ABSTRACT
Insight experiences are sudden, persuasive, and can accompany valuable new ideas in science and art. In this preregistered experiment, we aim to validate a novel visceral and continuous measure of insight problem solving and to test whether real-time and embodied feelings of insight can predict correct solutions. We report several findings. Consistent with recent work, we find a strong positive relationship between Aha moments and accuracy for problems that demand implicit processing. We also found that the intensity of the insight experience further predicted the accuracy of solutions and participants naturally embodied the intensity of their insight experiences by squeezing the dynamometer more tightly. Intriguingly, this unintentional embodiment further predicted the accuracy of solutions. We suggest that the dynamometer complements previous measures by (1) simultaneously capturing both process and feeling in real-time, (2) highlights the value of measuring Aha moments on a continuum of intensity, and (3) firmly establishes that the impulsive feeling of Aha can carry information about the veracity of an idea. We discuss the findings in light of a recent theoretical account of how feelings of insight may act as a heuristic to select ideas from the stream of consciousness.
Introduction
The psychological phenomena that interest humans the most are often deeply and fundamentally subjective; take for example, happiness, love, meaning, or consciousness. The challenge of understanding these phenomena – particularly in pursuing them scientifically – often lies in their subjective and ephemeral nature. This challenge is extant for the sudden feeling of insight, an enigmatic phenomenon where a solution to a problem, or a new idea, appears in the mind of its pursuer as if “out of nowhere”, immediately filling them with certainty about its truthfulness and value (Schooler & Melcher, Citation2009). Despite the ephemeral character of insight, researchers have been collecting data and pursuing a scientific explanation for such “sudden knowing” experiences for at least the past century (Köhler, Citation1921; Sternberg & Davidson, Citation1994). Although considerable progress has been made, there remain challenges to effectively working with insight experiences in laboratory settings. In this paper, we outline a new embodied and real-time measure of insight using a dynamometer that we believe captures the key features of an insight experience: its cognitive qualities (sudden and unexpected) as well as its affective or phenomenological quality (the Aha experience). As an application of this new tool, we also aim to complement previous research examining whether real-time and embodied insight experiences – and their intensity – are predictive of accurate ideas.
The Many faces of insight
To measure insights in the laboratory demands that we have a clear definition, but previous research has been somewhat heterogeneous in this regard (Laukkonen & Tangen, Citation2018). To integrate inconsistencies in the literature, Öllinger and Knoblich (Citation2009) proposed that insight can be defined as: (1) a particular cognitive process – i.e. a metacognitively sudden solution following a shift in perspective or representational change (Ohlsson, Citation1984), (2) a unique set of problem-solving tasks – i.e. problems that tend to elicit sudden solutions, and (3) a specific phenomenological state – i.e. the feeling of Aha that accompanies some sudden solutions.
The different methods of capturing and defining insight have also changed over time. Metcalfe and Wiebe (Citation1987) focused on measuring the cognitive-process dimension of insight employing a “feelings-of-warmth” measure that tracks metacognitions during problem solving. Here, a more metacognitively unexpected solution hints at a different cognitive process than a metacognitively predictable one (i.e. solving processes leading to solution were or were not available to introspection). In recent years, prompted by the desire to catch many insight experiences with compact problems in physiological and neuroimaging research, it became common practice to measure the phenomenological aspect of insight – i.e. the Aha experience (e.g. Bowden & Jung-Beeman, Citation2007; Kounios et al., Citation2006; Salvi et al., Citation2015). However, as yet there is no method that captures both the cognitive (sudden and unexpected) and the phenomenological (Aha! Experience) aspects of insight simultaneously.
Like Öllinger and Knoblich (Citation2009), Danek (Citation2018) emphasised that there is likely no single condition that can define insight, but that both the cognitive process and the affective components are necessary to speak of insight. Danek (Citation2018) also suggested that a “true insight” ought to be correct. Indeed, an exciting recent finding is that Aha experiences tend to correlate with accurate solutions to problems (Danek et al., Citation2014b, Citation2020; Danek & Wiley, Citation2017; Hedne et al., Citation2016; Salvi, Bricolo, et al., Citation2016; Threadgold et al., Citation2018; Webb et al., Citation2016, Citation2018), which we discuss further below.
In this paper we therefore assume that both the cognitive process (i.e. sudden and unexpected) as well as affective components (i.e. the Aha experience) together play a role in what we may call “insight”. We also align ourselves with more recent neuroimaging research, wherein the same problems can be solved either with or without insight, and thus needs to be captured on a case by case basis (Bowden & Jung-Beeman, Citation2007; Kounios & Beeman, Citation2014). In order to show how the dynamometer – a highly sensitive continuous measure of grip-strength (Creswell et al., Citation2016) – extends on existing tools for capturing insight, below we provide an overview of the self-report and warmth measures of insight.
Feelings of warmth: the cognitive dimension of insight
The warmth measure of insight assumes that humans have some metacognitive sense of how they are progressing during problem solving (Metcalfe, Citation1986; Metcalfe & Wiebe, Citation1987). Metcalfe (Citation1986) originally used “warmth” as an intuitive spectrum of progress, where a cold state indicates that one is far away from the solution and a hot state indicates the feeling that one is close to the solution. Thus, while solving the problem, a participant can be occasionally prompted to make a warmth rating. Perceived progress on the problem can then be estimated by warmth ratings over time. The cognitive dimension of insight, i.e. sudden or unexpected solutions, can then be inferred by a relatively sudden shift from a cold state to a solution state (e.g. in a matter of seconds).
In their study, Metcalfe and Wiebe (Citation1987) compiled insight problems and another set that was previously categorised as multi-step problems (termed analytic problems). The authors found that insight problems elicited more sudden transitions from cold states to solution states than the analytic problems, which showed more gradual warmth ratings preceding the solution. Metcalfe and Wiebe (Citation1987) recommended that insight therefore “ … be defined in terms of the antecedent phenomenology that may be monitored by metacognitive assessments by the subject” (p. 243). Although the warmth measure was an important contribution and one of the first objective demonstrations of the insight phenomenon, there are both practical and theoretical limitations (Laukkonen & Tangen, Citation2018; see Weisberg, Citation1992 for an early commentary on limitations of the warmth measure).
First, and as discussed earlier, insight solutions can have varying affective qualities including pleasure, relief, a sense of certainty, and surprise (Danek & Wiley, Citation2017; Webb et al., Citation2016). Second, gradual warmth patterns do not preclude the presence of a sudden solution because progress on a problem can be illusory. Participants can sense that they are making progress on a problem and then experience a sudden and unexpected solution, so long as the perceived progress was unrelated to the final solution. Consider the following insight problem:
A young boy turned off the lights in his bedroom and managed to get into bed before the room was dark. If the bed is ten feet from the light switch and the light bulb and he used no wires, strings, or other contraptions to turn off the light, how did he do it?
A few practical limitations of the warmth measure are also worth mentioning. In previous research, researchers retrieve no more than one data point every ten or fifteen seconds (Chein et al., Citation2010; Laukkonen & Tangen, Citation2017; Metcalfe & Wiebe, Citation1987), which is problematic because insights are sudden and immediate, so even ten seconds may provide a meaningful window to experience progress on a problem. Many problems can also be solved very quickly, and without at least two ratings it is not possible to infer what progress (if any) was experienced, meaning that several trials provide no useful data points. A more continuous measure – such as the dynamometer – would make it easier to capture rapidly changing metacognitions.
Self-report: the phenomenological dimension of insight
Aside from the warmth measure, another popular contemporary measure of insight is self-report (e.g. Aziz-Zadeh et al., Citation2009; Bowden et al., Citation2005; Danek et al., Citation2014a; Salvi et al., Citation2015; Salvi, Beeman, et al., Citation2020, Citation2020a, Citation2020b). The self-report method is easier to administer, it captures the affective or phenomenological qualities of the insight experience, and it is more amenable to neuroimaging (Aziz-Zadeh et al., Citation2009; Bowden & Jung-Beeman, Citation2007; Kounios et al., Citation2006; Luo et al., Citation2004). Capturing Aha moments via self-reports usually involves the administration of a set of problems where, after a solution is provided, the participant indicates whether that particular solution was accompanied by an Aha experience. The Aha experience can include various dimensions such as confidence, obviousness, pleasure (Webb et al., Citation2018), surprise, relief, and drive (Danek & Wiley, Citation2017). The self-report measure also clearly maps onto different physiological markers, including eye blinks, pupil dilation, and neural activity (Kounios et al., Citation2006, Citation2008; Salvi & Bowden, Citation2016; Salvi et al., Citation2015, Citation2020a, Citation2020b). Nevertheless, there are also limitations to the self-report measure.
One limitation is that the self-report measure relies on a retrospective judgment that demands a post-hoc introspection. We have previously argued (see Laukkonen & Tangen, Citation2018), that an issue herein is inconsistencies in the phenomenological definition of insight. For instance, Cushen and Wiley (Citation2012) relied on just two dimensions (surprise and suddenness). Other more contemporary work used a more multidimensional description, including features like pleasure, certainty, and relief (e.g. Danek & Wiley, Citation2017; Jung-Beeman et al., Citation2004; Webb et al., Citation2016). And yet others have tried to provide a more minimal description of the Aha experience, relying more on participants lay sense of what an Aha moment is (e.g. Salvi et al., Citation2016; Laukkonen & Tangen, Citation2017; Citation2020). Clearly, how an Aha moment is described to participants will have an impact on what is thereby recorded in the experiment.
Retrospective self-reports are also problematic for experiments interested in comparing Aha moments to other behavioural or metacognitive outcomes. Several recent studies have found that when Aha moments occur, the solution is likely to be correct (Danek et al., Citation2014b, Citation2020; Danek & Wiley, Citation2017; Hedne et al., Citation2016; Salvi, Bricolo, et al., Citation2016; Threadgold et al., Citation2018; Webb et al., Citation2016, Citation2018, Citation2019). However, the positive association between verbally reported Aha moments and accuracy could be influenced by the relationship between confidence and accuracy, or some other metacognitive reflection prior to reporting (although see below discussion and Danek & Salvi, Citation2020). For example, a participant could initially experience an Aha moment, but realise upon reflection that their answer is wrong, and then change their mind about having experienced Aha (since Aha moments are meant to be correct). This pattern of responding would artificially lead self-reported Aha experiences to be associated with correct responses, but not because of the feeling.
Nevertheless, there is some evidence that the Aha and accuracy relationship is not simply driven by confidence. In two studies, Aha moments predicted accurate problem solving without mentioning confidence in the description (Hedne et al., Citation2016; Salvi et al., Citation2016). Given these results, Danek and Salvi (Citation2020) argued that “ … it seems unlikely that the accuracy effect is solely based on high confidence serving as a cue for high Aha! ratings” (p. 485). While we agree that this is likely the case, it is plausible that participants’ own intuitive definition of an Aha moment includes confidence. For instance, Salvi et al. (Citation2016) examined to what extent insight moments versus analytic (gradual, step-by-step) were accurate. They found that compound remote associates (CRA), anagrams, rebus puzzles, and even a visual problem task (involving the discovery of an object in a noisy or ambiguous image), all showed greater accuracy when solved via insight. In the experiment, “Solving a CRA problem via insight was described as the answer suddenly coming to mind, being somewhat surprising, and with the participant having difficulty stating how the solution was obtained (‘feeling like a small Aha! Moment’)” (Salvi et al., Citation2016, p. 6–7). Thus, although there was no mention of confidence in this study, nevertheless mentioning an Aha moment may naturally prompt the problem solver to look out for solutions that feel confident, since this is a natural phenomenological characteristic of the Aha experience according to both Webb et al. (Citation2018) and Danek & Wiley (Citation2017). Thus, excluding confidence from the description may not automatically change the participant’s sense that Aha moments ought to be accurate and accompanied by confidence. It also does not account for the possibility that participants report Aha moments when they believe they have found a correct solution, regardless of felt confidence.
Crucially, we expect that consistent with previous work, the Aha moment does genuinely carry information about the accuracy of a solution, hence making them more likely to be correct (Laukkonen et al., Citation2018, Citation2020). Thus, it is our hope that using the dynamometer will clear up any lingering doubts about the relationship between insight and accuracy, and demonstrate that this exciting finding is robust. Using the dynamometer, discussed further below, we aim to strengthen previous work to show that indeed the insight experience, even when captured in real-time, can predict accurate solutions.
The best of both phenomenology and cognition: an embodied real-time measure
Here we suggest that a new tool, which captures both the cognitive and affective dimensions of insight, in real-time, as well as the intensity of the insight experience, can help to extend on previous work and to affirm the robust finding that Aha moments tend to be correct.
A dynamometer is a highly sensitive handheld measure of grip strength that can be used to capture moment-to-moment fluctuations in cognitive states. In one study, Creswell et al. (Citation2016) used the dynamometer for measuring hunger because they argued that the device’s non-verbal nature makes it less subject to the limitations of language and less likely to interfere with other task demands. The authors found that the dynamometer was a better predictor of actual eating behaviour than verbal reports. They also observed “verbal overshadowing”; if participants verbalised hunger and used the dynamometer, the dynamometer was no longer predictive of eating behaviour. Creswell et al. (Citation2016) argue that the participants were “losing touch” with their feelings due to the concurrent verbalising, potentially explaining why the dynamometer was a better predictor than verbal-reports. In a subsequent study Creswell et al. (Citation2019) demonstrated that the dynamometer was similarly useful in elucidating craving states associated with cigarettes. It may be that the problem solving domain,and particularly research on the subjective Aha experience,could likewise benefit from a more visceral and embodied measure that does not interfere with task demands.
Considering the limitations of the warmth measure and verbal reports, an ideal measure would capture perceived progress continuously without interfering with the task and capture the sudden onset of a solution, as well as the “Aha” experience in real-time (effectively capturing the strengths of both feelings-of-warmth and the self-report measure while avoiding the limitations). To this end, the dynamometer is a promising candidate.
Many theoretical frameworks also propose that feelings carry valuable information that aid decision-making in uncertain contexts (Damasio, Citation1996; Schwarz, Citation2012; Slovic et al., Citation2007). Consistent with these theories, we have recently proposed that the feeling of insight may act as a kind of informative “intuition” that aids the selection of new ideas (Laukkonen et al., Citation2018, Citation2020). For instance, we recently demonstrated that artificially elicited insight experiences could make facts (both true and false) appear true (Laukkonen et al., Citation2020). Thus, in addition to integrating existing measures, we hope to capture the insight experience with high sensitivity, fidelity, and prior to any opportunity of reflection, to remove any doubt that the feeling of Aha is an informative marker of an accurate solution. Crucially, although previous work indicates that insights tend to be correct, false insights do of course occur (see Ohlsson, Citation1984; Danek & Wiley, Citation2017).
Hypotheses
Using the dynamometer to capture problem solving progress and real-time Aha moments, we expected that analytic problems will elicit more gradual warmth ratings, and classical insight problems and remote associates ought to show more sudden transitions from cold states to solution states indicating more unexpected solutions (in line with Metcalfe & Wiebe, Citation1987). In other words, analytic problems should elicit a greater average increase in grip-strength over time, compared to insight and compound remote associate problems. Analytic problems were expected to elicit the fewest Aha experiences (spikes in the dynamometer), followed by compound remote associates, and insight problems were expected to elicit the most (Webb et al., Citation2016).
To check for convergence, self-reported insight moments were also expected to have a moderate to strong correlation with spikes in the dynamometer output (i.e. full strength squeezes), and show the same pattern of results across the problem types. Crucially, dynamometer spikes (i.e. real-time Aha moments) were expected to predict more accurate solutions and higher confidence in the solution. A positive association between real-time dynamometer insights and objective performance would be an important step towards confirming a genuine relationship between Aha moments and accurate solutions.
Methods
Participants, design and materials
This experiment was approved by The University of Queensland Human Research Ethics Committee (UQHREC), clearance number: 17-PSYCH-141-4-AH, and conducted in accordance with the Declaration of Helsinki. Sixty participants (20 males, 40 females; mean age = 19.73, SD = 3.87) were tested in exchange for course credit, which is a sufficient sample to detect medium effect sizes observed in similar research (e.g. Salvi et al., Citation2016), and we calculated that the power to determine an effect size of .5 with n = 60 is 0.968. Some of the problems involved language cues or cultural references so participation was restricted to native English speakers. In a fully within-participants design, participants were presented with the same 30 problems encompassing 10 insight problems, 10 analytic problems, and 10 compound remote associates (CRA). The problems (see Appendix B for the full set) were printed on 30 individual cards and presented to participants randomly, one-by-one. We chose these problems because they are the most commonly used in insight research (Bowden & Jung-Beeman, 2003; Citation2007; Chu & MacGregor, Citation2011; Cushen & Wiley, Citation2012; Metcalfe & Wiebe, Citation1987; Salvi et al., Citation2015; Webb et al., Citation2016). Participants were given a maximum of 90 s to attempt each problem. We didn’t record when the problem was solved, as participants were focused on their dynamometer ratings, and only provided their verbal response once they had followed the dynamometer protocol (which would lead to variable delays based on both the verbal report and recording by the experimenter). All materials, the experimental programme, and finer details for replication can be accessed on the Open Science Framework (https://osf.io/p6gqe).
The dynamometer was the same model used by Creswell et al. (Citation2016), developed by Vernier. The device records grip strength 10 times per second and displays the data graphically as a function of time and Newtons (N) of pressure. The device has an accuracy of ±0.6 N and an operating range between 0 and 600 N. The data are recorded on a computer using Logger Lite 1.9.1, and the ratings were recorded using the free open source program LiveCode Community 7.0.6 on another computer. Participants were asked to report whether an Aha moment occurred (yes or no), and if yes, how intense the experience was on a 12-point scale where 1 is mild and 12 is intense. They also reported confidence in the solution on a 12-point scale where 1 is “not at all confident” and 12 is “very confident.” Participants also completed the Mindful Attention Awareness Scale (Brown & Ryan, Citation2003), the results of which will be reported elsewhere.
Procedure
Subjects were tested individually in a one-hour session and were provided with written instructions overviewing the experiment and explaining how to respond to the questions. These instructions can be found in Appendix A. In the instructions, we deliberately avoided mentioning the affective qualities of Aha and made no mention of confidence as a dimension of Aha moments. Aside from the brief description of the Aha moment, the instructions stated that participants would need to rate the subjective intensity of their Aha moments, as well as their confidence in the accuracy of their solutions. We reasoned that by instructing participants to separately rate their feelings of Aha and confidence, participants will separate their feelings of confidence from their feelings of Aha and hopefully mitigate the potential confidence confound discussed earlier. In the transcript, participants were also instructed on how to use the dynamometer to express their feelings of closeness to the solution as well as any potential insight moments. They were told to grip the dynamometer in their dominant hand and squeeze the device according to how close they feel they are to solving the problem. They were told to do this up until they arrive at a solution, at which point they give the device a full-strength squeeze if they experienced an Aha moment. If no Aha moment occurred, they were told to simply relax their grip. They were then asked to verbalise their solution to each problem, with a maximum of 90 s allowed to solve the problem. A contingency matrix showing the four possible combinations of problem-solving progress and insight experiences is illustrated in .
Table 1. Four possible combinations of problem solving progress and insight that can be detected by the dynamometer.
Following the instructions, participants were asked to grip the dynamometer and imagine that they are solving a problem. They were shown the output graph using the Logger Lite software and asked to gradually increase and decrease their grip strength, and to simulate a full-strength Aha moment so that they can see how their actions are depicted on the graph. This procedure aimed to help the participants develop a greater understanding of the task and increase attention to their feelings of closeness when solving the problems. The 30 problems were then presented one-by-one in a randomised order.
After each response, participants were asked a set of meta-cognitive questions. They were asked to rate their confidence in their answer from 1 to 12, 1 being “not at all confident” and 12 being “very confident”. They were then asked whether they experienced an Aha moment and, if so, they were asked to rate the intensity of the Aha moment from mild to intense, also on a 1–12 scale. The order of the Aha moment and confidence questions were counterbalanced. Finally, participants completed the Mindful Attention Awareness Scale (MAAS; Brown & Ryan, Citation2003).
Results
Descriptives and preprocessing
Since no participants were removed, there was a total of 1800 responses to problems (60 participants and 30 problems per participant), and also 1800 dynamometer responses. A total of 1278 of the data points were used for analysis because participants rated 13 of the problems as familiar, and participants failed to provide an answer before timing out in 509 cases. For analyses of the accuracy of Aha moments, there were slightly fewer observations (e.g. some participants did not have any Aha moments in the analytic condition and thus there were no accuracy data for that condition for that participant).
The dynamometer data were standardised in order to detect spikes (i.e. full strength squeezes representing insight moments). Informed by a visual analysis of all trials by three independent raters (α = .96), we chose 6 SD above the mean as an appropriate cut off to capture spikes during problem solving. In the preregistration, we planned to use differential warmth (i.e. the difference between the first warmth rating and the last warmth rating) to measure perceived progress. However, we decided against this method because the dynamometer spikes recorded at the end of some trials would directly bias the analysis. Instead, the independent raters also coded the dynamometer patterns according to the size of the slope prior to solution or spike. They blindly rated the slope of every dynamometer pattern as 0 (no slope), 1 (some slope), or 2 (steep slope) in different random orders, again showing high inter-rater reliability, α = .88. The three ratings for each graph were averaged so that each trial had a single slope value ranging from 0 to 2. Since there is no generally accepted objective criterion for evaluating progress prior to solution (particularly with the dynamometer), we see the above method as the safest first pass. Data are available in the preregistration for more comprehensive analyses (and we recommend options in the discussion). The hypotheses remained the same. In below, we present descriptive statistics of insights, confidence, and accuracy, arranged by problem type.
Table 2. Descriptive statistics for each problem type.
Statistical analyses
In our analyses, we rely primarily on two techniques. For the first set of analyses we use repeated measures ANOVAs to assess the influence of the factors problem type (analytic vs. insight vs. CRA) and method (verbal vs. dynamometer) on Aha moments as the DV. In all ANOVAs, analyses are conducted at the level of participants to ensure that we do not violate assumptions of independence, and for clarity we also note how many observations are used in each analysis. This approach to analysis is conceptually analogous to previous work on similar research questions (see for example, Danek et al., Citation2014b; Salvi et al., Citation2016; Webb et al., Citation2018). The second set of analyses involves regressions, usually with Aha moments or Aha intensity as the IV and accuracy or confidence as the DV. Due to the hierarchical nature of the data in the second set of analyses, where Aha moments and their intensity are nested within participants, we employed linear mixed models (LMM) with participants as random intercepts and Aha moments or intensity as fixed factors. Where the DV is categorical (e.g. accuracy), we used a generalised linear mixed model (GLMM). All predictors were coded using mean-centered effects coding, and we reported statistics in accordance with similar previous work (Ding et al., Citation2021). All analyses were conducted using the open source software Jamovi (https://jamovi.org), and hierarchical linear mixed models using the GAMLj module, which is based on the lmer function within the lme4 package in R (Bates et al., Citation2015). Our data are available on the OSF for further analysis https://osf.io/w7kyc/.
Do verbal reports and the dynamometer converge?
Aha moments. We began by comparing the proportion of Aha moments recorded by the two methods across the different problem conditions. illustrates the incidence of self-reported Aha moments and dynamometer spikes for analytic, insight, and CRA problems. The general pattern of Aha moments appears similar for self-report (top) and for the dynamometer spikes (bottom): Both methods indicate that analytic problems elicit the fewest Aha moments, followed by insight problems, and then CRA problems (both involving 180 observations, i.e. three per participant).
Figure 1. Insights for self-report (top level) and dynamometer (bottom level) for the different problem types. The figure illustrates that the incidence of self-reported insights and those captured by the dynamometer converge (i.e. show a similar pattern). Blue circles represent individual participants with a random horizontal jitter to aid visualisation. Error bars represent 95% confidence intervals.
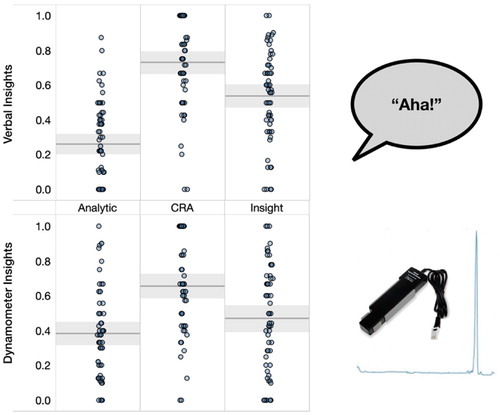
To test the above, we analysed whether the pattern of Aha moments is the same for the two methods across problem types using a repeated-measures ANOVA with problem type and method as factors and Aha moments as the DV. This analysis revealed a main effect of problem type F(2,118) = 61.89, p < .001, ηp² = .512. Follow up Tukey comparisons indicated that Analytic problems elicited fewer Aha moments than CRA problems, t(118) = 11.12, p < .001 and insight problems, t(118) = 5.43, p < .001, and CRA problems elicited more Aha moments than insight problems, t(118) = 5.7, p < .001. There was also a significant interaction between problem type and method, F(2,118) = 21.05, p < .001, ηp² = .263. Tukey comparisons revealed that, for analytic problems, self-reports showed more Aha moments than the dynamometer, t(147) = 4.17, p < .001, but there was no difference between the two methods for CRA and insight problems.
Do dynamometer warmth patterns dissociate problem types?
Previous research suggests that participants ought to be less able to predict solutions that appear in mind for insight problems and CRAs, whereas solutions to analytic problems follow from more gradual warmth patterns (Metcalfe & Wiebe, Citation1987). The size of the dynamometer slope preceding solutions (DV) was therefore evaluated for the three problem types as the IV (involving one mean slope size for each problem type and therefore 180 observations or three per participant) using a repeated measures ANOVA, F(2,118) = 19.8, p < .001, ηp² = .251 (illustrated in below). Follow up Tukey comparisons showed that analytic problems had a larger slope (i.e. greater perceived progress prior to solution) than CRA problems t(118) = 6.28, p < .001 and insight problems t(118) = 3.52, p = .002. Insight problems also showed greater perceived progress than CRA problems t(118) = 2.76, p = .018. The results align with Metcalfe and Wiebe (Citation1987) and underscore the different metacognitions associated with solving the different problems.
Figure 2. Average slope size by problem type. A greater slope in the dynamometer pattern indicates that the participant experienced more metacognitive progress towards solution. Solutions to analytic problems tended to follow from greater perceived progress (illustrative example provided on the bottom-left of the figure), compared to insight and CRA problems. Blue circles represent individual participants with a random horizontal jitter to aid visualisation. Shading represents 95% confidence intervals.
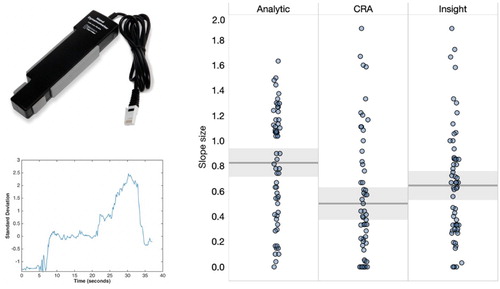
Do real-time Aha experiences predict accurate solutions?
Next we investigated the effect of Aha experiences (both verbal and visceral) on confidence and accuracy collapsing across the three different problem types (see ). In line with previous research, verbal Aha experiences predicted more accurate solutions to problems, t(58) = 12.6, p < .001, d = 1.64, and greater confidence in the solution t(58) = 14.4, p < .001, d = 1.87. Extending on previous research, the impulsive squeeze of the dynamometer also predicted more accurate responses, t(57) = 9.22, p < .001, d = 1.21, and predicted greater subsequent confidence, t(58) = 12.4, p < .001, d = 1.62. The key finding is that the feeling at the very moment of solution – as indicated by a full-strength squeeze of the dynamometer – is sufficient to predict more accurate responses.
Figure 3. The proportion correct of Aha and No Aha responses, split between verbal-report (left) and the dynamometer (right). Blue circles represent individual participants with a random horizontal jitter to aid visualisation. Shading represents 95% confidence intervals.
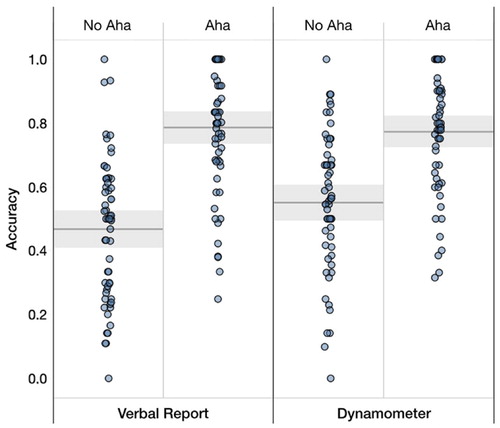
We also assessed the relationship between self-reported Aha moments and solution accuracy at each level of problem type (on a maximum of 6 observations for subjects who reported Aha and no Aha solutions for the three problem types) using a repeated measures ANOVA, F(5,115) = 19.7, p < .001, ηp² = .461. As predicted, Tukey comparisons revealed that Aha moments are only predictive of accuracy for insight problems, t(115) = 3.97, p = .002, d = 1.2, and CRAs, t(115) = 8.24, p < .001, d = 1.9, but not analytic problems (p = .99). The same test was carried out for the dynamometer spikes at problem type, which also indicated a main effect, F(5,215) = 17, p < .001, ηp² = .283. Tukey comparisons revealed the same pattern of results as self report, where dynamometer spikes predicted accurate responses only for insight, t(215) = 4.23, p < .001, d = .6 and CRA t(215) = 7.83, p < .001, d = .95 problems, but not analytic problems (p = .99).
Does Aha intensity predict accuracy and confidence?
Finally, we predicted that when an Aha moment occurs, the more intense the reported feeling is, the greater the subsequent confidence and accuracy. To assess whether Aha intensity predicted accuracy we used a GLMM, with Aha intensity as a fixed effect and participants as a random effect. The model indicated a positive effect of Aha intensity on accuracy b = 0.338, z = 6.48, odd ratios = 1.4, R2 = .324, χ2 = 42, p < .001. An LMM was used to assess the effect of Aha intensity (fixed effect) on confidence (DV), with participants as a random effect. This analysis also revealed that Aha intensity positively predicted confidence in the solution b = 0.514, R2 = .549, t = 14.7, F(1, 592.7) = 215, p < .001. These results indicate that, not only does the Aha moment predict objective performance, but the intensity of the experience carries additional predictive information beyond the mere presence or absence of the phenomenology, as illustrated in below.
Exploratory analysis: do warmth patterns predict accurate solutions?
We also conducted exploratory analyses on the relationship between average perceived progress (slope size in the dynamometer) and problem-solving accuracy. Metcalfe and Wiebe (Citation1987) found that perceived progress (feelings of warmth) was predictive of correct solutions for analytic problems, but not insight problems. Thus, we explored whether dynamometer derived perceived progress was predictive of accuracy separately for “creative” problems (collapsing over CRA and insight problems) and analytic problems using GLMM (see below). For creative problems, we found that the slope (i.e. perceived progress on the problem) was negatively predictive of correct solutions, b = −1.026, z = −6.66, odd ratios = 0.358, R2 = .276, χ2 = 44.4, p < .001. On the other hand, for analytic problems the slope was not significantly predictive of accuracy, p = .190. Therefore extending on Metcalfe and Wiebe (Citation1987), our results indicate that experiencing progress on a creative problem can indicate imminent failure in finding a solution.
Figure 5. Left: Regression plot for creative problems with slope size on the x-axis and accuracy on the y-axis. Right: Regression plot for analytic problems with slope size on the x-axis and accuracy on the y-axis. Shading represents 95% confidence intervals; grey lines represent (participant) random effects.
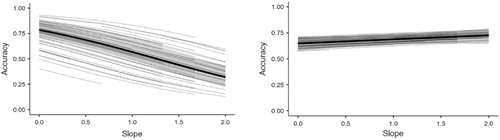
Exploratory analysis: embodying the Aha experience
As a final exploratory analysis, we investigated whether the maximum (max) dynamometer rating – the strongest squeeze at any point in the trial – could also be used to predict accuracy and the intensity of Aha moments. This hypothesis occurred to us posthoc because we did not instruct participants to squeeze the dynamometer more tightly for more intense Aha moments. Participants were simply instructed to give the device a full strength squeeze if an Aha moment occurred. Nevertheless, due to the embodied nature of the device, it may be that participants unintentionally squeezed the dynamometer more tightly when a more intense Aha moment occurred and this in turn may map onto the accuracy of solutions. An LMM revealed that the max rating positively predicted the intensity of Aha moments b = 0.189, R2 = .481, t = 12.4, F(1, 566.9) = 154, p < .001. Moreover, a GLMM revealed that max ratings also predicted the accuracy of solutions b = 0.087, z = 7.11, odd ratios = 1.09, R2 = .183, χ2 = 50.5, p < .001. These results,although exploratory,suggestt that the intensity of the insight experience was unintentionally embodied through the dynamometer and that this embodiment also predicted the accuracy of the solution (see ).
Figure 6. Left: Regression plot with maximum dynamometer ratings on the x-axis and Aha intensity on the y-axis. Right: Regression with maximum dynamometer ratings on the x-axis and accuracy on the y-axis. Shading represents 95% confidence intervals; grey lines represent (participant) random effects.
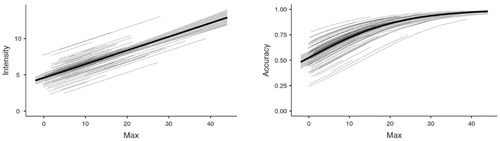
Discussion
It is an intriguing prospect that a feeling can somehow predict the discovery of a good idea in one’s own head. Although anecdotes of such sudden discoveries abound, it is only in the last decade that scientists have been able to show that humans can, and perhaps therefore should,trust their feelings of insight (Danek et al., Citation2014b, Citation2020; Danek & Wiley, Citation2017; Hedne et al., Citation2016; Salvi, Bricolo, et al., Citation2016; Threadgold et al., Citation2018; Webb et al., Citation2016, Citation2018). In this experiment, we aimed to develop a new tool for measuring insight moments that captures both the cognitive (suddenness) and phenomenological (Aha) dimensions of insight. As an application of this new tool, we hoped to complement previous research by showing that the impulsive feeling of insight embodied in real-time map onto accurate solutions.
Consistent with previous studies, we found that insight moments that were captured using a dynamometer predicted objective performance for creative problems. We also found that the intensity of the Aha experience predicted solution accuracy, and that this intensity was captured by the dynamometer. Participants naturally (i.e. without instruction) squeezed the dynamometer harder for more intense Aha experiences. In an exploratory analysis, we also found that this unintentional embodiment of Aha intensity predicted confidence and accurate problem solving. Thus, consistent with the findings of numerous previous studies (e.g. Danek & Wiley, Citation2017; Laukkonen et al., Citation2018; Salvi et al., Citation2016; Webb et al., Citation2016), these results firmly indicate that feelings of Aha – and crucially, the intensity of the feeling – can have informational value about the veracity of a problem-solving solution.
We also found that self-reported Aha moments and “spikes” in the dynamometer converged. That is, when the dynamometer showed a “spike” pattern indicative of insight, participants tended to also verbally report that they experienced an Aha moment. This finding indicates, as proposed by Danek and Salvi (Citation2020), that self-reported insights are not likely to be confounded by confidence or other metacognitive reflections and thereby strengthens previous work using self-reports. The conclusion that self-reported Aha moments are not a post-hoc interpretation is also supported by Shen et al. (Citation2018), where participants solved CRA problems while having their heart rate and skin conductance response continuously measured. They found that participants had significantly raised mean skin conductance on “Aha” trials compared to “non-Aha” trials. Thus, the dynamometer, skin conductance, and heart-rate all further strengthen the validity of the insight construct and suggest that participants can accurately introspect them.
The dynamometer also mirrored the pattern of results expected for different problem types, where solutions to analytic or multistep problems tended to be metacognitively predictable and solutions to insight problems and CRAs were subjectively more sudden and unpredictable (Metcalfe & Wiebe, Citation1987). Moreover, consistent with Webb et al. (Citation2018), we found that CRA problems lead to the most insights and showed the strongest insight-accuracy effect, followed by insight problems, with analytic problems eliciting the fewest insights and no insight-accuracy effect. With regard to analytic problems it is difficult herein to disentangle whether feelings of Aha are less “trustworthy” with such step-by-step problems, or if these problems (as was already argued by Metcalfe & Wiebe, Citation1987) simply do not lead to key components of insight, namely the cognitive process of restructuring (Ohlsson, Citation1984; Danek, Citation2018). It may be that feelings of insight (the Aha experience) are a weaker marker of accuracy in contexts that are not “creative”, i.e. do not involve implicit processing.
Relatedly, perceived problem-solving progress – or feelings of warmth – showed that greater perceived progress was negatively correlated with accurate solutions for creative problems, but trended positively for analytic problems. There is perhaps a relatively straightforward explanation for the above results. Analytic problems demand that an individual strategically and consciously solve a problem, often inducing greater working memory load than insight problems (Webb & Gilhooly, Citation2018). On the other hand, the important processes for solving insight and CRA problems often occur implicitly in the form of restructuring or complex associative processing (Bowden, Citation1997; Danek & Flanagin, Citation2019; Ohlsson, Citation1984; Pétervári & Danek, Citation2020). It therefore makes sense that perceived progress is not predictive of accuracy for creative problems because much of the important processes are hidden from awareness (though this should not be taken to downplay the important role of explicit processing during creative problem solving). On the other hand, since analytic problems are solved consciously and analytically, feelings of progress are more informative. Speculatively, since perceived progress predicts failure in finding a solution to creative problems, this “illusory” progress may be diagnostic of functional fixedness or getting “stuck” on an incorrect representation of the problem (Ohlsson, Citation1984). These results also further point to the important dissociation between feelings-of-warmth measures of suddenness and phenomenological measures of Aha as capturing distinct features of insight (Bialić et al., Citation2019; Kizilirmak et al., Citation2016, Citation2018; Laukkonen & Tangen, Citation2018; Metcalfe & Wiebe, Citation1987).
Insights are accurate, but how?
Danek and Salvi (Citation2020) recently provided a process account of why insight moments tend to be correct. They proposed that,
Correct insight solutions are qualitatively different from incorrect ones, because they require a restructuring of the initial problem representation (Ohlsson, 1992), leading to alternative interpretations of concepts that at first seem unrelated, but suddenly fit together as a whole or a good Gestalt. Correct solutions bring a sense of closure and pleasure. In contrast, for incorrect solutions, some elements may be missing, or do not fit, yielding an incomplete sense of Gestalt. (p. 485)
“Pros and cons” of the dynamometer
There are some practical and conceptual advantages to using a dynamometer to capture insight moments, particularly if one is also interested in measuring perceived progress. First, the dynamometer is continuous and substantially more sensitive than existing measures. Second, the device can be used without significantly impairing task performance. Overall performance was comparable or better for similar tasks compared to Webb et al. (Citation2016, Citation2018) and Salvi et al. (Citation2016). Our CRA problems were taken from Bowden and Jung-Beeman (Citation2003), wherein average performance for the same problems was 46.4% (30s solving time), whereas our participants solved 72% of problems (90s solving time). Thus, at the very least any distracting quality of the dynamometer can be mitigated by extending the time given to participants. Although we were not able to directly test it here, previous research also indicates that the dynamometer may help to mitigate verbal overshadowing effects and help participants communicate feelings more directly and viscerally compared to verbal methods (Brown et al., Citation2014; Creswell et al., Citation2016; Lieberman et al., Citation2007; Schooler & Engstler-Schooler, Citation1990; Schooler et al., Citation1993). Third, when a traditional warmth measure is used, substantial data (up to 70% of trials, Laukkonen & Tangen, Citation2018) are unusable because participants often solve the problem too quickly. With the dynamometer, no trials were removed. Fourth, the dynamometer can capture novel interactions between experiences of insight and cognitive components such as feelings of progress and suddenness (see ), discussed further below.
As noted above, the opportunity to capture interactions between perceived progress and Aha moments also affords a paradigm for answering novel questions. For example, it’s possible that gradual warmth patterns preceding Aha are instances of problem restructuring, or representational change (Danek & Flanagin, Citation2019; Ohlsson, Citation1984, Citation2011; Pétervári & Danek, Citation2020). That is, a participant perceives themselves as making progress on a problem using an incorrect representation – and therefore provides gradual warmth ratings – before suddenly arriving at the correct representation and discovering the solution in an Aha moment (Table 1B). Such cases may have unique cognitive or behavioural consequences relative to insight solutions that follow from sudden warmth patterns, which may be indicative of an impasse (Table 1A). Danek (Citation2018) recently highlighted two pathways of so-called non-monotonic problem solving, wherein a problem solver starts off with an incorrect representation, which can either lead to an impasse and a correct representation, or directly from an incorrect representation to a correct one. The dynamometer demonstrates the existence of these two pathways, wherein impasse-Aha (Table 1B) or restructuring-Aha (Table 1A) can lead to insight.
There are also certain disadvantages. While our impression is that the dynamometer does not substantially interfere with the primary task (Bowden & Jung-Beeman, Citation2003; Webb et al., Citation2016, Citation2018), it is inevitable that it divides attention to some degree. To ensure that it is not distracting, a control condition would be necessary. Given that participants can simply be given more time to solve the problem to mitigate the distraction issue, the real concern is whether there is a differential effect on solving the different problem types. But here too, our solution rates and Aha moments across the different problems was comparable to previous work (Bowden & Jung-Beeman, Citation2003; Salvi et al., Citation2016; Webb et al., Citation2016, Citation2018). One possibility is that squeezing the dynamometer with the dominant hand made participants better at solving the creative problems (see Goldstein et al., Citation2010). Therefore, if researchers are interested in the relative accuracy of problem solving, then it would be worthwhile counterbalancing the hand that is used to squeeze the instrument. Moreover, although the dynamometer clearly showed benefits of embodiment for the Aha moment (i.e. participants naturally squeezed the dynamometer harder for more intense insights, which mapped onto confidence and accuracy), we do not yet know whether the warmth measure also benefited from the visceral nature of the dynamometer. A useful next step would be to employ a control group wherein the dynamometer is compared to traditional warmth measures (Metcalfe & Wiebe, Citation1987). There is also room to improve future analyses. One alternative method for detecting spikes is acceleration, i.e. the rate of change over time. By using a moving average window, dynamometer ratings can be evaluated according to changes in acceleration at any point during the trial, which in turn could be used to infer an insight experience. Since acceleration is a measure of the speed of increase rather than the magnitude of the increase, the method navigates any issues regarding the strength of the participant’s grip. With regard to perceived progress, methods used to analyze task-evoked pupil dilation – another highly sensitive continuous measure – could be co-opted for the dynamometer (e.g. Beatty, Citation1982).
Conclusion
In this experiment, we investigated whether a dynamometer – a highly sensitive measure of grip strength – could complement previous measures of insight by capturing both the cognitive (suddenness) and feeling (Aha) components of insight, embodied in real-time. Our data largely converged with other similar measures (e.g. Metcalfe & Wiebe, Citation1987; Webb et al., Citation2016; Citation2018), and also demonstrated that real-time Aha moments are highly predictive of correct solutions to problems, so long as those problems involve implicit processing. Moreover, participants naturally embodied the intensity of their insight experiences through the dynamometer, which mapped onto confidence and objective performance. Out in the world, this means that our Eureka moments may mark important discoveries in complex, multivariate situations, but are perhaps less helpful when a problem demands a more linear problem-solving process. It was also compelling to find that the dynamometer captured the intensity of the Aha moment even though participants were not instructed to communicate it, and further predicted the accuracy of solutions. Overall,and consistent with previous work (Danek et al., Citation2014b, Citation2020; Danek & Wiley, Citation2017; Hedne et al., Citation2016; Salvi, Bricolo, et al., Citation2016; Threadgold et al., Citation2018; Webb et al., Citation2016, Citation2018), our results re-affirm the exciting finding that Eureka moments tend to be correct and likely play an important and adaptive role in decision-making.
Data availability statement
The data that support the findings of this study are openly available in the Open Science Framework at https://osf.io/w7kyc/.
Disclosure statement
No potential conflict of interest was reported by the author(s).
References
- Aziz-Zadeh, L., Kaplan, J. T., & Iacoboni, M. (2009). “Aha!”: The neural correlates of verbal insight solutions. Human Brain Mapping, 30(3), 908–916. https://doi.org/10.1002/hbm.20554
- Bates, D. M., Machler, M., Bolker, B. M., & Walker, S. (2015). Fitting linear mixed-effects models using lme4. Journal of Statistical Software, 67(1), 1–48. https://doi.org/10.18637/jss.v067.i01
- Beatty, J. (1982). Task-evoked pupillary responses, processing load, and the structure of processing resources. Psychological Bulletin, 91(2), 276–292. https://doi.org/10.1037/0033-2909.91.2.276
- Bialić, M., Graf, M., Vaci, N., & Danek, A. H. (2019). When the solution is on the doorstep: Better solving performance, but diminished Aha! experience for chess experts on the mutilated checkerboard problem. Cognitive Science, 43(8), e12771. https://doi.org/10.1111/cogs.12771
- Bowden, E. M. (1997). The effect of reportable and unreportable hints on anagram solution and the Aha! experience. Consciousness and Cognition, 6(4), 545–573. https://doi.org/10.1006/ccog.1997.0325
- Bowden, E. M., & Jung-Beeman, M. (2003). Normative data for 144 compound remote associate problems. Behavior Research Methods, Instruments, & Computers, 35, 634–639. https://doi.org/10.3758/BF03195543
- Bowden, E. M., & Jung-Beeman, M. (2007). Methods for investigating the neural components of insight. Methods, 42(1), 87–99. https://doi.org/10.1016/j.ymeth.2006.11.007
- Bowden, E. M., Jung-Beeman, M., Fleck, J., & Kounios, J. (2005). New approaches to demystifying insight. Trends in Cognitive Sciences, 9(7), 322-328. https://doi.org/10.1016/j.tics.2005.05.012
- Brown, C., Brandimonte, M. A., Wickham, L. H., Bosco, A., & Schooler, J. W. (2014). When do words hurt? A multiprocess view of the effects of verbalization on visual memory. Journal of Experimental Psychology: Learning, Memory, and Cognition, 40(5), 1244–1256. https://doi.org/10.1037/a0037222
- Brown, K. W., & Ryan, R. M. (2003). The benefits of being present: Mindfulness and its role in psychological well-being. Journal of Personality and Social Psychology, 84(4), 822–848. https://doi.org/10.1037/0022-3514.84.4.822
- Chein, J., Weisberg, R., Streeter, N., & Kwok, S. (2010). Working memory and insight in the nine-dot problem. Memory & Cognition, 38(7), 883–892. https://doi.org/10.3758/MC.38.7.883
- Chu, Y., & MacGregor, J. N. (2011). Human performance on insight problem solving: A review. The Journal of Problem Solving, 3(2), 119–150. https://doi.org/10.7771/1932-6246.1094
- Creswell, K. G., Sayette, M. A., Schooler, J. W., Wright, A. G. G., & Pacilio, L. E. (2016). Visceral states call for visceral measures: Verbal overshadowing of hunger ratings across assessment modalities. Assessment, 25(2), 173–182. https://doi.org/10.1177%2F1073191116645910 doi:10.1177/1073191116645910
- Creswell, K. G., Sayette, M. A., Skrzynski, C. J., Wright, A. G., Schooler, J. W., & Sehic, E. (2019). Assessing cigarette craving with a squeeze. Clinical Psychological Science, 7(3), 597–611. https://doi.org/10.1177/2167702618815464
- Cushen, P. J., & Wiley, J. (2012). Cues to solution, restructuring patterns, and reports of insight in creative problem solving. Consciousness and Cognition, 21(3), 1166–1175. https://doi.org/10.1016/j.concog.2012.03.013
- Damasio, A. R. (1996). The somatic marker hypothesis and the possible functions of the prefrontal cortex. Philosophical Transactions of the Royal Society of London. Series B: Biological Sciences, 351(1346), 1413–1420. https://doi.org/10.1098/rstb.1996.0125
- Danek, A. H. (2018). Magic tricks, sudden restructuring, and the Aha! experience: A new model of nonmonotonic problem solving. In F. Vallee-Tourangeau (Ed.), Insight: On the origins of new ideas (1st ed., pp. 51–78). Routledge. https://doi.org/10.4324/9781315268118
- Danek, A. H., & Flanagin, V. L. (2019). Cognitive conflict and restructuring: The neural basis of two core components of insight. AIMS Neuroscience, 6(2), 60–84. https://doi.org/10.3934/Neuroscience.2019.2.60
- Danek, A. H., Fraps, T., von Müller, A., Grothe, B., & Öllinger, M. (2014a). It’s a kind of magic—what self-reports can reveal about the phenomenology of insight problem solving. Frontiers in Psychology, 5, 1408–1408. https://doi.org/10.3389/fpsyg.2014.01408
- Danek, A. H., Fraps, T., von Müller, A., Grothe, B., & Öllinger, M. (2014b). Working wonders? Investigating insight with magic tricks. Cognition, 130(2), 174–185. https://doi.org/10.1016/j.cognition.2013.11.003
- Danek, A. H., & Salvi, C. (2020). Moment of truth: Why Aha experiences are correct. Journal of Creative Behavior, 54(2), 484–486. https://doi.org/10.1002/jocb.380
- Danek, A. H., & Wiley, J. (2017). What about false insights? Deconstructing the Aha! experience along its multiple dimensions for correct and incorrect solutions separately. Frontiers in Psychology, 7, 2077. https://doi.org/10.3389/fpsyg.2016.02077
- Danek, A. H., Williams, J., & Wiley, J. (2020). Closing the gap: Connecting sudden representational change to the subjective Aha! experience in insightful problem solving. Psychological Research, 84(1), 111–119. https://doi.org/10.1007/s00426-018-0977-8
- Ding, K., Chen, Q., Yang, W., Wang, X., Yang, D., Ding, C., & Qiu, J. (2021). Recognizing ideas generated in a creative thinking task: Effect of the subjective novelty. Current Psychology, 1–13. https://doi.org/10.1007/s12144-020-01342-7
- Ericsson, K. A., & Charness, N. (1994). Expert performance: Its structure and acquisition. American Psychologist, 49(8), 725–747. https://doi.org/10.1037/0003-066X.49.8.725
- Goldstein, A., Revivo, K., Kreitler, M., & Metuki, N. (2010). Unilateral muscle contractions enhance creative thinking. Psychonomic Bulletin & Review, 17(6), 895–899. https://doi.org/10.3758/PBR.17.6.895
- Hedne, M. R., Norman, E., & Metcalfe, J. (2016). Intuitive feelings of warmth and confidence in insight and noninsight problem solving of magic tricks. Frontiers in Psychology, 7, 1314. https://doi.org/10.3389/fpsyg.2016.01314
- Jung-Beeman, M., Bowden, E. M., Haberman, J., Frymiare, J. L., Arambel-Liu, S., Greenblatt, R., Reber, P. J., & Kounios, J. (2004). Neural activity when people solve verbal problems with insight. PLoS Biology, 2(4), e97. https://doi.org/10.1371/journal.pbio.0020097
- Kizilirmak, J., Galvao Gomes da Silva, J., Imamoglu, F., & Richardson-Klavehn, A. (2016). Generation and the subjective feeling of “Aha!” are independently related to learning from insight. An International Journal of Perception, Attention, Memory, and Action, 80(6), 1059–1074. https://doi.org/10.1007/s00426-015-0697-2
- Kizilirmak, J. M., Serger, V., Kehl, J., Öllinger, M., Folta-Schoofs, K., & Richardson-Klavehn, A. (2018). Feelings-of-warmth increase more abruptly for verbal riddles solved with in contrast to without Aha! experience. Frontiers in Psychology, 9, 1404. https://doi.org/10.3389/fpsyg.2018.01404
- Köhler, W. (1921). Intelligenzprüfungen am menschenaffen. Springer. https://doi.org/10.1007/978-3-642-47574-0
- Kounios, J., & Beeman, M. (2014). The cognitive neuroscience of insight. Annual Review of Psychology, 65(1), 71–93. https://doi.org/10.1146/annurev-psych-010213-115154
- Kounios, J., Fleck, J. I., Green, D. L., Payne, L., Stevenson, J. L., Bowden, E. M., & Jung-Beeman, M. (2008). The origins of insight in resting-state brain activity. Neuropsychologia, 46(1), 281–291. https://doi.org/10.1016/j.neuropsychologia.2007.07.013
- Kounios, J., Frymiare, J. L., Bowden, E. M., Fleck, J. I., Subramaniam, K., Parrish, T. B., & Jung-Beeman, M. (2006). The prepared mind: Neural activity prior to problem presentation predicts subsequent solution by sudden insight. Psychological Science, 17(10), 882–890. https://doi.org/10.1111/j.1467-9280.2006.01798.x
- Laukkonen, R., Kaveladze, B., Tangen, J. M., & Schooler, J. (2020). The dark side of Eureka: Artificially induced Aha moments make facts feel true. Cognition, 196, 104122–104122. https://doi.org/10.1016/j.cognition.2019.104122
- Laukkonen, R., & Tangen, J. (2017). Can observing a Necker cube make you more insightful? Consciousness and Cognition, 48, 198–211. https://doi.org/10.1016/j.concog.2016.11.011
- Laukkonen, R. E., & Tangen, J. M. (2018). How to detect insight moments in problem solving experiments. Frontiers in Psychology, 9, 282. https://doi.org/10.3389/fpsyg.2018.00282
- Laukkonen, R. E., Webb, M. E., Salvi, C., Tangen, J. M., & Schooler, J. (2018). Eureka heuristics: How feelings of insight signal the quality of a new idea. PsyArXiv. doi:10.31234/osf.io/ez3tn.
- Lieberman, M. D., Eisenberger, N. I., Crockett, M. J., Tom, S. M., Pfeifer, J. H., & Way, B. M. (2007). Putting feelings into words: Affect labeling disrupts amygdala activity to affective stimuli. Psychological Science, 18(5), 421–428. https://doi.org/10.1111/j.1467-9280.2007.01916.x
- Luo, J., Niki, K., & Phillips, S. (2004). Neural correlates of the ‘Aha! reaction’. Neuroreport, 15(13), 2013–2017. https://doi.org/10.1097/00001756-200409150-00004
- Metcalfe, J. (1986). Premonitions of insight predict impending error. Journal of Experimental Psychology: Learning, Memory, and Cognition, 12(4), 623–634. https://doi.org/10.1037/0278-7393.12.4.623
- Metcalfe, J., & Wiebe, D. (1987). Intuition in insight and noninsight problem solving. Memory & Cognition, 15(3), 238–246. https://doi.org/10.3758/BF03197722
- Ohlsson, S. (1984). Restructuring revisited. Scandinavian Journal of Psychology, 25(1), 65–78. https://doi.org/10.1111/j.1467-9450.1984.tb01001.x
- Ohlsson, S. (1992). Information-processing explanations of insight and related phenomena. Advances in the Psychology of Thinking, 1, 1-44.
- Ohlsson, S. (2011). Deep learning: How the mind overrides experience. Cambridge University Press. https://doi.org/10.1017/CBO9780511780295
- Öllinger, M., & Knoblich, G. (2009). Psychological research on insight problem solving. In H. Atmanscpacher and H. Primas (Eds.), Recasting reality: Wolgang Pauli’s philosophical ideas and contemporary science (pp. 275–300). Springer. https://doi.org/10.1007/978-3-540-85198-1
- Pétervári, J., & Danek, A. H. (2020). Problem solving of magic tricks: Guiding to and through an impasse with solution cues. Thinking & Reasoning, 26(4), 502–533. https://doi.org/10.1080/13546783.2019.1668479
- Salvi, C., Beeman, M., Bikson, M., McKinley, R., & Grafman, J. (2020a). TDCS to the right anterior temporal lobe facilitates insight problem-solving. Scientific Reports, 10(1), 946. https://doi.org/10.1038/s41598-020-57724-1
- Salvi, C., Simoncini, C., Grafman, J., & Beeman, M. (2020b). Oculometric signature of switch into awareness? Pupil size predicts sudden insight whereas microsaccades problem-solving via analysis. NeuroImage, 217, 116933. https://doi.org/10.1016/j.neuroimage.2020.116933
- Salvi, C., & Bowden, E. M. (2016). Looking for creativity: Where do we look when we look for new ideas? Frontiers in Psychology, 7, 161. https://doi.org/10.3389/fpsyg.2016.00161
- Salvi, C., Bricolo, E., Franconeri, S. L., Kounios, J., & Beeman, M. (2015). Sudden insight is associated with shutting out visual inputs. Psychonomic Bulletin & Review, 22(6), 1814–1819. https://doi.org/10.3758/s13423-015-0845-0
- Salvi, C., Bricolo, E., Kounios, J., Bowden, E., & Beeman, M. (2016). Insight solutions are correct more often than analytic solutions. Thinking & Reasoning, 22(4), 443–460. https://doi.org/10.1080/13546783.2016.1141798
- Schooler, J. W., & Engstler-Schooler, T. Y. (1990). Verbal over-shadowing of visual memories: Some things are better left unsaid. Cognitive Psychology, 22(1), 36–71. https://doi.org/10.1016/0010-0285(90)90003-M
- Schooler, J. W., & Melcher, J. (2009). The ineffability of insight. In S. Smith, T. Ward, & R. Finke (Eds.), The creative cognition approach (pp. 97–133). MIT Press. https://doi.org/10.7551/mitpress/2205.001.0001
- Schooler, J. W., Ohlsson, S., & Brooks, K. (1993). Thoughts beyond words: When language overshadows insight. Journal of Experimental Psychology: General, 122(2), 166–183. https://doi.org/10.1037/0096-3445.122.2.166
- Schwarz, N. (2012). Feelings-as-information theory. In P. A. M. Van Lange, A. W. Kruglanski, & E. T. Higgins (Eds.), Handbook of theories of social psychology (pp. 260–308). https://doi.org/10.4135/9781446249215
- Shen, W., Tong, Y., Yuan, Y., Zhan, H., Liu, C., Luo, J., & Cai, H. (2018). Feeling the insight: Uncovering somatic markers of the “aha” experience. Applied Psychophysiology and Biofeedback, 43(1), 13–21. https://doi.org/10.1007/s10484-017-9381-1
- Slovic, P., Finucane, M. L., Peters, E., & Macgregor, D. G. (2007). The affect heuristic. European Journal of Operational Research, 177(3), 1333–1352. https://doi.org/10.1016/j.ejor.2005.04.006
- Sternberg, R. J., & Davidson, J. E. (1994). The nature of insight. The MIT Press. https://doi.org/10.7551/mitpress/4879.001.0001
- Threadgold, E., Marsh, J. E., & Ball, L. J. (2018). Normative data for 84 UK English rebus puzzles. Frontiers in Psychology, 9, 2513. https://doi.org/10.3389/fpsyg.2018.02513
- Webb, M. E., & Gilhooly, K. (2018). Working memory in insight problem solving. In F. Vallee-Tourangeau (Ed.), Insight: On the origin of new ideas (1st ed., pp. 105–119). Routledge. https://doi.org/10.4324/9781315268118
- Webb, M. E., Laukkonen, R. E., Cropper, S. J., & Little, D. R. (2019). Commentary: Moment of (perceived) truth: Exploring accuracy of aha! experiences. The Journal of Creative Behavior, 1–5. https://doi.org/10.1002/jocb.433
- Webb, M. E., Little, D. R., & Cropper, S. J. (2016). Insight is not in the problem: Investigating insight in problem solving across task types. Frontiers in Psychology, 7(1424), 1424. https://doi.org/10.3389/fpsyg.2016.01424
- Webb, M. E., Little, D. R., & Cropper, S. J. (2018). Once more with feeling: Normative data for the aha experience in insight and noninsight problems. Behavior Research Methods, 50(5), 2035–2056. https://doi.org/10.3758/s13428-017-0972-9
- Weisberg, R. W. (1992). Metacognition and insight during problem solving: Comment on Metcalfe. Journal of Experimental Psychology: Learning, Memory and Cognition, 18(2), 426–431. https://doi.org/10.1037/0278-7393.18.2.426
Appendix A
Written instructions
You will be asked to solve a number of problems.
Some will look like this …
“A prisoner was attempting to escape from a tower. He found a rope in his cell that was half the length required for him to reach the ground safely. He divided the rope in half, tied the two parts together, and escaped. How could he have done this?”
Other problems may look like this …
“Aid/Rubber/Wagon”
Here you are asked to identify the target word that links these three words together. In this case the target word would be Band which forms Band-Aid, Rubber Band, and Bandwagon.
While solving each problem, you will use the hand grip device to give a continuous indication of how close you feel you are to arriving at a solution. You will start by resting the device in your dominant hand. As you feel like you are progressing and getting closer to a solution, increase your grip proportionally. If you feel like you are getting further away from a solution, lessen your grip. At any given moment, the grip intensity should be representative of how close you feel to the solution.
In solving some of these problems, you may experience an Aha! moment in doing so. An Aha! moment is when the solution comes to you as if out of nowhere – akin to a lightbulb moment, mini epiphany, or mini eureka moment. For example, “Oh! Of course! It’s … ”
If you feel as though you experienced an Aha! moment in solving a problem, give the device a full-strength squeeze.
If you don’t experience an Aha! moment, simply relax your grip once you have arrived at a solution.
You’ll be given 90 s to solve each problem. After you have verbalised your solution to me, you will be asked questions regarding your confidence in your answer as well as any potential Aha! moment you experienced.
Each problem has one correct answer. There are no hints so carefully think about your answer as the first response you give will be the one recorded. Some problems are more difficult than others, and you’re not expected to perform perfectly, so don’t be disheartened if you don’t solve a problem. Just do the best that you can.
If you have any questions about any of the above, please ask them now.
Appendix B
Table of problems
Insight and analytic problems, unless otherwise cited, were taken from Laukkonen and Tangen (Citation2017). Compound Remote Associates were taken from Bowden (2003).