ABSTRACT
This special issue of Cognition and Emotion assembles recent advances in theorising and empirical research on the automaticity of evaluative learning. Based on a taxonomy of automatic processes in evaluative learning, we distinguish between processes that are involved in translating evaluative experiences into evaluative mental representations (acquisition), and processes that translate these representations into evaluative biases in perception, thought, and action (activation and application). We emphasise that automaticity concerns the operating conditions of these processes (unawareness, unintentionality, uncontrollability, efficiency), not their operating principles, and thus can vary within specific processes (e.g. inferences can occur in either an automatic or non-automatic fashion). We review and discuss contemporary theories and methodological approaches to automatic processes in evaluative learning against the backdrop of our framework, and we highlight the contributions of the papers of this special issue to the question whether and when evaluative changes can occur in an automatic manner.
The study of attitudes and evaluations has been a focal topic of psychology for almost a century for both its theoretical and explanatory value for behaviour (Allport, Citation1935). In particular, the possibility that the acquisition and retrieval of evaluations may operate outside of awareness, with little effort, without our intention, or even in an uncontrollable manner has intrigued many generations of researchers. Several theories postulate that automatic processes contribute to the acquisition or change of evaluations (e.g. Gawronski & Bodenhausen, Citation2006; Rydell & McConnell, Citation2006). Much research has been devoted to studying the measurement and the induction of evaluations that can be characterised as automatic. Moreover, the methodological difficulties underlying this research endeavour have received high scrutiny (e.g. Bargh, Citation1994; Melnikoff & Bargh, Citation2018; Moors & De Houwer, Citation2006; Sweldens, Tuk, & Hütter, Citation2017).
The issue of automaticity is at the core of recent theorising on evaluative learning, yet empirical research has not yet provided strong evidence for automaticity claims (see Corneille & Stahl, Citation2019, for a review), and the implications of many of these findings are still unclear. Only by critically addressing questions of automaticity with regard to its identification and assessment in evaluative learning, we will advance research and theory development on automaticity in evaluative learning substantially.
In this editorial, we set the stage for this special issue by developing a taxonomy of processes that are involved in the acquisition and activation or application of evaluative representations. Based on this taxonomy, we discuss contemporary theoretical and methodological approaches in the study of automatic evaluative learning, as well as their advantages and limitations. Next, we outline the contents of this special issue and summarise the articles’ implications. Finally, from this discussion we derive several avenues for future research.
A taxonomy of processes involved in the acquisition and activation or application of evaluative representations
Evaluative learning essentially involves a change in evaluative mental representations that is due to experience. As mental representations are hypothetical constructs and cannot be observed directly, investigating evaluative change also requires an assessment of evaluative biases in perception, thought, or action in order to establish evaluative learning effects. represents the transitions from evaluative experience to mental representations (acquisition), and from mental representations to manifest evaluative biases (activation/application).
Figure 1. A two-stage model of evaluative learning: Taxonomy of processes translating evaluative experiences into mental representations of evaluative change during acquisition (Stage 1), and retrieving mental representations of evaluation during evaluative assessment (Stage 2).
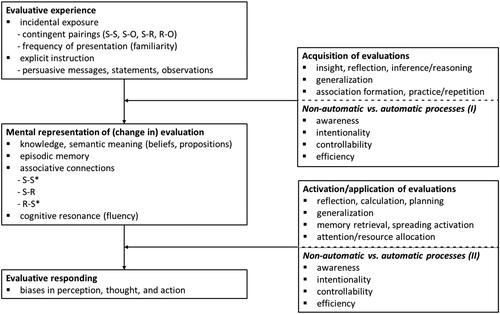
Research has relied on a large range of evaluative experiences to study evaluative learning, comprising mere exposure to certain stimuli (frequency of presentation of a stimulus object; Zajonc, Citation1968, Citation1980), inhibition of distracting or irrelevant stimuli (Fenske & Raymond, Citation2006; Raymond, Fenske, & Tavassoli, Citation2003; Raymond, Fenske, & Westoby, Citation2005), exposure to contingent pairings between stimuli and other evaluative stimuli (evaluative conditioning; De Houwer, Citation2007), between stimuli and evaluative responses (e.g. approach-avoidance training; Huijding, Muris, Lester, Field, & Joosse, Citation2011; Woud, Maas, Becker, & Rinck, Citation2013; evaluative stimulus-response learning; Gast & Rothermund, Citation2011a, Citation2011b; valence contingency learning; Schmidt & De Houwer, Citation2012; see also Gast, Richter, & Ruszpel, Citation2020 this issue), between responses and evaluative stimuli (e.g. emotional action-effect learning, Eder & Rothermund, Citation2013), and exposure to information regarding evaluative characteristics of the relevant object (e.g. persuasion, Petty & Briñol, Citation2012; evaluative learning by mere instructions, De Houwer, Citation2006).
When evaluative learning occurs, these evaluative manipulations and experiences are assumed to result in (changes in) mental representations of stimuli or objects. Different types of mental representations of evaluative learning have been assumed, ranging from semantic knowledge (attitudes and beliefs) and memory for certain facts or episodes to less propositional forms of evaluative representations comprising associations between stimuli and evaluative concepts or valent stimuli (S-S*; Fazio, Citation2001), between stimuli and evaluative responses (S-R; Gast & Rothermund, Citation2011a, Citation2011b), between actions and outcomes (R-S*; Colwill & Rescorla, Citation1986; Eder & Rothermund, Citation2013), or changes in the cognitive resonance and ease of processing of certain stimuli (“fluency”; Reber, Winkielman, & Schwarz, Citation1998; “inhibition”; Raymond et al., Citation2003, Citation2005).
The final criterion for evaluative learning is its effect on observable consequences in perception, thought, and action. The scope of possible outcome measures that can be used to detect these evaluative influences is extremely broad, ranging from directly assessed evaluative statements and judgments to indirectly assessed evaluative biases in perception and behaviour.
As outlined in , questions of automaticity refer to the processes involved in (a) the acquisition (i.e. encoding and consolidation) or (b) the activation, retrieval, and application of evaluations. The acquisition of evaluations regards the processes that are involved in translating evaluative experience into evaluative mental representations. Qualitatively distinct processes have been proposed in this regard, ranging from reflection and inference to association formation and increases in familiarity. Although it is tempting to do so, researchers should not equate the nature or quality of the learning process (i.e. its operating principles) with its operating conditions (see Gawronski & Bodenhausen, Citation2009). The question of automaticity versus controllability depends on processing characteristics like (un-)awareness, (un-)controllability, (un-)intentionality, or (in-)efficiency (Bargh, Citation1994; Moors & De Houwer, Citation2006). Rather than concluding that, for instance, inferential processes are always non-automatic, this is in fact an empirical question (see, e.g. De Houwer, Citation2018).
Similarly, different types of processes have been suggested that can be involved in activating evaluative representations, and in translating the resulting activation into evaluative biases in perception, thought, and action. Reflective processes (planning, calculation of optimal outcomes based on evaluative means-end relations, construction of meaning, developing of efficient behavioural strategies) can play a role in translating evaluative mental representations into evaluative biases, but processes like spreading activation connecting mental representations of objects and actions with evaluative meanings, or episodic retrieval, attention or resource allocation may also be involved. Again, the nature of the process is not to be equated with its operating conditions, because in principle, one and the same process may operate in both an automatic and non-automatic fashion (e.g. memory retrieval may occur spontaneously or as a result of a directed effort to retrieve information; behavioural strategies in a task may result from consciously deciding on a strategy or from automatically capitalising on redundancies and contingencies of which the person may not necessarily become aware).
At first sight, processes of activation and application may seem to be unrelated to evaluative learning proper, because they are located at a later stage, when the exposure to evaluative experiences and its translation into mental representations has already been completed. Although we emphasise that it is important to distinguish the two stages conceptually (and possibly also methodologically), we want to highlight that both stages are closely connected to learning. For instance, experimental manipulations employed during acquisition might affect acquisition, activation/application, or both stages. For example, employing a secondary task during acquisition as a means of reducing the cognitive resources available to the learner could influence evaluative responses by affecting processes operating during acquisition, processes operating at retrieval, or both (i.e. the manipulation affects the representation and the way it is retrieved). Moreover, learning and testing situations may interact. In particular, (changes in) evaluations may not have occurred during the learning episode, but may be instigated only in response to a new situation. For example, in some cases, the learning phase may not yet have resulted in the acquisition of generalised evaluations. Specifically, the learning phase may have altered the representation of individual stimuli, but only by testing evaluations of the stimulus category, a generalised evaluation will be formed. Likewise, a learning procedure may increase the familiarity of a stimulus, but the translation of familiarity into either positive or negative evaluations may depend on the test context highlighting either novelty or familiarity as desirable.
Empirically, it may thus be difficult to clearly distinguish between processes that affect either acquisition or activation and application. Because mental representations cannot be observed directly, every instance of assessing effects of evaluative learning necessarily comprises both, a learning manipulation (acquisition stage), and an assessment of its effects (application stage). It may seem natural to assume that automatic and non-automatic acquisition processes should result in mental representations that will typically be used for automatic and non-automatic processes of activation and application, respectively. However, this correspondence is an empirical assumption, not a logical necessity. It is easy to conceive of situations in which automatic acquisition processes result in mental representations that will later also influence non-automatic application processes, and vice versa (e.g. De Houwer, Citation2006; Pleyers, Corneille, Luminet, & Yzerbyt, Citation2007; Pleyers, Corneille, Yzerbyt, & Luminet, Citation2009).
To summarise the implications of our conceptual taxonomy (see ), we argue that claims of automaticity regarding the processes of evaluative learning can refer to the acquisition or activation and application stages. Specifying exactly which transition is meant when the automaticity question is raised is an important step for (a) finding theoretical justifications of the respective claim, (b) for developing appropriate measures to evaluate the claim, and (c) for discussing the theoretical and practical implications of a study.
Theories on automaticity in evaluative learning
In virtually all evaluative learning paradigms mentioned above, debates are ongoing about whether the processes underlying evaluative learning can be characterised by automaticity features. The most intensely researched paradigm is evaluative conditioning. Evaluative conditioning (EC) consists in an effect that a pairing of a neutral, conditioned stimulus (CS) with a valent, unconditioned stimulus (US) has on its evaluation. Early theories postulated an association formation between CS and US that is based merely on their spatio-temporal contiguity (Baeyens, Eelen, Crombez, & Van den Bergh, Citation1992; see also Hofmann, De Houwer, Perugini, Baeyens, & Crombez, Citation2010). This association-formation account subscribes to automaticity features such as unawareness. A more recent theory suggests a mechanism of implicit misattribution of affect (Jones, Fazio, & Olson, Citation2009; Jones, Olson, & Fazio, Citation2010). Such a misattribution of the valence of the US to the CS is thought to operate without individuals even realising that an attribution process is taking place. Thus, such a process can be characterised by automaticity features such as unawareness and unintentionality. Yet another account attributes EC effects to the formation of associations between the CS and an evaluative response that is typically elicited by the US (S-R learning; Gast & Rothermund, Citation2011a, Citation2011b). As processes of S-R learning are typically assumed to reflect a more basic, non-cognitive form of conditioning, these authors assume that evaluative changes reflecting S-R learning are automatic (see also Sweldens, van Osselaer, & Janiszewski, Citation2010). Finally, inspired by propositional accounts of learning (De Houwer, Citation2018; Mitchell, De Houwer, & Lovibond, Citation2009), some theorists claim that EC effects reflect the operation of propositional processes that endow the CS-US pairings with a specified meaning (e.g. Fiedler & Unkelbach, Citation2011; Förderer & Unkelbach, Citation2012; Hughes, Ye, Van Dessel, & De Houwer, Citation2019). Contrary to the standard claim that is made by dual-process accounts, which is to equate propositional processes with non-automatic processes, some proponents of propositional learning allow for the possibility that propositional processes can also operate in an automatic fashion (De Houwer, Citation2018). In our view, the distinction between processes and their operating conditions is an important one (Gawronski & Bodenhausen, Citation2009). That is, one should not infer operating principles from the conditions under which the respective processes occur. By contrast, it may be wise to consider the possibility that most processes can operate both under automatic and non-automatic conditions. From this perspective, neither of the claims regarding the underlying processes in EC necessitates a strong implication regarding the (non-)automaticity of evaluative learning. Nevertheless, it is desirable from an empirical perspective to make assumptions about the operating conditions of specific operating principles. The better the processes and their operating conditions are specified, the more valuable a theory is. In particular, the falsifiability of a theory constitutes an important premise for the design of research allowing for strong inference (Platt, Citation1964).
The prominent associative-propositional evaluation (APE) model juxtaposes association formation with a propositional evaluative learning process (Gawronski & Bodenhausen, Citation2006). While association formation is based on the joint activation of two stimuli or events, propositional learning is concerned with reasoning about the joint activation. Propositional processes thus differ from associative processes in that they consist in an assessment of the relationship between stimuli and the perceived validity of this stated or observed relation. While the earliest version of the model (e.g. Gawronski & Bodenhausen, Citation2006) states that associative processes operate under conditions of automaticity whereas propositional processes do not, more recent publications emphasise that there is no perfect mapping between acquisition processes and operating conditions (e.g. Gawronski & Bodenhausen, Citation2007, Citation2018; see also Moors, Citation2014). Across all publications, however, the APE model assumes that individuals do not have to be aware of the CS-US pairings to form a mental link between the two and that associative processes are more efficient than propositional ones. Moreover, both associative and propositional processes can influence mental representations unintentionally and uncontrollably (Gawronski & Bodenhausen, Citation2018).
The systems of evaluation model (SEM; McConnell & Rydell, Citation2014; Rydell & McConnell, Citation2006) additionally assumes that associative processes are experiential in nature and lead to evaluations in a slow, cumulative manner. It also assumes that there is a mapping between the way in which a representation is established and the way it is retrieved. While the outcomes of associative learning are retrieved in a spontaneous, unreflective manner, the outcomes of rule-based learning are retrieved via deliberative processes. The APE model does not share this assumption. By contrast, Gawronski and Bodenhausen (Citation2014) assume that propositional processes can access and interpret associations and that representations formed in a propositional manner can be reflected in spontaneous evaluations. Recently, the dual-process perspective has been contrasted with a single-process view that assumes that propositional processes that do not operate in an automatic manner are sufficient for explaining the wealth of findings (De Houwer, Citation2009; Mitchell et al., 2009) and that dissociations between measures or experimental conditions can be explained by means of memory maintenance and retrieval (Stahl & Aust, Citation2018).
As the operating principles of the postulated processes are generally less well defined than their operating conditions, most research into the processes underlying EC effects has focused on whether these effects can occur under conditions of automaticity. A recently published comprehensive review of this literature established that the current evidence does not provide conclusive support for automatic evaluative learning and concluded that more and better research is required to make progress on this front (Corneille & Stahl, Citation2019).
Research into mere exposure effects has seen a similar debate that revolves around the dependence of effects on supraliminal versus subliminal presentation of stimuli and dependence on recognition of the stimuli (see Montoya, Horton, Vevea, Citkowicz, & Lauber, Citation2017, for an overview of the different accounts and a meta-analysis). Finally, the processes by which effects of approach or avoidance behaviours on evaluations are mediated are also subject of an ongoing debate (e.g. Van Dessel, Eder, & Hughes, Citation2018).
Dual-process theories have also been proposed in the persuasion literature, the most prominent of which are the elaboration likelihood model (Petty & Cacioppo, Citation1986) and the heuristic-systematic model (Chaiken, Liberman, & Eagly, Citation1989). Rather than focusing on specific mechanisms (e.g. associative vs. propositional processes) the theories broadly distinguish between peripheral/heuristic and central/systematic routes via which evaluative changes can be achieved. These routes comprise a potentially unlimited set of processes that are characterised by abstract operating principles. Evaluative conditioning, mere exposure, cognitive re-balancing, and application of heuristics are possible examples of the peripheral route, which is characterised by shallow processing and roughly corresponds to the automatic side of the dual-process theories discussed above. These theories’ focus is more on the non-automatic modes of processing (central/systematic route), and they are particularly interested in specifying the conditions that have to be fulfilled in order to activate this pathway. The interest in these processes stems from the assumption that evaluative changes that were accomplished via systematic processing in the central route are more persistent and enduring, and are more predictive for behaviour, whereas attitudes and attitude changes that result from heuristic processes in the peripheral route are more ephemeral in their consequences (Petty & Briñol, Citation2012). Compared to the theories discussed above, these theories are less specific in their assumptions regarding automatic evaluative learning. Evidence regarding the automaticity of processes that contribute to evaluative changes in the persuasion context is much more indirect and less specific.
Adding to the complexities of the existing debates, and cutting across the different paradigms of evaluative learning, we want to highlight that several findings in the literature do not clearly distinguish between the processes that are involved during acquisition and activation/application. In particular, effects of manipulations that are located at the testing stage (e.g. contrasting evaluative bias for indirect versus direct measures of evaluations, or with regard to inclusion vs. exclusion instructions during testing/retrieval, or assessing interactions between manipulations during testing and learning stages) may not unambiguously allow conclusions regarding the operating conditions of the processes during the learning stage (Gawronski & De Houwer, Citation2014). We thus want to sensitise researchers to the distinction between the two stages and to be clear about whether their claims refer to processes involved in acquisition, activation/application, or both.
Another important observation regards the fact that most theories distinguish only between two types of learning processes – evaluations are assumed to result from either reflection and/or association. Such a narrow focus has a strong appeal and has been the underlying inspiration of several highly influential dual-process theories (e.g. Kahneman, Citation2011; Strack & Deutsch, Citation2004; but see Petty & Briñol, Citation2012). In our view, however, focusing on only two allegedly opposing types of processes has not only blurred the distinction between operating principles and operating conditions, it also typically leads to an oversimplified equation of reflective with non-automatic processes, and of associative with automatic processes (see above).
The juxtaposition of reflective and associative processes, treating them as mutually complementary opposites, has also prevented researchers from considering alternative theoretical ideas. For instance, it is no coincidence that evaluative effects of mere exposure or inhibition have typically been ignored by theorists developing theories of EC, because it is unlikely that the evaluative effects of familiarity, fluency, or inhibition can be reframed in terms of associations. They may reflect evaluative side effects of attentional processes (Fenske & Raymond, Citation2006; Raymond et al., Citation2003, Citation2005) or they may involve bindings between stimuli and response tendencies (Chen, Veling, Dijksterhuis, & Holland, Citation2016; see also Gast & Rothermund, Citation2011a, Citation2011b). Fluency effects, or conditional fluency effects that are based on learned familiarity, however, may also be part of what is learned in EC. Learning to expect a certain valence in the context of a CS may alter the processing conditions for valence in the presence of the CS. Rather than associating the CS with the concepts “good” or “bad”, the CS may simply function as a context cue that alters the ease of processing for positive and negative information. Again, we hasten to add that changes in familiarity for positive/negative information in the presence of a CS can occur automatically (e.g. mediated via feelings of fluency) or non-automatically (e.g. mediated by conscious expectations).
Similarly, ongoing discussions in the more general field of automatic action regulation contrasting associative accounts of habitual behaviour with episodic retrieval models of S-R bindings (e.g. Frings et al., Citationin press; Giesen, Schmidt, & Rothermund, Citationin press; Schmidt, Giesen, & Rothermund, Citationin press) have largely been ignored in the literature on evaluative learning. These episodic retrieval models, however, might provide an interesting alternative conception also for evaluative learning (see Gast & Rothermund, Citation2011a, Citation2011b).
Methodological approaches to automaticity in evaluative learning
The prominence and informativeness of experimental approaches to automaticity is apparent across evaluative learning research. Roughly corresponding with the proposed conceptual framework (), two types of methodological approaches to studying automaticity in evaluative learning can be distinguished: A first approach capitalises on manipulations during the learning stage that aim at either eliminating or enhancing the influence of non-automatic processes during learning. A second approach focuses on the activation/application stage, typically by contrasting direct and indirect measurement procedures and/or by employing sophisticated analytic techniques in order to disentangle automatic and non-automatic influences when learned evaluations become activated or applied. In addition, a third approach consists in a combination of the two methodological strategies. Here, researchers focus on interactions between manipulations that are applied during the learning stage (e.g. relational qualifiers of CS-US pairings) with different indicators of evaluative learning (e.g. direct vs. indirect measures) that are applied during the testing stage. We will discuss each of these strategies in turn.
Experimental approaches targeting the acquisition stage
A straightforward way of testing automaticity in evaluative learning is to manipulate the conditions under which evaluative experiences are presented during the learning stage. Presenting the to-be-learned information under processing conditions that prevent conscious identification and/or intentional processing (e.g. by presenting the stimuli or pairings subliminally, under distracting conditions, with time pressure, or under strong capacity limitations) ensures that any resulting effects of evaluative learning reflect the result of unconscious and/or unintentional processes (cf. Stahl, Haaf, & Corneille, Citation2016). Similarly, evaluative information can be presented either incidentally, that is, without being directly referred to in the instructions and focusing on the mere experience of contingencies, or explicitly, that is, in the form of direct statements and descriptions, or as part of the task instructions (Corneille, Mierop, Stahl, & Hütter, Citationin press; Hütter & De Houwer, Citation2017; Van Dessel, De Houwer, Gast, & Tucker Smith, Citation2015).
All measures can reflect automatic and non-automatic processes to varying degrees (Jacoby, Citation1991). Process dissociation approaches are a combination of procedural manipulations (e.g. inclusion and exclusion instructions) with statistical techniques aimed at separating the processes that contribute to the outcome of a given task (e.g. an evaluative response). Through the combination of task versions in which processes lead to converging versus opposing responses, process dissociation approaches have a higher potential of estimating the contribution of automatic and non-automatic processes to task performance. The strongest evidence can be gained from combining processing tree models that separate different classes of processes within a single task with an experimental approach (Hütter & Klauer, Citation2016; Sweldens, Corneille, & Yzerbyt, Citation2014). The number of such applications to evaluative learning is increasing (e.g. Hütter & De Houwer, Citation2017; Hütter & Sweldens, Citation2013; Hütter, Sweldens, & Andrade, Citation2018; Hütter, Sweldens, Stahl, Unkelbach, & Klauer, Citation2012; Mierop, Hütter, Stahl, & Corneille, Citation2019) and they indeed suggest important constraints to the operation of automatic processes during acquisition.
Although straightforward, strategies to prevent conscious and controlled processing often come with the cost that these manipulations influence not only the processes that are involved in the acquisition of evaluative representations (i.e. the processes that translate evaluative experiences into mental representations of evaluations), but also might interfere with the mere registration of the information that is presented during learning. Employing very strict manipulations regarding unawareness thus typically requires additional measures in order to show that evaluative experiences were in fact registered by the cognitive system. Otherwise, null effects cannot be interpreted as counterevidence against the possibility of automatic evaluative learning.
Measurement strategies targeting the activation/application stage: indirect vs. direct measures of evaluation
Secondly, the study of automaticity is strongly tied to the development of measurement procedures. The goal to conceal the measurement purpose to participants led to the creation of measures that infer participants’ evaluations of an attitude object or category from patterns of responses. The evaluative priming task (EPT), for instance, requires participants to categorise valent targets as positive or negative that are preceded by positive or negative primes (Fazio, Sanbonmatsu, Powell, & Kardes, Citation1986; Fazio, Jackson, Dunton, & Williams, Citation1995). In the EPT, primes are strictly task irrelevant, which renders any influence of the primes on the resulting target evaluations unintentional. Furthermore, the EPT puts participants under severe time pressure and thus limits their potential to strategically influence the outcomes of the task (but see Teige-Mocigemba & Klauer, Citation2013). To further reduce the influence of strategic processes, primes can be presented subliminally (e.g. by masking), which eliminates the possibility to control the outcome of the task.
The Implicit Association Test (IAT, Greenwald, McGhee, & Schwartz, Citation1998) has participants categorise stimuli that vary along a target dimension (e.g. “White” vs. “Black”). In the relevant blocks of this task, these trials are combined with the categorisation of positive and negative stimuli. Both types of categorisation make use of the same pair of response keys so that one target group is categorised by using the “positive” key, while the other one is categorised by using the “negative” key. Both the EPT and the IAT compare response times and accuracies between the congruent and incongruent versions of the task. A positive evaluation is inferred to the degree that responses on congruent trials are faster (or more accurate) than responses on incongruent trials. The Affect Misattribution Procedure (AMP; Payne, Cheng, Govorun, & Stewart, Citation2005) relies on a different procedure. Participants evaluate target stimuli such as Chinese ideographs that are preceded by primes that participants are asked to ignore (structurally corresponding to an EPT with neutral targets). The evaluation of the primes is inferred from the bias they introduce to the rating of the targets.
Initially, researchers turned to indirect measures to safeguard against demand artefacts as explanations for evaluative learning effects (e.g. Hermans, Vansteenwegen, Crombez, Baeyens, & Eelen, Citation2002). With the rise of dual-process theories of evaluative learning and judgment (e.g. Rydell & McConnell, Citation2006; Strack & Deutsch, Citation2004) researchers started to embrace the idea that direct and indirect measures could be differentially reflective of implicit learning and retrieval. Hence, researchers started looking for dissociations between measures as evidence for dual-process and dual-systems theories (e.g. Rydell & McConnell, Citation2006; but see Heycke, Gehrmann, Haaf, & Stahl, Citation2018). Importantly, such dissociations can also be a result of measures instigating different retrieval processes. Hence, such dissociations are informative regarding evaluative learning (Stage 1) only to the degree that retrieval processes (Stage 2) are strongly related to the learning processes or the representations these generate (e.g. Rydell & McConnell, Citation2006). If one assumes that learning and retrieval are independent, such as the APE model (Gawronski & Bodenhausen, Citation2014), these dissociations are inconclusive regarding the automaticity of evaluative learning. To illustrate this point with an empirical study, De Houwer (Citation2006) showed that a learning procedure that consisted merely of instructions can induce IAT effects which are mediated by propositions, which were previously assumed to be reflective of associative learning effects. Moreover, factors that have been shown to influence direct measures also seem to influence indirect measures (Cone & Ferguson, Citation2015).
If the choice of measure cannot unambiguously inform us about the nature of the learning process (Stage 1) underlying the targeted evaluations, is the use of indirect measures at least informative on the automaticity criteria that characterise evaluative judgment (Stage 2)? Evidence is accumulating that the choice of measure is not always informative regarding the operating conditions of the cognitive processes (i.e. automatic vs. non-automatic) that are producing the effects. Both direct and indirect measures have been shown to reflect both automatic and non-automatic processes. For instance, seminal work by Schwarz and Clore (Citation1983) shows that under certain conditions momentary feelings influence direct judgments such as judgments of life satisfaction. Conversely, indirect measures have been shown to be influenced by controlled and strategic responding (Fiedler & Bluemke, Citation2005; Steffens, Citation2004).
Of course, these critical findings should not be taken as evidence that it is impossible to construct indirect measures that are fairly process pure (for recent reviews, see Meissner, Grigutsch, Koranyi, Müller, & Rothermund, Citation2019; Meissner & Rothermund, Citationin press; Wentura & Rothermund, Citation2014). Both procedural variations and statistical analysis techniques may help to develop indirect measures that allow for an unbiased estimation of automatic evaluative processes. For instance, automaticity of the underlying processes producing effects in a measure may be fostered by time pressure (e.g. via response deadlines), or by using variants of the IAT that eliminate strategic recoding (e.g. single block variants of the task like the IAT-RF, Rothermund, Teige-Mocigemba, Gast, & Wentura, Citation2009, or the SB-IAT, Teige-Mocigemba, Klauer, & Rothermund, Citation2008). On the analytical side, multinomial processing tree models have been proposed for the IAT and for the AMP that offer options to disentangle automatic from non-automatic processes (e.g. the ReAL model, Meissner & Rothermund, Citation2013, or the Quad model, Conrey, Sherman, Gawronski, Hugenberg, & Groom, Citation2005, for the IAT; for the AMP, see Payne, Hall, Cameron, & Bishara, Citation2010). Other analytical tools like diffusion modelling have also been used to distinguish between different process components that influence responding in the IAT (Klauer, Voss, Schmitz, & Teige-Mocigemba, Citation2007), although it is not clear whether and how these components can be mapped onto the automatic/non-automatic distinction. Independent of the modelling technique being used, we want to emphasise that also in the domain of activation and application an experimental approach is required for validating the parameters and for testing hypotheses derived from pertinent theories (see e.g. Hütter & Klauer, Citation2016; Meissner & Rothermund, Citation2013).
Whereas previous research indicates that scores on direct and indirect measures can reflect both automatic and non-automatic responses to varying degrees, direct and indirect measures conventionally differ in a number of respects that can influence the relative proportion of both types of processes. For instance, indirect measures typically present the attitudinal stimuli only briefly (for instance, as primes) and participants are requested to respond within a short time frame. Direct, self-report measures can typically be completed at the participants’ own pace so that participants have the time to reflect on their answers.
This analysis invites a research approach that equates direct and indirect measures on the dimensions they typically differ in, such as the emphasis on speed. For instance, Ranganath, Smith, and Nosek (Citation2008) had participants complete IATs as well as speeded and unspeeded self-report attitude measures. They demonstrate that IAT scores corresponded more strongly with speeded self-report measures than unspeeded ones. The finding that operating conditions present at retrieval influence the measurement outcome in the predicted direction, supports the notion that measures differ in the proportions of automatic and non-automatic responses contributing to the outcome. These results also concur with dual-process theories that specify the conditions under which judgments can be described as intuitive versus reflective (e.g. Fazio, Citation1990; Strack & Deutsch, Citation2004).
Given that direct and indirect measures differ on several dimensions and given that these differences potentially influence the measurement outcomes, dissociations between measures can always be due to several factors. Recent research into the role of the operating conditions present during retrieval thus suggested to use parallel versions of the same task that differ only in the automaticity criterion of interest (Payne, Burkley, & Stokes, Citation2008). Payne et al. (Citation2008) used two versions of the AMP that were structurally equivalent and differed only in whether the attitude object of interest was rated intentionally or unintentionally. Specifically, participants were either told to ignore the prime and rate the target as in the standard version, or they were told to rate the prime instead of the target. Despite the increase in structural fit, the measures reacted differently to a manipulation of the motivation to hide prejudiced reactions, attesting to the validity of the distinction (Payne et al., Citation2008, Study 4). While the indirect AMP (i.e. the original version of the measure) seems to consist of a relatively larger proportion of a misattribution process, in the direct AMP the proportion of deliberate processes is relatively larger.
Combining the two stages: Investigating interactions between manipulations during acquisition and activation/application
Perhaps the most promising methodological approach consists in a combination of manipulations regarding the learning and measurement stages. Specifically, researchers are investigating the operating conditions of learning and retrieval processes simultaneously (e.g. Stahl et al., Citation2016). A prominent study by Moran and Bar-Anan (Citation2013) revealed that relational qualifiers that were introduced in combination with CS-US pairings during the learning stage could reverse the meaning of CS-US pairings for direct evaluations, but had no influence on an indirect assessment of valence (but see Bading, Stahl, & Rothermund, Citation2020 this issue). In principle, studies combining manipulations during acquisition and measurement allow researchers to investigate how the two stages are related. Dissociations of effects as a function of the learning procedure would potentially support the claim that automatic and non-automatic processes contribute to the acquisition of evaluative representations under clearly specified conditions. However, any such dissociation must be critically examined as it could be driven by processes related to acquisition and/or processes related to retrieval. For instance, in the study by Moran and Bar-Anan (Citation2013), the dissociation between the direct and the indirect measure could be driven by differential effects of automatic versus non-automatic processes on evaluative representations or differential retrieval effects instigated by the two types of measures (e.g. incomplete retrieval of stored information on indirect measure vs. complete retrieval of stored information on direct measure [see also Kukken, Hütter, & Holland, Citation2020 this issue]).
To summarise, all three methods of testing automaticity in evaluative learning have pervasively been used in previous research and have garnered a host of interesting findings. Still, there is at best no consensus regarding the operating conditions of evaluative learning, because most research suffers from methodological problems and complexities that prevent unambiguous conclusions. Much research arguably demonstrating automatic processes is vulnerable to alternative explanations (Corneille & Stahl, Citation2019). Experimental manipulations that aim at guaranteeing automaticity during acquisition might prevent evaluative experiences from being registered (Stahl et al., Citation2016), direct and indirect measures are often not process pure, and conclusions regarding the automaticity of acquisition processes may be hard to justify on the basis of distinctions regarding the retrieval and activation stage. Finally, previous research frequently did not clearly distinguish between operating principles and operating conditions, inferring operating principles (e.g. associative vs. propositional) from automaticity or the lack thereof.
Contents of the special issue
Overview
The contributions to this special issue address core issues relating to the influence of automatic processes in acquiring or changing evaluations and have direct implications for theoretical accounts of evaluative learning. With regard to paradigms, most of the authors employed EC and related paradigms such as contingency learning (Gast et al., Citation2020 this issue) and category learning (Högden, Stahl, & Unkelbach, Citation2020 this issue) that also involve the pairing of neutral with valent stimuli. Next to the EC paradigm, Smith, Calanchini, Hughes, Van Dessel, and De Houwer (Citation2020 this issue) cover the mere exposure and approach-avoidance paradigms in their comparison of experienced and described evaluative learning procedures.
A core feature characterising nearly all articles in this special issue is that they combine manipulations targeting the quality of evaluative learning during the acquisition stage with other manipulations regarding the activation, retrieval, or expression of evaluative mental representations during the assessment stage within the same study. As we argued above, such a design combining manipulations during the acquisition and assessment stages may be best suited to investigate the complex interplay of automatic and non-automatic processes during learning and application of evaluative mental representations, and may allow the deepest insight into questions regarding automaticity in evaluative learning.
Manipulations that were used during acquisition regard the awareness of evaluative influences (Sava, Payne, Măgurean, Iancu, & Rusu, Citation2020 this issue), the aversive quality of the US (Benedict & Gast, Citation2020 this issue), the diagnosticity, primacy and sequential position of evaluative information (Van Dessel, Cone, Gast, & De Houwer, Citation2020 this issue; Hutchings et al., Citation2020 this issue), the experiential versus instructed character of evaluative information (Smith et al., Citation2020 this issue), the meaning of CS-US pairs based on relational qualifiers (Bading et al., Citation2020 this issue; Moran & Bar-Anan, Citation2020 this issue; Kukken et al., Citation2020 this issue), and rule-based versus similarity-based learning (Högden et al., Citation2020 this issue).
On the assessment side, many studies used multinomial processing tree modelling to disentangle different components underlying the expression of evaluations (Kukken et al., Citation2020 this issue; Smith et al., Citation2020 this issue), and/or compared evaluative learning effects that were assessed with direct versus indirect measures (Bading et al., Citation2020 this issue; Gast et al., Citation2020 this issue; Van Dessel et al., Citation2020 this issue). Other papers compared evaluations with and without control options (Sava et al., Citation2020 this issue), speeded and unspeeded evaluations (Hutchings et al., Citation2020 this issue; Moran & Bar-Anan, Citation2020, this issue; Smith et al., Citation2020 this issue), or investigated cued versus non-cued retrieval during assessment (Hutchings et al., Citation2020 this issue; Van Dessel et al., Citation2020 this issue).
Summaries of the individual contributions
Smith et al. (Citation2020 this issue) compared the parameters of the Quad model (Conrey et al., Citation2005) between experience-based and description-based learning procedures. The Activation parameter was of particular interest. The Activation parameter is typically assumed to constitute a measure of automatically activated associations. The SEM (Rydell & McConnell, Citation2006) assumes that association formation is a slow process that requires repeated learning opportunities. Smith et al. (Citation2020 this issue) document very small effects on this parameter that are independent of learning mode. These results can be interpreted in (at least) two ways. First, they can be interpreted as questioning the validity of dual-system theories like the SEM. Second, they can be interpreted as questioning the Quad model's Activation parameter's ability to capture associations. Interestingly, there were no differences between EC, mere exposure, and approach-avoidance trainings, suggesting that these three learning paradigms do not differ in their potency of altering the Quad model parameters.
Benedict and Gast (Citation2020 this issue) tested whether stimulus characteristics influence whether EC effects could be found in the absence of explicit memory. They compared fear- and disgust-evoking stimuli to negative stimuli that do not evoke fear or disgust. Benedict and Gast found only tentative evidence for EC effects in the absence of explicit memory and no differences between fearful or disgusting stimuli and other negative stimuli, indicating that EC is mostly driven by cognitive rather than affective factors.
Gast et al. (Citation2020 this issue) employed a valence contingency learning task (Schmidt & De Houwer, Citation2012) that requires participants to classify target stimuli as positive or negative. The targets are preceded by CSs. While some CSs mostly precede positive targets, others mostly precede negative targets. In this task, participants learn to respond faster on trials that conform to the contingency than on trials that do not conform, even when they cannot report the contingency. However, the evaluative ratings showed EC effects only in the presence of explicit memory (contrary to Schmidt & De Houwer, Citation2012). In their integrative analysis of the existing evidence, Gast et al. (Citation2020 this issue) further question the notion of EC effects in the absence of explicit memory.
Van Dessel et al. (Citation2020 this issue) investigated the effects of diagnosticity, primacy, and retrieval cues on two parallel versions of the AMP that differed only in whether participants were asked to evaluate the targets or the primes. Both variants demonstrated an effect of diagnosticity, whereas none of these measures demonstrated consistent effects of the other factors. Thus, Van Dessel et al. replicated previous findings on the role of diagnosticity in impression formation (Cone & Ferguson, Citation2015) and extended these findings to a new set of evaluative measures. In essence, showing effects of diagnosticity on both direct and indirect measures of evaluation is in line with the view that both automatic and non-automatic processes of evaluative learning follow the same operating principles, that is, both reflect the logic of propositional reasoning. Alternatively, the study might also be taken as an indication that even “implicit” versions of the AMP in which participants are asked to ignore the primes might not be truly automatic and reflect also strategic and intentional application of prime valence.
Hutchings et al. (Citation2020 this issue) investigated the effect of a counter-attitudinal retrieval cue on evaluations in a generalisation paradigm. These authors first presented negative information about a target person against a coloured background. In the second phase of the experiment, negative information was presented about the target against another background colour. When presented with the counter-attitudinal information (i.e. during the second phase), participants additionally wore a wristband, whose effects on renewal (i.e. evaluations given in the same context as in the first phase) and generalisation (i.e. evaluations given in a novel context) were assessed on a speeded evaluative measure and a feeling thermometer. They showed that counter-attitudinal retrieval cues did not influence any of these measures. The context cues, however, did demonstrate a consistent direct influence on the ratings of the target person and novel persons. This effect was also independent of the type of evaluative measure (speeded vs. unspeeded) used, suggesting that context cues exert their influence efficiently. The absence of reliable effects of the retrieval cues speaks against theories that posit context-dependency of evaluative changes that are acquired for stimuli with an already established valence. By comparing context and retrieval effects for CSs and against novel stimuli that are used as a baseline, the study makes an important methodological contribution to the study of generalisation and renewal effects.
Högden et al. (Citation2020 this issue) used a category learning task in which individual stimuli belong to a different category than compound stimuli comprised of the individual stimuli. In Högden et al.'s variant, the categories carry positive or negative valence. This set-up allows for the distinction of similarity-based from rule-based generalisation. Participants who verbalised the rule used it for category judgments of novel stimuli. However, evaluative ratings of the same stimuli showed similarity-based generalisation. Högden et al. (Citation2020 this issue) investigated this discrepancy by manipulating the scale format of the dependent measures. Their findings suggest that participants who verbalised the rule are more likely to apply the rule to the dependent measure, the more similar it is to the category learning task. Thus, rather than establishing a special role for similarity-based generalisation processes in evaluative learning, the findings of Högden et al. seem to suggest that similarity-based generalisation generally depends on features of task correspondence.
Bading et al. (Citation2020 this issue) scrutinised the wide-spread assumption that the IAT assesses associative learning effects that are insensitive to propositional information. Specifically, Moran and Bar-Anan (Citation2013) relied on the comparison of an IAT with a self-report measure to conclude that individuals show both an associatively and a propositionally mediated EC effect in a relational EC paradigm. Thus, they claimed that the information that the CS “ends” a positive US, concurrently leads to a positive and a negative EC effect. Based on the notion of flexible similarity construction, Bading et al. (Citation2020 this issue) reasoned that the structure of the IAT is responsible for this dissociation. Consistent with expectations, the impact of relational information on IAT performance depended on the type of similarity that was emphasised by the IAT. If the IAT contrasted positively with negatively paired CSs, the influence of the relations was not apparent. If, however, the IAT contrasted “starting positive” and “ending negative” with “ending positive” and “starting negative” CSs, the IAT reflected the influence of the relation on evaluations. Thus, both information on US valence as well as on CS-US relations influenced IAT effects. Consequently, the IAT does not provide access to qualitatively different representations. Moreover, the IAT measures evaluations that are flexibly construed.
Moran and Bar-Anan (Citation2020 this issue) provided an experimental approach to disentangle two possible explanations for their earlier finding that relational paradigms produce a reversed, but smaller EC effect in the condition in which the relational qualifier indicates that US valence has to be reversed (e.g. in the “ending” condition). This effect may be explained by a complete, but weaker reversal of the evaluations or by two concurrent, antagonistic processes that lead to opposite evaluations. Moran and Bar-Anan chose to apply time pressure at judgment to suppress the deliberate process assumed to lead to reversed evaluations. Only one out of three experiments provided evidence in favour of this hypothesis.
Sava et al. (Citation2020 this issue) present a measurement approach that allows investigating whether participants are aware of the influence that a learning procedure has on their evaluations. Half of their participants got an option to “pass” whenever they felt that their evaluation of the CS was influenced by the pairing with the US. Their results indicate that participants are often unaware of the influence that the US has on the CS. Their data also indicate that memory for the CS-US pairing increases influence awareness.
Kukken et al. (Citation2020 this issue) addressed the same question using a different approach. These authors employed a multinomial processing tree model to separate assimilative learning processes that link the CS to the US from processes that regard the relational qualifier in a direct evaluative measure (while also controlling for guessing). Their findings suggest that two independent EC effects contribute to overall evaluative change in a relational EC paradigm. This interpretation was supported by indicators of model fit as well as correlational analyses that related the parameter estimates to conventional evaluative ratings. Moreover, the parameters were validated experimentally via a manipulation of the instructions that participants received prior to learning. Some participants were asked to focus on the role of the CS as in the original work (i.e. participants were asked to encode US valence and the relational qualifier; e.g. “end positive”). Other participants were asked to encode the meaning (i.e. the meaning of the action; e.g. “negative”). Whereas this manipulation had a strong effect on the parameter representing learning processes that regard the relational qualifier, the assimilative parameter was not affected attesting to the independence of these parameters.
Theoretical and methodological implications
The articles assembled in this special issue have implications for broader issues of cognition, learning, and evaluative responses.
Is there evidence for automaticity in evaluative learning?
Of the 10 articles in this special issue only two provide support for automatic evaluative learning. Sava et al. (Citation2020 this issue) demonstrated that individuals are often not aware of the impact that CS-US pairings have on their evaluation of the CS. Thus, they addressed awareness at a different processing stage than most other EC studies that investigated this automaticity feature (cf. Sweldens et al., Citation2017). That is, the awareness debate has primarily focused on whether participants must be aware of the joint presentation of CS and US in order to produce an EC effect. As discussed above, this research generally documents the necessity of contingency awareness for EC effects to occur. Indeed, it may be necessary for participants to pay attention and to encode the pairings (Benedict & Gast, Citation2020 this issue; Gast et al., Citation2020 this issue), but Sava et al. suggest that participants might still not be aware of the influence of the US on their evaluation of the CS. The conclusions that can be drawn from this research are two-fold. First, participants might not have to be aware of potential effects of US valence on the CS in order to show EC effects. Second, it might not be necessary for participants to make inferences about the valence of the CS. This finding thus suggests that while propositional processes (e.g. De Houwer, Citation2009; Van Dessel, Hughes, & De Houwer, Citation2018) underlie some or even many EC effects, they might not be a causal factor for all EC effects.
Second, the processing tree approach to evaluative learning introduced by Kukken et al. (Citation2020 this issue; see also Heycke & Gawronski, Citationin press) shows that the parameter reflecting assimilative processes (i.e. reflecting an influence of the US on the CS) is independent of the relational qualifier and instructions to process the pairings in a certain way. Thus, the assimilative learning parameter is a candidate for automatic evaluative learning. However, it remains to be shown whether the model parameters differ in their sensitivity to operating conditions generally assumed to reflect automaticity features. Moreover, as relational paradigms that focus on operating principles are structurally similar to paradigms investigating the controllability of evaluative learning as an operating condition, the question regarding the overlap between relational and controllable learning as well as assimilative and uncontrollable learning seems particularly relevant (Hütter et al., Citation2018).
Nevertheless, purely associative theories of evaluative learning are clearly not supported. The articles in this special issue demonstrate novel evidence that speaks to a role of propositional processes. For instance, Van Dessel et al.’s (Citation2020 this issue) results lend credence to an inferential account of evaluative learning (Van Dessel et al., Citation2018) in that they demonstrate an effect of diagnosticity on evaluations. Moreover, strict dual-system theories (Rydell & McConnell, Citation2006) do not receive support from the articles in this special issue. That is, evidence does not speak to slowly accumulating associative processes (Smith et al., Citation2020 this issue) that affect indirect but not direct evaluative measures (Bading et al., Citation2020 this issue; Van Dessel et al., Citation2020 this issue).
Gast et al.’s (Citation2020 this issue) findings could be interpreted as evidence speaking to qualitative differences between evaluative learning and learning of motor responses or perceptual categories which have been demonstrated in the absence of conscious knowledge (e.g. Eberhardt, Esser, & Haider, Citation2017; see also Greenwald & De Houwer, Citation2017). Illuminating the commonalities and differences between various learning effects and learning paradigms could help outline the boundary conditions of (automatic) evaluative learning (see also Walther, Halbeisen, & Blask, Citation2018).
Is evidence for evaluative learning dependent on the measurement task?
Based on the results reported in this special issue and previous results, the choice of task is not informative on automaticity characteristics. Importantly, previous research relied on the IAT to assess so-called “implicit attitudes” that are assumed to be unconscious or acquired in an associative manner (e.g. Moran & Bar-Anan, Citation2013). However, as demonstrated by Bading et al. (Citation2020 this issue) the IAT does not provide access to implicit attitudes. Instead, dissociations of evaluative effects between different measures are directly related to the structure of the measure and might reflect processes of similarity construction rather than evaluative associations. Moreover, Hutchings et al.’s (Citation2020 this issue) work speaks to the equivalence of speeded and unspeeded measures (although their measures differed in more than just speed instructions). Finally, Van Dessel et al. (Citation2020 this issue) demonstrate that diagnosticity affects both a direct and an indirect version of the AMP. These findings suggest that either there are no “implicit attitudes” in terms of separate representations in line with the prominent APE model (Gawronski & Bodenhausen, Citation2006) or that “implicit measures” or measurements under “implicit conditions” do not guarantee access to such implicit representations. In line with this analysis, previous research suggests that equating the retrieval conditions between direct and indirect tasks increases the correspondence between those attitude measures (Payne et al., Citation2008; Ranganath et al., Citation2008).
It may thus not be surprising that many authors of this special issue relied on direct evaluative measures to assess the automaticity of evaluative learning. Authors either assessed the effectiveness of EC under various conditions or separated processes underlying EC effects on direct measures using multinomial processing tree modelling. A particularly interesting approach was presented by Högden et al. (Citation2020 this issue) who assessed generalisation as an indicator of (automatic) evaluative learning. While their main analyses support the notion of automaticity in evaluative learning, Högden et al.'s experimental investigation of the structural properties of evaluative and semantic tasks suggests the discrepancies between evaluative and semantic measures in their paradigm may be explained by means of their structural similarity to the learning task. This is in line with previous work on expectancy-liking dissociations that shows that dissociations between expectancy and evaluative measures disappear when evaluative and expectancy measures are equated structurally. Specifically, Aust, Haaf, and Stahl (Citation2019) demonstrated that evaluative measures are by default more integrative than expectancy measures, which are sensitive to trial-to-trial changes. Increasing the temporal density of repeated liking measures increases the similarity of evaluative measures and expectancy measures. Thus, the two research lines have in common that some measures require participants to abstract from a certain learning episode, thereby inducing a divergence from measures that do not require abstraction.
Is evidence for evaluative learning dependent on the specific learning paradigm?
As the contributions to the special issue illustrate, there is a large variety of EC paradigms. In fact, there is little standardisation in learning procedures apart from the fact that a pairing takes places (Walther et al., Citation2018). The present articles comprise picture-picture, nonword-word, picture-sound, and picture-trait pairings. The CSs reach from simple brand logos to comic creatures to realistic faces. Number of CSs differs tremendously between paradigms, reaching from one (Hutchings et al., Citation2020 this issue) or two (Smith et al., Citation2020 this issue) up to 80 CSs per participant (Kukken et al., Citation2020 this issue). While EC effects are still significant at large numbers of pairings, large stimulus numbers should increase the likelihood that participants cannot reliably encode all the pairings in explicit memory (e.g. Craik & Tulving, Citation1975; Lustig, May, & Hasher, Citation2001).
Automaticity and implicit processes
In the present editorial, we resorted to the term “automatic” rather than “implicit” to refer to the phenomena under scrutiny for several reasons. Implicitness is often used as an umbrella term for processes that operate (even) under conditions of automaticity. However, researchers use the implicitness terminology in research into attitudes not only to refer to automaticity features, but to a host of other definitions (e.g. indirectly assessed, acquired in an associative manner, driven by gut reactions). Moreover, “implicitness” is strongly semantically related to dual-process theories on the one hand (e.g. Gawronski & Bodenhausen, Citation2006; Rydell & McConnell, Citation2006) and to indirect measurement procedures on the other hand (e.g. Greenwald & Banaji, Citation2017; Greenwald et al., Citation1998). As is demonstrated in the present special issue, recent research contradicts the notion that measures such as the IAT have preferential access to implicit attitudes (Bading et al., Citation2020 this issue).
Despite this somewhat sceptical conclusion, it should be noted that new developments with regard to procedural features or analytical techniques might allow for more sophisticated assessments of spontaneous evaluations with indirect measures (e.g. Meissner et al., Citation2019; Meissner & Rothermund, Citationin press). Making use of these refinements in indirect measures – in combination with manipulations targeting the acquisition stage – is a promising avenue for further research on automatic evaluative learning.
As we have shown in this article, automaticity too should not be treated as a unitary construct (Bargh, Citation1994; Moors & De Houwer, Citation2006). Researchers should always specify which automaticity feature is of interest in their research programme. Moreover, neither should other automaticity features be inferred from a demonstrated automaticity feature, nor should automaticity be treated as an indicator of a particular operating principle. Instead, these relationships should be investigated via empirical investigations.
Relations to our understanding of the cognition–emotion interface
The role of automatic processes in evaluative learning has been a topic of discussion ever since Zajonc's (Citation1968, Citation1980, Citation1984) seminal demonstration of mere exposure effects despite a lack of conscious recognition, resulting in the claim that “preferences need no inferences”. Interestingly, even at that time – more than 30 years ago – the appraisal theorists’ reply already emphasised the distinction between operating principles and operating conditions. Despite being inaccessible to consciousness, the processes involved in mediating these effects could still reflect (automatic) cognition or might not reflect emotion proper (Lazarus, Citation1984; Leventhal & Scherer, Citation1987).
A similar position can be sketched with regard to automatic evaluative learning. Demonstrating the automatic nature of evaluative learning does not necessarily tell us much about the quality (i.e. the operating principles) of the processes that translate evaluative experiences into mental representations. In principle, these processes might still be propositional in nature (as suggested by propositional single-process theories of learning, e.g. De Houwer, Citation2018). Thus, rather than focusing on the automatic versus non-automatic quality of evaluative learning, our understanding of how our cognitive system processes evaluative experiences might profit more from the theoretical and empirical analysis of the operating principles of these processes.
Of course, some variants of dual-process theories contain claims that equate specific processes with specific operating conditions (associative = automatic, inferential = non-automatic). Although these claims regarding a relation between operating principles and operating conditions are intuitively plausible (e.g. the more complex reflective processes become, the more cognitive resources may be needed for their successful execution), the evidence that speaks against a strict equivalence is overwhelming, in both evaluative and non-evaluative learning. Demonstrations of non-automatic as well as automatic influences on evaluative learning thus cannot be taken as unambiguous evidence for the associative or reflective nature of evaluative learning, respectively. Additional evidence (e.g. regarding the influence of relational qualifiers during acquisition, effects of retrieval instructions, context dependency or patterns of generalisation) is needed to understand the nature of evaluative learning, and also may help to clarify the role of cognitive processes in shaping evaluative responses.
Future directions in the study of automaticity in evaluative learning
Whereas our list of research avenues for future work on automaticity of evaluative learning cannot be exhaustive, we assemble critical questions that relate to current debates in the evaluative learning literature.
Specification of the operating principles, operating conditions, and their relation
The investigation of automaticity in evaluative learning can profit from further specification of the operating principles that can lead to evaluative learning under conditions of automaticity. Theorising on the processes underlying automatic learning effects would help researchers design experiments that are better suited for the study of automaticity. One positive example is the misattribution principle proposed by Jones et al. (Citation2009) that allows clear predictions regarding the boundary conditions of automatic EC effects. Whereas such an endeavour rests on the assumption that automatic processes exist, recent research has often led to negative views on the existence of automaticity. However, some of the failures to document automatic evaluative learning effects may result from the particular procedures that were implemented. As there is currently little agreement on what an ideal evaluative learning procedure looks like, research is fairly unsystematic in this regard.
Another question regards whether operating conditions are related to specific operating principles (see above). A systematic investigation of this issue requires an orthogonal manipulation of operating conditions (e.g. by manipulating awareness, availability of processing resources, or task relevance of the presented evaluative materials) and an assessment of the quality of the resulting evaluative learning process (e.g. by comparing effects between different CS-US relations, or between retrieval instructions, different measures tapping into mental representations of evaluations or generalisation patterns). As we already stated in the previous section, such a systematic study is crucial for an understanding of the role of cognition in evaluative learning.
Our review also showed that the current evaluative learning literature is characterised by a rather narrow focus on dual (or single) process theories that restrict the range of mediating processes to associations and propositions. In our view, broadening the scope of theoretical constructs and processes (e.g. episodic retrieval of S-R episodes, evaluative action effects, attentional capture, familiarity, processing fluency), and investigating their interrelation and relative contribution to resulting evaluative responding will help researchers to integrate a wide range of empirical phenomena (EC, approach-avoidance tasks, mere exposure, persuasion), and to identify the most relevant mediators for various evaluative learning paradigms (see also Walther et al., Citation2018).
Generalisation
Another important goal is to better understand context-dependency and generalisation in evaluation and evaluative learning. These phenomena are at the very heart of evaluative learning, both theoretically (e.g. regarding the mental representation of evaluations and conditions under which these representations become activated) and also with regard to practical implications (e.g. regarding interventions aiming at changing maladaptive evaluations in addiction or social discrimination). Current research captures only the peak of the iceberg in this regard (see Högden et al., Citation2020 this issue; Hutchings et al., Citation2020 this issue). In order to address issues of generalisation and context-dependency in evaluative learning more systematically, experimental manipulations and measurement procedures should be developed that allow for an identification of modulating influences of situational contexts on the acquisition and retrieval of evaluations.
Developing improved versions of indirect measures
Another important avenue for further research consists in optimising indirect measures of evaluation (Meissner et al., Citation2019; Meissner & Rothermund, Citationin press). Priming measures have been proposed that tap into an (automatic) activation of evaluations by single stimuli. These measures typically suffer from low reliability, which may be due to the fact that they do not take into account the context dependency of evaluations (Blair, Citation2002; Wittenbrink, Judd, & Park, Citation2001). Incorporating contexts and specific situations into the priming procedure might help to overcome this shortcoming, and has already successfully been implemented in dual-prime measures to assess the automatic activation of social stereotypes in context (Casper, Rothermund, & Wentura, Citation2010, Citation2011; Wigboldus, Dijksterhuis, & van Knippenberg, Citation2003).
In comparison to priming measures, the IAT suffers from the opposite type of problems. Despite high reliability, the IAT is prone to the influence of processes that are unrelated to an evaluation of the target categories of interest. These processes can be subsumed under the label of task recoding (Gast & Rothermund, Citation2010; Rothermund et al., Citation2009; Teige-Mocigemba et al., Citation2008) or strategic control/suppression of evaluative responding (Conrey et al., Citation2005). Furthermore, IAT effects might reflect knowledge about evaluations rather than personal endorsement of these evaluations (Karpinski & Hilton, Citation2001). Procedural and analytical tools have been developed to overcome these problems (e.g. single-block variants of the IAT have been proposed that eliminate recoding, Rothermund et al., Citation2009; Teige-Mocigemba et al., Citation2008, multinomial processing tree models allow for a separate identification of evaluative associations, recoding, or controlled responding, Conrey et al., Citation2005; Meissner & Rothermund, Citation2013, and personalised task instructions can be used to assess personally endorsed evaluations, Olson & Fazio, Citation2004).
As with the standard priming measures, IATs typically assess evaluations of target categories in a contextual vacuum, which limits their use as a measure of context-dependent evaluations, which is of crucial relevance also for many studies on evaluative learning (e.g. Hutchings et al., Citation2020 this issue). To capture these more specific context-dependent evaluations, researchers might consider using recently developed paradigms that allow for an indirect assessment of personal beliefs (e.g. the Propositional Evaluation Paradigm, PEP, Müller & Rothermund, Citation2019; see also Wiswede, Koranyi, Müller, Langner, & Rothermund, Citation2013). This paradigm assesses the personal endorsement of (evaluative) propositions (sentences) rather than assessing evaluations of objects or categories, and thus can easily be adapted to capture personal endorsement of statements that express context-specific evaluations (e.g. via assessing automatic truth inferences for sentences like “women are bad at driving cars” or “old people are incompetent in operating electronic devices”).
Developing indirect measures that allow for an unambiguous separation of automatic and non-automatic, associative and propositional processes in retrieving and applying evaluative knowledge will help to improve our understanding of how evaluations are mentally represented and activated.
Processing tree modelling
An alternative pathway to disentangling different sources of influence on evaluative responses is provided by process dissociation procedures (Jacoby, Citation1991). The core element of these procedures consists in a comparison of different task instructions (inclusion vs. exclusion) during retrieval in order to estimate the influence of automatic and non-automatic processes. Originally developed to distinguish between implicit and explicit sources of memory, processing tree modelling offers a wider range of options to investigate processes involved in the acquisition and retrieval of evaluative knowledge (e.g. Kukken et al., Citation2020 this issue; Sava et al., Citation2020 this issue; see also Hütter & Klauer, Citation2016). The potential of processing tree approaches has not yet been fully exploited in research on evaluative learning (Sweldens et al., Citation2014). In particular, it is extremely promising to combine processing tree modelling with manipulations of operating conditions during acquisition in order to investigate the correspondence of processes and effects during acquisition and retrieval.
Conclusion
The phenomenon of automatic evaluative learning has fascinated researchers for decades, and it will continue to do so. In the present editorial, we offer a framework for the study of automaticity in evaluative learning that distinguishes the acquisition stage from the activation/application stage. In our review, we highlighted not only the achievements of research on evaluative learning, but also identified shortcomings regarding theoretical and empirical approaches in this field of research. The contributions to this special issue form a representative, albeit non-exhaustive collection of articles that highlight the complexities of evaluative learning and ongoing debates, and that will set the stage for a new era of theoretical and empirical development in this field of research.
Acknowledgements
We thank Olivier Corneille and Bertram Gawronski for helpful comments on a previous version of this paper.
Disclosure statement
No potential conflict of interest was reported by the authors.
Additional information
Funding
References
- Allport, G. W. (1935). Attitudes. In C. Murchison (Ed.), Handbook of social psychology (pp. 798–844). Worcester, MA: Clark University Press.
- Aust, F., Haaf, J. M., & Stahl, C. (2019). A memory-based judgment account of expectancy-liking dissociations in evaluative conditioning. Journal of Experimental Psychology: Learning, Memory, and Cognition, 45(3), 417–439.
- Bading, K., Stahl, C., & Rothermund, K. (2020). Why a standard IAT effect cannot provide evidence for associative learning: The role of similarity construction. Cognition & Emotion, 34(1), 128–143.
- Baeyens, F., Eelen, P., Crombez, G., & Van den Bergh, O. (1992). Human evaluative conditioning: Acquisition trials, presentation schedule, evaluative style and contingency awareness. Behaviour Research and Therapy, 30(2), 133–142.
- Bargh, J. A. (1994). The four horsemen of automaticity: Awareness, intention, efficiency, and control in social cognition. In R. S. J. Wyer & T. K. Srull (Eds.), Handbook of social cognition ( Vol. 1: Basic processes, pp. 1–40). Hillsdale, NJ: Erlbaum.
- Benedict, T., & Gast, A. (2020). Evaluative conditioning with fear- and disgust-evoking stimuli: No evidence that they increase learning without explicit memory. Cognition & Emotion, 34(1), 42–56.
- Blair, I. V. (2002). The malleability of automatic stereotypes and prejudice. Personality and Social Psychology Review, 6, 242–261.
- Casper, C., Rothermund, K., & Wentura, D. (2010). Automatic stereotype activation is context dependent. Social Psychology, 41, 131–136.
- Casper, C., Rothermund, K., & Wentura, D. (2011). The activation of specific facets of age stereotypes depends on individuating information. Social Cognition, 29, 393–414.
- Chaiken, S., Liberman, A., & Eagly, A. H. (1989). Heuristic and systematic processing within and beyond the persuasion context. In J. S. Uleman & J. A. Bargh (Eds.), Unintended thought (pp. 212–252). New York: Guilford.
- Chen, Z., Veling, H., Dijksterhuis, A., & Holland, R. W. (2016). How does not responding to appetitive stimuli cause devaluation: Evaluative conditioning or response inhibition? Journal of Experimental Psychology: General, 145, 1687–1701.
- Colwill, R. M., & Rescorla, R. A. (1986). Associative structures in instrumental learning. In G. H. Bower (Ed.), The psychology of learning and motivation (Vol. 20, pp. 55–104). New York: Academic Press.
- Cone, J., & Ferguson, M. J. (2015). He did what? The role of diagnosticity in revising implicit evaluations. Journal of Personality and Social Psychology, 108, 37–57.
- Conrey, F. R., Sherman, J. W., Gawronski, B., Hugenberg, K., & Groom, C. J. (2005). Separating multiple processes in implicit social cognition: The quad model of implicit task performance. Journal of Personality and Social Psychology, 89, 469–487.
- Corneille, O., Mierop, A., Stahl, C., & Hütter, M. (in press). Evidence suggestive of uncontrollable attitude acquisition replicates in an instruction-based evaluative conditioning paradigm: Implications for associative attitude formation. Journal of Experimental Social Psychology.
- Corneille, O., & Stahl, C. (2019). Associative attitude learning: A closer look at evidence and how it relates to attitude models. Personality and Social Psychology Review, 23(2), 161–189.
- Craik, F. I. M., & Tulving, E. (1975). Depth of processing and the retention of words in episodic memory. Journal of Experimental Psychology: General, 104, 268–294.
- De Houwer, J. (2006). Using the Implicit Association Test does not rule out an impact of conscious propositional knowledge on evaluative conditioning. Learning and Motivation, 37, 176–187.
- De Houwer, J. (2007). A conceptual and theoretical analysis of evaluative conditioning. The Spanish Journal of Psychology, 10, 230–241.
- De Houwer, J. (2009). The propositional approach to associative learning as an alternative for association formation models. Learning and Behavior, 37, 1–20.
- De Houwer, J. (2018). Propositional models of evaluative conditioning. Social Psychological Bulletin, 13(3). doi: 10.5964/spb.v13i3.28046
- Eberhardt, K., Esser, S., & Haider, H. (2017). Abstract feature codes: The building blocks of the implicit learning system. Journal of Experimental Psychology: Human Perception and Performance, 43(7), 1275–1290.
- Eder, A. B., & Rothermund, K. (2013). Emotional action: An ideomotor model. In C. Mohiyeddini, M. W. Eysenck, & S. Bauer (Eds.), Handbook of psychology of emotions: Recent theoretical perspectives and novel empirical findings (Vol. 1, pp. 11–38). Hauppauge, NY: Nova Science Publishers.
- Fazio, R. H. (1990). Multiple processes by which attitudes guide behavior: The MODE model as an integrative framework. In M. P. Zanna (Ed.), Advances in experimental social psychology (Vol. 23, pp. 75–109). San Diego, CA: Academic Press.
- Fazio, R. H. (2001). On the automatic activation of associated evaluations: An overview. Cognition and Emotion, 15, 115–141.
- Fazio, R. H., Jackson, J. R., Dunton, B. C., & Williams, C. J. (1995). Variability in automatic activation as an unobtrusive measure of racial attitudes: A bona fide pipeline? Journal of Personality and Social Psychology, 69, 1013–1027.
- Fazio, R. H., Sanbonmatsu, D. M., Powell, M. C., & Kardes, F. R. (1986). On the automatic activation of attitudes. Journal of Personality and Social Psychology, 50, 229–238.
- Fenske, M. J., & Raymond, J. E. (2006). Affective influences of selective attention. Current Directions in Psychological Science, 15, 312–316.
- Fiedler, K., & Bluemke, M. (2005). Faking the IAT: Aided and unaided response control on the implicit association tests. Basic and Applied Social Psychology, 27(4), 307–316.
- Fiedler, K., & Unkelbach, C. (2011). Evaluative conditioning depends on higher order encoding processes. Cognition and Emotion, 25, 639–656.
- Förderer, S., & Unkelbach, C. (2012). Hating the cute kitten or loving the aggressive pit-bull: EC effects depend on CS-US relations. Cognition & Emotion, 26, 534–540.
- Frings, C., Koch, I., Rothermund, K., Dignath, D., Giesen, C., Hommel, B., … Philipp, A. (in press). Merkmalsintegration und Abruf als wichtige Prozesse der automatischen Handlungssteuerung – eine Paradigmen-übergreifende Perspektive. Psychologische Rundschau.
- Gast, A., Richter, J., & Ruszpel, B. (2020). Is there evidence for unaware evaluative conditioning in a valence contingency learning task? Cognition & Emotion, 34(1), 57–73.
- Gast, A., & Rothermund, K. (2010). When old and frail is not the same. Dissociating category-based and stimulus-based influences on compatibility effects in four implicit measurement methods. Quarterly Journal of Experimental Psychology, 63, 479–498.
- Gast, A., & Rothermund, K. (2011a). I like it because I said that I like it: Evaluative conditioning effects can be based on stimulus-response learning. Journal of Experimental Psychology: Animal Behavior Processes, 37, 466–476.
- Gast, A., & Rothermund, K. (2011b). What you see is what will change: Evaluative conditioning effects depend on a focus on valence. Cognition and Emotion, 25, 89–110.
- Gawronski, B., & Bodenhausen, G. V. (2006). Associative and propositional processes in evaluation: An integrative review of implicit and explicit attitude change. Psychological Bulletin, 132(5), 692–731.
- Gawronski, B., & Bodenhausen, G. V. (2007). Unraveling the processes underlying evaluation: Attitudes from the perspective of the APE model. Social Cognition, 25, 687–717.
- Gawronski, B., & Bodenhausen, G. V. (2009). Operating principles versus operating conditions in the distinction between associative and propositional processes. Behavioral and Brain Sciences, 32, 207–208.
- Gawronski, B., & Bodenhausen, G. V. (2014). Implicit and explicit evaluation: A brief review of the associative–propositional evaluation model. Social and Personality Psychology Compass, 8(8), 448–462.
- Gawronski, B., & Bodenhausen, G. V. (2018). Evaluative conditioning from the perspective of the associative-propositional evaluation model. Social Psychological Bulletin, 13(3), e28024.
- Gawronski, B., & De Houwer, J. (2014). Implicit measures in social and personality psychology. In H. T. Reis & C. M. Judd (Eds.), Handbook of research methods in social and personality psychology (2nd ed., pp. 283–310). New York, NY: Cambridge University Press.
- Giesen, C., Schmidt, J. R., & Rothermund, K. (in press). The law of recency: An episodic stimulus-response retrieval account of habit acquisition. Frontiers in Psychology. doi: 10.3389/fpsyg.2019.02927
- Greenwald, A. G., & Banaji, M. R. (2017). The implicit revolution: Reconceiving the relation between conscious and unconscious. American Psychologist, 72(9), 861–871.
- Greenwald, A. G., & De Houwer, J. (2017). Unconscious conditioning: Demonstration of existence and difference from conscious conditioning. Journal of Experimental Psychology: General, 146, 1705–1721.
- Greenwald, A. G., McGhee, D. E., & Schwartz, J. K. L. (1998). Measuring individual differences in implicit cognition: The Implicit Association Test. Journal of Personality and Social Psychology, 74, 1464–1480.
- Hermans, D., Vansteenwegen, D., Crombez, G., Baeyens, F., & Eelen, P. (2002). Expectancy-learning and evaluative learning in human classical conditioning: Affective priming as an indirect and unobtrusive measure of conditioned stimulus valence. Behaviour Research and Therapy, 40(3), 217–234.
- Heycke, T., & Gawronski, B. (in press). Co-occurrence and relational information in evaluative learning: A multinomial modeling approach. Journal of Experimental Psychology: General.
- Heycke, T., Gehrmann, S., Haaf, J., & Stahl, C. (2018). Of two minds or one? A registered replication of Rydell et al. (2006). Cognition and Emotion, 32(8), 1708–1727.
- Hofmann, W., De Houwer, J., Perugini, M., Baeyens, F., & Crombez, G. (2010). Evaluative conditioning in humans: A meta-analysis. Psychological Bulletin, 136(3), 390–421.
- Högden, F., Stahl, C., & Unkelbach, C. (2020). Similarity-based and rule-based generalization in the acquisition of attitudes via evaluative conditioning. Cognition & Emotion, 34(1), 105–127.
- Hughes, S., Ye, Y., Van Dessel, P., & De Houwer, J. (2019). When people co-occur with good or bad events: Graded effects of relational qualifiers on evaluative conditioning. Personality and Social Psychology Bulletin, 45, 196–208.
- Huijding, J., Muris, P., Lester, K. J., Field, A. P., & Joosse, G. (2011). Training children to approach or avoid novel animals: Effects on self-reported attitudes and fear beliefs and information-seeking behaviors. Behaviour Research and Therapy, 49, 606–613.
- Hutchings, R., Calanchini, J., Huang, L., Rees, H., Rivers, A., Roth, J., & Sherman, J. (2020). Retrieval cues fail to influence contextualized evaluations. Cognition & Emotion, 34(1), 86–104.
- Hütter, M., & De Houwer, J. (2017). Examining the contributions of memory-dependent and memory-independent components to evaluative conditioning via instructions. Journal of Experimental Social Psychology, 71, 49–58.
- Hütter, M., & Klauer, K. C. (2016). Applying processing trees in social psychology. European Review of Social Psychology, 27, 116–159.
- Hütter, M., & Sweldens, S. (2013). Implicit misattribution of evaluative responses: Contingency-unaware evaluative conditioning requires simultaneous stimulus presentations. Journal of Experimental Psychology: General, 142(3), 638–643.
- Hütter, M., Sweldens, S., & Andrade, E. (2018). Dissociating controllable and uncontrollable effects of affective stimuli on attitudes and consumption. Journal of Consumer Research, 45(2), 320–349.
- Hütter, M., Sweldens, S., Stahl, C., Unkelbach, C., & Klauer, K. C. (2012). Dissociating contingency awareness and conditioned attitudes: Evidence of contingency–unaware evaluative conditioning. Journal of Experimental Psychology: General, 141(3), 539–557.
- Jacoby, L. L. (1991). A process dissociation framework: Separating automatic from intentional uses of memory. Journal of Memory and Language, 30, 513–541.
- Jones, C. R., Fazio, R., & Olson, M. (2009). Implicit misattribution as a mechanism underlying evaluative conditioning. Journal of Personality and Social Psychology, 96(5), 933–948.
- Jones, C. R., Olson, M. A., & Fazio, R. H. (2010). Evaluative conditioning: The “how” question. Advances in Experimental Social Psychology, 43, 205–255.
- Kahneman, D. (2011). Thinking, fast and slow. New York: Farrar, Straus, and Giroux.
- Karpinski, A., & Hilton, J. L. (2001). Attitudes and the implicit association test. Journal of Personality and Social Psychology, 81, 774–788.
- Klauer, K. C., Voss, A., Schmitz, F., & Teige-Mocigemba, S. (2007). Process components of the Implicit Association Test: A diffusion-model analysis. Journal of Personality and Social Psychology, 93, 353–368.
- Kukken, N., Hütter, M., & Holland, R. (2020). Are there two independent evaluative conditioning effects in relational paradigms? Dissociating the effects of CS-US pairings and their meaning. Cognition & Emotion, 34(1), 170–187.
- Lazarus, R. S. (1984). On the primacy of cognition. American Psychologist, 39, 124–129.
- Leventhal, H., & Scherer, K. (1987). The relationship of emotion to cognition: A functional approach to a semantic controversy. Cognition and Emotion, 1, 3–28.
- Lustig, C., May, C. P., & Hasher, L. (2001). Working memory span and the role of proactive interference. Journal of Experimental Psychology: General, 130, 199–207.
- McConnell, A. R., & Rydell, R. J. (2014). The systems of evaluation model: A dual-systems approach to attitudes. In J. W. Sherman, B. Gawronski, & Y. Trope (Eds.), Dual-process theories of the social mind (pp. 204–218). New York: Guilford.
- Meissner, F., Grigutsch, L. A., Koranyi, N., Müller, F., & Rothermund, K. (2019). Predicting behavior with implicit measures: Disillusioning findings, reasonable explanations, and sophisticated solutions. Frontiers in Psychology, 10. doi: 10.3389/fpsyg.2019.02483
- Meissner, F., & Rothermund, K. (2013). Estimating the contributions of associations and recoding in the Implicit Association Test: The ReAL model for the IAT. Journal of Personality and Social Psychology, 104, 45–69.
- Meissner, F., & Rothermund, K. (in press). Increasing the validity of implicit measures: New solutions for assessment, conceptualization, and action explanation. In J. A. Krosnick (Ed.), Implicit bias. New York: Cambridge University Press.
- Melnikoff, D. E., & Bargh, J. A. (2018). The mythical number two. Trends in Cognitive Sciences, 22(4), 280–293.
- Mierop, A., Hütter, M., Stahl, C., & Corneille, O. (2019). Does attitude acquisition in evaluative conditioning without explicit CS-US memory reflect implicit misattribution of affect? Cognition and Emotion, 33(2), 173–184.
- Mitchell, C. J., De Houwer, J., & Lovibond, P. (2009). The propositional nature of human associative learning. Behavioral and Brain Sciences, 32, 183–198.
- Montoya, R. M., Horton, R. S., Vevea, J. L., Citkowicz, M., & Lauber, E. A. (2017). A re-examination of the mere exposure effect: The influence of repeated exposure on recognition, familiarity, and liking. Psychological Bulletin, 143(5), 459–498.
- Moors, A. (2014). Examining the mapping problem in dual process models. In J. W. Sherman, B. Gawronski, & Y. Trope (Eds.), Dual process theories of the social mind (pp. 20–34). New York, NY: Guilford Press.
- Moors, A., & De Houwer, J. (2006). Automaticity: A theoretical and conceptual analysis. Psychological Bulletin, 132, 297–326.
- Moran, T., & Bar-Anan, Y. (2013). The effect of object–valence relations on automatic evaluation. Cognition & Emotion, 27(4), 743–752.
- Moran, T., & Bar-Anan, Y. (2020). The effect of co-occurrence and relational information on speeded evaluation. Cognition & Emotion, 34(1), 144–155.
- Müller, F., & Rothermund, K. (2019). The Propositional Evaluation Paradigm (PEP): indirect assessment of personal beliefs and attitudes. Frontiers in Psychology, 10. doi: 10.3389/fpsyg.2019.02385
- Olson, M. A., & Fazio, R. H. (2004). Reducing the influence of extrapersonal associations on the Implicit Association Test: Personalizing the IAT. Journal of Personality and Social Psychology, 86, 653–667.
- Payne, B. K., Burkley, M., & Stokes, M. B. (2008). Why do implicit and explicit attitude tests diverge? The role of structural fit. Journal of Personality and Social Psychology, 94, 16–31.
- Payne, B. K., Cheng, C. M., Govorun, O., & Stewart, B. D. (2005). An inkblot for attitudes: Affect misattribution as implicit measurement. Journal of Personality and Social Psychology, 89, 277–293.
- Payne, B. K., Hall, D. L., Cameron, C. D., & Bishara, A. J. (2010). A process model of affect misattribution. Personality and Social Psychology Bulletin, 36, 1397–1408.
- Petty, R. E., & Briñol, P. (2012). The elaboration likelihood model. In P. A. M. Van Lange, A. Kruglanski, & E. T. Higgins (Eds.), Handbook of theories of social psychology (Vol. 1, pp. 224–245). London, UK: Sage.
- Petty, R. E., & Cacioppo, J. T. (1986). The elaboration likelihood model of persuasion. In L. Berkowitz (Ed.), Advances in experimental social psychology (Vol. 19, pp. 123–205). New York: Academic Press.
- Platt, J. R. (1964). Strong inference: Certain systematic methods of scientific thinking may produce much more rapid progress than others. Science, 146, 347–353.
- Pleyers, G., Corneille, O., Luminet, O., & Yzerbyt, V. (2007). Aware and (dis)liking: Item-based analyses reveal that valence acquisition via evaluative conditioning emerges only when there is contingency awareness. Journal of Experimental Psychology: Learning, Memory, and Cognition, 33, 130–144.
- Pleyers, G., Corneille, O., Yzerbyt, V., & Luminet, O. (2009). Evaluative conditioning may incur attentional costs. Journal of Experimental Psychology: Animal Behavior Processes, 35, 279–285.
- Ranganath, K. A., Smith, C. T., & Nosek, B. A. (2008). Distinguishing automatic and controlled components of attitudes from direct and indirect measurement methods. Journal of Experimental Social Psychology, 44, 386–396.
- Raymond, J. E., Fenske, M. J., & Tavassoli, N. T. (2003). Selective attention determines emotional responses to novel visual stimuli. Psychological Science, 14, 537–542.
- Raymond, J. E., Fenske, M. J., & Westoby, N. (2005). Emotional devaluation of distracting patterns and faces: A consequence of attentional inhibition during visual search? Journal of Experimental Psychology: Learning, Memory, and Cognition, 31, 1404–1415.
- Reber, R., Winkielman, P., & Schwarz, N. (1998). Effects of perceptual fluency on affective judgments. Psychological Science, 9, 45–48.
- Rothermund, K., Teige-Mocigemba, S., Gast, A., & Wentura, D. (2009). Eliminating the influence of recoding in the Implicit Association Test: The Recoding-Free Implicit Association Test (IAT-RF). Quarterly Journal of Experimental Psychology, 62, 84–98.
- Rydell, R. J., & McConnell, A. R. (2006). Understanding implicit and explicit attitude change: A systems of reasoning analysis. Journal of Personality and Social Psychology, 91(6), 995–1008.
- Sava, F. A., Payne, B. K., Măgurean, S., Iancu, D. E., & Rusu, A. (2020). Beyond contingency awareness: The role of influence awareness in resisting conditioned attitudes. Cognition & Emotion, 34(1), 156–169.
- Schmidt, J. R., & De Houwer, J. (2012). Contingency learning with evaluative stimuli: Testing the generality of contingency learning in a performance paradigm. Experimental Psychology, 59, 175–182.
- Schmidt, J. R., Giesen, C., & Rothermund, K. (in press). Contingency learning as binding? Testing an exemplar view of the colour-word contingency learning effect. Quarterly Journal of Experimental Psychology.
- Schwarz, N., & Clore, G. L. (1983). Mood, misattribution, and judgments of well-being: Informative and directive functions of affective states. Journal of Personality and Social Psychology, 45, 513–523.
- Smith, C. T., Calanchini, J., Hughes, S., Van Dessel, P., & De Houwer, J. (2020). The impact of instruction- and experience-based evaluative learning on IAT performance: A Quad model perspective. Cognition & Emotion, 34(1), 21–41.
- Stahl, C., & Aust, F. (2018). Evaluative conditioning as memory-based judgment. Social Psychological Bulletin, 13(3), e28589.
- Stahl, C., Haaf, J., & Corneille, O. (2016). Subliminal evaluative conditioning? Above-chance CS identification may be necessary and insufficient for attitude learning. Journal of Experimental Psychology: General, 145, 1107–1131.
- Steffens, M. C. (2004). Is the implicit association test immune to faking? Experimental Psychology, 51, 165–179.
- Strack, F., & Deutsch, R. (2004). Reflective and impulsive determinants of social behavior. Personality and Social Psychology Review, 8, 220–247.
- Sweldens, S., Corneille, O., & Yzerbyt, V. (2014). The role of awareness in attitude formation through evaluative conditioning. Personality and Social Psychology Review, 18(2), 187–209.
- Sweldens, S., Tuk, M. A., & Hütter, M. (2017). How to study consciousness in consumer research, a commentary on Williams and Poehlman. Journal of Consumer Research, 44, 266–275.
- Sweldens, S., van Osselaer, S. M. J., & Janiszewski, C. (2010). Evaluative conditioning procedures and the resilience of conditioned brand attitudes. Journal of Consumer Research, 37(3), 473–489.
- Teige-Mocigemba, S., & Klauer, K. C. (2013). On the controllability of evaluative-priming effects: Some limits that are none. Cognition and Emotion, 27, 632–657.
- Teige-Mocigemba, S., Klauer, K. C., & Rothermund, K. (2008). Minimizing method-specific variance in the IAT: A single block IAT. European Journal of Psychological Assessment, 24, 237–245.
- Van Dessel, P., Cone, J., Gast, A., & De Houwer, J. (2020). The impact of valenced verbal information on implicit and explicit evaluation: The role of information diagnosticity, primacy, and memory cueing. Cognition & Emotion, 34(1), 74–85.
- Van Dessel, P., De Houwer, J., Gast, A., & Tucker Smith, C. (2015). Instruction-based approach-avoidance effects: Changing stimulus evaluation via the mere instruction to approach or avoid stimuli. Experimental Psychology, 62, 161–169.
- Van Dessel, P., Eder, A., & Hughes, S. J. (2018). Mechanisms underlying effects of approach-avoidance training on stimulus evaluation. Journal of Experimental Psychology: Learning, Memory, and Cognition, 44, 1224–1241.
- Van Dessel, P., Hughes, S., & De Houwer, J. (2018). Consequence-based approach-avoidance training: A new and improved method for changing behavior. Psychological Science, 29(12), 1899–1910.
- Walther, E., Halbeisen, G., & Blask, K. (2018). What you feel is what you see: A binding perspective on evaluative conditioning. Social Psychological Bulletin, 13(3), e27551.
- Wentura, D., & Rothermund, K. (2014). Priming is not priming is not priming. Social Cognition, 32, 47–67.
- Wigboldus, D. H. J., Dijksterhuis, A., & van Knippenberg, A. (2003). When stereotypes get in the way: Stereotypes obstruct stereotype-inconsistent trait inferences. Journal of Personality and Social Psychology, 84, 470–484.
- Wiswede, D., Koranyi, N., Müller, F., Langner, O., & Rothermund, K. (2013). Validating the truth of propositions: Behavioral and ERP indicators of truth evaluation processes. Social Cognitive and Affective Neuroscience, 8, 647–653.
- Wittenbrink, B., Judd, C. M., & Park, B. (2001). Spontaneous prejudice in context: Variability in automatically activated attitudes. Journal of Personality and Social Psychology, 81, 815–827.
- Woud, M. L., Maas, J., Becker, E. S., & Rinck, M. (2013). Make the manikin move: Symbolic approach–avoidance responses affect implicit and explicit face evaluations. Journal of Cognitive Psychology, 25, 738–744.
- Zajonc, R. B. (1968). Attitudinal effects of mere exposure. Journal of Personality and Social Psychology, 9(2, Pt. 2), 1–27.
- Zajonc, R. B. (1980). Feeling and thinking: Preferences need no inferences. American Psychologist, 35, 151–175.
- Zajonc, R. B. (1984). On the primacy of affect. American Psychologist, 39, 117–123.