ABSTRACT
Cognitive control is a set of mechanisms that help us process conflicting stimuli and maintain goal-relevant behaviour. According to the Affective Signalling Hypothesis, conflicting stimuli are aversive and thus elicit (negative) affect, moreover – to avoid aversive signals – affective and cognitive systems work together by increasing control and thus, drive conflict adaptation. Several studies have found that affective stimuli can indeed modulate conflict adaptation, however, there is currently no evidence that phasic affective states not triggered by conflict also trigger improved cognitive control. To investigate this possibility, we intermixed trials of a conflict task and trials involving the passive viewing of emotional words. We tested whether affective states induced by affective words in a given trial trigger improved cognitive control in a subsequent conflict trial. Applying Bayesian analysis, the results of four experiments supported the lack of adaptation to aversive signals, both in terms of valence and arousal. These results suggest that phasic affective states by themselves are not sufficient to elicit an increase in control.
To succeed in everyday life, we often need to control our habitual reactions to environmental stimuli. This cognitive control (J. D. Cohen, Citation2017) is typically studied in experimental designs, where the habitual and instructed responses are in conflict in some of the trials. In the Stroop task (Stroop, Citation1935), for example, colour words are presented in either matching or non-matching colours (congruent and incongruent trials, respectively) to the participants, whose task is to correctly identify these colours as quickly as possible. People’s tendency to respond less quickly and less accurately to incongruent compared to congruent stimuli is commonly referred to as the congruency effect. While conflict reduces accuracy and speed, cognitive control is thought to be able to adapt to conflict on the next trial. This can be investigated using sequential analysis that have revealed that people tend to respond faster and more accurately on incongruent trials that were preceded by another incongruent trial compared to when preceded by a congruent trial. The performance on current congruent trials however are impaired when preceded by incongruent trials. Because it reflects flexible adaptation, the explanation of this so-called congruency sequence effect (CSE, also known as the Gratton effect, Gratton et al., Citation1992) became an important focus of research in cognitive psychology and neuroscience (see e.g. Duthoo et al., Citation2014; Egner, Citation2007; Erb & Aschenbrenner, Citation2019).
The Conflict Monitoring Theory (Botvinick et al., Citation2001), arguably the most classic theory on conflict adaptation, identifies two components in conflict resolution: (1) a conflict monitoring component which assesses the level of conflict, and (2) a control adaptation component that increases the level of cognitive control in the next trial after conflict has been detected by this conflict monitor. According to this theory, when conflict occurs between stimulus and response, a conflict monitoring unit in the anterior cingulate cortex (ACC) detects it, and then signals to the control unit to upregulate control by selectively focusing on task-relevant information. As such, this model defines control adaptation as a function of the level of perceived conflict. There are, however, several competing hypotheses that aim to identify the driving force of control adaptation. In this article, we present a sequence of experiments to explore the empirical support of the Affective Signalling Hypothesis of control adaptation.
Conflicts as affective signals
Botvinick (Citation2007) argued that conflict works as a teaching signal that motivates avoidance. Van Steenbergen et al. (Citation2009) suggested that sequential control adaptation (i.e. the CSE) might be triggered by stimulus aversiveness, rather than response conflict. They also argue that conflict stimuli in stimulus-response tasks, such as incongruent trials on the flanker task (Eriksen & Eriksen, Citation1974), are aversive by nature and they elicit negative affect which motivates individuals to downregulate conflict or prevent it from occurring again. Sequential control modulation is thus motivated by the aversiveness of conflict stimuli. Several behavioural studies show the aversive nature of conflict trials: Morsella et al. (Citation2009) have found that incongruent trials are more aversive than congruent trials, while in a different study (Dreisbach & Fischer, Citation2012) conflicting Stroop trials facilitated negative stimulus evaluation. A facial EMG experiment (Berger et al., Citation2020) showed that incongruent trials in conflict tasks were followed by increased activation of the corrugator supercilii, a muscle involved in the expression of negative emotions (Dimberg, Citation1990; Larsen et al., Citation2003). A recent neuroimaging study (Vermeylen et al., Citation2020) has also shown that different conflict and affective tasks share neural pattern responses in the medial frontal cortex.
Affective modulation of control adaptation
Dreisbach and Fischer (Citation2015) proposed that while the conflict-control loop is adaptive, it is not clear why the occurrence of conflict should lead to upregulated control by itself, while the conflict level of the next stimulus is unknown. The authors suggested that adaptation is motivated by the aversive nature of conflict. By introducing affective signals into sequential conflict designs, this link between affect regulation and conflict adaptation can be investigated further: a wide body of evidence indeed showed that tonic (long-term, such as mood) affect has a clear effect on control adaptation (e.g.: van Steenbergen et al., Citation2010; Wang et al., Citation2014; Yang & Pourtois, Citation2018). Most of the studies showed that negative valence or high arousal tonic manipulations increased control adaptations. Previous findings also showed that phasic (short-termed) manipulations of affect as well can lead to modulated control processes. van Steenbergen et al. (Citation2009) conducted a study where unexpected monetary gain, loss or neutral signals were inserted in flanker task sequences. The authors found the conflict adaptation effect only in the loss and neutral conditions, but not in the gain condition. They explained this finding as a result of the cancellation of the aversive conflict signal following incongruent flanker trials by the positive affect elicited by the gain signal. In a study of Kanske and Kotz (Citation2011) emotional words were used as the part of colour flanker stimuli which led to decreased congruency effect on emotional stimuli compared to neutral stimuli. In a similar design with affective word flanker stimuli, increased conflict adaptation was also found, but it was related to the arousal of the words, regardless of the valence (Zeng et al., Citation2017). These findings support the notion that affective signals have a modulating effect on the adaptation to conflict. Based on recent findings and theoretical works (Dignath et al., Citation2020; Dreisbach & Fischer, Citation2015; van Steenbergen, Citation2015) a framework was proposed called Affective Signalling Hypothesis, a system that describes the control adaptation mechanism as a process from conflict to control with affective regulation as a link between them. According to this theory conflict between responses elicits a short negative affective reaction which then motivates the regulation of control.
Adaptation without conflict?
Dreisbach and Fischer (Citation2011) have found that evoking negative affect with low perceptual fluency stimuli lead to elevated mobilisation of cognitive effort. In this study they have found sequential modulation of the fluency effect which was similar to the CSE. This result shows that sequential modulation of cognitive effort can indeed be triggered not only by response conflicts, but by disfluent stimuli as well. The authors proposed that this similarity between CSE and the sequential fluency effect is due to the aversive nature of both response conflict and disfluency.
Although the above findings and reviews support the notion that affective signals have a modulating effect on control adaptation, they do not provide a conclusive answer to the question whether aversive signals are sufficient to elicit control adaptation by themselves, even in the absence of conflict.
In this work, we hypothesise that the causal relation between conflict and adaptation of control is not dependent on conflict, but solely on affect. Notably, this is the strongest possible formulation of the Affective Signalling Hypothesis, and thus, needs to be confirmed or disconfirmed first. To investigate this notion, affective signals need to be separated from traditional control trials so that we can observe the effect of these signals in isolation. To see if signal aversiveness can trigger adaptation effects, we have to separate it from all kinds of cognitive effort, be it triggered by response conflict, or disfluency. In a study by Dignath et al. (Citation2017), a design was used that was in line with the above-mentioned intentions: affective pictures of different valence and arousal dimensions were intermixed with a traditional Simon task. They found that valence and arousal have no influence on control adaptation. Several studies (Aczel et al., Citation2021; Funes et al., Citation2010; Lee & Cho, Citation2013), however, showed that switching between cognitive domains might inhibit the CSE. It is possible that switching between the processing of complex images and responding to the Simon trials might constitute a drastic change in cognitive domains that could weaken cross-trial effects. In contrast to the above results, Fröber et al. (Citation2017) have found a typical suppressed lateralised readiness potential (LRP) after trials rated as unpleasant which is in line with conflict adaptation effects, however, this suppression was relaxed after pleasant trials, regardless of the previous conflict. This suggested that negative affect even in the absence of conflict, triggers control adaptation.
If we aim to separate affective and control-related stimuli, we have to tackle several of the above-mentioned issues. We have to make sure these affective signals are irrelevant to the primary task goal, so they do not have any direct motivational effects. This way we can rule out other motivational explanations of enhanced performance. We also have to make sure that the domain switching effect between affective and cognitive trials are as small as possible. In line with the current approaches of CSE studies, we have to rule out contingency learning and feature integration effects that might affect performance across trials and dwarf the effect of the affective signals. In this study we created a design that addresses these prerequisites: We chose words as affective signals on the basis of the notion that affective words (Larsen et al., Citation2003) elicit similar objective emotional responses as conflict signals (Berger et al., Citation2020). These words were goal-irrelevant, and their affective dimensions were measured prior to using them as stimuli in the design. We chose words as affective signals on the basis of the assumption that the switch between verbal affective and verbal cognitive trials is the smallest possible domain-shift we can implement in a design like this. Affective words were used in several studies investigating their influence on cognitive control (e.g.: Kanske & Kotz, Citation2011; Li et al., Citation2014), however in these experiments affective words and flanker stimulus information overlapped. According to the conflict monitoring model (Botvinick et al., Citation2001), adaptation of control occurs when conflict is detected on a given trial and control is increased in a way that it affects performance on the next trial. Here, we propose that if we assume the affective component of said conflict trial to be responsible for the increase in control (like a strong interpretation of the affective signalling hypothesis would suggest), the most direct way to test it is to separate the affective content (the “inducer” of adaptive control [see in Braem et al., Citation2014]) from conflict stimuli. In this study, we separated the two stimuli (affective vs. cognitive) into different trials. To make sure we are controlling for feature integration and contingency learning confounds, we used a modified version of Weissman et al. (Citation2014) design. To address the question whether it is the valence or the arousal of affective signals that is relevant for control (see e.g. Zeng et al., Citation2017), we manipulated the valence and the arousal of the affective words used. In summary, with this study we aimed to investigate if aversive signals by themselves are able to drive control adaptation.
Experiments
In this online study, we aimed to create a design that allows us to elicit phasic affect that can be intermixed with classic stimulus-response incompatibility tasks such as the flanker task and the prime-probe task. As a first step, we created a Hungarian wordset of affective words that can be used as affective stimuli in such an intermixed design. Then, we measured the usefulness of the available stimulus-response incompatibility tests by running two control experiments, one for the unmodified prime-probe task, and one for the flanker task. Followed by this, we then created four different experiments, where we used the previously created valence and arousal wordlists in combination with the prime-probe and the flanker tasks respectively. We created designs where affective stimuli and stimulus-response incompatibility trials were separated in time to investigate whether the affective words have any effect on the congruency effect, and thus be responsible for sequential control adaptation by themselves, without any conflict content and regardless of the effect of previous congruency. Instead of using only stimulus-response incompatibility trials, we added affective words and blank screens into the trial sequence. By this, stimulus-response incompatibility trials were further separated from each other and were always preceded by an affective word. A blank-screen/affective word/stimulus-response incompatibility trial sequence was repeated throughout the whole experiment.
General method
Before data analysis, we preregistered the study in three preregistration documents on the Open Science Framework website (Affective wordset analysis: https://osf.io/rpcw7/; Prime-probe experiments: https://osf.io/jzp9w/; Flanker experiments: https://osf.io/z7w5c/). All deviations from the preregistrations are indicated in the section where the deviation occurs.
During the experiments, we used the participant pool made available by a university course called “Participation in Psychological Experiments”. In this course undergraduate students received course credit for participating in various experiments. All participants in the below detailed experiments were recruited through this course and were native Hungarian undergraduate students.
We followed the sequential stopping rule in defining the sample size of our data analyses that allows additional data collection if the Bayesian analysis is inconclusive (see e.g. Schönbrodt et al., Citation2017). For every data collection, we recruited the greatest sample size we could reach through the participation course. If the results were not conclusive, we recruited an additional number of participants from the course pool at the next available time.
In every data analysis wave, we calculated the Bayes factor (B) that is a measure of relative evidence provided by the data for the alternative hypothesis over the null hypothesis. This way we can distinguish between insensitive evidence and evidence for the null. We used the B values of 3 and ⅓ as cut-offs to distinguish between evidence for H1 and H0, respectively. In all of the analyses, we used the R script of Dienes and Mclatchie (Citation2018) to calculate the Bayes factor of the effects estimated by the regression models (to be described below). As priors for our Bayes factor calculations, we used the original results of Weissman et al. (Citation2014) on the same tasks. In analyses for the interaction between affective words and conflict trials in the critical experiments we kept these priors as we expected a similar adaptation effect as in a regular conflict-conflict interaction. We modelled the predictions of all alternative hypotheses with a half-normal distribution with a mode of zero as smaller effects are more probable than large ones. We specified the SD of the H1 models in the Result sections of the experiments. We report Bayes factors as BH(M, SD) where B is the Bayes factor in favour of the alternative hypothesis (B10) for a half-normal prior distribution H, with M mean and SD standard deviation.
We also reported the Bayesian Robustness Regions (RR) notated as RR[SDsmallest, SDlargest] that covers all priors that result in the same conclusion (B > 3, or B<⅓) as the original prior SD. For instance, if the Bayes Factor is higher than 3 with our original prior SD, we also report the highest and the lowest SD that results in a B of 3 or higher.
In all of our hypothesis testing analyses we implemented mixed effect linear regression models to predict trial reaction time. In all our models, we specified random intercepts for participants, and random slopes for current trial congruency, on the theoretical basis that both general reaction time, and the magnitude of the congruency effect is likely to differ across individuals. More complex random effects structures would have likely led to convergence problems for our models.
In control experiments, we tested the flanker and prime-probe tasks without any affective signals, and we hypothesised that the CSE will occur in both two tasks. To test it, we used a model where reaction time was predicted by the previous trial congruency, current congruency, and their interaction (rt ∼ previous congruency * current congruency).
In the critical experiments we implemented affective words and blank screens to the trial sequences which led to flanker (or prime – probe) trials to be separated from each other by a blank screen and an affective word trial. We hypothesised a decreased congruency effect on flanker (or prime-probe) trials, after negative valence (or high arousal) words compared to after neutral (or low arousal) words. To test this, we used a model where flanker (or prime – probe) reaction time was predicted by the interaction between the flanker (or prime – probe) congruency and affective word valence (or arousal) (rt ∼ word valence * current congruency), and their corresponding main effects.
Creating the affective word set
Since no set of Hungarian affective words were available, candidate words were selected from an English language collection then, to adjust our collection to our local Hungarian population, their affective dimensions were measured in our participant pool.
After all exclusions we obtained two wordlists in their categories:
Valence wordlist.
negative valence – 66 words (Valence M = 2.14, SD = 0.38; Arousal M = 4.86, SD = 0.57)
neutral valence – 51 words (Valence M = 4.97, SD = 0.54; Arousal M = 3.90, SD = 0.82)
positive valence – 55 words (Valence M = 7.47, SD = 0.30; Arousal M = 3.98, SD = 0.80)
Arousal wordlist.
low arousal – 105 words (Valence M = 5.18, SD = 0.91; Arousal M = 2.93, SD = 0.53)
high arousal – 85 words (Valence M = 3.94, SD = 2.11; Arousal M = 5.91, SD = 0.51)
In the test experiments (see below) we used negative and neutral sets from the valence wordlist and low and high sets from the arousal wordlist. The positive valence wordset was not used in the experiments.
For details about the creation of the Hungarian word set, see the supplementary materials.
Control experiments
For our flanker and prime-probe tasks, we used the unmodified design of Weissman et al. (Citation2014) who used a confound-free online experiment.
Participants
Both prime-probe and flanker data was collected in two separate waves from the local university participant pool. Every participant was a native Hungarian speaker. A total of 538 participants took part in the prime-probe and 635 in the flanker experiment. After all exclusions (for details see the results section), data from 502 (median age = 21 years; 80.5% female) participants was analysed for the prime-probe experiment and from 616 (median age = 21; 80.9% female) in the flanker experiment.
Apparatus and stimuli
To collect data, we used a self-hosted online tool using the code based on Gyurkovics et al. (Citation2020) which can be found on our OSF page for the study.
In the prime-probe test (), two stimuli (a prime and a probe stimulus) follow each other in a short period of time on each trial. First, a prime stimulus is shown with 3 identical direction words (e.g. “LEFT”) stacked on top of each other (presentation duration 133 ms). Then after a short blank screen (33 ms) the probe, or target, stimulus is shown with only one direction word (presentation duration 133 ms). The prime and the probe stimuli together constitute a prime-probe trial which can be congruent (the prime and the probe stimulus directions are the same) or incongruent (prime and probe stimulus directions are different). Then a fixation cross is shown for another 1,701 ms. The participants are instructed to press one of the 4 response buttons (“f” – left; “g” – right; “n” – down; “j” – up) corresponding to the current probe stimulus. Participants have 1,834 ms in total to respond to the current trial (probe duration + fixation cross duration) before the next trial starts.
Figure 1. The figure shows the experimental sequence in the prime-probe task. Each panel shows a trial and each smaller panel shows a stimulus screen, where the three word screen is a prime stimulus and the one word screen is the probe stimulus.
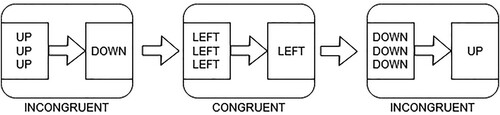
In the flanker task (), an array of 7 letters (1 target letter and six flanker letters, 3 on each side) is shown to the participants for a short period of time (250 ms) on each trial, then a fixation cross is shown until the participant presses any key. The participants are instructed to respond to the stimulus with a given keypress that corresponds to the middle letter of the stimulus array. The target or flanker stimulus letters could be “M”, “T”, “H”, or “S”. Flanking letters may be the same (congruent trial), or different (incongruent trial) as the target letter. In the flanker task, keyboard mapping of the task was randomised for every participant. The potential keys for the randomised mapping were “x”, “c”, “n”, and “m”. Each of these keys was assigned to one of the stimulus letters described above.
Procedure
In the prime-probe task vertical (up – down) and the horizontal (left – right) dimensions alternated on every trial, as such, every vertical trial was followed by a horizontal trial and vice versa. Prime stimuli and probe stimuli could only contain directions from the given trial dimension; there were no incongruent trials that had, for instance, left and down in them. Similarly in the flanker trials “M” and “T” stimuli belonged to one dimension, “H” and “S” to another and these dimensions alternated throughout the whole experiment.
After the instructions, participants performed a practice block with 24 trials. In the practice block after every response a “correct”, an “incorrect”, or a “too late” feedback was shown to inform participants about their performance.
After the practice block participants performed 4 separate test blocks with 96 trials in each in the prime-probe experiment. In the flanker experiment the 4 test blocks contained 80 trials each. No performance feedback was shown in the test blocks. Between every block there was an information screen which allowed the participants to take a break.
Prime probe task
Data preprocessing
36 participants were excluded due to their accuracy being below 70% or a mean RT more than 2.5SDs from the group mean. After participant level exclusion, 4% of trials were dropped for being incorrect, 4% o for being post error trials, 2% for being outliers (2.5SDs from the conditional mean of the participant) and 2% for being subsequent to outliers. We then analysed the remaining trials of 502 participants.
Results
As described in General methods, a linear mixed-effect model was run to determine the effect of current trial congruency, previous trial congruency, and their interaction on performance. We found evidence that RT was influenced by the congruency of the current trial. The estimated RT difference between congruent and incongruent trials was 61.94 ms (SE = 1.59), t(685.8) = −38.48, BH(0,73) = 1.11 * 10170, RRB > 3 = [0.07, 24306].
We also found evidence that RT was influenced by the interaction between previous and current trial congruency (i.e. the CSE was present). Estimated effect was 23.33 ms (SE = 1.21), t(1.695 *105) = −19.29, BH(0,36.5) = 2.72*1079, RRB > 3 = [0.07, 9248] .
Figure 3. The figure shows the mean reaction time broken down by the congruency of the current and the previous trials for the prime – probe control experiment. The Y-axis shows the mean RTs in ms. The X axis shows the congruency of the previous trial. The legend shows the congruency of the current trial. Error bars represent the standard error.
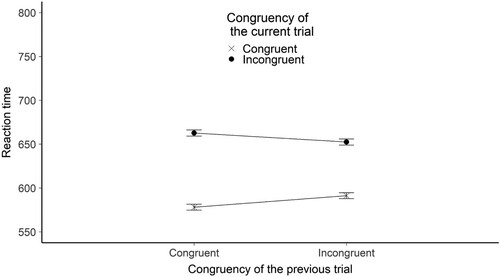
Flanker task
Data preprocessing
While the data collection programme in the flanker task allowed the participants longer response times, we excluded from the analysis every trial that exceeded the 2000 ms threshold to make sure that extreme outliers do not influence the group mean. This threshold exclusion was not preregistered. 26 participants were excluded due to accuracy below 70% or a mean RT more than 2.5SDs from the group mean. 3.5% of trials were removed for their reaction time being longer than 2000ms. 4.1% of trials were removed for being preceded by an erroneous trial. 4.1% of trials were removed for being error trials. 2.6% of trials were removed for being outliers (2.5SDs from the conditional mean of the participant) and 2.6% of trials were dropped for being preceded by an outlier. We then analysed the remaining trials of 607 participants.
Results
We found evidence that RT was influenced by the congruency of the current trial. The estimated RT difference between congruent and incongruent trials was 40.49 ms (SE = 1.9), t(1351.28) = –21.390, BH(0,53) = 2.3*1084, RRB > 3 = [0.13, 16036].
We found evidence that RT was influenced by the interaction between previous and current trial congruency. Estimated effect is 11.5 ms (SE = 2.19), t(165864.84) = −5.25, BH(0,26.5) = 141134.6, RRB > 3 = [0.46, 3955] .
Figure 4. The figure shows the mean reaction time broken down by the congruency of the current and the previous trials for the flanker control experiment. The Y-axis shows the mean RTs in ms. The X axis shows the congruency of the previous trial. The legend shows the congruency of the current trial. Error bars represent the standard error.
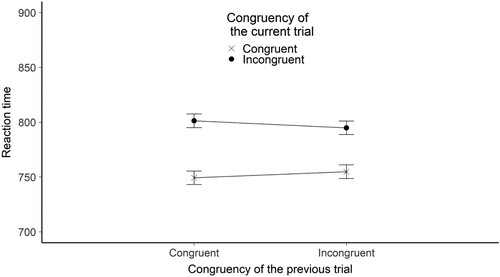
Test experiments
To test the effect of affective signals on control adaptation, we intermixed the two cognitive tasks and the two word lists described in the supplementary materials. Through this procedure, we got 4 different experimental paradigms.
Participants
Every participant was a native Hungarian speaker. Prime-probe data collections were conducted before preregistering the study, but the preregistration was completed before data was analysed.
In both flanker experiments we preregistered the study after data collection, but before analysing the data. With the flanker valence experiment, first we collected data from 110 participants. As stated before, this data collection was conducted before preregistering the study, but the preregistration was completed before data was analysed. The first analysis showed inconclusive results, therefore we proceeded with an additional data collection wave in line with the sequential stopping rule and collected data from 264 more participants adding up to a total of 374. See the participant details in .
Table 1. Total participant number before and after exclusions median age and female percentage in the critical experiment.
Apparatus and stimuli
The above-described self-hosted tool was used to collect data in this case as well. Two kinds of stimuli were used: the above described prime-probe or flanker stimuli with the exact same time and stimulus specifications as in the control experiments; and the affective word stimuli (from the arousal and valence wordlists). Affective word stimuli were shown for 1967ms (target duration) on a grey background with light blue font colour. The participants were instructed to passively read the words but to not respond to them with any keypresses.
Procedure
After the instructions participants performed a practice block with 24 prime-probe or flanker trials. In the practice block after every response a “correct”, an “incorrect”, or a “too late” feedback was shown to inform participants about their performance. After the practice trial, participants were informed that not only prime-probe or flanker trials, but words will appear on the screen as well.
In the prime-probe experiments 4 separate test blocks were shown to participants with 96 trials each. In the flanker experiments test blocks had 80 trials in each. The trial sequence was the following ( and ): A blank screen (1,967 ms in duration); an affective word (high arousal/ low arousal || negative valence / neutral valence, depending on the experiment; 1,967 ms presentation duration); a prime-probe or a flanker stimulus (congruent or incongruent). This meant that only every third trial was a prime-probe or a flanker trial (please note that the newly added blank screens are included in the trial number totals). After every block there was an information screen which allowed the participants to take a break .
Figure 5. The figure shows the experimental sequence in the affective prime-probe task. First a blank screen, then an affective word trial, and finally a prime-probe trial. Each panel shows a trial and each smaller panel shows a stimulus screen, where the three word screen is a prime stimulus and the one word screen is the probe stimulus.
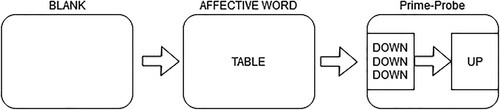
Experiments 1 and 2 – prime-probe with affective words
Arousal and valence words
The only difference between Experiments 1 and 2 was the affective words used for the affective manipulation.
Prime-probe with valence words
Data preprocessing
20 participants were excluded due to accuracy below 70% or a mean RT more than 2.5SDs from the group mean. 3.1% of trials were removed for being incorrect and 2.5% for being outliers (2.5SDs from the conditional mean of the participant). We then analysed the remaining trials of 245 participants.
Results
The models used to test our predictions were identical to the ones used in the control experiments, with the exception that previous word valence replaced previous trial congruency as a predictor. We found evidence that RT is influenced by the congruency of the current trial. Estimated RT difference between congruent and incongruent trials were 70.31 ms (SE = 2.49), t(29020) = −20.47, BH(0,73) = 1.44*1063, RRB > 3 = [0.35, 27692].
In the sequential control adaptation analysis we found evidence supporting the null hypothesis – RT was not influenced by the interaction between previous word valence and current trial congruency. The estimated effect was −5.58 ms (the direction of the interaction is the opposite as in the alternative hypothesis) (SE = 3.52), t(29024.17) = 1.587, BH(0,36.5) = 0.04, RRB < 1/3 = [3.8, infinite] .
Figure 6. The figure shows the mean reaction time broken down by the congruency of the current trial and the valence of the previous word stimulus for the prime – probe valence experiment. The Y-axis shows the mean RTs in ms. The X axis shows the valence of the previous word. The legend shows the congruency of the current trial. Error bars represent the standard error.
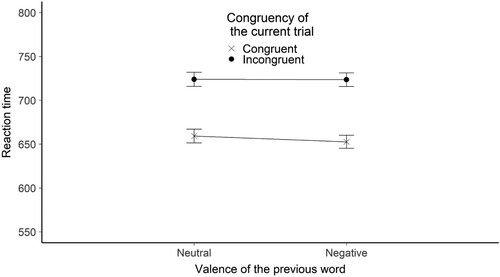
Prime-probe with arousal words
Data preprocessing
45 participants were excluded due to accuracy below 70% or a mean RT more than 2.5SDs from the group mean. 3.6% of trials were dropped for being incorrect and 2.4% for being outliers (2.5SDs from the conditional mean of the participant). Error and post error trials were also removed. We then analysed the remaining trials of 379 participants.
Results
We found evidence that RT was influenced by the congruency of the current trial. Estimated RT difference between congruent and incongruent trials was 68.28 ms (SE = 2.74), t(745.53) = −24.88, BH(0,73) = 4.55*1095 RRB > 3 = [0.22, 27009].
In the sequential control adaptation analysis, we found evidence supporting the null hypothesis – RT was not influenced by the interaction between previous word arousal and the current trial congruency. Estimated effect was −3.12 ms (the direction of the interaction is the opposite as in the alternative hypothesis) (SE = 2.88), t(44685.71) = 1.083, BH(0,36.5) = 0.04, RRB < 1/3 = [3.95, infinite] .
Figure 7. The figure shows the mean reaction time broken down by the congruency of the current trial and the arousal of the previous word stimulus for the prime – probe arousal experiment. The Y-axis shows the mean RTs in ms. The X axis shows the arousal of the previous word. The legend shows the congruency of the current trial. Error bars represent the standard error.
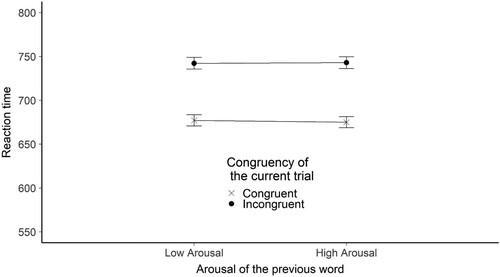
Experiment 3 and 4 – flanker with affective words
Flanker with valence words
Data preprocessing
6.2% of trials were excluded for being over 2000 ms long in reaction times. 20 participants were excluded due to accuracy below 70% or a mean RT more than 2.5SDs from the group mean. 4.5% of trials were removed for being incorrect and 1.5% of trials were removed for being outliers (2.5SDs from the conditional mean of the participant). Error and post error trials were also removed. We then analysed the remaining trials of 352 participants .
Results
We found evidence that RT was influenced by the congruency of the current trial. Estimated RT difference between congruent and incongruent trials was 50.66 ms (SE = 4.55), t(895.86) = −11.14, BH(0,53) = 2.05*1024, RRB > 3 = [0.50, 19636].
In the sequential control adaptation analysis we found evidence for the null hypothesis in the analysis on the RT: the estimated effect was −4 ms (the interaction’s direction was the opposite as in the alternative hypothesis) (SE = 5.66), t(33141.3) = 0.704, BH(0,26.5) = 0.13, RRB < 1/3 = [9.9, infinite] .
Figure 9. The figure shows the mean reaction time broken down by the congruency of the current trial and the valence of the previous word stimulus for the flanker valence experiment. The Y-axis shows the mean RTs in ms. The X axis shows the valence of the previous word. The legend shows the congruency of the current trial. Error bars represent the standard error.
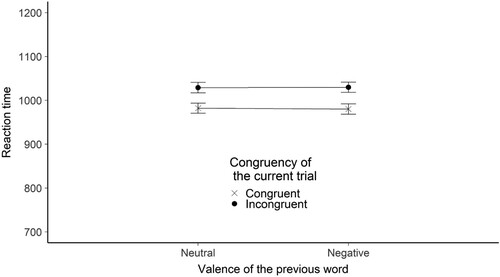
Flanker with arousal words
Data preprocessing
5.1% of trials were removed for exceeding a reaction time of 2000ms. 7 participants were excluded due to accuracy below 70% or a mean RT more than 2.5SDs from the group mean. 4.8% of trials were dropped for being incorrect, and 4.3% of trials were removed for being outliers (2.5SDs from the conditional mean of the participant). Error and post error trials were also removed. We then analysed the remaining trials of 126 participants.
Results
We found evidence that RT was influenced by the congruency of the current trial. Estimated RT difference between congruent and incongruent trials was 61.5 ms (SE = 7.82), t(292.7) = −7.87, BH(0,53) = 2.63*1011, RRB > 3 = [1.29, 22969].
In the sequential control adaptation analysis we found evidence supporting the null hypothesis – RT was not influenced by the interaction between previous word arousal and current trial congruency. Estimated effect was −16.41 ms (the interaction’s direction was the opposite as in the alternative hypothesis) (SE = 9.36), t(11926.87) = 1.75, BH(0,26.5) = 0.13, RRB < 1/3 = [9.29, infinite] .
Figure 10. The figure shows the mean reaction time broken down by the congruency of the current trial and the arousal of the previous word stimulus for the flanker valence experiment. The Y-axis shows the mean RTs in ms. The X axis shows the arousal of the previous word. The legend shows the congruency of the current trial. Error bars represent the standard error.
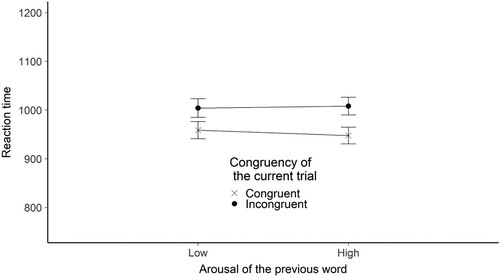
Additional exploratory analyses
Although the affective experimental procedures were constructed with an attempt to avoid sequential control modulations between consecutive conflict trials, we conducted non-preregistered analyses to determine whether such modulations occurred nonetheless, and whether they interacted with the affective content of stimuli between adjacent conflict trials. We found conflict adaptation effects between consecutive flanker (or prime-probe) trials in three of the four crucial experiments, regardless of the blank and affective word stimuli between them. This finding is in line with the recent findings of Schiltenwolf et al. (Citation2022), where the CSE was found to be robust to temporal decay.
We have also found effects that suggest affective modulation of the sequential adaptation of control. These results are somewhat in line with several studies related to the affective signalling theory (N. Cohen et al., Citation2011; van Steenbergen et al., Citation2009; Zeng et al., Citation2017). For more details, see the supplementary materials.
Discussion
The Affective Signalling Hypothesis (Dignath et al., Citation2020; Dreisbach & Fischer, Citation2012; van Steenbergen, Citation2015) implies that signal aversiveness can activate an affect monitoring system and as a consequence, lead to control adaptation. There are several studies (Dignath et al., Citation2020; van Steenbergen, Citation2015) that show a connection between affect regulation and conflict adaptation, but they did not explore the main implication of the strongest form of the hypothesis: that stimulus aversiveness could drive conflict adaptation in and of itself. The present study investigated the effect of affective signals on control adaptation. By conducting the above experiments, based on recent findings, we expected aversive signals to lead to an increase in cognitive control levels, mimicking the effect of conflicting stimuli, and lending support to the idea that affective regulation mediates conflict-related adaptations.
We collected data among university students with an online experimenting tool specifically designed for this task. In Experiments 1 and 2, prime-probe tasks were interspersed by affective words that were manipulated on the valence dimension in Experiment 1 and arousal dimension in Experiment 2. In Experiments 3 and 4, we intermixed flanker tasks and affective words that were manipulated on the valence or arousal dimension, respectively. Our Bayesian analysis approach let us differentiate between inconclusive evidence for the alternative hypothesis and evidence for the null effect. While the control tasks (i.e. conflict tasks with no affective material) resulted in evidence for the CSE which showed that the design is capable of measuring conflict adaptation, the affective experiments yielded evidence for no effect between affective words and conflict tasks.
Our results provide evidence that suggests that affective signals without response or stimulus conflict cannot trigger control adaptation on their own. This finding is novel and is in contrast to recent theories regarding affective signals.
Dreisbach and Fischer (Citation2011) found that sequential fluency manipulations can lead to elevated control. The pattern of this effect is in line with the conflict adaptation effect but without response conflict. The authors proposed that the aversiveness of disfluent signals is responsible for these performance adjustments. It is to be noted, however, that this can also indicate that the underlying mechanism behind the CSE is more general than we thought before and it is not exclusive to response conflicts. It is possible that fluency was confounded by factors other than aversiveness, for instance the elevated effort on disfluent trials that could sequentially modulate subsequent performance.
A viable explanation to the above results is that the affective signal effect is present, however, the noise caused by task switching masks it. Braem et al. (Citation2014) suggest that differences in task relevant information between two tasks reduces cross-task adaptation effects. By using verbal stimuli in both the affective word reading tasks and the conflict tasks we aimed to reduce the possibility of domain-switching effects. However, reading passively a task-irrelevant word and responding to a conflict task still engage different task sets. A replication of an influential study (Kan et al., Citation2013) showed that conflict adaptation may not transfer between different tasks, therefore it is possible that conflict adaptation effects are task-specific despite the recent assumption of domain-general control (Aczel et al., Citation2021). It is also worth noting that the negative results may be due to the lack of self-relevance: Landman and van Steenbergen (Citation2020) have found that self-related affective stimuli increase conflict adaptation, however, when it is not self-related the increase is not observable. By implementing affective signals without any relevance to the task or the participant, the effect of those signals might be reduced. Furthermore, the informational role of affective stimuli also needs to be considered: According to Schwarz (Citation1990) negative affective states inform the individual about a threat, or a possibility of negative outcomes and thus might motivate the individual to heighten cognitive efforts. This however can only be observed in situations where the affective states and goals of an individual (e.g. solve an experimental task) are related. In our case it is possible that affective stimuli were not interpreted as information related to the situation and thus did not elicit increased cognitive effort. Another parallel effect might be the unrelated arousal of affective stimuli: Zeng et al. (Citation2017) have found increased control adaptation in high arousal affective tasks, however, they did not separate affective cues from the task stimuli. Contradicting this, Pessoa (Citation2009) provides a framework in which high arousal stimuli are suggested to impair executive control by expending or diverting needed resources. A later study (Padmala et al., Citation2011) has indeed shown results that suggested that phasic high arousal negative emotions impair, rather than increase conflict adaptation.
The exploratory investigations (see in the supplementary materials) we conducted, however, provided results similar to recent findings that suggest the modulating effects of affective signals on sequential control adaptation. We found CSE between consecutive flanker and prime-probe trials in 3 of 4 experiments, regardless of the long gap (approximately 4 s) between them. This CSE was twice as big after a negative word than after a neutral word in the flanker task. In the flanker with arousal words experiment, we have also found a pattern similar to recent findings (N. Cohen et al., Citation2011) where preceding incongruent flankers impaired the effect of emotion on subsequent task performance. Notably, these findings are based on complex, exploratory interactions, as such they provide no conclusive evidence for any hypothesis, however, paired with the null-findings on the original analyses, they suggest a pattern where phasic affective states indeed have a modulating role in conflict adaptation, however, they are not sufficient to elicit it by themselves.
An important limitation of this study is that affective words were measured on the relevant affective dimensions, however, there is no data on how the subjective, perceived valence or arousal differed between subjects in the experimental sample. The above-mentioned self-relevance domain has not been addressed by the design either. The passively observed words might elicit affect, but the lack of task might reduce the relevance of the words, and also break the flow of attention during the experiment. It is possible that consciously, or unconsciously realising that there is no challenge, or any relevant benefit in reading the words, participants used word trials as a break in attentional effort, thus their affective effects were smaller than hypothesised. Additionally, not only the irrelevance, but the aversiveness of the words could also drive participants to avoid reading them which possibility was not accounted for in this study. The usage of affective words also introduced a minor limitation to our design because we could not fully control for valence or arousal in the arousal and valence arousal wordlists, respectively, due to the closely intertwined nature of these two dimensions in verbal stimuli. These dimensions however often co-occur in any real-word aversive stimuli; hence we find it unlikely that this lack of separation has affected our findings.
The above limitations could be addressed by a design, where participants are more involved in the processing of affective words (albeit this could increase the chance of task switching effects between affective words and conflict trials), and presentation length could also be shortened for more immediate phasic effects.
Our hypothesis was that phasic affective signals by themselves can trigger adaptive control, in this study we now have found evidence against it. A wide range of recent studies report, however, the modulating effect of affective signals in conflict tasks suggesting an important role of such signals in the adaptation of control. In fact, aversive signals may still play a causal role in control dynamics, but if they do, they likely need to stem from the control demands relevant to the task at hand. Further investigations are needed to uncover the mechanisms of affective signals’ modulating effect on cognitive control.
Ethics
Eotvos Lorand University’s institutional ethical review board agrees with the proposed data collection. Ethical permission numbers: [2020/330; 2020/362; 2020/473]. This study was conducted in accordance with the Declaration of Helsinki.
Data accessibility
All of our analyses were publicly preregistered on the OSF site Collected raw data is publicly shared on the OSF pages of the project. Code for data management and statistical analyses were written in R and are open access. All materials of the experiments are available through OSF: (Affective wordset analysis: https://osf.io/rpcw7/; Prime-probe experiments: https://osf.io/jzp9w/; Flanker experiments: https://osf.io/z7w5c/).
Supplemental Material
Download Zip (745 KB)supplementary_materials_rev2.docx
Download MS Word (737 KB)Acknowledgements
We are grateful to Zsuzsa Székely for her help in the data collection, Bence Pálfi for his insights in the statistical methodology, Matt Jaquiery for his help with the experiment script and Márton Kovács for his help with the analysis software. Last but not least we are grateful to the editor Dr. Andreas Eder and the two reviewers for their valuable comments and suggestions. Authors contributions: Balazs Aczel: Conceptualization, Funding acquisition, Supervision, Validation, and Writing – review & editing. Henk van Steenbergen: Supervision and Writing – review & editing. Mate Gyurkovics: Conceptualization, Supervision, Validation, and Writing – review & editing. Miklos Bognar: Conceptualization, Data curation, Formal analysis, Investigation, Methodology, Project administration, Resources, Software, Visualization, Writing – original draft, and Writing – review & editing.
Disclosure statement
No potential conflict of interest was reported by the author(s).
Additional information
Funding
References
- Aczel, B., Kovacs, M., Bognar, M., Palfi, B., Hartanto, A., Onie, S., Tiong, L. E., & Evans, T. R. (2021). Is there evidence for cross-domain congruency sequence effect? A replication of Kan et al. (2013). Royal Society Open Science, 8(3), 191353. https://doi.org/10.1098/rsos.191353
- Berger, A., Mitschke, V., Dignath, D., Eder, A., & van Steenbergen, H. (2020). The face of control: Corrugator supercilii tracks aversive conflict signals in the service of adaptive cognitive control. Psychophysiology, 57(4), e13524. https://doi.org/10.1111/psyp.13524
- Botvinick, M. M. (2007). Conflict monitoring and decision making: Reconciling two perspectives on anterior cingulate function. Cognitive, Affective, & Behavioral Neuroscience, 7(4), 356–366. https://doi.org/10.3758/CABN.7.4.356
- Botvinick, M. M., Braver, T. S., Barch, D. M., Carter, C. S., & Cohen, J. D. (2001). Conflict monitoring and cognitive control. Psychological Review, 108(3), 624–652. https://doi.org/10.1037/0033-295X.108.3.624
- Braem, S., Abrahamse, E. L., Duthoo, W., & Notebaert, W. (2014). What determines the specificity of conflict adaptation? A review, critical analysis, and proposed synthesis. Frontiers in Psychology, 5, 1134.https://doi.org/10.3389/fpsyg.2014.01134
- Cohen, J. D. (2017). Cognitive control: Core constructs and current considerations. In T. Egner (Ed.), The wiley handbook of cognitive control (pp. 3–28). Wiley Blackwell. https://doi.org/10.1002/9781118920497.ch1
- Cohen, N., Henik, A., & Mor, N. (2011). Can emotion modulate attention? Evidence for reciprocal links in the attentional network test. Experimental Psychology, 58(3), 171–179. https://doi.org/10.1027/1618-3169/a000083
- Dienes, Z., & Mclatchie, N. (2018). Four reasons to prefer Bayesian analyses over significance testing. Psychonomic Bulletin & Review, 25(1), 207–218. https://doi.org/10.3758/s13423-017-1266-z
- Dignath, D., Eder, A. B., Steinhauser, M., & Kiesel, A. (2020). Conflict monitoring and the affective-signaling hypothesis—An integrative review. Psychonomic Bulletin & Review, 27(2), 193–216. https://doi.org/10.3758/s13423-019-01668-9
- Dignath, D., Janczyk, M., & Eder, A. (2017). Phasic valence and arousal do not influence post-conflict adjustments in the Simon task. Acta Psychologica, 174, 31–39. https://doi.org/10.1016/j.actpsy.2017.01.004
- Dimberg, U. (1990). Facial electromyographic reactions and autonomic activity to auditory stimuli. Biological Psychology, 31(2), 137–147. https://doi.org/10.1016/0301-0511(90)90013-M
- Dreisbach, G., & Fischer, R. (2011). If it’s hard to read … try harder! processing fluency as signal for effort adjustments. Psychological Research, 75(5), 376–383. https://doi.org/10.1007/s00426-010-0319-y
- Dreisbach, G., & Fischer, R. (2012). Conflicts as aversive signals. Brain and Cognition, 78(2), 94–98. https://doi.org/10.1016/j.bandc.2011.12.003
- Dreisbach, G., & Fischer, R. (2015). Conflicts as aversive signals for control adaptation. Current Directions in Psychological Science, 24(4), 255–260. https://doi.org/10.1177/0963721415569569
- Duthoo, W., Abrahamse, E. L., Braem, S., Boehler, C. N., & Notebaert, W. (2014). The heterogeneous world of congruency sequence effects: An update. Frontiers in Psychology, 5, 1001. https://doi.org/10.3389/fpsyg.2014.01001
- Egner, T. (2007). Congruency sequence effects and cognitive control. Cognitive, Affective, & Behavioral Neuroscience, 7(4), 380–390. https://doi.org/10.3758/CABN.7.4.380
- Erb, C. D., & Aschenbrenner, A. J. (2019). Multiple expectancies underlie the congruency sequence effect in confound-minimized tasks. Acta Psychologica, 198, 102869. https://doi.org/10.1016/j.actpsy.2019.102869
- Eriksen, B. A., & Eriksen, C. W. (1974). Effects of noise letters upon the identification of a target letter in a nonsearch task. Perception & Psychophysics, 16(1), 143–149. https://doi.org/10.3758/BF03203267
- Fröber, K., Stürmer, B., Frömer, R., & Dreisbach, G. (2017). The role of affective evaluation in conflict adaptation: An LRP study. Brain and Cognition, 116, 9–16. https://doi.org/10.1016/j.bandc.2017.05.003
- Funes, M. J., Lupiáñez, J., & Humphreys, G. (2010). Analyzing the generality of conflict adaptation effects. Journal of Experimental Psychology. Human Perception and Performance, 36(1), 147–161. https://doi.org/10.1037/a0017598
- Gratton, G., Coles, M. G., & Donchin, E. (1992). Optimizing the use of information: Strategic control of activation of responses. Journal of Experimental Psychology. General, 121(4), 480–506. https://doi.org/10.1037/0096-3445.121.4.480
- Gyurkovics, M., Kovacs, M., Jaquiery, M., Palfi, B., Dechterenko, F., & Aczel, B. (2020). Registered replication report of Weissman, D. H., Jiang, J., & Egner, T. (2014). Determinants of congruency sequence effects without learning and memory confounds. Attention, Perception, & Psychophysics, 82(8), 3777–3787. https://doi.org/10.3758/s13414-020-02021-2
- Kan, I. P., Teubner-Rhodes, S., Drummey, A. B., Nutile, L., Krupa, L., & Novick, J. M. (2013). To adapt or not to adapt: The question of domain-general cognitive control. Cognition, 129(3), 637–651. https://doi.org/10.1016/j.cognition.2013.09.001
- Kanske, P., & Kotz, S. A. (2011). Emotion triggers executive attention: Anterior cingulate cortex and amygdala responses to emotional words in a conflict task. Human Brain Mapping, 32(2), 198–208. https://doi.org/10.1002/hbm.21012
- Landman, L. L., & van Steenbergen, H. (2020). Emotion and conflict adaptation: The role of phasic arousal and self-relevance. Cognition and Emotion, 34(6), 1083–1096. https://doi.org/10.1080/02699931.2020.1722615
- Larsen, J. T., Norris, C. J., & Cacioppo, J. T. (2003). Effects of positive and negative affect on electromyographic activity over zygomaticus major and corrugator supercilii. Psychophysiology, 40(5), 776–785. https://doi.org/10.1111/1469-8986.00078
- Lee, J., & Cho, Y. S. (2013). Congruency sequence effect in cross-task context: Evidence for dimension-specific modulation. Acta Psychologica, 144(3), 617–627. https://doi.org/10.1016/j.actpsy.2013.09.013
- Li, W., Jiang, Z., Liu, Y., Wu, Q., Zhou, Z., Jorgensen, N., Li, X., & Li, C. (2014). Positive and negative emotions modulate attention allocation in color-flanker task processing: Evidence from event related potentials. Motivation and Emotion, 38(3), 451–461. https://doi.org/10.1007/s11031-013-9387-9
- Morsella, E., Gray, J. R., Krieger, S. C., & Bargh, J. A. (2009). The essence of conscious conflict: Subjective effects of sustaining incompatible intentions. Emotion (Washington, D.C.), 9(5), 717–728. https://doi.org/10.1037/a0017121
- Padmala, S., Bauer, A., & Pessoa, L. (2011). Negative emotion impairs conflict-driven executive control. Frontiers in Psychology, 2, 192. https://doi.org/10.3389/fpsyg.2011.00192
- Pessoa, L. (2009). How do emotion and motivation direct executive control? Trends in Cognitive Sciences, 13(4), 160–166. https://doi.org/10.1016/j.tics.2009.01.006
- Schiltenwolf, M., Kiesel, A., & Dignath, D. (2022). No temporal decay of cognitive control in the congruency sequence effect. Journal of Experimental Psychology: Learning, Memory, and Cognition. https://doi.org/10.1037/xlm0001159
- Schönbrodt, F. D., Wagenmakers, E.-J., Zehetleitner, M., & Perugini, M. (2017). Sequential hypothesis testing with Bayes factors: Efficiently testing mean differences. Psychological Methods, 22(2), 322–339. https://doi.org/10.1037/met0000061
- Schwarz, N. (1990). Feelings as information: Informational and motivational functions of affective states. In R. M. Sorrentino & E. T. Higgins (Eds.), Handbook of motivation and cognition: Foundations of social behavior (Vol. 2, pp. 527–561). The Guilford Press.
- Stroop, J. R. (1935). Studies of interference in serial verbal reactions. Journal of Experimental Psychology, 18(6), 643–662. https://doi.org/10.1037/h0054651
- van Steenbergen, H. (2015). Affective modulation of cognitive control: A biobehavioral perspective. In G. H. E. Gendolla, M. Tops, & S. L. Koole (Eds.), Handbook of biobehavioral approaches to self-regulation (pp. 89–107). Springer. https://doi.org/10.1007/978-1-4939-1236-0_7
- van Steenbergen, H., Band, G. P. H., & Hommel, B. (2009). Reward counteracts conflict adaptation: Evidence for a role of affect in executive control. Psychological Science, 20(12), 1473–1477. https://doi.org/10.1111/j.1467-9280.2009.02470.x
- van Steenbergen, H., Band, G. P. H., & Hommel, B. (2010). In the mood for adaptation: How affect regulates conflict-driven control. Psychological Science, 21(11), 1629–1634. https://doi.org/10.1177/0956797610385951
- Vermeylen, L., Wisniewski, D., González-García, C., Hoofs, V., Notebaert, W., & Braem, S. (2020). Shared neural representations of cognitive conflict and negative affect in the medial frontal cortex. The Journal of Neuroscience, 40(45), 8715–8725. https://doi.org/10.1523/JNEUROSCI.1744-20.2020
- Wang, Y., Yang, L., & Wang, Y. (2014). Suppression (but Not reappraisal) impairs subsequent error detection: An ERP study of emotion regulation’s resource-depleting effect. PLOS ONE, 9(4), e96339. https://doi.org/10.1371/journal.pone.0096339
- Weissman, D. H., Jiang, J., & Egner, T. (2014). Determinants of congruency sequence effects without learning and memory confounds. Journal of Experimental Psychology. Human Perception and Performance, 40(5), 2022–2037. https://doi.org/10.1037/a0037454
- Yang, Q., & Pourtois, G. (2018). Conflict-driven adaptive control is enhanced by integral negative emotion on a short time scale. Cognition and Emotion, 32(8), 1637–1653. https://doi.org/10.1080/02699931.2018.1434132
- Zeng, Q., Qi, S., Li, M., Yao, S., Ding, C., & Yang, D. (2017). Enhanced conflict-driven cognitive control by emotional arousal, not by valence. Cognition and Emotion, 31(6), 1083–1096. https://doi.org/10.1080/02699931.2016.1189882