ABSTRACT
Previous research has found that individuals vary greatly in emotion differentiation, that is, the extent to which they distinguish between different emotions when reporting on their own feelings. Building on previous work that has shown that emotion differentiation is associated with individual differences in intrapersonal functions, the current study asks whether emotion differentiation is also related to interpersonal skills. Specifically, we examined whether individuals who are high in emotion differentiation would be more accurate in recognising others’ emotional expressions. We report two studies in which we used an established paradigm tapping negative emotion differentiation and several emotion recognition tasks. In Study 1 (N = 363), we found that individuals high in emotion differentiation were more accurate in recognising others’ emotional facial expressions. Study 2 (N = 217), replicated this finding using emotion recognition tasks with varying amounts of emotional information. These findings suggest that the knowledge we use to understand our own emotional experience also helps us understand the emotions of others.
Correctly inferring emotions from others is an important component of social life. Beyond merely inferring that something is wrong, knowing that the other is sad rather than angry or afraid enables us to engage in social interactions that are tailored to the other’s specific emotional state. The degree to which an individual is able to make fine-grained, subtle distinctions between similar emotions has been referred to as emotion differentiation or emotion granularity (Barrett, Gross, Christensen, & Benvenuto, Citation2001; Smidt & Suvak, Citation2015). To date, however, emotion differentiation has been mainly studied with regard to one’s own emotions and has not been applied to the recognition of others’ emotions. In the present paper, we therefore focus on the question of whether people who make fine-grained distinctions between their own experienced emotions are also more accurate in recognising other people’s emotions.
We define emotion differentiation as the ability to use emotion words with such specificity that one’s emotion words vary across emotional situations. Thus, people low in emotion differentiation tend to use emotion labels like ‘worry’ and ‘sad’ interchangeably to express their negative emotions in a variety of situations. In contrast, people high in emotion differentiation use emotion categories like ‘sad’ and ‘worry’ to differentiate how they feel in different emotional situations. Thus, emotion differentiation does not refer to the richness of one’s emotion vocabulary per se, but rather to the adequate and differentiated use of emotion words targeted to specific situations. Accordingly, emotion differentiation is measured by examining the degree of consistency with which individuals use specific emotion terms, either in response to events that occur in daily life (by methods like experience sampling; Barrett, Citation2004; Erbas, Sels, Ceulemans, & Kuppens, Citation2016), or in response to a variety of emotional stimuli in lab-based experiments (e.g. Erbas, Ceulemans, Lee Pe, Koval, & Kuppens, Citation2014).
Emotion differentiation is closely related to the well-known trait of ‘alexithymia’, which refers to a deficiency in describing and understanding emotions, and a focus on external events rather than internal experiences. Despite the conceptual overlap between emotion differentiation and alexithymia, findings on their relationship at the level of individual differences are mixed. One previous study found no significant relationship (Lundh & Simonsson-Sarnecki, Citation2001), while another study found a consistent negative relationship with one of the three subscales of the Toronto Alexithymia Scale (TAS; Erbas et al., Citation2014). In contrast with alexithymia, which is predominantly seen as a deficiency, emotion differentiation is considered an ability (see Kashdan, Barrett, & McKnight, Citation2015).
The importance of emotion differentiation for one’s well-being has been shown across multiple studies in which a relation between emotion differentiation and psychopathology has been demonstrated (Barrett et al., Citation2001; Emery, Simons, Clarke, & Gaher, Citation2014; Erbas et al., Citation2014; Suvak et al., Citation2011). For example, higher emotion differentiation is related to better emotion regulation capacity (Barrett et al., Citation2001), as well as to lower negative self-esteem, lower intensity of felt negative emotions, less neuroticism and depression, and higher levels of meta-knowledge about emotions (Erbas et al., Citation2014). The overarching explanation for these findings is that the more people are able to make fine-grained distinctions between emotions by the use of different words across situations, the more they are aware of their emotional reactions, and the more they are able to adapt and to regulate their emotions (see Erbas et al., Citationin press; Kashdan et al., Citation2015, for reviews). Although the relationship between emotion differentiation and intrapersonal functioning is well-established, it is still an open question to what extent emotion differentiation also relates to a person’s interpersonal emotion skills.
Emotion recognition
Research on emotion recognition is abundant and has shown that people are able to recognise a variety of prototypical expressions of emotions from facial, vocal, and postural cues (e.g. Ekman, Citation1993; Hawk, Van Kleef, Fischer, & Van Der Schalk, Citation2009; Kret & De Gelder, Citation2013; Sauter, Eisner, Calder, & Scott, Citation2010). This ability to recognise emotions above chance levels has not only been found for so called basic emotions (Ekman, Citation1992), such as happiness, fear, anger, sadness, disgust, and surprise, but also for other emotions, such as embarrassment (e.g. Keltner, Moffitt, & Stouthamer-Loeber, Citation1995), pride (e.g. Tracy & Robins, Citation2004), and contempt (e.g. Ekman & Heider, Citation1988). There is, however, considerable individual variability in the extent to which people are able to recognise target emotions from prototypical displays.
Several factors have been proposed to account for individuals’ differential success in emotion recognition from nonverbal expressions. One line of research has focused on empathic concern, suggesting that greater concern for others should motivate attention to others’ social signals, thereby aiding accurate emotion recognition (Laurent & Hodges, Citation2009; Clements, Holtzworth-Munroe, Schweinle, & Ickes, Citation2007). Another line of research has examined more automatic and spontaneous reactions to other’s emotions via mimicry. The argument is that mimicry of another’s emotional display leads to the simulation of the other’s emotional state, thereby facilitating accurate inferences about the other’s emotional state (e.g. Oberman, Winkielman, & Ramachandran, Citation2007). Findings on the relationship between mimicry and emotion recognition have been inconsistent, however (see Hess & Fischer, Citation2013, Citation2014).
In addition to factors related to empathy, cognitive factors may also relate to emotion recognition. In particular, people may draw on the knowledge that they have about emotions and more specifically, the words that they have available to describe different emotions. For example, Barrett (Citation2012) proposes that both emotional experience and emotion perception depend upon the conceptual system. According to this view, conceptual emotion knowledge shapes the way people perceive emotions in the face (see also Lindquist & Gendron, Citation2013). In addition, Izard and colleagues (Citation2001, Citation2011) have suggested that emotion knowledge, in which the development of language plays a critical role, is crucial for the regulation of emotions, and the ability to recognise and label emotion expressions. Following these perspectives, having a fine-grained set of emotion concepts may not only impact a person’s own emotional experience but also a person’s understanding of other people’s emotions.
The fields of alexithymia and emotional intelligence also point to a relationship between emotion knowledge and emotion recognition. Studies on alexithymia and emotion recognition demonstrate that alexithymia is related to difficulties in detecting negative emotions. More specifically, research has shown that having difficulties in describing emotions is related to inferring less intensity from emotional displays (Parker, Prkachin, & Prkachin, Citation2005; Prkachin, Casey, & Prkachin, Citation2009), and increased reaction times when labelling emotion expressions (Ihme et al., Citation2014). In theory and research on Emotional Intelligence (EI; Mayer, Caruso, & Salovey, Citation2000), emotion knowledge and emotion recognition are conceptualised as two crucial branches, and have been part of measures of EI. One of the most used ability tests of EI, the MSCEIT (Mayer, Salovey, & Caruso, Citation2002) indeed consists of emotion perception tasks as well as emotional understanding tasks, which are highly correlated (see Papadogiannis, Logan, & Sitarenios, Citation2009). Recent studies, however, have cast doubt on the reliability of the MSCEIT (Fiori et al., Citation2014), and additionally, the emotion knowledge branche of the MSCEIT has usually not been used as a separate index. Thus, evidence that individual differences in the use of emotion knowledge are related to the recognition of discrete emotions is currently lacking.
Emotion knowledge can be operationalised in different ways, one of which is the size and use of one’s emotion vocabulary, referred to as emotion differentiation. To date, only one study (Erbas et al., Citation2016) has explicitly addressed the relationship between emotion differentiation in oneself and the perception of emotions from others. Erbas and colleagues (Citation2016) investigated whether emotion differentiation would be related to empathic accuracy in romantic couples. They found that people high in negative (but not positive) emotion differentiation made more accurate inferences about their partners’ reported valence scores (how positive or negative they felt). This study provides preliminary evidence that negative emotion differentiation may affect the accuracy of perceiving other’s emotions. Yet, such a conclusion is still premature, since the participants in Erbas et al. (Citation2016) study were romantic partners. Their findings could therefore also be explained by the possibility that individuals who are high in emotion differentiation may be more inclined to have romantic partners who are expressive, a characteristic that makes it easier to recognise emotions (see Zaki, Bolger, & Ochsner, Citation2008). In addition, recognising feelings of close others (e.g. a friend or romantic partner) is generally easier than recognising those of a complete stranger (e.g. Thomas, Fletcher, & Lange, Citation1997). And finally, Erbas and colleagues examined how accurately participants perceived the valence of their romantic partner’s feeling state. More research is thus needed to answer the question of whether emotion differentiation is associated with the recognition of specific emotions and beyond intimate relationships.
We report two studies that examined this question. Our general hypothesis was that the degree to which people make subtle distinctions between emotions (i.e. emotion differentiation) would be positively related to accurate emotion recognition. In both studies we also examined to what extent emotion differentiation would be related to verbal IQ, because previous research on alexithymia has suggested that verbal intelligence mediates the impairment of emotion recognition of individuals high in alexithymia (see e.g. Montebarocci, Surcinelli, Rossi, & Baldaro, Citation2011). Therefore, we thought that the ability to use different emotion words in different contexts (i.e. emotion differentiation) may also be related to verbal intelligence. Given that emotion differentiation requires specific knowledge about emotions, we expected a low, but significant, correlation between emotion differentiation and verbal intelligence. In Study 1 we measured accurate emotion recognition using a standardised emotion recognition test (see Hawk et al., Citation2009). Study 2 was a preregistered replication and extension of Study 1. Furthermore, Study 2 employed a different sample population and included several different emotion recognition tests.
Study 1: aim
The primary aim of Study 1 was to examine whether there is a positive association between the ability to use specific emotion words across different emotional situations to describe one’s own emotions, and the ability to accurately recognise emotions in other people. We employed an Emotion Recognition (ER) test in which facial expressions of negative emotions were included, to match the measure of negative Emotion Differentiation (ED) previously validated by Erbas et al. (Citation2014). Study 1 tested whether negative emotion differentiation is positively related to negative emotion recognition.
Method
Participants and design
Three hundred ninety-nine individuals participated in a collective testing session at the University of Amsterdam (32% men; Mage = 19, SDage = 2.63). All participants were first year students of psychology. The study was approved by the Ethical Committee of the Faculty of the Social and Behavioral Sciences of the University of Amsterdam. In order to check for an effect of the sequence in which the ER and ED tests were completed on recognition performance, we created two conditions: one in which the ED was administered first (condition 1, N = 200) and one in which the ER was administered first (condition 2, N = 199). The postexperimental observed power was 0.81.
Materials and procedure
Emotion differentiation (ED)
We used the ED task designed by Erbas and colleagues (Citation2014). In this task, participants were asked to rate their emotional reactions to a set of 20 standardised emotional stimuli from the International Affective Picture System (IAPS; Lang, Bradley, & Cuthbert, Citation1995). Each IAPS picture had to be rated on 20 different scales labelled with the following emotion words: anger, anxiety, depression, disgust, embarrassment, envy, fear, guilt, inferior (2 Dutch synonyms), irritation, jealousy, loneliness, nervousness, rage, regret, sadness, shame, unhappiness, worry (in Dutch respectively: boos, vrees, depressief, walging, gêne, afgunstig, angst, schuld, minderwaardig, inferieur, irritatie, jaloers, eenzaam, zenuwachtig, razend, spijt, bedroefd, schaamte, ongelukkig, ongerust). Participants were asked to rate the emotional intensity of each one of these 20 emotions on a 7-point rating scale, ranging between 0 (not at all) and 6 (very much). No time limit was imposed for rating the emotions in the ED task. IAPS pictures were randomly presented to participants; each IAPS picture remained on screen until the next button was pressed and participants moved to the next trial.
An ED Index was computed by calculating, for each participant, the Intra Class Correlation (ICC) between all the ratings of all the pictures. This consistency ICC measure reflects the extent to which the participant used similar terms across the different pictures. Consequently, the higher the index, the more consistent the participant's ratings, representing lower differentiation between the ratings of the different emotional stimuli. In line with previous research (Erbas et al., Citation2014, Citation2016), participants with a negative ICC score (approximately 10%) were excluded from all analyses, because negative ICCs are not interpretable for this index and presumed to reflect random error (Erbas et al., Citationin press; Giraudeau, Citation1996). Next, the ICCs were transformed to Fisher’s Z scores and then reversed in order to be able to interpret the index intuitively, such that a higher index implies higher ED (see Erbas et al., Citation2016 for the same procedure). The mean transformed ICC was .187 (SD = .40).
Amsterdam emotion recognition test (AERT)
We used an ER task that was developed on the basis of stills from the Amsterdam Dynamic Faces Emotional expression Set (ADFES, see Van Der Schalk, Hawk, Fischer, & Doosje, Citation2011). We used 24 photos of low intensity, which are harder to decode than high intensity photos, in order to enhance variability and counter a ceiling effect (Wingenbach, Ashwin, & Brosnan, Citation2016). The photos contained only negative expressions (anger, fear, sadness, embarrassment, contempt and disgust) in line with the negative emotions in the ED task. Four models (2 male and 2 female) displayed each of the 6 emotions. Participants were asked to label the emotion they believed they saw on the face, by ticking one of 6 emotion labels (chance accuracy of 17%), or ‘I do not know’ (see Frank & Stennett, Citation2001). Each photo remained on screen until the next button was pressed and participants moved to the next trial.
Accurate ER was operationalised by calculating the percentage of correct answers across the 24 pictures. The overall recognition rate ranged from 13% to 100%. We also calculated an Unbiased Hit Rate (Hu) score to correct for potentially disproportionate use of some emotion labels (see Wagner, Citation1993). However, as these Hu scores were nearly identical (rp = .95) to the original Raw Hit Rates (H) scores, we used the proportion of correct answers as the measure of accurate emotion recognition.
Verbal intelligence
Because ED is based on the use and knowledge of specific emotion words, there might be a positive relationship between verbal IQ and ED. We used the verbal subscale of the Intelligence Structure Test (IST), a Dutch version of the IST-2000R (Liepmann, Beauducel, Brocke, Amthauer, & Vorst, Citation2010), which is a German test of verbal intelligence (Amthauer, Brocke, Liepmann, & Beauducel, Citation2001) to explore the possible relationship between ED and verbal intelligence. The Verbal IQ consists of three tasks. The first of the three verbal reasoning tasks is Sentence Completion (SC), in which participants have to choose a word from five possible answers to complete a sentence. The second verbal reasoning task is Verbal Analogies (VA). This test presents an analogy in the form a: b = c:?. The participants have to choose the correct final term from a total of five possible words. The third verbal reasoning task is Verbal Similarities (VS) in which participants have to choose two words from a number of six possibilities that are similar to each other in some way. We used the proportion of correct answers across all three tasks as the measure of Verbal IQ. The overall Verbal IQ rate ranged from 21% to 98%.
Procedure
Half of the participants completed the ER test before the ED task, and half of the participants completed the tasks in the opposite sequence. In a separate session, which took place within a month after the original session, all participants completed the Verbal IQ test.
Results
Emotion differentiation (ED)
To examine whether the performance on the ED task was related with verbal intelligence, we calculated the correlation of ED with the verbal IQ test and found a small, but significant positive association, rp = .118, 95%, CI [.013, .231], p < .05, indicating that people who score high on verbal IQ tend to score higher on the ED test as well.
Emotion recognition (ER)
In order to test our main hypothesis that individuals’ ability to differentiate between emotions would predict accurate emotion recognition, we performed a linear multiple regression analysis, with the proportion of correct answers on the AERT as the dependent variable and the ED index as the first predictor. To account for potential order effects, the categorical variable of sequence was dummy-coded (d1: ED-first = 0, ER-first = 1) and entered as additional predictor in the same step as ED. Because the assumption of normality was violated, the significance of all effects was assessed by the bootstrap technique, with 5,000 samples (Efron & Tibshirani, Citation1993). The model was significant and explained 4% of the variance in the emotion recognition task, F(2, 361) = 9.131, p < .001, R2 adj. = .043. The results of the regression analysis (see ) indicated that emotion recognition was better among participants who differentiated more between emotional words. Controlling for emotional intensity levels (i.e. average rating of negative emotions on the ED task) as a covariate, did not change the significant relationship between ED and emotion recognition (for more details, see the supplemental materials Table 1). In addition, emotion recognition was higher when participants had completed the AERT before, rather than after the ED task.
Table 1. Results of linear multiple regression analysis to predict accurate emotion recognition (AERT), based on sequence and emotion differentiation index (Study 1, N = 363).
Discussion
Study 1 provides initial support for the notion that differentiating between one’s own feelings and making sense of others’ feelings are connected. Individuals who made finer distinctions between their own negative emotions when watching emotional pictures were also better at recognising others’ facial expressions of specific emotional states. This finding, using a standardised recognition test, extends previous results from Erbas et al. (Citation2016) where participants predicted the valence of their romantic partner’s feelings. Thus, the current findings suggest that emotion differentiation is not only positively related to understanding the broad feeling states of familiar others, but also to accurately recognising specific emotional states of unfamiliar others.
We unexpectedly found a small effect of sequence on emotion recognition, such that when the emotion recognition task was administered first, recognition rates were higher. We think this may have been due to reduced concentration when the emotion recognition task was administered second. We therefore consistently administered all ER tests first in Study 2 and further kept the order constant.
Study 2
In Study 1 we found that participants who were better at differentiating their own emotions were also more accurate in recognising the emotions of the target models in the Amsterdam Emotion Recognition Test. The aim of Study 2 was to replicate these findings with (1) a more representative sample and (2) with different emotion stimuli. We therefore included participants with a different nationality, age group, and cultural background by recruiting participants on MTurk, focusing on American English-speaking participants with a broad age range. Second, we sought to include a wider range of emotional stimuli. The AERT consists of standardised, posed stimuli, which, even at low intensity, are moderately easy to recognise (e.g. Fischer, Kret, & Broekens, Citation2018). In order to examine whether the effect we found in Study 1 was merely due to the nature of the stimuli, we included another ER task, the Geneva Emotion Recognition Test (GERT), which uses different channels of nonverbal expressions (face, body, and voice). We further hypothesised that individuals may make more use of emotion knowledge when there is less emotion information available, that is, when expressive cues are minimal. This is the case in the Reading the Mind in the Eyes Test (RMET), which consists of complex stimuli with minimal emotional information (only eyes). These stimuli are paired with labels of difficult, infrequently used words referring to both emotional states and mental states (Engel, Woolley, Jing, Chabris, & Malone, Citation2014). It has therefore been argued that RMET is strongly related to vocabulary and should be considered as a test of conceptual and verbal knowledge rather than a perception task (Olderbak et al., Citation2015). In contrast, both the AERT and the GERT provide rich emotional information and the response alternatives require less complex conceptual knowledge. We included the RMET, expecting that it would be more difficult than both the AERT and the GERT and that ED should be most strongly associated with the performance on the RMET. The study, including hypotheses, exclusion criteria, and analysis plan, was preregistered at OSF (see osf.io/y76rq).
Method
Participants
Participants were 245 US citizens who were recruited via Amazon Mechanical Turk (Mturk), an online crowdsourcing platform. Our target sample was 200 participants, based on an a-priori power analysis showing that a sample of 200 is sufficient to establish correlations of .20 with a power of .80. We collected an additional 45 participants to allow us to exclude people who did not meet the following criteria: a) having spent a minimal amount of time (15 min) on the questionnaire, b) having English as native language (in order to control for differences in language and use of emotion terms); c) participants with a negative ICC, because negative ICCs reflect random error (see Erbas et al., Citation2014, Citation2016). Based on these criteria, we excluded 28 participants, who were omitted before all statistical analyses. This yielded a sample of 217 participants (47% men; Mage = 37, SDage = 12). The description of the study was “View people in various situations and rate their emotions”. Each participant received 3$ in compensation for their time.
Design and procedure
Study 2 used the same design as Study 1, with the following change. First, participants completed three emotion recognition tests: the Amsterdam Emotion Recognition Task (AERT), the Reading the Mind in the Eyes Test (RMET), and the Geneva Emotion Recognition Test (GERT). Because of the sequence effect in Study 1, the three ER tasks were administered first (in randomised order), after which we administered the ED task, and the Verbal IQ task.Footnote1
Measures
Amsterdam emotion recognition test (AERT)
This was the same test as in Study 1. The overall recognition rate in Study 2 ranged from 8% to 100%.
Geneva emotion recognition test (GERT)
We used the short version of the Geneva Emotion Recognition Test (Schlegel, Grandjean, & Scherer, Citation2014). The test consists of 42 short video clips with sound (duration 1–3 s), in which ten professional Caucasian actors (five male, five female) express 14 different positive and negative emotions: joy, amusement, pride, pleasure, relief, interest, surprise, anger, fear, despair, irritation, anxiety, sadness, and disgust. In each video clip, the actors are visible from their upper torso upward (conveying facial and postural/gestural emotional cues) and pronounce a sentence made up of syllables without semantic meaning (conveying emotional cues through the intonation in their voice). After each clip, participants were asked to choose which of the 14 emotions best described the emotion the actor intended to express (chance accuracy of 7%). Responses were scored as correct (1) or incorrect (0). Then, similar to AERT, the final GERT score was calculated as the percentage of accurate trials. The range of accuracy on this task was 7% to 83%.
Reading the Mind in the eyes test (RMET)
The RMET comprises 36 photographs depicting the eye region of 36 Caucasian posers (Baron-Cohen, Wheelwright, Hill, Raste, & Plumb, Citation2001). Participants are asked to identify the emotional state of a target person, whose eye region is shown in a photograph, by choosing between four words that each represent an emotional state (chance accuracy of 25%). Responses are scored as correct (1) or incorrect (0); the RMET score is calculated by summing the correct answers. Similar to the other ER tests (AERT, GERT), performance on RMET was determined by calculating the percentage of correct responses. The average recognition rate ranged between 17% and 100%.
Verbal intelligence (Verbal IQ)
We used The Shipley Vocabulary Test (Shipley, Citation1940). For each item, participants are instructed to decide which of four words is most similar to a prompted word. Verbal IQ was estimated by calculating the percentage of correct answers.
Results
Preliminary analysis
Emotion differentiation
We calculated the ED index in the same way as in Study 1. Next, we computed the correlation of ED with the verbal IQ test and found, similarly to Study 1, a significant positive association, rp = .298, 95%CI [.126, .449], p < .001. This pattern indicates that people who have high verbal IQ tend to score higher on ED.
Emotion recognition
To test whether individuals’ ability to differentiate between their own experienced emotions was associated with accurate emotion recognition, we conducted one simple regression analysis for each of the three DVs (AERT, GERT, RMET), with ED index as the predictor in each analysis. Similar to Study 1, the significance of all effects was assessed using bootstrap technique with 5,000 samples to overcome violations of normality. All three models were significant: AERT: F(1, 215) = 14.295, p < .001; GERT: F(1, 215) = 12.565, p < .001; RMET: F(1, 215) = 24.935, p < .001. The variance in ER scores explained by ED ranged between 5 and 10% (see for all statistics). The results of the regression analyses clearly indicate that ED is positively related to ER across different emotion recognition tests (see for illustration). Furthermore, the correlation between ED and ER remained the same when controlling for the intensity levels (for more details, see the supplemental materials Table 2).
Figure 1. Illustration of the linear relation between ED and the AERT (Study 1: a, Study 2; b), the GERT (Study 2: c) and the RMET (Study 2: d). R2 refers to the percentage of explained variance for each of the models.
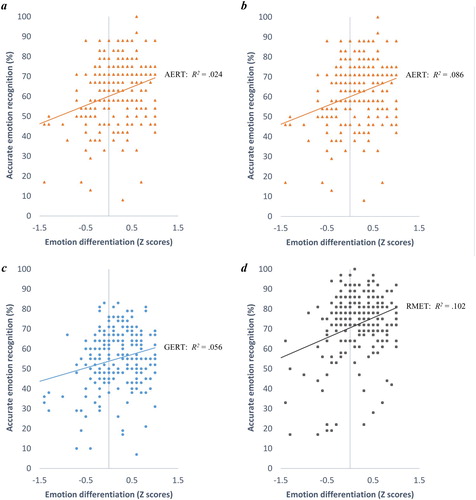
Table 2. Standardised weights of ED in predicting accurate emotion recognition on AERT, GERT, RMET in separate Linear Simple Regression analyses (Study 2, N = 217).
We did not find support for the hypothesis that ED is more strongly associated with accurate emotion recognition in the test with the least emotion information (only eyes: RMET), compared with the test with most elaborate emotional stimuli (face [AERT] and face, body and voice [GERT]). The difference in correlations between each pair of ED and ER test (RMET, AERT and GERT) was not significant for the linear trend, all Z < −1.5, ns (calculation based on Hittner, May, & Silver, Citation2003).
Discussion
Study 2 provided further support for the idea that better ED is positively associated with more accurate emotion recognition. This finding was similar to the pattern observed in Study 1, again suggesting that better emotion differentiation is generally associated with more accurate emotion recognition. Contrary to our expectation, the (linear) relationship between emotion differentiation and emotion recognition was similar across all recognition tests (but see supplementary materials for exploratory analysis of a quadratic trend). This finding is unexpected, given that these tests require different amounts of verbal knowledge. The RMET consists of complex stimuli with minimal emotional information (only eyes). In contrast, the GERT provides rich emotional information and uses fewer complex words. The absence of differences between the tests thus suggest that the effect of emotion differentiation on emotion recognition can be generalised and does not rely on the specific type of task or use of more or less complex emotion labels. This conclusion of further supported by our finding that the relationship between ED and ER remains significant even when controlling for individual differences in verbal IQ, although only for the RMET and the AERT (see Supplementary Materials).
General Discussion
The main goal of the current research was to examine the role of emotion differentiation in the recognition of others’ emotions. Emotion differentiation reflects the extent to which people make fine-grained distinctions between emotions by using different emotion words in reporting their feelings in reaction to different emotional situations. Consistently across two studies, we found that negative emotion differentiation was associated with better recognition of others’ emotions, as indicated by performance on several different emotion recognition tests. This effect was small, but robust across two samples of participants with different cultural backgrounds, language, and age.
Previous studies have found that emotion differentiation is positively related to well-being, and negatively related to stress, negative emotions and emotion pathology (Barrett, Citation2004; Barrett et al., Citation2001; Erbas et al., Citation2014). Notwithstanding the important role ascribed to emotion differentiation with regard to intrapersonal processes, the role of emotion differentiation in interpersonal functioning has received less attention. To date, only one study (Erbas et al., Citation2016) has examined the relationship between emotion differentiation and emotion recognition. The current results are in line with those of Erbas and colleagues (Citation2016) in establishing a positive relationship between emotion differentiation and emotion recognition. However, our findings go beyond previous research in two ways. Erbas et al. (Citation2016) found that emotion differentiation predicts accurate inferences about partners’ reported valence scores. However, it is possible that people who make finer distinctions between their own emotions have romantic partners who are more expressive, which makes it easier to recognise their partners’ emotions (see Zaki et al., Citation2008). Thus, the first contribution of the current research is to establish that the positive relation between emotion differentiation and emotion recognition can be generalised beyond romantic relationships. Furthermore, in order to show empathy, it is important to recognise whether the other feels bad (i.e. to infer negative affective valence), but it is arguably equally important to identify the specific emotion (e.g. sadness, anger, or disappointment) in order to be able to respond adequately. The current findings provide the first evidence that emotion differentiation relates to the accuracy of the recognition of specific emotions.
Secondly, past research has addressed the importance of emotion differentiation in the intra-personal domain. Accordingly, the negative relation of emotion differentiation to stress and emotional pathology (Barrett, Citation2004; Barrett et al., Citation2001; Erbas et al., Citation2014; Erbas et al., Citationin press) was interpreted as an indication that negative emotions and stressful experiences can be affected by people’s emotion-differentiation skill (Kashdan et al., Citation2015). Consistent with Erbas et al. (Citation2016), we suggest that the inter-personal domain may be an additional pathway that explains why individuals with low emotion differentiation suffer from poor well-being – because they do not understand the emotional cues of the social word around them. We believe that these pathways are complementary; future research should gain more insight into the estimation of their relative contributions.
Limitations
The current research has several limitations. First, the use of a correlational design allowed us to establish the relationship between emotion differentiation and emotion recognition. However, experimental research will be needed to illuminate whether emotion differentiation predicts emotion recognition or vice versa. A second limitation is that the current studies only examined negative emotion differentiation. We did not assess differentiation of positive emotions, because we wanted to use validated measures, and there is presently no validated measure of positive emotion differentiation. However, there is growing interest in the field of emotion to differentiate between discrete positive emotions (Sauter, Citation2017; Shiota et al., Citationin press) and it will be important to test the extent to which positive and negative emotion differentiation converge. A third limitation is that, as with many standardised tests, the emotional expressions we used to evaluate accurate recognition were posed by actors. Although there is evidence that recognition of posed and spontaneous facial expressions overlaps considerably (Sauter & Fischer, Citation2018), more research with emotional expressions in real life situations may provide additional insight into the role of emotion differentiation in emotion recognition. This work may also include other kinds of emotional expressions, such as speech content.
Conclusion
The ability to express one’s feelings in words with a high degree of differentiation is an important predictor of subjective well-being. However, to date, it has remained unclear whether emotion differentiation also relates to interpersonal processes. The current research provides compelling evidence that, across multiple samples and emotion recognition tests, people who make fine-grained differentiations between emotion words in relation to themselves are also better at recognising the feelings of others from nonverbal expressions. Being able to understand one’s own emotions in a differentiated way is thus linked to accurately interpreting the emotions of others, an important component of empathy. Knowing how we feel ourselves may thus pave the way to understanding others, and thereby bring us closer to those around us.
Supplementary_Materials.docx
Download MS Word (732.8 KB)Disclosure statement
No potential conflict of interest was reported by the authors.
ORCID
Jacob Israelashvili http://orcid.org/0000-0003-1289-223X
Notes
1 Participants also completed Davis’ (Citation1983) Interpersonal Reactivity Index, a self-report measure of trait empathy. Full description of means, standard deviations and correlations between the IRI subscales and all measures in Study 2 can be found in Supplementary Materials Table 4.
References
- Amthauer, R., Brocke, B., Liepmann, D., & Beauducel, A. (2001). Intelligenz-Struktur-Test2000 R [Intelligence Structure Test 2000 R]. Göttingen: Hogrefe.
- Baron-Cohen, S., Wheelwright, S., Hill, J., Raste, Y., & Plumb, I. (2001). The “Reading the Mind in the Eyes” test revised version: A study with normal adults, and adults with Asperger syndrome or high-functioning autism. Journal of Child Psychology and Psychiatry, 42(2), 241–251.
- Barrett, L. F. (2004). Feelings or words? Understanding the content in self-report ratings of experienced emotion. Journal of Personality and Social Psychology, 87(2), 266–281.
- Barrett, L. F. (2012). Emotions are real. Emotion, 12(3), 413.
- Barrett, L. F., Gross, J., Christensen, T. C., & Benvenuto, M. (2001). Knowing what you're feeling and knowing what to do about it: Mapping the relation between emotion differentiation and emotion regulation. Cognition & Emotion, 15(6), 713–724.
- Clements, K., Holtzworth-Munroe, A., Schweinle, W., & Ickes, W. (2007). Empathic accuracy of intimate partners in violent versus nonviolent relationships. Personal Relationships, 14(3), 369–388.
- Davis, M. H. (1983). Measuring individual differences in empathy: Evidence for a multidimensional approach. Journal of Personality and Social Psychology, 44(1), 113–126.
- Efron, B., & Tibshirani, R. (1993). An introduction to the bootstrap (Monographs on statistics and applied probability 57). Boca Raton, FL: Chapman & Hall/CRC.
- Ekman, P. (1992). An argument for basic emotions. Cognition & Emotion, 6(3–4), 169–200.
- Ekman, P. (1993). Facial expression and emotion. American Psychologist, 48(4), 384–392.
- Ekman, P., & Heider, K. G. (1988). The universality of a contempt expression: A replication. Motivation and Emotion, 12(3), 303–308.
- Emery, N. N., Simons, J. S., Clarke, C. J., & Gaher, R. M. (2014). Emotion differentiation and alcohol-related problems: The mediating role of urgency. Addictive Behaviors, 39(10), 1459–1463.
- Engel, D., Woolley, A. W., Jing, L. X., Chabris, C. F., & Malone, T. W. (2014). Reading the mind in the eyes or reading between the lines? Theory of mind predicts collective intelligence equally well online and face-to-face. PloS One, 9(12), e115212.
- Erbas, Y., Ceulemans, E., Blanke, E., Sels, L., Fischer, A. H., & Kuppens, P. (in press). Emotion differentiation dissected: Between-category, within-category, and integral emotion differentiation, and their relation to well-being. Cognition and Emotion. doi:10.1080/02699931.2018.1465894
- Erbas, Y., Ceulemans, E., Lee Pe, M., Koval, P., & Kuppens, P. (2014). Negative emotion differentiation: Its personality and well-being correlates and a comparison of different assessment methods. Cognition & Emotion, 28(7), 1196–1213.
- Erbas, Y., Sels, L., Ceulemans, E., & Kuppens, P. (2016). Feeling Me, Feeling You: The relation between emotion differentiation and empathic accuracy. Social Psychological and Personality Science, 7(3), 240–247.
- Fiori, M., Antonietti, J. P., Mikolajczak, M., Luminet, O., Hansenne, M., & Rossier, J. (2014). What is the ability emotional intelligence test (MSCEIT) good for? An evaluation using item response theory. PLoS One, 9(6), e98827.
- Fischer, A. H., Kret, M. E., & Broekens, J. (2018). Gender differences in emotion perception and self-reported emotional intelligence: A test of the emotion sensitivity hypothesis. PloS one, 13(1), e0190712.
- Frank, M. G., & Stennett, J. (2001). The forced-choice paradigm and the perception of facial expressions of emotion. Journal of Personality and Social Psychology, 80(1), 75–85.
- Giraudeau, B. (1996). Negative values of the intraclass correlation coefficient are not theoretically possible. Journal of Clinical Epidemiology, 49, 1205–1206.
- Hawk, S. T., Van Kleef, G. A., Fischer, A. H., & Van Der Schalk, J. (2009). “Worth a thousand words”: Absolute and relative decoding of nonlinguistic affect vocalizations. Emotion, 9(3), 293–305.
- Hess, U., & Fischer, A. (2013). Emotional mimicry as social regulation. Personality and Social Psychology Review, 17(2), 142–157.
- Hess, U., & Fischer, A. (2014). Emotional mimicry: Why and when we mimic emotions. Social and Personality Psychology Compass, 8(2), 45–57.
- Hittner, J. B., May, K., & Silver, N. C. (2003). A Monte Carlo evaluation of tests for comparing dependent correlations. The Journal of General Psychology, 130(2), 149–168.
- Ihme, K., Sacher, J., Lichev, V., Rosenberg, N., Kugel, H., Rufer, M., … Villringer, A. (2014). Alexithymia and the labeling of facial emotions: Response slowing and increased motor and somatosensory processing. BMC Neuroscience, 15(1), 40.
- Izard, C., Fine, S., Schultz, D., Mostow, A., Ackerman, B., & Youngstrom, E. (2001). Emotion knowledge as a predictor of social behavior and academic competence in Children at risk. Psychological Science, 12(1), 18–23.
- Izard, C. E., Woodburn, E. M., Finlon, K. J., Krauthamer-Ewing, E. S., Grossman, S. R., & Seidenfeld, A. (2011). Emotion knowledge, emotion utilization, and emotion regulation. Emotion Review, 3(1), 44–52.
- Kashdan, T. B., Barrett, L. F., & McKnight, P. E. (2015). Unpacking emotion differentiation: Transforming unpleasant experience by perceiving distinctions in negativity. Current Directions in Psychological Science, 24(1), 10–16.
- Keltner, D., Moffitt, T. E., & Stouthamer-Loeber, M. (1995). Facial expressions of emotion and psychopathology in adolescent boys. Journal of Abnormal Psychology, 104, 644–652.
- Kret, M. E., & De Gelder, B. (2013). When a smile becomes a fist: The perception of facial and bodily expressions of emotion in violent offenders. Experimental Brain Research, 228(4), 399–410.
- Lang, P. J., Bradley, M. M., & Cuthbert, B. N. (1995). The International Affective Picture System (IAPS). Gainesville: University of Florida. Center for Research in Psychophysiology, 893–899.
- Laurent, S. M., & Hodges, S. D. (2009). Gender roles and empathic accuracy: The role of communion in reading minds. Sex Roles, 60(5–6), 387–398.
- Liepmann, D., Beauducel, A., Brocke, B., Amthauer, R., & Vorst, H. (2010). IST – Intelligentie-Structuur-Test. Amsterdam: Hogrefe Uitgevers.
- Lindquist, K. A., & Gendron, M. (2013). What’s in a word? Language constructs emotion perception. Emotion Review, 5(1), 66–71.
- Lundh, L.-G., & Simonsson-Sarnecki, M. (2001). Alexithymia, emotion, and somatic complaints. Journal of Personality, 69, 483–510.
- Mayer, J. D., Caruso, D. R., & Salovey, P. (2000). Selecting a measure of emotional intelligence: The case for ability scales.
- Mayer, J. D., Salovey, P., & Caruso, D. R. (2002). Mayer-Salovey-Caruso emotional intelligence test: MSCEIT. Item booklet. MHS.
- Montebarocci, O., Surcinelli, P., Rossi, N., & Baldaro, B. (2011). Alexithymia, verbal ability and emotion recognition. Psychiatric Quarterly, 82(3), 245–252.
- Oberman, L. M., Winkielman, P., & Ramachandran, V. S. (2007). Face to face: Blocking facial mimicry can selectively impair recognition of emotional expressions. Social Neuroscience, 2, 167–178.
- Olderbak, S., Wilhelm, O., Olaru, G., Geiger, M., Brenneman, M. W., & Roberts, R. D. (2015). A psychometric analysis of the reading the mind in the eyes test: Toward a brief form for research and applied settings. Frontiers in Psychology, 6, Article no. 1503.
- Papadogiannis, P. K., Logan, D., & Sitarenios, G. (2009). An ability model of emotional intelligence: A rationale, description, and application of the Mayer Salovey Caruso emotional intelligence test (MSCEIT). In Assessing emotional intelligence (pp. 43–65). Boston, MA: Springer.
- Parker, P. D., Prkachin, K. M., & Prkachin, G. C. (2005). Processing of facial expressions of negative emotion in alexithymia: The influence of temporal constraint. Journal of Personality, 73(4), 1087–1107.
- Prkachin, G. C., Casey, C., & Prkachin, K. M. (2009). Alexithymia and perception of facial expressions of emotion. Personality and Individual Differences, 46(4), 412–417.
- Sauter, D. A. (2017). The nonverbal communication of positive emotions: An emotion family approach. Emotion Review, 9(3), 222–234.
- Sauter, D. A., Eisner, F., Calder, A. J., & Scott, S. K. (2010). Perceptual cues in nonverbal vocal expressions of emotion. Quarterly Journal of Experimental Psychology, 63, 2251–2272.
- Sauter, D. A., & Fischer, A. H. (2018). Can perceivers recognise emotions from spontaneous expressions? Cognition and Emotion, 32(3), 504–515.
- Schlegel, K., Grandjean, D., & Scherer, K. R. (2014). Introducing the Geneva emotion recognition test: An example of Rasch-based test development. Psychological Assessment, 26(2), 666–672.
- Shiota, M. N., Campos, B., Oveis, C., Hertenstein, M., Simon-Thomas, E., & Keltner, D. (in press). Beyond happiness: Toward a science of discrete positive emotions. Manuscript accepted for publication in American Psychologist.
- Shipley, W. C. (1940). A self-administering scale for measuring intellectual impairment and deterioration. The Journal of Psychology, 9(2), 371–377.
- Smidt, K. E., & Suvak, M. K. (2015). A brief, but nuanced, review of emotional granularity and emotion differentiation research. Current Opinion in Psychology, 3, 48–51.
- Suvak, M. K., Litz, B. T., Sloan, D. M., Zanarini, M. C., Barrett, L. F., & Hofmann, S. G. (2011). Emotional granularity and borderline personality disorder. Journal of Abnormal Psychology, 120(2), 414–426.
- Thomas, G., Fletcher, G. J., & Lange, C. (1997). On-line empathic accuracy in marital interaction. Journal of Personality and Social Psychology, 72(4), 839–850.
- Tracy, J. L., & Robins, R. W. (2004). Show your pride: Evidence for a discrete emotion expression. Psychological Science, 15(3), 194–197.
- Van Der Schalk, J., Hawk, S. T., Fischer, A. H., & Doosje, B. (2011). Moving faces, looking places: Validation of the Amsterdam Dynamic facial expression Set (ADFES). Emotion, 11(4), 907–920.
- Wagner, H. L. (1993). On measuring performance in category judgment studies of nonverbal behavior. Journal of Nonverbal Behavior, 17(1), 3–28.
- Wingenbach, T. S. H., Ashwin, C., & Brosnan, M. (2016). Validation of the Amsterdam Dynamic facial expression Set – Bath intensity Variations (ADFES-BIV): A Set of Videos Expressing Low, Intermediate, and high intensity emotions. PLoS ONE, 11(1), e0147112.
- Zaki, J., Bolger, N., & Ochsner, K. (2008). It takes two: The interpersonal nature of empathic accuracy. Psychological Science, 19(4), 399–404.