ABSTRACT
Affective Working Memory (AWM) is the ability to maintain an emotion after the emotion-eliciting stimulus is no longer present. Emotions are dynamic, and emotion-eliciting stimuli are encountered simultaneously and sequentially. Therefore, this research aimed to examine AWM when more than one emotion is being maintained. We aimed to re-examine previous findings, that people are better at maintaining positive than negative emotions in the context of dynamic presentations of multiple stimuli. We introduce a modified maintenance task, and present a novel metric that models the latent maintenance processes to acquire an accurate measure of AWM. Participants (N = 49) were asked to complete the study online. On each trial, participants were presented with a sequence of three images, and were asked to compare the intensity of the emotion elicited by image 1 to image 3, whilst maintaining the emotion elicited by image 2 to rate it at the end of the trial. The results showed that people are successful at simultaneously maintaining two emotions in AWM, and they replicate previous findings concerning the advantage of maintaining positive compared to negative emotions. Overall, the study highlights the complexity of AWM and provides insight into the processes involved in maintaining multiple emotions simultaneously.
Imagine that while you are excited and on your way to talk about your life achievements at your old school, you see a kid falling. This incident might affect your ability to maintain your ongoing excitement. As in this example, in everyday life, emotional experiences are often mixed, and people respond to different emotion-eliciting information at the same time (Cacioppo et al., Citation2011). In this research, we explore such simultaneous maintenance of emotions and focus on how experiencing an emotion elicited by one event affects the maintenance of another emotion elicited by a different event. As in the example, simultaneous emotional experiences can be of mixed valence, but one can also attempt to maintain two same-valence emotional experiences. Therefore, we compare maintenance of positive and negative emotions when experienced with congruent or incongruent emotions.
The system responsible for maintaining and manipulating information in the service of goal-directed behaviour is working memory (WM; Baddeley, Citation2021). Specifically, WM is a set of cognitive processes responsible for maintaining and manipulating representations of stimuli for a short duration of time to serve higher-order cognitive processes such as reasoning and problem-solving (Baddeley, Citation2021; Brady & Störmer, Citation2022).
Emotions have been shown to play an important role in WM, and researchers have defined several modes in which emotions and WM interact (Mikels & Reuter-Lorenz, Citation2019). One mode in which emotions and WM interact is when the emotions themselves constitute mental representations in WM. This ability to maintain and manipulate mental representations of emotions in the absence of the emotion-eliciting stimulus has been termed affective working memory (AWM; Mikels et al., Citation2008; Mikels & Reuter-Lorenz, Citation2019).
AWM is a goal-directed process that involves active maintenance and “holding in mind” of an emotion for a later time. AWM is needed because emotions consist of valence, intensity, and hedonic qualities that are critical for guiding goal-directed thoughts and actions (Tamir & Millgram, Citation2017). AWM shares commonalities with WM, particularly in their reliance on similar neural substrates, such as the prefrontal cortex, and their involvement in executive-control processes (Baddeley, Citation2003; Waugh et al., Citation2014). However, they differ fundamentally in the type of information processed; AWM addresses emotional complexities, encompassing valence and intensity (Mikels & Reuter-Lorenz, Citation2019).
In this research, as in Mikels and Reuter-Lorenz (Citation2019), we adopt the multicomponent view (Cowan, Citation2017) of AWM: like verbal and visuospatial WM, AWM is a domain-specific WM subsystem with specialised processes for maintaining feelings, and executive-control processes that likely overlap, in whole or in part, with other WM subsystems (Christophel et al., Citation2017; Lewis-Peacock et al., Citation2012). Recent work has shown that multiple representations in WM can simultaneously guide attention or be concurrently represented in active neural state (Fan et al., Citation2023; Sutterer et al., Citation2019). However, until now, research on AWM has focused on the maintenance of a single emotional state (Gruber et al., Citation2013; Waugh et al., Citation2014). Emotional states co-occur (Berrios et al., Citation2015), and simultaneous emotions can arise in response to a single stimulus or in response to a sequence of stimuli that elicit sustained emotions (Cacioppo et al., Citation2011; Norris et al., Citation2010). Drawing parallels with WM, which actively retains specific mental contents while performing cognitive operations on them or on other mental contents (Miller et al., Citation2018; Oberauer et al., Citation2000), this study explores a similar concept in the emotional domain. Specifically, the first aim of this research is to investigate how maintaining one emotional state may be affected by the concurrent maintenance of another.
The effect of maintaining one emotion on the ability to maintain simultaneously an emotional response to another stimulus may depend on whether the two emotions are of similar valence (congruent) or not (incongruent). Specifically, WM performance is worse when the information being held in WM and the new input is of matching valence (Hur et al., Citation2017). A possible mechanism for such interference in WM is that congruent stimuli require more inhibition than stimuli that elicit different emotions (congruence interference effect; Mather, Citation2009). This explanation is in line with prior work that suggests that positive and negative emotions are created in different “dimensions” and form a bivariate space, in which they can occur concurrently (Cacioppo et al., Citation2011). Holding in mind two congruent emotions may result in competition over the same AWM resources which could interfere with the maintenance process (Hur et al., Citation2017; van Dillen & Hofmann, Citation2023). Therefore, the second aim of this study is to examine whether maintenance is dependent on valence congruence of the two maintained emotions. We predicted that maintaining congruent emotions would interfere more with the maintenance process, compared to maintaining incongruent emotions.
Understanding the maintenance of positive and negative emotions is pivotal for insights into well-being and psychopathology (LeMoult & Gotlib, Citation2019). For example, people with bipolar disorder show a deficit in AWM maintenance of negative but not positive emotions (Gruber et al., Citation2013). Moreover, whereas the tendency to maintain positive emotions (Waugh et al., Citation2019) is related to increased positive mood and well-being (Seligman, Citation2018), maintenance of negative affect contributes to persistent negative mood, a core feature of depression (LeMoult & Gotlib, Citation2019). Previous studies yielded mixed results: while some found that maintenance of negative emotions is better than that of positive ones (Mikels et al., Citation2005; Mikels et al., Citation2008) others did not report differences between positive and negative emotions (Frank et al., Citation2023; Gard et al., Citation2011). Waugh et al. (Citation2019) using improved metrics of AWM (see below), found that people are better at maintaining positive than negative emotions. They argued that while negative emotions signal danger and lose relevance once the threat subsides (Baumeister et al., Citation2001), people are naturally inclined to prolong pleasurable states and thus invest more in enhancing positive emotions (Tamir & Millgram, Citation2017). In light of the inconsistent findings regarding the maintenance of positive and negative emotions, the third aim of this study is to compare maintenance of positive and negative emotions. Consistent with Waugh et al. (Citation2019) and based on the metrics used in that study, we predicted that people would show greater maintenance of positive compared to negative emotions even when simultaneously maintaining another emotion.
Mikels et al. (Citation2008) developed a novel paradigm to assess AWM. In this paradigm, two images were presented for a short duration, separated by a delay period. Participants in the emotion maintenance condition were asked to maintain the emotion elicited by the first image, then compare the intensity of that emotion to another emotion elicited by the second image, and finally indicate which image elicited a more intense emotional response. Participants in the non-emotional maintenance condition were asked to compare the brightness of two images. Using this paradigm, Mikels et al. (Citation2008) showed that AWM is partially separate from maintenance of the visual and verbal representations of emotion-eliciting stimuli.
Several metrics have been used to assess AWM: 1. The accuracy in choosing the image with the higher emotional intensity as compared to previously normed intensity values of the images (normative concordance, Mikels et al., Citation2008), 2. Accuracy based on idiographic intensity ratings that take into account the individual's own ratings of the images (Waugh et al., Citation2019), 3. Accuracy in trials in which the first image should have been selected as higher or accuracy in trials in which the second image should have been selected (1gtr2 and 2gtr1 respectively; Waugh et al., Citation2019). These metrics have several limitations. The normative concordance metric does not distinguish between incorrect responses that are due to a failure to maintain the emotional intensity and those that are attributed to individual differences in participants’ judgements of the emotional intensity elicited by the images. Moreover, the normative concordance and the idiographic accuracy metrics do not differentiate between AWM maintenance and other WM processes such as recency effects. Although 1gtr2 and 2gtr1 metrics were developed to address these limitations, they do not distinguish between the maintenance process and people's bias to choose either the first or the second image. In particular, the 1gtr2 metric is affected by a bias to select the first image (B1), and the 2gtr1 metric is affected by a bias to select the second image (1 − B1).
To overcome the limitations of available metrics of AWM maintenance, we modelled the latent maintenance construct to assess AWM. In contrast to the previous metrics, this latent maintenance construct accurately estimates AWM by separating people's bias to choose the first or the last image when asked which image elicits higher emotional intensity, from their ability to maintain the emotion in AWM.
In order to examine AWM for co-occurring emotional states, we modified the AWM task to include three images instead of two, and asked participants to maintain concurrently two emotional states. First, as in the original AWM task, participants compared the emotional intensity elicited by the first and the third images (target emotion 1). Then, at the end of the trial, participants rated the emotional valence of the second image (target emotion 2). This delayed rating of image 2 served to induce the maintenance of the emotion elicited by that image (Waugh et al., Citation2019). Participants were given different instructions for maintaining emotional characteristics of images 1 and 3 compared to image 2 in order to separate the maintenance processes and to differentiate target emotion 2 from target emotion 1. Thus, the participants were encouraged to maintain two different emotions at once instead of blending them into a single emotion. Moreover, the different instructions allowed the participants to differentiate between the tasks easily. As in the original AWM task, the compared stimuli (images 1 and 3) were always of the same valence (positive or negative), whereas image 2 was either positive, negative or neutral.
We explored the following questions: 1. Can people maintain an emotion while simultaneously experiencing another emotion, 2. Is maintenance lower when the simultaneously maintained emotions are valence-congruent as opposed to incongruent, and 3. Is maintenance higher for positive than negative target emotions. We utilised accuracy in 1gtr3 trials and the latent maintenance construct to measure maintenance. To test the superiority of the latent maintenance construct over accuracy in 1gtr3 trials, we assessed whether participants show a bias to choose image 1 or 3.
Method
Participants
A total of 49 participants (25 males, 23 females, 1 other) were recruited via the Prolific Academic platform (www.prolific.co) in return for payment. All participants were native English speakers from the US or UK. To be eligible, participants had to be between 18 and 40 years of age, with correct or corrected vision and a minimum of 95% approval rate (the percent of previous submissions that were approved). Sample size was determined using a power analysis software (G*Power; Faul et al., Citation2007). N of 46 was selected to provide power = .90 to detect a small-medium effect (η2 = .03), based on Waugh et al. (Citation2019) that found small to large effect sizes. Additional participants were recruited to allow removal of participants with high numbers of invalid trials (trials in which participant's post task ratings of image 1 and 3 match). Data from only one participant was removed due to a high number (4SD above mean) of invalid trials, based on the interquartile method (Laurikkala et al., Citation2000; Smiti, Citation2020).Footnote1 Therefore, the final number of participants was 48. Participants’ mean age was 30 years (SD = 5.46). The majority of the sample identified as Caucasian, three participants were of African American ethnicity, one participant was of Asian ethnicity, and one participant described themselves as mixed. Most participants had at least a bachelor's degree (59%).
Tasks and measures
AWM task
We used a modified version of the task developed by (Mikels et al., Citation2008) and (Waugh et al., Citation2019). On each trial of the modified task, participants were presented with a sequence of three emotional images (). Participants were asked to hold in mind the emotional intensity of the first image (target emotion 1) and to compare it to the emotional intensity of the third image, and to hold in mind the valence of image 2 and rate its valence later (target emotion 2). Following the presentation of the three images, participants were asked to decide whether the emotional intensity of the third image was higher or lower than the first image, and then to rate the valence of image 2 (from (1) very negative to (7) very positive).
Figure 1. Sample trial of affective working memory task.
Notes: A sample trial presenting a positive target picture 1 (picture 1 and 3) and a negative target picture 2 (picture 2). The images are example images retrieved from Microsoft office 365, to protect IAPS copyright.
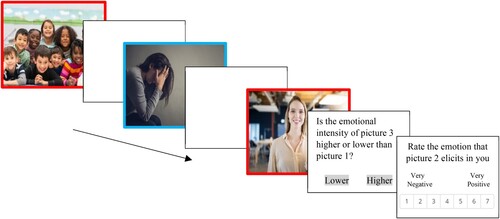
In order to make the distinction between the images, images 1 and 3 were presented in a red frame and image 2 was presented in a blue frame. Each image was presented for 3 s with a 3-s delay between each image, resulting in a 9 s maintenance time from image 1 and image 3. The task consisted of a total of 78 trials. Half the trials consisted of positive images 1 and 3 to depict positive target emotion 1, whereas the other half consisted of negative images 1 and 3 to depict negative target emotion 1. Each target emotion 1 was presented with either a positive, negative, or neutral image 2. Overall, positive/negative target emotion 1 was presented with each target emotion 2 in 13 trials. The trial order was randomised across participants.
Stimuli
The images used in the AWM task were taken from the International Affective Image System (IAPS; Lang et al., Citation1999). We used norms of emotional intensity by Mikels et al. (Citation2008), to select positive (M = 3.48, SD = 0.70) and negative (M = 3.68, SD = .72) image sets (image 1 and 3, i.e. target emotion 1) that did not differ in mean intensity (t(154) = 1.76, p = .08). Moreover, we used the IAPS (Lang et al., Citation1999) norms to select positive (valence: M = 7.14, SE = 0.12, arousal: M = 5.04, SE = 0.15), negative (valence: M = 2.93, SE = .12, arousal: M = 5.39, SE = 1.5) and neutral (valence: M = 5.02, SE = 0.12, arousal: M = 3.10, SE = 0.15) images for image 2 (see supplementary materials for additional considerations for choosing the stimuli).
Post task idiographic ratings
After completing the AWM task and a distraction task, participants were asked to rate the intensity of all the images displayed in the AWM task (images 1, 2, 3). Ratings were on a scale ranging from 0 “low emotional intensity” to 10 “high emotional intensity”. These ratings were used to calculate the participants' idiographic accuracy in the task.
AWM performance
To assess participants’ maintenance of emotional states, we calculated an idiographic metric of performance (e.g. Waugh et al., Citation2019). We calculated the percentage of trials for which participants’ responses matched their post-task ratings (e.g. participant chose image 3 as higher than image 1 in intensity, and rated the intensity of image 3 higher than image 1). For example, a total of 70% accuracy indicated that in 70% of trials, the participants’ responses matched their idiographic ratings. Trials were only counted if participants’ post-task ratings differed between the two images (valid trials). Trials with exactly the same post-task ratings (equals) were not included in the analyses (16% invalid trials). We also calculated separately the idiographic accuracy for the trials in which participants’ post-task ratings indicated that they should have selected the first image as being more intense than the third (1gtr3) and vice-versa (3gtr1). Lastly, we calculated the latent maintenance construct for each participant, and their tendency to choose either image 1 or image 3 as higher regardless of their actual assessment of which image had higher intensity.
Calculations of the latent maintenance construct
Using a multinomial processing tree (MPT), we estimated the probabilistic weighting of latent affective states in binary decision tasks (Batchelder & Riefer, Citation1999). Then, we constructed an AWM model () in which we estimated latent states that represent the successful maintenance (M) of the affective state for 1gtr3 and 3gtr1 conditions. Unsuccessful maintenance (1 − M) allowed us to estimate latent states that represent a bias toward choosing the first image as more intense (B1) or a bias toward choosing the third image as more intense (1 − B1), which allowed us to estimate M (see supplementary material for additional calculations).
Procedure
The study was administered online. After signing a consent form, participants completed the AWM task. Then, they completed a 2-minute distraction task, in which they were presented with four words and were asked to use the letters of each word to create new words. Next, they completed the idiographic rating task. Finally, they were asked to complete a demographics questionnaire (and an additional questionnaire that is not reported in this study). The experimental session took approximately 45 min to complete.
Results
Analytic plan
We conducted two preliminary analyses. First, to assess whether 1gtr3 is a reliable indicator of maintenance, we checked for a preference for image 1 by assessing whether the bias for image 1 (B1) is significantly greater than zero. Second, to ascertain that participants indeed maintained the second image in WM, we compared their idiographic valence ratings with the normative valence ratings from the IAPS (Lang et al., Citation1999), following the reasoning that such correspondence indicates maintenance of the image.Footnote2
Because of concerns over carry-over effects of the simultaneous maintenance on maintaining a single emotion, a control condition of single-emotion maintenance was not included in the study. Instead, to address our first aim, we compared our idiographic accuracy scores to those in similar studies in which a single emotion was maintained (studies 1–3 in Waugh et al., Citation2019). To address our second and third aims, we used both the accuracy in 1gtr3 trials and the latent maintenance construct (M) and conducted repeated measures analyses with valence of target emotions 1 and 2 as within-subjects predictors.
Preliminary analyses
Participants’ bias to choose image 1 (B1) was significantly greater than zero (M = 0.41, SD = 0.15, t(47) = 18.63, p < .001). Thus, participants showed a bias to choose image 1 and therefore, the 1gtr3 measure is not a pure measure of AWM maintenance (see supplementary materials for additional analyses of B1). Therefore, the interpretation of the analysis pertaining to the 1gtr3 measure (below) is done with caution.
Subsequently, we assessed the correspondence (by computing a within-person correlation) between each participants’ idiographic valence ratings for image 2 and normative IAPS ratings of the images. The mean correlation was high (r = 0.66, p > .001), implying that participants were able to maintain the valence of image 2 accurately.
Simultaneous AWM maintenance
In line with our first aim, we examined participants’ overall idiographic accuracy rates (M = 0.70, SD = 0.11). Accuracy rates were not significantly different (t(47) = −0.55, p = .58) than the average accuracy rate in a similar previous research (studies 1–3; Waugh et al., Citation2019), indicating that participants were able to maintain emotions simultaneously.
The effects of valence and valence congruence on simultaneous emotion maintenance
Idiographic accuracy for 1gtr3 trials was submitted to a 2 (valence of target emotion 1: positive, negative) × 3 (valence of target emotion 2: negative, neutral, positive) repeated measures analysis of variance (ANOVA). The analysis showed a significant main effect for valence of target emotion 1 (F(1,47) = 17.0, p < .001, η2 = .26). In line with our prediction, accuracy for positive emotions (M = .72, SE = .02) was higher than for negative emotions (M = .60, SE = .02). The analysis also revealed a significant main effect for the valence of target emotion 2 (F(2,46) = 6.66, p = .003, η2 = .22). Pairwise comparisons with Bonferroni correction showed that accuracy on trials with negative target emotion 2 (M = 0.71, SE = 0.03) was higher than on trials in which emotion 2 was positive (M = 0.62, SE = 0.03) or neutral (M = 0.64, SE = 0.03). This indicated that negative image 2 may have facilitated maintenance of emotion 1 intensity. Accuracy rates for positive and neutral image 2 were not significantly different from each other. Furthermore, contrary to our congruency hypothesis, the interaction between valence of target emotions 1 and 2 (F(2,46) = 1.45, p = .25) was non-significant. Thus, we concluded that maintenance was not impaired more by simultaneous maintenance of congruent as opposed to incongruent emotions (). Notably, we examined the effects of valence and valence congruence on normed intensity ratings and received largely similar results (see supplementary materials).
Figure 3. Maintenance metrics by valence of target emotion 1 and 2.
Notes: Panel a = Idiographic accuracy in 1gtr3 trials. Panel b = Latent maintenance construct. Dark bars represent negative target emotion 2 and light bars represent positive target emotion 2. Error bars represent 95% confidence intervals.
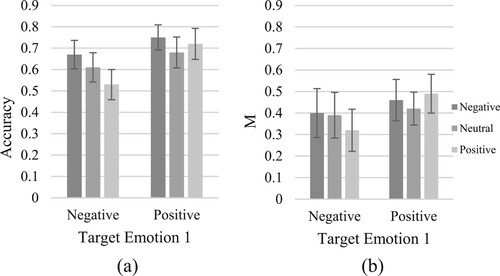
Because of the bias in 1gtr3 trials, we also examined our predictions using the M metric. M was submitted to a 2 (valence of target emotion 1: positive, negative) × 3 (valence of target emotion 2: negative, neutral, positive) repeated measures ANOVA. The results showed a significant main effect for valence of target emotion 1 (F(1,47) = 4.19, p = .046, η2 = .08). In line with our prediction, M for positive emotions (M = .46, SE = .03) was higher than for negative emotions (M = .37, SE = .04). The main effect of valence of target emotion 2 (F(2,46) = .68, p = .509) and the interaction between target emotions 1 and 2 (F(2,46) = 1.16, p = .321) were non-significant. This analysis indicates that people maintained positive emotions better than they did negative emotions, regardless of their bias to choose positive image 1. Importantly, this analysis indicates that maintenance was not impaired more by simultaneous maintenance of congruent as opposed to incongruent emotions ().
In summary, participants were able to maintain emotions simultaneously. Furthermore, in line with our prediction, maintenance of positive emotions was better than that of negative emotions. Contrary to our congruency prediction, maintenance was not impaired more by simultaneous maintenance of congruent as opposed to incongruent emotions. Examining accuracy on 1gtr3 trials, showed that negative emotion 2 resulted in higher accuracy for positive and negative target emotion 1. However, this effect of negative emotion 2 on accuracy in 1gtr3 was not replicated when testing the M metric. Because the 1gtr3 measure is influenced by bias to choose either image 1 or 3, it is unclear what this effect means.
Discussion
The current study examined how individuals maintain more than one emotion in AWM and whether simultaneous maintenance was influenced by valence and valence congruency. Overall, the findings showed that participants were able to successfully maintain two different emotions simultaneously, and that people are better at maintaining positive as compared to negative emotions, regardless of simultaneous maintenance of congruent or incongruent emotions.
Although we did not have a control condition in which participants were asked to maintain only one emotion at a time, a comparison of idiographic accuracy in this study to a previous study with similar metrics (Waugh et al., Citation2019), revealed similar idiographic accuracy levels (∼70%). This suggests that the processing of an additional emotion does not necessarily impair the maintenance process. A possible caveat to the conclusion regarding maintenance of multiple emotions is that participants were requested to maintain the intensity of one emotional stimulus but the valence of the other. It is possible that maintaining valence and intensity of different stimuli is easier than maintaining the same feature of the two separate emotions. Prior research (Vlasenko et al., Citation2021) suggests that people often process intensity and valence in a similar manner. Nonetheless, it is critical that future research establishes whether maintaining intensity and valence are similar in their demands.
Our research contributes to the ongoing exploration of the similarities and differences between AWM and WM, and suggests that maintaining multiple representations in AWM may not impose additional cognitive load. The results of our study align with Mikels et al. (Citation2008) that also showed that AWM is not significantly influenced by cognitive load, and with theory and findings concerning simultaneous processing of stimuli in WM (e.g. Bahle et al., Citation2018; Beck et al., Citation2012). While WM is typically impaired under increased cognitive load (e.g. Lemaire & Portrat, Citation2018; Oberauer et al., Citation2012), this effect is not always observed (De Schrijver & Barrouillet, Citation2017; Ricker & Vergauwe, Citation2020). Similarly, although some research indicates that AWM is affected by cognitive load (e.g. de Voogd et al., Citation2018; Van Dillen et al., Citation2013), our findings suggest the contrary. We observed that individuals can maintain two emotions, including those of congruent valence (which theoretically could impose more cognitive load), without significant difficulty.
The results replicate and strengthen prior findings (Waugh et al., Citation2019), that people are better at maintaining positive than negative emotions. This finding was found regardless of whether the emotions were maintained with congruent or incongruent emotions. We suggest that superior maintenance of positive emotions may be due to people's goals to maintain and increase positive emotions (Eldesouky & English, Citation2019), and the use of regulation strategies such as infusing events with positive meaning and savouring positive emotional feelings in order to maintain positive emotions (Colombo et al., Citation2021).
This was the first study in which we used the latent maintenance construct. The metric provides a more nuanced understanding of the ways in which emotions are maintained in working memory, as it considers people's bias to choose the first or third image as higher. The consistent findings regarding the maintenance superiority of positive compared to negative emotions between maintenance metrics (accuracy in 1gtr3 trials and M) confirm and support the use of the latent construct. This approach may have important implications for future research on AWM, as it allows for a more thorough examination of the maintenance processes involved in emotional responses. Interestingly, the results showed that simultaneous maintenance of negative emotion 2 increased accuracy in 1gtr3 trials for positive and negative emotion 1. However, this effect was not replicated with the M metric. Although it is possible that the effect was not strong enough to be detected with the M analysis, we note that many processes can contribute to this effect (including those involved with processing B1 and emotion 3), and render its meaning unclear.
The ability to maintain and process multiple emotions simultaneously may have evolutionary significance, offering adaptive advantages in social interactions and decision-making (Orzechowski et al., Citation2020). This capacity in AWM may have significant implications for well-being in several ways. For instance, holding in mind two emotions requires cognitive effort that may help the regulation of emotions (Maranges et al., Citation2017). Moreover, maintaining multiple emotions in AWM may enhance cognitive flexibility, by allowing individuals to shift their attention and thinking patterns between different emotional states (Schweizer et al., Citation2019). This flexibility can promote creativity, problem-solving, and adaptive decision-making by enabling individuals to consider multiple perspectives and solutions (Miyake et al., Citation2000). Frank et al. (Citation2021) further underscored the unique role of AWM in emotional processes. Their research highlighted a selective relationship between AWM and affective forecasting, the ability to predict future emotional states, pivotal for decision-making. Given these findings, there is a compelling case for continued exploration into the complex relationships among shifting, emotion regulation, cognitive load, and AWM.
Several limitations of the current research should be noted. First, performance on the task may be influenced by the moment-to-moment cognitive demands that differ by trial characteristics (Jha & Kiyonaga, Citation2010; Witkin et al., Citation2020). Because the task was not designed to investigate this phenomenon, potential carry-over effects could not be examined in the current study and await further exploration. Second, when judging the valence of the second image, participants might have opted for a simple cognitive strategy: categorising the valence (to positive, negative or neutral) and recalling that category post-delay. Consequently, rather than preserving the valence of the second image, participants might have recalled the broadly identified valence category – a process potentially rooted more in verbal or cognitive recall than in emotional maintenance. Although we demonstrated the accuracy of image 2 processing using a comparison to normed ratings, fully discerning the exact cognitive processes at play during the maintenance period remains challenging. Third, our findings resonate with previous research that suggests that emotional responses can occur both simultaneously and sequentially (Berrios et al., Citation2015), but it did not directly compare simultaneous vs. sequential AWM performance, leaving this exploration to future endeavours. Notably, Mikels et al. (Citation2008) explored AWM performance when two images were presented simultaneously and observed no differences in valence. Lastly, this study was conducted online, which may have introduced variability in the way participants completed the task. Future research could address this limitation by using different administration modalities to assess AWM.
In conclusion, the current study provides important insights into the complexity of AWM when processing multiple emotions. The modified maintenance task and novel metric offer useful tools for assessing AWM and understanding the processes involved in maintaining emotional responses. Our findings have important implications for understanding emotions and may help inform interventions to improve emotional well-being. Future research could build on these findings to develop more targeted interventions to improve emotional maintenance and well-being.
Ethics approval
Ethics approval was obtained from the School of Education Ethics Committee, Hebrew University of Jerusalem (2022Y2402).
Consent to participate
Informed consent was collected from all participants.
Supplemental Material
Download MS Word (19.5 KB)Disclosure statement
No potential conflict of interest was reported by the author(s).
Data availability statement
Data and materials can be accessed at https://doi.org/10.17605/OSF.IO/CFS5R
.Additional information
Funding
Notes
1 Outlier cutoff was based on interquartile range method, which defines outliers as any data which lies beyond 2.7σ from the mean. According to this method the lower bound for outliers is: Q1 − 1.5 * IQR (Q3 − Q1) and the upper bound is: Q3 + 1.5 * IQR.
2 The post task ratings consisted of intensity ratings and not valence, therefore we could not use them to ascertain accuracy of image 2’s valence.
References
- Baddeley, A. (2003). Working memory: Looking back and looking forward. Nature Reviews Neuroscience, 4(10), 829–839. https://doi.org/10.1038/nrn1201
- Baddeley, A. (2021). Developing the concept of working memory: The role of neuropsychology. Archives of Clinical Neuropsychology, 36(6), 861–873. https://doi.org/10.1093/arclin/acab060
- Bahle, B., Beck, V. M., & Hollingworth, A. (2018). The architecture of interaction between visual working memory and visual attention. Journal of Experimental Psychology: Human Perception and Performance, 44(7), 992. https://doi.org/10.1037/xhp0000509
- Batchelder, W. H., & Riefer, D. M. (1999). Theoretical and empirical review of multinomial process tree modeling. Psychonomic Bulletin & Review, 6(1), 57–86. https://doi.org/10.3758/BF03210812
- Baumeister, R. F., Bratslavsky, E., Finkenauer, C., & Vohs, K. D. (2001). Bad is stronger than good. Review of General Psychology, 5(4), 323–370. https://doi.org/10.1037/1089-2680.5.4.323
- Beck, V. M., Hollingworth, A., & Luck, S. J. (2012). Simultaneous control of attention by multiple working memory representations. Psychological Science, 23(8), 887–898. https://doi.org/10.1177/0956797612439068
- Berrios, R., Totterdell, P., & Kellett, S. (2015). Eliciting mixed emotions: A meta-analysis comparing models, types and measures. Frontiers in Psychology, 6, 428 . https://doi.org/10.3389/fpsyg.2015.00428.
- Brady, T. F., & Störmer, V. S. (2022). The role of meaning in visual working memory: Real-world objects, but not simple features, benefit from deeper processing. Journal of Experimental Psychology: Learning, Memory, and Cognition, 48(7), 942. https://doi.org/10.1037/xlm0001014
- Cacioppo, J. T., Berntson, G. G., Norris, C. J., & Gollan, J. K. (2011). The evaluative space model. In P. A. M. Van Lange, A. W. Kruglanski, & E. T. Higgins (Eds.), Handbook of theories of social psychology (Vol. 1, pp. 50–72). Thousand Oaks, CA: Sage Press.
- Christophel, T. B., Klink, P. C., Spitzer, B., Roelfsema, P. R., & Haynes, J.-D. (2017). The distributed nature of working memory. Trends in Cognitive Sciences, 21(2), 111–124. https://doi.org/10.1016/j.tics.2016.12.007
- Colombo, D., Pavani, J.-B., Fernandez-Alvarez, J., Garcia-Palacios, A., & Botella, C. (2021). Savoring the present: The reciprocal influence between positive emotions and positive emotion regulation in everyday life. PLoS One, 16(5), e0251561. https://doi.org/10.1371/journal.pone.0251561
- Cowan, N. (2017). The many faces of working memory and short-term storage. Psychonomic Bulletin & Review, 24(4), 1158–1170. https://doi.org/10.3758/s13423-016-1191-6
- De Schrijver, S., & Barrouillet, P. (2017). Consolidation and restoration of memory traces in working memory. Psychonomic Bulletin & Review, 24(5), 1651–1657. https://doi.org/10.3758/s13423-017-1226-7
- de Voogd, L. D., Hermans, E. J., & Phelps, E. A. (2018). Regulating defensive survival circuits through cognitive demand via large-scale network reorganization. Current Opinion in Behavioral Sciences, 24, 124–129. https://doi.org/10.1016/j.cobeha.2018.08.009
- Eldesouky, L., & English, T. (2019). Regulating for a reason: Emotion regulation goals are linked to spontaneous strategy use. Journal of Personality, 87(5), 948–961. https://doi.org/10.1111/jopy.12447
- Fan, L., Diao, L., Xu, M., & Zhang, X. (2023). Multiple representations in visual working memory can simultaneously guide attention. Current Psychology, 42(26), 22320–22327. https://doi.org/10.1007/s12144-022-03332-3
- Faul, F., Erdfelder, E., Lang, A.-G., & Buchner, A. (2007). G* Power 3: A flexible statistical power analysis program for the social, behavioral, and biomedical sciences. Behavior Research Methods, 39(2), 175–191. https://doi.org/10.3758/BF03193146
- Frank, C. C., Iordan, A. D., Ballouz, T. L., Mikels, J. A., & Reuter-Lorenz, P. A. (2021). Affective forecasting: A selective relationship with working memory for emotion. Journal of Experimental Psychology: General, 150(1), 67–82. https://doi.org/10.1037/xge0000780
- Frank, C. C., Iordan, A. D., & Reuter-Lorenz, P. A. (2023). Biden or Trump? Working memory for emotion predicts the ability to forecast future feelings. Emotion, 24(1), 139–149. https://doi.org/10.1037/emo0001258
- Gard, D. E., Cooper, S., Fisher, M., Genevsky, A., Mikels, J. A., & Vinogradov, S. (2011). Evidence for an emotion maintenance deficit in schizophrenia. Psychiatry Research, 187(1–2), 24–29. https://doi.org/10.1016/j.psychres.2010.12.018
- Gruber, J., Purcell, A. L., Perna, M. J., & Mikels, J. A. (2013). Letting go of the bad: Deficit in maintaining negative, but not positive, emotion in bipolar disorder. Emotion, 13(1), 168–175. https://doi.org/10.1037/a0029381
- Hur, J., Iordan, A. D., Dolcos, F., & Berenbaum, H. (2017). Emotional influences on perception and working memory. Cognition and Emotion, 31(6), 1294–1302. https://doi.org/10.1080/02699931.2016.1213703
- Jha, A. P., & Kiyonaga, A. (2010). Working-memory-triggered dynamic adjustments in cognitive control. Journal of Experimental Psychology: Learning, Memory, and Cognition, 36(4), 1036. https://doi.org/10.1037/a0019337
- Lang, P. J., Bradley, M. M., & Cuthbert, B. N. (1999). International affective picture system (IAPS): Instruction manual and affective ratings. University of Florida.
- Laurikkala, J., Juhola, M., Kentala, E., Lavrac, N., Miksch, S., & Kavsek, B. (2000). Informal identification of outliers in medical data. Fifth International Workshop on Intelligent Data Analysis in Medicine and Pharmacology, Vol. 1, pp. 20–24.
- Lemaire, B., & Portrat, S. (2018). A computational model of working memory integrating time-based decay and interference. Frontiers in Psychology, 9, 416. https://doi.org/10.3389/fpsyg.2018.00416
- LeMoult, J., & Gotlib, I. H. (2019). Depression: A cognitive perspective. Clinical Psychology Review, 69, 51–66. https://doi.org/10.1016/j.cpr.2018.06.008
- Lewis-Peacock, J. A., Drysdale, A. T., Oberauer, K., & Postle, B. R. (2012). Neural evidence for a distinction between short-term memory and the focus of attention. Journal of Cognitive Neuroscience, 24(1), 61–79. https://doi.org/10.1162/jocn_a_00140
- Maranges, H. M., Schmeichel, B. J., & Baumeister, R. F. (2017). Comparing cognitive load and self-regulatory depletion: Effects on emotions and cognitions. Learning and Instruction, 51, 74–84. https://doi.org/10.1016/j.learninstruc.2016.10.010
- Mather, M. (2009). When emotion intensifies memory interference. Psychology of Learning and Motivation, 51, 101–120. https://doi.org/10.1016/S0079-7421(09)51003-1
- Mikels, J. A., Larkin, G. R., Reuter-Lorenz, P. A., & Carstensen, L. L. (2005). Divergent trajectories in the aging mind: Changes in working memory for affective versus visual information with age. Psychology and Aging, 20(4), 542. https://doi.org/10.1037/0882-7974.20.4.542
- Mikels, J. A., & Reuter-Lorenz, P. A. (2019). Affective working memory: An integrative psychological construct. Perspectives on Psychological Science, 14(4), 543–559. https://doi.org/10.1177/1745691619837597
- Mikels, J. A., Reuter-Lorenz, P. A., Beyer, J. A., & Fredrickson, B. L. (2008). Emotion and working memory: Evidence for domain-specific processes for affective maintenance. Emotion, 8(2), 256–266. https://doi.org/10.1037/1528-3542.8.2.256
- Miller, E. K., Lundqvist, M., & Bastos, A. M. (2018). Working memory 2.0. Neuron, 100(2), 463–475. https://doi.org/10.1016/j.neuron.2018.09.023
- Miyake, A., Friedman, N. P., Emerson, M. J., Witzki, A. H., Howerter, A., & Wager, T. D. (2000). The unity and diversity of executive functions and their contributions to complex “frontal lobe” tasks: A latent variable analysis. Cognitive Psychology, 41(1), 49–100. https://doi.org/10.1006/cogp.1999.0734
- Norris, C. J., Gollan, J., Berntson, G. G., & Cacioppo, J. T. (2010). The current status of research on the structure of evaluative space. Biological Psychology, 84(3), 422–436. https://doi.org/10.1016/j.biopsycho.2010.03.011
- Oberauer, K., Lewandowsky, S., Farrell, S., Jarrold, C., & Greaves, M. (2012). Modeling working memory: An interference model of complex span. Psychonomic Bulletin & Review, 19(5), 779–819. https://doi.org/10.3758/s13423-012-0272-4
- Oberauer, K., Süß, H.-M., Schulze, R., Wilhelm, O., & Wittmann, W. W. (2000). Working memory capacity – facets of a cognitive ability construct. Personality and Individual Differences, 29(6), 1017–1045. https://doi.org/10.1016/S0191-8869(99)00251-2
- Orzechowski, J., Śmieja, M., Lewczuk, K., & Nęcka, E. (2020). Working memory updating of emotional stimuli predicts emotional intelligence in females. Scientific Reports, 10(1), 20875. https://doi.org/10.1038/s41598-020-77944-9
- Ricker, T. J., & Vergauwe, E. (2020). Consistent failure to produce a cognitive load effect in visual working memory using a standard dual-task procedure. Journal of Cognition, 3(1), 15. https://doi.org/10.5334/joc.108
- Schweizer, S., Satpute, A. B., Atzil, S., Field, A. P., Hitchcock, C., Black, M., Barrett, L. F., & Dalgleish, T. (2019). The impact of affective information on working memory: A pair of meta-analytic reviews of behavioral and neuroimaging evidence. Psychological Bulletin, 145(6), 566–609. https://doi.org/10.1037/bul0000193
- Seligman, M. (2018). PERMA and the building blocks of well-being. The Journal of Positive Psychology, 13(4), 333–335. https://doi.org/10.1080/17439760.2018.1437466
- Smiti, A.. (2020). A critical overview of outlier detection methods. Computer science review, 38, 100306. https://doi.org/10.1016/j.cosrev.2020.100306..
- Sutterer, D. W., Foster, J. J., Adam, K. C. S., Vogel, E. K., & Awh, E. (2019). Item-specific delay activity demonstrates concurrent storage of multiple active neural representations in working memory. PLoS Biology, 17(4), e3000239. https://doi.org/10.1371/journal.pbio.3000239
- Tamir, M., & Millgram, Y. (2017). Motivated emotion regulation. In A. J. Elliot (Ed.), Advances in motivation science (Vol. 4, pp. 207–247). Cambridge, MA: Elsevier Ltd.
- van Dillen, L. F., & Hofmann, W. (2023). Room for feelings: A “working memory” account of affective processing. Emotion Review, 15(2), 145–157https://doi.org/10.1177/17540739221150233.
- Van Dillen, L. F., Papies, E. K., & Hofmann, W. (2013). Turning a blind eye to temptation: how cognitive load can facilitate self-regulation. Journal of Personality and Social Psychology, 104(3), 427–443 https://doi.org/10.1037/a0031262.
- Vlasenko, V. V., Rogers, E. G., & Waugh, C. E. (2021). Affect labelling increases the intensity of positive emotions. Cognition and Emotion, 35(7), 1350–1364. https://doi.org/10.1080/02699931.2021.1959302
- Waugh, C. E., Lemus, M. G., & Gotlib, I. H. (2014). The role of the medial frontal cortex in the maintenance of emotional states. Social Cognitive and Affective Neuroscience, 9(12), 2001–2009. https://doi.org/10.1093/scan/nsu011
- Waugh, C. E., Running, K. E., Reynolds, O. C., & Gotlib, I. H. (2019). People are better at maintaining positive than negative emotional states. Emotion, 19(1), 132–145. https://doi.org/10.1037/emo0000430
- Witkin, J. E., Zanesco, A. P., Denkova, E., & Jha, A. P. (2020). Dynamic adjustments in working memory in the face of affective interference. Memory & Cognition, 48(1), 16–31. https://doi.org/10.3758/s13421-019-00958-w