HD and the CNTRL-HD groups, the linear-mixed model showed no effect of Mode; nor of Group; a main effect of Order (p < 0.001) with a decrease of RTs throughout the task; and a significant interaction between Mode and Group (p < 0.001) revealing a significant difference between normal and whispered speech only in HD patients, with 35 ms faster initialisation in whispered speech in this patient’s group.
See detailed results in .
Table 4. Results from the linear mixed model on response latencies.
AoS versus HD
The linear mixed model on RTs comparing the AoS and the HD groups revealed no effect of Mode; a main effect of Group (p = 0.03) with longer RTs for the AoS compared to the HD group; a main effect of Order (p < 0.001); and a significant interaction between Mode and Group showing difference between normal and whispered speech in both groups. However, while RTs are 58 ms longer for the whispered relative to the normal speech condition in the AoS group, they are 35 ms faster in the whispered speech condition for the HD group. See detailed results in .
Acoustic duration
The results on the acoustic durations aligned to the vowel per speech mode (Mode) and per Group are presented in .
Clinical versus control groups
The results on the V.CV duration revealed a significant difference between normal (446.53 ms, SD = 118.09) and whispered (480.72 ms, SD = 123.87) speech, with whispered speech having longer acoustic duration in all groups. Comparing the AoS versus CNTRL-AoS groups, a main effect of Group was revealed (p = 0.05) showing longer acoustic durations for the AoS compared to the control group, but there was no interaction between Mode and Group. When comparing the HD to their matched control group, there was no effect of Group; a main effect of Order; and a marginal interaction effect between Mode and Group (p = 0.06). See detailed results in .
Table 5. Results from the linear mixed model on acoustic durations.
AoS versus HD
The linear mixed model on acoustic durations comparing the AoS and the HD groups revealed a main effect of Mode (p < 0.001, still with longer durations for the whispered compared to the normal speech condition); no effect of Group; a main effect of Order (p = 0.02) with a decrease of acoustic durations throughout the task; and a significant interaction between Mode and Group (p = 0.004). When decomposed, this interaction revealed a significant difference in terms of acoustic duration in both AoS and HD groups, but with the highest estimates in the AoS group (48 ms) and the lowest estimate in the HD group (16 ms). See detailed results in .
Discussion
This study investigated the effects of speech mode (normal vs. whispered) on accuracy, initialisation time, and acoustic duration in individuals with MSD compared to their age-matched controls. On accuracy, no significant differences were observed between speech modes, indicating that individuals with MSD could maintain similar levels of precision across speech conditions. However, syllable complexity influenced accuracy in the clinical groups regardless of speech mode, with illegal CCV being particularly impaired in the AoS group. On RTs, whispered speech yielded longer initialisation times in the group of AoS compared to their controls, while RTs were faster in the HD group compared to their controls. Finally, on acoustic duration, whispered speech had longer acoustic duration than normal speech across all participant groups, but lengthening was greater in the AoS group than in the HD group. In the following sections we will discuss those results in terms of speech mode encoding. The observation that accuracy was not affected by speech mode in any group is hardly informative as it may be due to the mild impairment in the clinical groups, we will therefore base the discussion mainly on the results on RTs and on acoustic durations.
Initialization and duration of whispered speech compared to normal speech in AoS and HD
The patterns of results summarised above is clearly in contradiction with the prediction that speaking in different speech modes should be particularly challenging for participants with dysarthria but not for participants with AoS. RTs results were in the opposite direction for AoS and dysarthria, with a cost observed only in the AoS patients’ group (58 ms longer for whispered compared to normal speech). Conversely, in the HD patients’ group, RTs were 35 ms faster for whispered speech. Importantly, these results are independent of the order of presentation of the tasks/stimuli. These results suggest that the impact of whispering on initialisation time is specific to individuals with AoS, potentially reflecting that the speech motor control difficulties associated with this disorder (motor speech planning in the FLF and the speech sound map in LaDIVA) are also involved in encoding whispered speech, and possibly speech modes in general. Concerning HD patients, the absence of an encoding cost for whispered speech, with longer RTs in normal than in whispered speech, might be specific to this subtype of dysarthria. It is plausible that whispered speech is more easily produced by participants with HD given the extrapyramidal dysfunction underlying PD. Indeed, hypophonia is characteristic of HD and is generally manifested by glottal incompetence due to bowing of the vocal folds, in some cases with accompanying atrophy (Watts, Citation2016). These changes result from rigidity in respiratory and laryngeal muscles due to the extrapyramidal dysfunction underlying the disease (Blumin et al., Citation2004; Zarzur et al., Citation2009) as well as impaired scaling of vocal effort (Sapir, Citation2014). Despite this possible specificity of HD for whispered speech, whispering seems particularly challenging in AoS not only in terms of RTs but also in terms of articulation as reflected by acoustic durations. Indeed, while an increase in the acoustic duration of the pseudo-words is observed in whispered compared to normal speech for all participant groups, the AoS group showed higher increase in acoustic duration (48 ms), compared to the HD group (16 ms). The lengthening of speech sequences in whispered speech observed in all groups aligns with previous research indicating that modulated speech tends to have longer acoustic durations. For example, Zhang & Hansen (Citation2007) reported longer sentence length in modulated speech compared to normal speech across various speech modes (whispered, soft, loud, and shouted speech). This lengthening in whispered speech duration might be influenced by linguistic predictability within the context, since whispered speech, being less predictable, tends to have longer durations (e.g. Lieberman, Citation1963; Meynadier & Gaydina, Citation2013).
What is crucial and novel in the present study is the alignment of results between acoustic duration and RTs. Specifically, the group displaying the longest duration in whispered speech also exhibited longer RTs. Conversely, the HD group demonstrated faster initialisation of whispered speech, leading to a smaller increase in acoustic durations and no difference with the control participants. Those findings may be explained by greater articulatory difficulties in AoS, as demonstrated in the literature, especially for more complex syllables such as clusters (Aichert & Ziegler, Citation2004; Galluzzi et al., Citation2015; Romani & Galluzzi, Citation2005), a result that is also replicated here in particular for illegal clusters. These articulatory difficulties could be intensified when whispering, resulting in extended acoustic durations as well as longer RTs in that speech mode. Indeed, specific difficulties related to voicing characteristics have already been highlighted in the AoS group, with authors demonstrating more errors produced by individuals with AoS on unvoiced stimuli compared to voiced stimuli, as opposed to patients with aphasia without associated AoS (Bislick & Hula, Citation2019). In this paper, as initialisation latencies are aligned on the vowel (see the Method section for rationale), articulatory difficulties on the preceding cluster could lead to longer RTs. In addition, articulatory initiation difficulties (such as articulatory efforts well described in AoS, e.g. Duffy, Citation2019) could cause lengthening of RTs. Since our analyses on acoustic durations and RTs are derived from a V.CV sequence, we were unable to verify the influence of syllabic complexity on speech mode, but this makes the present results even more noteworthy as they are based on a short speech sequence of low syllabic complexity (V.CV). Thus, it appears that individuals with impairments in motor speech planning encounter specific challenges when attempting to generate speech modes that deviate from normal speech. For participants with HD, the smaller increase in acoustic durations during whispered speech could be attributed to reduced amplitude of articulatory movements associated with PD (e.g. Martínez-Sánchez et al., Citation2016). Thus, the amplitude could be even more reduced during whispered compared to normal speech, resulting in shorter durations.
Speech modes in models of motor speech control
In terms of encoding of speech modes in the motor speech control models, those results could be explained in two ways. Speech modes may be encoded during motor speech planning, which would explain why people with AoS have difficulty producing whispered speech, whereas dysarthric or neurotypical participants do not. Indeed, producing a whispered version of a pseudo-word may compromise the already activated motor plan, requiring the individuals to inhibit that plan or to create a new motor plan online, resulting in slower RTs and longer durations. However, these results can also be explained by a cascade effect of planning impairment on programming for the AoS group. Indeed, according to Van Der Merwe (Citation2021), speech mode encoding occurs at the motor speech programming processing stage, but she also suggests that prosodic and speech rate impairments observed in AoS (Duffy, Citation2019; McNeil et al., Citation2009) might not be primarily related to planning impairment, but they could reflect a secondary effect of planning impairment on subsequent programming. This secondary effect could also apply to speech modes, where the difficulties with whispered speech in AoS come from a cascade effect of motor speech planning difficulties on programming. Results observed in participants with HD can be explained assuming that normal speech represents a change in speech mode, the by-default mode being whispered speech. Following this hypothesis, previous observation that this clinical group is particularly impaired in producing loud speech (Fox et al., Citation2012) can also be understood.
In summary, it remains challenging to define whether the encoding of speech modes occurs at the motor speech planning or programming processing stages. Similar results supporting both encoding processes have been obtained in a study with neurotypical speakers (Bourqui et al., Citation2023, subm.), which addressed speech modes in the context of motor speech control models. This study revealed a cognitive-motor processing cost associated with speech modes compared to normal speech, but also failed to locate their exact encoding. Considering the difficulties in producing modulated speech by both patient groups and the rationale developed above for the opposite results observed in HD, along with the cascading across encoding processes, it is likely that speech modes are encoded at the motor speech programming processing stage.
Conclusion
The present study investigated the effects of speech modes on individuals with motor speech disorders (MSD), specifically apraxia of speech (AoS) and hypokinetic dysarthria (HD). We observed that individuals with mild MSD could maintain similar levels of accuracy across different speech modes. This suggests their adaptability in adjusting articulatory strategies to maintain accuracy even in challenging speech conditions. However, the two patient groups displayed distinct patterns in terms of initialisation time and acoustic duration: opposite to the predictions from the literature and the models, AoS patients were the most affected by the whispered speech mode, with longer latencies and longer acoustic duration compared to normal speech, while participants with HD showed longer initialisation times in normal speech and less prolonged acoustic duration in whispered speech. These results highlight the specific and unexpected difficulties faced by individuals with AoS in the non-standard speech modes and prompts a reconsideration of how speech modes are encoded in speech motor control models. Further research is needed to investigate the encoding mechanisms of different speech modes and their impact on various MSD, including other types of dysarthria and other types of speech modes.
Supplemental Material
Download PDF (381.6 KB)Acknowledgments
The authors gratefully acknowledge their colleague Cyrielle Demierre for her help as the second rater for patient accuracy assessments. They would also like to express their gratitude to Bertrand Glize for the data collection sessions in Bordeaux.
Disclosure statement
No potential conflict of interest was reported by the author(s).
Data availability statement
The datasets generated during and/or analysed during the current study are available in the [Yareta institutional open science archive (https://yareta.unige.ch/home)] repository’. The link for the material is the following: https://yareta.unige.ch/archives/6dde9793-1bb4-4e85-8a98-61eed2b43ab1.
Supplementary material
Supplemental data for this article can be accessed online at https://doi.org/10.1080/02699206.2024.2345353
Additional information
Funding
References
- Aichert, I., & Ziegler, W. (2004). Syllable frequency and syllable structure in apraxia of speech. Brain and Language, 88(1), 148–159. https://doi.org/10.1016/S0093-934X(03)00296-7
- Allison, K. M., Cordella, C., Luzzini-Seigel, J., & Green, J. R. (2020). Differential diagnosis of apraxia of speech in children and adults: A scoping review. Journal of Speech, Language and Hearing Research, 63(9), 2952–2994. https://doi.org/10.1044/2020_JSLHR-20-00061
- Auzou, P. & Rolland-Monnoury, V. (2019). Batterie Clinique D’évaluation de la Dysarthrie. Isbergues.
- Baayen, R. H., Davidson, D. J. & Bates, D. M. (2008). Mixed-effects modeling with crossed random effects for subjects and items. Journal of Memory and Language, 59(4), 390–412.
- Bates, D., Maechler, M., Bolker, B., Walker, S., Christensen, R. H. B., Singmann, H. & Bolker, M. B. (2015). Package ‘Lme4’. Convergence, 12(1), 2.
- Bislick, L., & Hula, W. D. (2019). Perceptual characteristics of consonant production in Apraxia of speech and aphasia. American Journal of Speech-Language Pathology, 28(4), 1411–1431. https://doi.org/10.1044/2019_AJSLP-18-0169
- Blumin, J. H., Pcolinsky, D. E., & Atkins, J. P. (2004). Laryngeal findings in advanced Parkinson’s disease. Annals of Otology, Rhinology & Laryngology, 113(4), 253258. https://doi.org/10.1177/000348940411300401
- Blumstein, S. (1990). Phonological deficits in aphasia: Theoretical perspectives. In A. Caramazza (Ed.), Cognitive neuropsychology and neurolinguistics (pp. 33–53). Lawrence Erlbaum.
- Boersma, P. & Weenink, D. (2018). Praat: Doing phonetics by computer [ Computer program]. Version 6.0. 37.
- Bourqui, M. (2023). Differentiating motor speech planning versus programming: Exploring the role of speech modes in speech production.
- Brooks, V. B. (1986). The neural basis of motor control. Oxford University Press.
- Chang, S.-E., Kenney, M. K., Loucks, T. M., Poletto, C. J., & Ludlow, C. L. (2009). Common neural substrates support speech and non-speech vocal tract gestures. Neuroimage: Reports, 47(1), 314–325. https://doi.org/10.1016/j.neuroimage.2009.03.032
- Chicherio, C., Genoud-Prachex, T., Assal, F. & Laganaro, M. (2016). Cyber-neuro-psychologie ou l’application des nouvelles technologies à l’évaluation précoce en phase aiguë des patients cérébro-lésés. In Journée d’Hiver de la Société de Neuropsychologie de Langue Française. Paris.
- D’Alessandro, D., Pernon, M., Fougeron, C. & Laganaro, M. (2019, June). Anticipatory VtoV coarticulation in French in several Motor Speech Disorders. In Phonetics and Phonology in Europe (PAPE 2019). Lecce, Italy.
- Duffy, J. R. (2019). Motor speech disorders-E-Book: Substrates, differential diagnosis. Elsevier Health Sciences.
- Duffy, J. R. (2019). Motor speech disorders e-book : Substrates, differential diagnosis, and management. Elsevier Health Sciences.
- Enderby, P. (2013). Chapter 22 - disorders of communication : Dysarthria. In M. P. Barnes & D. C. Good (Eds.), Handbook of clinical neurology (Vol. 110, p. 273281). Elsevier. https://doi.org/10.1016/B978-0-444-52901-5.00022-8
- Folstein, M. F., Folstein, S. E. & McHugh, P. R. (1975). “Mini-mental state”: A practical method for grading the cognitive state of patients for the clinician. Journal of Psychiatric Research, 12(3), 189–198.
- Fougeron, C., Kodrasi, I. & Laganaro, M. (2022). Differentiation of Motor Speech Disorders through the seven deviance scores from MonPaGe-2.0.S. Brain Sciences, 12(11), 1471. https://doi.org/10.3390/brainsci12111471
- Fox, C., Ebersbach, G., Ramig, L., & Sapir, S. (2012). LSVT LOUD and LSVT BIG: Behavioral treatment programs for speech and body movement in parkinson disease. Parkinson’s Disease, 2012, e391946. https://doi.org/10.1155/2012/391946
- Galluzzi, C., Bureca, I., Guariglia, C., & Romani, C. (2015). Phonological simplifications, apraxia of speech and the interaction between phonological and phonetic processing. Neuropsychologia, 71, 64–83. https://doi.org/10.1016/j.neuropsychologia.2015.03.007
- Guenther, F. H. (2016). Neural control of speech. MIT Press.
- Guenther, F. H., Hampson, M., & Johnson, D. (1998). A theoretical investigation of reference frames for the planning of speech movements. Psychological Review, 105(4), 611–633. https://doi.org/10.1037/0033-295X.105.4.611-633
- Kawamoto, A. H., Liu, Q., Mura, K., & Sanchez, A. (2008). Articulatory preparation in the delayed naming task. Journal of Memory and Language, 58(2), 347–365. https://doi.org/10.1016/j.jml.2007.06.002
- Kelly, F. & Hansen, J. H. L. (2018). Detection and calibration of whisper for speaker recognition. 2018 IEEE Spoken Language Technology Workshop (SLT), Athens, Greece (pp. 1060–1065. https://doi.org/10.1109/SLT.2018.8639595
- Kodrasi, I., Pernon, M., Laganaro, M., & Bourlard, H. (2020). Automatic discrimination of apraxia of speech and dysarthria using a minimalistic set of handcrafted features. Interspeech, 2020, 49914995. https://doi.org/10.21437/Interspeech.2020-2253
- Kuznetsova, A., Brockhoff, P. B. & Christensen, R. H. B. (2017). lmerTest package: Tests in linear mixed effects models. Journal of Statistical Software, 82(13).
- Laganaro, M. (2019). Phonetic encoding in utterance production: A review of open issues from 1989 to 2018. Language, Cognition and Neuroscience, 34(9), 1193–1201. https://doi.org/10.1080/23273798.2019.1599128
- Laganaro, M. & Alario, F. X. (2006). On the locus of the syllable frequency effect in speech production. Journal of Memory and Language, 55(2), 178–196.
- Lancheros, M., Jouen, A.-L., & Laganaro, M. (2020). Neural dynamics of speech and non-speech motor planning. Brain and Language, 203, 104742. https://doi.org/10.1016/j.bandl.2020.104742
- Lancheros, M., Pernon, M., & Laganaro, M. (2023). Is there a continuum between speech and other oromotor tasks? Evidence from motor speech disorders. Aphasiology, 37(5), 715–734. https://doi.org/10.1080/02687038.2022.2038367
- Landis, J. R. & Koch, G. G. (1977). An application of hierarchical kappa-type statistics in the assessment of majority agreement among multiple observers. Biometrics Bulletin, 363–374.
- Lenth, R., Singmann, H., Love, J., Buerkner, P. & Herve, M. (2018). Emmeans: Estimated marginal means. 1(7). AKA least-squares means.
- Lieberman, P. (1963). Some effects of semantic and grammatical context on the production and perception of speech. Language and Speech, 6(3), 172 187.
- Martínez-Sánchez, F., Meilán, J. J. G., Carro, J., Íniguez, C. G., Millian-Morell, L., Valverde, I. P. M., Lopez-Alburquerque, T., & Lopez, D. E. (2016). Speech rate in Parkinson’s disease: A controlled study. Neurologia, 31(7), 466–472. https://doi.org/10.1016/j.nrl.2014.12.002
- McNeil, M. R., Robin, D. A. & Schmidt, R. A. (2009). Apraxia of speech: Definition, differentiation, and treatment. In M. R. McNeil (Ed.), Clinical management of sensorimotor speech disorders (pp. 249–268).
- Melle, N., & Gallego, C. (2012). Differential diagnosis between Apraxia and Dysarthria based on acoustic analysis. The Spanish Journal of Psychology, 15(2), 495504. https://doi.org/10.5209/rev_SJOP.2012.v15.n2.38860
- Meynadier, Y., & Gaydina, Y. (2013). Aerodynamic and durational cues of phonological voicing in whisper. Interspeech, 335–339. https://hal.archives-ouvertes.fr/hal-01211117
- Miller, H. E., & Guenther, F. H. (2021). Modelling speech motor programming and apraxia of speech in the DIVA/GODIVA neurocomputational framework. Aphasiology, 35(4), 424441. https://doi.org/10.1080/02687038.2020.1765307
- New, B., Pallier, C., Brysbaert, M. & Ferrand, L. (2004). Lexique 2: A new French lexical database. Behavior Research Methods Instruments & Computers, 36(3), 516–524.
- Palmer, R., & Enderby, P. (2007). Methods of speech therapy treatment for stable dysarthria: A review. Advances in Speech Language Pathology, 9(2), 140153. https://doi.org/10.1080/14417040600970606
- Parrell, B., Lammert, A. C., Ciccarelli, G., & Quatieri, T. F. (2019). Current models of speech motor control: A control-theoretic overview of architectures and properties. The Journal of the Acoustical Society of America, 145(3), 1456–1481. https://doi.org/10.1121/1.5092807
- Pernon, M., Assal, F., Kodrasi, I., & Laganaro, M. (2022). Perceptual classification of motor speech disorders: The role of severity, speech task, and Listener’s expertise. Journal of Speech, Language, and Hearing Research, 65(8), 2727–2747. https://doi.org/10.1044/2022_JSLHR-21-00519
- Ramig, L. O., Countryman, S., Thompson, L. L., & Horii, Y. (1995). Comparison of two forms of intensive speech treatment for parkinson disease. Journal of Speech, Language, and Hearing Research, 38(6), 1232–1251. https://doi.org/10.1044/jshr.3806.1232
- Ridouane, R. & Fougeron, C. (2011). Schwa elements in Tashlhiyt word-initial clusters. Laboratory Phonology, 2(2), 275–300.
- Romani, C., & Galluzzi, C. (2005). Effects of syllabic complexity in predicting accuracy of repetition and direction of errors in patients with articulatory disorders and phonological difficulties. Cognitive Neuropsychology, 27(7), 817–850. https://doi.org/10.1080/02643290442000365
- Sapir, S. (2014). Multiple factors are involved in the dysarthria associated with Parkinson’s disease: A review with implications for clinical practice and research. Journal of Speech, Language, and Hearing Research, 57(4), 1330–1343. https://doi.org/10.1044/2014_JSLHR-S-13-0039
- Sapir, S., Spielman, J. L., Ramig, L. O., Story, B. H. & Fox, C. (2007). Effects of Intensive Voice Treatment (the Lee Silverman Voice Treatment [LSVT]) on vowel articulation in dysarthric individuals with idiopathic parkinson disease : Acoustic and perceptual findings. Journal of Speech, Language, & Hearing Research, 50(4), 899–912.
- Schneider, W., Eschman, A. & Zuccolotto, A. (2002). E-Prime reference guide. Psychology Software Tools, Incorporated.
- Schultz, W., & Romo, R. (1992). Role of primate basal ganglia and frontal cortex in the internal generation of movements. I. Preparatory activity in the anterior striatum. Experimental Brain Research, 91(3), 363–384. https://doi.org/10.1007/BF00227834
- Skodda, S. (2011). Aspects of speech rate and regularity in Parkinson’s disease. Journal of the Neurological Sciences, 310(1), 231–236. https://doi.org/10.1016/j.jns.2011.07.020
- Strand, E. A., Duffy, J. R., Clark, H. M. & Josephs, K. (2014). The apraxia of speech rating scale: A tool for diagnosis and description of apraxia of speech. Journal of Communication Disorders, 51, 43–50.
- Tjaden, K., & Wilding, G. E. (2004). Rate and loudness manipulations in dysarthria. Journal of Speech, Language, and Hearing Research, 47(4), 766–783. https://doi.org/10.1044/1092-4388(2004/058)
- Utianski, R. L., Duffy, J. R., Martin, P. R., Clark, H. M., Stierwalt, J. A. G., Botha, H., Ali, F., Whitwell, J. L., & Josephs, K. A. (2023). Rate modulation abilities in acquired motor speech disorders. Journal of Speech, Language and Hearing Research, 66(8), 3194–3205. https://doi.org/10.1044/2022_JSLHR-22-00286
- Utianski, R. L., Martin, P. R., Hanley, H., Duffy, J. R., Botha, H., Clark, H. M., Whitwell, J. L., & Josephs, K. A. (2021). A longitudinal evaluation of speech rate in primary progressive apraxia of speech. Journal of Speech, Language, and Hearing Research, 64(2), 392–404. https://doi.org/10.1044/2020_JSLHR-20-00253
- Van der Merwe, A. (1997). A theoretical framework for the characterization of pathological speech sensorimotor control. In M. R. McNeil (Ed.), Clinical management of sensorimotor speech disorders (pp. 1–25). Thieme.
- Van Der Merwe, A. (2021). New perspectives on speech motor planning and programming in the context of the four- level model and its implications for understanding the pathophysiology underlying apraxia of speech and other motor speech disorders. Aphasiology, 35(4), 397423. https://doi.org/10.1080/02687038.2020.1765306
- Varley, R., & Whiteside, S. (2001). What is the underlying impairment in acquired apraxia of speech. Aphasiology, 15, 39–49. https://doi.org/10.1080/02687040042000115
- Watts, C. R. (2016). A retrospective study of long-term treatment outcomes for reduced vocal intensity in hypokinetic dysarthria. BMC Ear, Nose and Throat Disorders, 16(1), 2. https://doi.org/10.1186/s12901-016-0022-8
- Weerathunge, H. R., Alzamendi, G. A., Cler, G. J., Guenther, F. H., Stepp, C. E., Zañartu, M., & Theunissen, F. E. (2022). LaDIVA: A neurocomputational model providing laryngeal motor control for speech acquisition and production. PLOS Computational Biology, 18(6), e1010159. https://doi.org/10.1371/journal.pcbi.1010159
- Zarzur, A. P., Duprat, A. C., Shinzato, G., & Eckley, C. A. (2009). Laryngeal electromyography in adults with Parkinson’s disease and voice complaints. The Laryngoscope, 117(5), 831–834. https://doi.org/10.1097/MLG.0b013e3180333145
- Zhang, C. & Hansen, J. H. (2007, August). Analysis and classification of speech mode: Whispered through shouted. Interspeech, 7, 2289–2292.
- Ziegler, W. (2002). Task-related factors in oral motor control: Speech and oral diadochokinesis in dysarthria and apraxia of speech. Brain and Language, 80(3), 556–575. https://doi.org/10.1006/brln.2001.2614
- Ziegler, W., Aichert, I., & Staiger, A. (2012). Apraxia of speech: Concepts and controversies. Journal of Speech, Language and Hearing Research, 55(5), 1485–1501. https://doi.org/10.1044/1092-4388(2012/12-0128)