ABSTRACT
Sewage treatment plant (STP) effluent has been used as ecological supplementary water in dry and polluted urban rivers. However, more consideration should be given to the impact of STP effluent on water quality and the environment. In this paper, a typical urban tributary river of Dianchi was selected to study the impact of STP effluent on water quality and plankton communities. Using a paired-sample t-test, factor analysis and canonical correspondence analysis, changes in water quality, plankton communities and their relationships were analyzed. The results showed that STP effluent decreased the concentrations of suspended solid, chemical oxygen demand, five-day biological oxygen demand (BOD5) and total phosphorus. However, it significantly increased the concentration of nitrate, total nitrogen and nitrate nitrogen (NO3-N). A distinct shift in the phytoplankton community from Chlorophyta (green algae) to Bacillariophyta (diatoms) occurred after the influx of STP effluent and further analysis indicated that this shift was primarily caused by the increase in NO3-N and facilitated by the seasonal rise in water temperature. Zooplankton communities were less sensitive to changes in water pollution condition than phytoplankton communities and the zooplankton community structure of the Chuanfang River was mainly influenced by water temperature.
Introduction
Dianchi Lake (24°51′N, 102°42′E) is a well-known shallow plateau lake located in Kunming, Yunnan province in the south-west of China. With the rapid expansion of urban land use and the lack of sewage treatment systems, large amounts of sanitary sewage were carried into Dianchi Lake via the inflowing rivers. This process caused eutrophication and a rapid deterioration in water quality in Dianchi Lake (Huang et al. Citation2014; Li et al. Citation2014). To reduce the pollution in Dianchi Lake, the Kunming city government has invested tens of billions of Chinese Yuan to promote six types of pollution control projects in the Dianchi Lake basin: (1) a lakeside sewage interception and transportation system construction project, (2) a water diversion and water conservation project, (3) an inflowing river regulation project, (4) an agricultural and rural non-point source management project, (5) an ecological restoration and construction project and (6) an ecological dredging project. Among the six projects, the inflowing river regulation project plays a very important role because inflowing river water is the main contributor of exogenous pollution in Dianchi Lake. Statistically, Dianchi Lake received an average of 72%, 78% and 80% of its chemical oxygen demand (COD), total phosphorus (TP) and total nitrogen (TN), respectively, from 35 major inflowing rivers (KEPB Citation2011). Although the sewage interception of the rivers effectively prevented point source pollution, it also decreased the river flow, dramatically changing the hydrological conditions of the urban rivers due to an insufficient natural water supply (Gren Citation2008).
To cope with the dilemma of water shortage and water pollution in urban rivers, in 2009, the Kunming city government began to recharge the urban rivers with sewage treatment plant (STP) effluent. STP effluent can meet the Class 1A standard stipulated by the Chinese ‘Discharge Standard of Pollutants for Municipal Wastewater Treatment Plants (GB 18918-2002)’ (e.g. COD ≤ 50 mg/L, TP ≤ 0.5 mg/L, ammonia nitrogen (NH3-N) ≤ 5 mg/L and TN ≤ 15 mg/L). From the perspective of landscape values, the reuse of STP effluent created significant social benefits, as the malodorous black river water was largely replaced by the ‘relatively cleaner water’ obtained from the advanced treatment (coagulation-filtration and disinfection) of secondarily treated effluent (via chemical, physical and biological treatment methods; Sun et al. Citation2013). With regard to the protection of Dianchi Lake, the main focus was to reduce the pollutants in the inflowing rivers. However, the effects of the influx of reused STP effluent on the water environment and the ecological components of the ‘recharged urban rivers’ remained unknown.
To identify the impact of STP effluent on the urban river eco-environment, a typical urban river, the Chuanfang River in the Dianchi Lake basin, was studied. A large proportion of the river water came from the reused STP effluent (Li et al. Citation2012). Using a series of statistical methods, including correlation analysis, a paired-sample t-test, factor analysis and canonical correspondence analysis (CCA), the overall changes in water quality and plankton communities in the Chuanfang River and the reasons for these changes, as well as the relationship between water quality and plankton community structure in different seasons, were analyzed and discussed.
Methods
Study area
The Chuanfang River, located in Xishan District of Kunming City, is a typical urban tributary river of Dianchi Lake. To mitigate pollution in the Chuanfang River, a river regulation project was conducted between 2006 and 2010 to intercept and divert the wastewater from the upper reaches to the First Sewage Treatment Plant of Kunming. The wastewater was treated with an oxidation ditch, a biological filter and UV disinfection reach the Class 1A standard of GB 18918-2002. The wastewater was discharged into the lower reach of the Chuanfang River, a 5.4-km-long open channel that was also restored in the regulation project (). To investigate the water quality and plankton species of the Chuanfang River, five sampling points, S0, S1, S2, S3 and S4, were established along the river approximately 1 km apart. Among the five sampling points, S0 is hardly affected by the influx of STP effluent because it is approximately 400 m upstream of the recharging point. The water environments of S1, S2, S3 and S4 are all influenced by the STP effluent, as the STP serves as a constant water source of the Chuanfang River.
The water quality was monitored monthly from September 2013 to August 2014, and the planktonic species were investigated in October 2013, December 2013, March 2014 and July 2014. The water samples were collected at a depth of 0.3–0.5 m below the water surface using an organic glass sampler. Water temperature (WT), dissolved oxygen (DO) and pH were measured in situ via a multi-parameter meter (ARDREL/2800, Hach Company, USA; http://www.hach.com). The samples were then stored at 4 °C in a polyethylene bottle rinsed with the same sampling water. Suspended solid (SS, Gravimetric method, GB/T 11901-1989), COD (Potassium dichromate titration method, GB 11914-1989), BOD5 (Dilution and seeding method, HJ 505-2009), NH3-N (Nessler's reagent spectrophotometry, HJ 535-2009), NO3-N (UV spectrophotometry, HJ/T 346-2007), nitrite nitrogen (NO2-N, N-(1-Naphthyl) ethylenediamine dihydrochloride spectrophotometry, GB 7493-1987), TN (Potassium persulfate digestion UV spectrophotometry, HJ 636-2012) and TP (Ammonium molybdate spectrophotometry, GB/T 11893-1989) were measured according to Chinese standard methods.
To count cladocerans and copepods in the zooplankton, a 5000-mL sample was filtered through a 10-μm mesh, and the filters were stored frozen with 4% buffered formalin. The 1000 mL samples of phytoplankton and zooplankton were preserved with 1.5% Lugol's iodine solution on site and then precipitated for 48 h to concentrate the volume to 5%. The zooplankton were identified and quantified following the methods proposed by Shen et al. (Citation1979) and Hu and Wei (Citation2006). The phytoplankton were identified and counted in a hemocytometer under an automated microscope using a method mainly based on the work of Hu and Wei (Citation2006). Phytoplankton species were identified to the lowest taxonomic level possible (species or genus level), and taxa abundances, including colonial and filamentous forms, were quantified by counting single cells.
Statistical analysis
A Pearson correlation analysis was performed to explain the changes in water quality parameters before and after the influx of STP effluent. A paired-sample t-test was used to explore the difference in water quality between S1 and the other four sampling sites (i.e. S0, S2, S3 and S4; Mustapha et al. Citation2012). The paired-sample t-test eliminated the influence of seasons and enabled us to focus on spatial changes in the river water quality. The null hypothesis was that the paired samples were not significantly different. Meanwhile, Pearson correlation coefficients of the paired samples were also calculated to determine the spatial associations of water quality parameters between S1 and the other four sampling sites.
To elucidate the effects of STP effluent and sewage on the plankton species of the Chuanfang River, a factor analysis technique was adopted to process the selected simultaneously monitored water quality parameters (Ghorbani-Aghdam et al. Citation2013; Sulthana et al. Citation2014). Before the factor analysis, the selected water quality parameters, except pH, were log(x + 1) transformed to reduce skewed distributions. The standard scores of the main factors (eigenvalue >1) were calculated and then used as the environmental variables of phytoplankton and zooplankton assemblages in a CCA. WT was also used as an individual environmental variable and was log(x + 1) transformed and standardized. To simplify the analysis and reduce the interference of rare species, the phytoplankton in a CCA were categorized by genus, and only the dominant genera were included. For phytoplankton, the dominant taxon (genus) was defined based on the following two rules: (1) the relative abundance of the taxon was more than 10% in at least one sample, and (2) the taxon was identified in at least four samples. For zooplankton, the dominant taxon was defined as having an occurrence frequency of no less than 25% (i.e. 5 out of 20 samples). Species data were square-root transformed and the significance of the first canonical axis and all canonical axes was evaluated using a Monte Carlo permutation test (999 permutations). The paired-samples t-test, Pearson correlation analysis and factor analysis were performed using the SPSS 19.0 package, while CCA was performed using CANOCO 4.5 (TerBraak et al. Citation2002).
Results and discussions
Analysis of water quality
The values of the water quality parameters of the Chuanfang River are shown in (a,b). The subtropical monsoon climate prevails in the Dianchi Lake basin, and the WT of the Chuanfang River, which ranged from 8 °C in December 2013 to 22.7 °C in July 2014 during the monitoring period, was consistent with the features of the local climate (Huang et al. Citation2014; Wu et al. Citation2014). Monthly changes in WT at the five sampling sites were generally similar. There were eight months during the monitoring period when the WT at S1 was higher than at S0, with an apparent difference of as much as 3.5 °C in December 2013. In contrast, in March 2014, when the STP effluent was pumped to S0, the WT at S0 became higher than at S1. These phenomena were thought to be caused by the influx of STP effluent at S0. The temperature differences may be related to the type of treatment, such as using an oxidation ditch and UV disinfection. Generally, the pH values ranged from 7 to 9, but the changes did not show any regular pattern with the seasons or spatial factors. The pH of water in the Chuanfang River could be influenced by many factors, such as acids and alkalis, photosynthesis and respiration.
Figure 2. (a) Water quality parameters of Chuanfang River during the monitoring period, September 2013 to August 2014. March is marked with a circle because in this month, the STP effluent was pumped to the S0 to clean the river channel, making the data at S0 very different from the other months. Water temperature (WT), dissolved oxygen (DO), suspension solid (SS), five-day biological oxygen demand (BOD5) and chemical oxygen demand (COD). (b) Water quality parameters of Chuanfang River during the monitoring period, September 2013 to August 2014. March is marked with a circle because in this month, the STP effluent was pumped to the S0 to clean the river channel, making the data at S0 very different from the other months. Ammonia nitrogen (NH3-N), nitrite nitrogen (NO2-N), nitrate nitrogen (NO3-N), total nitrogen (TN) and total phosphorus (TP).
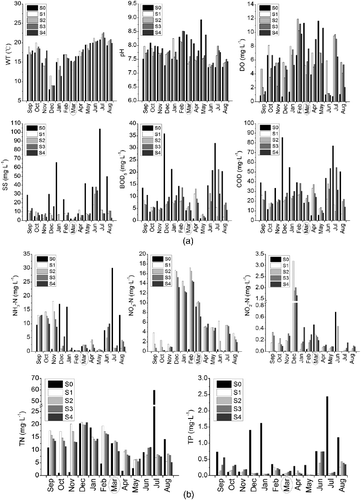
The concentrations of DO varied considerably, ranging from 0.7 to 12 mg/L. The concentration of DO at S1 was relatively high throughout the entire monitoring process (from 4.72 to 12 mg/L), while at S2, S3 and S4, the minimum concentration of DO was only approximately 1 mg/L. However, cultivated submerged plants (which were abundant in February 2014) and green algae (mainly observed at S0 and S4, where the water was relatively stagnant) were commonly found in the Chuanfang River during the monitoring period. The oxygen produced by the photosynthesis of these plants or micro-plants was an important component of DO in the water of the Chuanfang River, which could largely explain why the river water was oversaturated with DO in certain months.
The concentration of suspended solid (SS) at S1 was relatively low, ranging from 1 to 18 mg/L, and its mean value was 8.7 mg/L. This result indicated that the removal rate of SS in the First STP of Kunming was satisfactory. However, the concentration of SS at S0 ranged widely, from 5 to 104 mg/L. The water at S0 was mainly from the overflow of upstream wastewater, where the flow condition was poor. Therefore, we assumed that the polluted water at S0 could be substantially purified by self-purification over several months until the next overflow event. This assumption could explain the fact that the water pollution at S0 was mitigated before the rainy season, whereas it was suddenly aggravated in June when the rainy season was just beginning.
As indicators of organic pollution, BOD5 and COD showed similar changes. The concentrations of BOD5 and COD were lower at S1, ranging from 1.55 to 11.08 mg/L and from 6.59 to 33.27 mg/L, respectively, than at all other sampling points. In addition, the spatial changes in the concentrations of BOD5 and COD after the influx of STP effluent were moderate in most of the months, which could be explained by variation in the amount of inflowing sewage (particularly the storm sewage) into the Chuanfang River in addition to other unknown sources.
Unlike SS, BOD5 or COD, the concentrations of NH3-N and NO3−-N at S1 changed greatly from month to month, ranging from 0.248 to 18.11 mg N/L and from 1.511 to 17.24 mg N/L, respectively. Moreover, there was a significant negative correlation between NH3-N and NO3-N (p < 0.05), indicating the instability of the nitrification process in the First STP of Kunming. Moreover, while the concentration of NO3−-N at S0 was very low (less than 0.5 mg N/L), it increased significantly after the influx of STP effluent. This result indicated that the STP effluent was the main source of NO3-N in the Chuanfang River (Roach and Grimm Citation2009). The concentration of TN remained high after the influx of STP effluent, with mean values over 10 mg N/L at S1, S2, S3 and S4. Fundamentally, the nitrification process of the STP only changed the species of nitrogen but did not remove the nitrogen from the water (Kelso et al. Citation1997). Throughout the monitoring period, the concentration of TP at S1 remained relatively low, ranging from 0.040 to 0.256 mg/L, and the mean value was only 0.1 mg/L, which indicated the high phosphorus removal rate of the First STP of Kunming. However, in most of the months, the concentration of TP in the lower river course showed a clear increase, which indicated increased pollution levels in the Chuanfang River.
The results of the paired-samples t-test () showed that there were significant differences between S1 and S0 in the water quality parameters of DO, SS, COD, BOD5, NO3-N and TP after the influx of STP effluent (p < 0.05). The correlation coefficients () also showed that all the water quality parameters except WT had no significant correlations between S0 and S1 (p > 0.05). Both analyses indicated the strong impact of the influx of STP effluent. There were significant positive correlations between S1 and all the three downstream sampling sites (S2, S3 or S4) for SS, NH3-N, NO3-N, NO2-N and TN. However, with increasing distance from S1 to the lower reach, the correlations between S1 and S4 for the other parameters (pH, DO, COD, BOD5 and TP) were not significant (p < 0.05). This result indicated that the downstream concentrations of SS and nitrogen species in the Chuanfang River were mainly determined by the influx of STP effluent. More interestingly, the concentration of NO3-N was significantly different between S1 and all the downstream sampling sites, although the difference was relatively small. This result could be attributed to the denitrification process in the Chuanfang River (p < 0.05) (Newcomer Citation2012; Chen Citation2015).
Table 1. The paired-samples t-test and correlation coefficients of water quality indicators.
A total of 120 phytoplankton taxa, belonging to 52 genera and five classes, were identified and quantified (). At S0, Chlorophyta were dominant in autumn, winter and summer, with high relative abundances. However, after the influx of STP effluent, the relative abundance of Chlorophyta noticeably decreased. Meanwhile, the relative abundance of Bacillariophyta increased substantially in autumn, winter and summer. A closer comparison revealed that the main Chlorophyta genera involved in these community transitions were Pediastrum and Scenedesmus, while the main Bacillariophyta genera were Cyclotella, Fragilaria and Melosira. In spring, when the STP effluent was pumped to S0, the total phytoplankton abundances at all five monitoring sites along the Chuanfang River were almost the same, ranging from 3.5 × 105 to 4.5 × 105 cells·L−1, and the distributions of phytoplankton phyla were also very similar. Cryptophyta were observed only in autumn, and its maximum relative abundance was only 15.4% at S4.
A total of 79 zooplankton taxa belonging to four groups were identified and quantified ((b)). There were some differences in the compositions of zooplankton collected at S0 and the other four sampling sites. In winter, Cladocera were identified at S1, S2, S3 and S4 but not at S0. Only in the spring, when the STP effluent was pumped to S0, could Cladocera be found in large quantities. The abundance of Protozoa and Rotifera was relatively high and stable in spring and summer; the average density of Protozoa was 1876 ind./L in spring and 2585 ind./L in summer, while the average density of Rotifera was 240 ind./L in spring and 300 ind./L in summer. Few Cladocera could be found in the Chuanfang River, and their density ranged from 0 to 6.4 ind./L. With an average density of 10.2 ind./L, Copepoda were easier to find in winter, while in the other seasons, the average density was less than 2 ind./L.
Relationships between water quality and the dominant plankton species
The main pollution sources of the Chuanfang River were well characterized by most of the water quality parameters. Therefore, we can deduce that the shift in plankton species structure could also reflect the influence of the main pollution sources. The water quality controlling factors were obtained from the factor analysis of the eight selected water quality parameters: pH, DO, SS, BOD5, NH3-N, NO3-N, NO2-N and TP. These parameters were monitored at the same time as the plankton survey. WT was excluded from the factor analysis because it mainly depended on the seasons, while COD and TN were excluded to reduce redundant information (i.e. organic pollution and nitrogen pollution were adequately characterized by BOD5 and the nitrogen species, respectively). In the factor analysis, the KMO (Kaiser–Meyer–Olkin) measure of sampling adequacy was 0.649, and the result of Bartlett's sphericity test was statistically significant (p < 0.001). Two factors (components), F1 and F2 (eigenvalue >1), were extracted and could explain 75.1% of the total variance in the data matrix ((a)), indicating that F1 and F2 were the controlling factors of the eight selected water quality parameters. The factor loadings showed that DO, BOD5, TP, and pH were well explained by F1, with the absolute values of factor loadings on F1 over 0.8. NO3-N and NO2-N were mainly determined by F2, with high loadings on F2 but relatively low loadings on F1. Based on the results in analysis of water quality on the spatial and temporal variations of the water quality parameters, which were consistent with the inflow of sewage (in the upper reach of in the Chuanfang River), F1 was labeled ‘Sewage Pollution Level (SPL).’ Similarly, F2 could be named ‘Nitrate & Nitrite Pollution Level (NPL),’ primarily caused by the nitrification process of the STP. The standard scores of SPL (F1) and NPL (F2) were calculated, and the results are shown in (b). The scores were the quantitative estimations of SPL and NPL. A high score indicated a strong influence of a certain factor on the water quality at a sampling site (Honti Citation2010).
Figure 4. Factor analysis of water quality and the dominant plankton species. (a) Plot of factor loadings after rotation. (b) Standard scores of the extracted factors by regression method. The extraction method is principal component analysis. The rotation method is Varimax with Kaiser normalization.
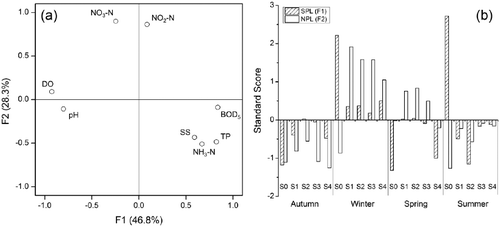
The scores of SPL, NPL and WT were used as the environmental variables in a CCA, and their influences on the dominant phytoplankton genera and zooplankton taxa were analyzed. There were 14 dominant phytoplankton genera, with a maximum relative abundance above 10% and an occurrence frequency approximately 20%, and their abundances were included as species data in a CCA. In terms of zooplankton, total of 26 taxa had an occurrence frequency of approximately 25%. To obtain the final CCA model, the explanatory powers of different environmental variables and their combinations were explored by changing the input environmental data (). Based on the results in , WT had the strongest influence on the plankton community structure (p < 0.01), which could individually explain 15.2% of the variance in the dominant phytoplankton genera and 17.0% of the variance in the dominant zooplankton taxa. Although SPL and NPL both provided considerable explanation (>8%) of the dominant phytoplankton genera, the first canonical axis only became significant (p <0.05) when WT was included in the CCA. This result verified that the influence of WT on the dominant phytoplankton genera was more statistically significant and foundational, while SPL and NPL mainly explained the remaining variance that could not be explained by WT (p < 0.01). Consequently, WT, SPL and NPL were all included as environmental variables in the final CCA model to explain the phytoplankton community structure. All the three canonical axes could explain 33.2% of the total variance in the phytoplankton genera, and the first two canonical axes could explain 27.6%. Regarding dominant zooplankton taxa, NPL alone could explain 8.3% of the total variance. Therefore, in the final CCA model of zooplankton, only WT and NPL were included as environmental variables, and they explained 22.3% of the total variance in the dominant zooplankton taxa. It is worth noting that in the phytoplankton group, the explanatory power (27.0%) of the combination between WT and NPL (WT&NPL) was greater than the sum (24.1%) of explanatory power of WT (15.2%) and NPL (8.9%, WT + NPL), indicating that NPL could effectively explain the variance in phytoplankton that could not be explained by WT.
Table 2. Explanatory power of different environmental variables in canonical correspondence analysis.
The ordination biplot of the dominant phytoplankton genera and environmental variables is shown in (a). The weighted correlation coefficient (weighted r) between WT and Axis 1 was −0.827 (p < 0.01), indicating that the phytoplankton communities in the Chuanfang River were significantly influenced by WT. The weighted correlation coefficient between NPL and Axis 2 was 0.923 (p < 0.01), indicating that NPL could adequately explain the variance that was not included in Axis 1. The CCA model of zooplankton illustrated that WT exhibited a strong relationship with Axis 1 (weighted r = −0.990, p < 0.01; (b)), while NPL was mainly related to Axis 2 (weighted r = −0.857, p < 0.01). However, the inclusion of NPL only increased the explanation of the total variance by 5.3% (22.3%–17.0%), whereas NPL alone could explain 8.3%. This result indicated that a considerable amount of useful information regarding the influence of NPL on the dominant zooplankton taxa was actually included in WT.
The transition mechanisms of plankton community structure
Phytoplankton and zooplankton are two basic components of a water ecosystem, and they are sensitive to changes in the physical environmental conditions (Roach and Crimm Citation2009; Lázár et al. Citation2012; Tornés et al. Citation2014; Zębek Citation2015). Therefore, the homogeneity of phytoplankton and zooplankton caused by the water flow can greatly interfere with the analysis of the influence of water pollution on the plankton community structure in a river. In the case of the Chuanfang River, the compositions of plankton species or taxa at sampling sites S1–S4 were generally similar in each season. In spring, when the STP effluent was pumped to S0, the similarity among all the five sampling sites was enhanced. This homogeneity caused by the water flow may have weakened the influence of variation in water quality on the plankton communities in the river, which may explain why the SPL explained very little of the variance in the dominant zooplankton taxa (Zhao et al. Citation2012).
Many studies show that WT can significantly influence plankton community structure (Huertas et al. Citation2011; Mutshinda et al. Citation2013; Brito et al. Citation2015; Li et al. Citation2015; Teittinen et al. Citation2015; Zębek Citation2015). In this study, WT had a relatively large range (from 8 to 22.7 °C) because the four plankton surveys were conducted once in each of the four seasons of the year. Therefore, the seasonal transitions of the plankton community structure in the Chuanfang River can be largely attributed to the variation in WT in the CCA (). However, the results of the CCA also showed that the joint effect of SPL and NPL (17.1%) on the phytoplankton compositions was greater than the effect of WT (15.2%). Furthermore, the explanatory power increased by 18.0% (from 15.2% to 33.2%) when SPL and NPL were used as environmental variables. This result suggests that the phytoplankton communities in the Chuanfang River were more strongly influenced by water pollution than by WT, although the effects of WT on the phytoplankton communities were more statistically significant (Sato et al. Citation2002).
Generally, the substantial differences in the phytoplankton community structure between S0 and the other four sampling sites in the Chuanfang River can be attributed to the influx of STP effluent, and the results of the CCA further confirmed that the degree of water pollution (SPL and NPL) exerted an important influence on phytoplankton composition. However, a closer and more in-depth analysis is required to determine the specific mechanisms of the phytoplankton community transitions. In December 2013 (winter) and July 2014 (summer), the SPL at S0 was extremely high, and Chlorophyta (especially Pediastrum in summer and Scenedesmus in winter) exhibited absolute dominance. The plankton survey in October 2013 (autumn) showed that Chlorophyta could still thrive at S0 even if sewage pollution was reduced to a lower level. Hence, in this study, the phytoplankton community shift from Chlorophyta (green algae) to Bacillariophyta (diatoms) was not determined by the mitigation of sewage pollution. However, considering the pollution caused by the inflow of sewage in the river, the most distinctive change in water quality in the Chuanfang River after the influx of STP effluent was actually the sudden increase in NO3-N concentration. Thus, in terms of water quality, NO3-N can be considered a key parameter that caused the dominant phytoplankton taxa to shift to diatoms in the Chuanfang River (Iriarte et al. Citation2013; Zhang et al. Citation2013; Deng et al. Citation2014; Baek et al. Citation2015). This inference is highly consistent with the laboratory experiments conducted by Deng et al. (Citation2014). In their experiments, they also found that the dominant phytoplankton taxa shifted from green algae to diatoms after adding KNO3 and K2HPO4 to enhance the nutrient levels of the treatments. Furthermore, the increase in diatom abundance in summer and autumn also indicated that the dominance of diatoms can be facilitated by increasing WT, which is also consistent with the experiments by Deng et al. (Citation2014). Therefore, we conclude that the increase of NO3-N and the seasonal rise in WT made the diatoms dominant in the Chuanfang River after the influx of the STP effluent. This conclusion is also well supported by the results of the CCA, which showed that the explanatory power of WT&NPL (27.0%) was stronger than the explanatory power of WT&SPL (21.1%), SPL&NPL (17.1%) or WT + NPL (24.1%). The zooplankton communities in the Chuanfang River were also significantly influenced by WT (15.9%). However, the zooplankton also showed a few distinct changes in species composition with changes in the degree of water pollution in the Chuanfang River. In the CCA model of zooplankton, NPL alone provided a relatively good explanation (8.3%) of the variance in the dominant zooplankton taxa, and the combination of WT&NPL only explained 5.3% more of the variance than WT alone. Therefore, we conclude that in the Chuanfang River, zooplankton communities are less sensitive than phytoplankton to changes in water pollution status, and the zooplankton community structure of the Chuanfang River is predominantly influenced by WT (Czerniawski et al. Citation2013; Wu et al. Citation2014; Emerson and Bollens Citation2015).
Conclusions
Overall, the recharge of STP effluent is beneficial to the mitigation of water pollution in the Chuanfang River caused by the inflow of sewage, especially when the nitrification process of the STP works properly. However, STP effluent also significantly increases the concentration of nitrate nitrogen, which together with the seasonal rise in WT, makes the dominant phytoplankton species shift from Chlorophyta to Bacillariophyta. The phytoplankton community structure of the Chuanfang River is more strongly influenced by changes in water pollution than by variation in WT, although the effects of WT on phytoplankton communities are more statistically significant. Zooplankton communities in the Chuanfang River show a much weaker relationship with changes in water pollution than do phytoplankton communities and zooplankton are mainly influenced by WT.
In order to reduce the high TN and TP loading in river after the water recharge, several methods can be implemented. First, the water quality from the water treatment plant (WTP) could be improved by decreasing the nitrogen loading. Second, in situ treatment projects could be implemented to increase the river's self-purification capacity. These in situ projects could include the use of ecological concrete to repair the hardened riverbed and fish stocking to increase the biomass in the river. Finally, an estuarine wetland could be built to further decrease pollutant loading.
Acknowledgments
The authors are grateful to JufenNie of the Yunnan Institute of Environmental Science for her assistance in water quality monitoring. This paper was supported by the Chinese National Key Projects of Water Pollution and Reclamation (No. 2012ZX07102-002, No. 2009ZX07102-003).
Disclosure statement
No potential conflict of interest was reported by the authors.
Additional information
Funding
Notes on contributors
Zhujing Jin
Zhujing Jin is currently a PhD candidate in School of Environmental Science and Engineering, Shanghai Jiao Tong University, under the direction of professor Baoxue Zhou. She received her MSc degree in Shanghai Jiao Tong University. Her research focuses on water pollution control.
Xianzhi Zhang
Xianzhi Zhang is currently an MSc candidate in School of Environmental Science and Engineering, Shanghai Jiao Tong University, under the direction of professor Baoxue Zhou. His research focuses on water pollution control.
Jinhua Li
Jinhua Li is an associate professor of the environmental function material group at School of Environmental Science and Engineering, Shanghai Jiao Tong University. She received her doctoral degree from Shanghai Jiao Tong University. Her research focuses on water pollution control.
Fengle Yang
Fengle Yang is a professor of the Yunnan Institute of Environmental Science. He received his MSc degree from Kunming University of Science and Technology. Her research focuses on water pollution control.
Deping Kong
Deping Kong is a professor of the Yunnan Institute of Environmental Science. He received his doctoral degree from Kunming University of Science and Technology. Her research focuses on water pollution control.
Ran Wei
Ran Wei is currently an MSc candidate in School of Environmental Science and Engineering, Shanghai Jiao Tong University, under the direction of professor Baoxue Zhou. Her research focuses on water pollution control.
Ke Huang
Ke Huang is currently a PhD candidate in School of Environmental Science and Engineering, Shanghai Jiao Tong University, under the direction of professor Baoxue Zhou. She received her MSc degree in Donghua University. Her research focuses on water pollution control.
Baoxue Zhou
Baoxue Zhou is a professor and head of the environmental function material group at the School of Environmental Science and Engineering, Shanghai JiaoTong University. He received his doctoral degree from the Harbin Institute of technology. His research is focused on photocatalytic/photoelectron catalytic technology of nanomaterials for organic pollutant treatments, hydrogen production from water decomposition and environmental sensor.
References
- Baek SH, Kim D, Son M, Yun SM, Kim YO. 2015. Seasonal distribution of phytoplankton assemblages and nutrient-enriched bioassays as indicators of nutrient limitation of phytoplankton growth in Gwangyang Bay, Korea. Estuar Coast Shelf Sci. https://doi.org/10.1016/j.ecss.2014.12.035
- Brito AC, Moita T, Gameiro C, Silva T, Anselmo T, Brotas V. 2015. Changes in the phytoplankton composition in a temperate estuarine system (1960 to 2010). Estuar Coast. 38:1678–1691.
- Chen WM, Andrus H. 2015. Intracellular nitrite accumulation: the cause of growth inhibition of Microcystis aeruginosa exposure to high nitrite level. Phycol Res. 63:197–201.
- Czerniawski R, Pilecka-Rapacz M, Domagała J. 2013. Zooplankton communities of inter-connected sections of lower River Oder (NW Poland). Cent Eur J Biol. 8:18–29.
- Deng JM, Qin BQ, Hans WP, Zhang YL, Wu P, Ma JR, Chen YW. 2014. Effects of nutrients, temperature and their interactions on spring phytoplankton community successionin Lake Taihu, China. PLoS ONE. 9(12):e113960.doi:10.1371/journal.pone.0113960
- Emerson JE, Bollens SM. 2015. Seasonal dynamics of zooplankton in Columbia-Snake river reservoirs, with special emphasis on the invasive copepod Pseudodiaptomusforbesi. Aquat Invasions. 10:25–40.
- Ghorbani-Aghdam M, Dinpashoh Y, Mostafaeipour A. 2013. Application of factor analysis in defining drought prone areas in Lake Urmia Basin. Nat Hazards. 69:267–277.
- Gren I M. 2008. Adaptation and mitigation strategies for controlling stochastic water pollution, application to the Baltic Sea. Ecol Econ. 66:337–347.
- Honti M, Istvánovics V, Kovács ÁS. 2010. Balancing between retention and flushing in river networks – optimizing nutrient management to improve trophicstate. Sci Total Environ. 408:4712–4721.
- Hu HJ, Wei YX. 2006. The freshwater algae of china: systematics, taxonomy and ecology. Beijing: Science Press.
- Huang CC, Wang XL, Yang H, Li YM, Wang YH, Chen X, Xu L. 2014. Satellite data regarding the eutrophication response to human activities in the plateau lake Dianchi in China from 1974 to 2009. Sci Total Environ. 485–486:1–11.
- Huertas EI, Rouco M, López-Rodas V, Costas E. 2011. Warming will affect phytoplankton differently: evidence through a mechanistic approach. Proc R Soc Lond B Biol Sci. 278:3534–3543.
- Iriarte JL, Pantoja S, González HE, Silva G, Paves H, Labbé P, Rebolledo L, Van AM, Häussermann V. 2013. Assessing the micro-phytoplankton response to nitrate in Comau Fjord (42°S) in Patagonia (Chile), using a microcosms approach. Environ Monit Assess.185:5055–5070.
- Kelso BHL, Smith RV, Laughlin RJ, Lennox SD.1997. Dissimilatory nitrate reduction in anaerobic sediments leading to river nitrite accumulation. Appl Environ Microbiol. 63:4679–4685.
- KEPB: Kunming Environmental Protection Bureau. 2011. Kunming: Kunming environmental quality bulletin; [access 2011]. http://www.kmepb.gov.cn/info/iList.jsp?cat_id=10269.
- Lázár AN, Wade AJ, Whitehead PG, Neal C, Loewenthal M. 2012. Reconciling observed and modelled phytoplankton dynamics in a major lowland UK river, the Thames. Hydrol Res. 43:576–588.
- Li GB, Li L, Pan M, Xie ZC, Li ZX, Xiao BD, Liu GH, Chen J, Song LR. 2014. The degradation cause and pattern characteristics of Lake Dianchi ecosystem and new restoration strategy of ecoregion and step-by-step implementation. J Lake Sci. 26(4):485–496.
- Li H, Wang Y, Shi LQ, Song D, Pan XJ. 2012. Distribution and fractions of phosphorus and nitrogen in surface sediments from Dianchi Lake. China Int J Environ Res. 6(1):195–208.
- Li RN, Chen QW, Zhang XQ, Recknagel F. 2015. Effects of temperature and macronutrients on phytoplankton communities across three largely different lakes identified by a time-space trade-off approach. Ecol Inform. 29(P2):174–181.
- Mustapha A, Aris AZ, Ramli MF, Juahir H. 2012. Spatial-temporal variation of surface water quality in the downstream region of the Jakara River, north-western Nigeria: a statistical approach. J Environ Sci Health A. 47:1551–1560.
- Mutshinda CM, Finkel ZV, Irwin AJ. 2013. Which environmental factors control phytoplankton populations? A Bayesian variable selection approach. Ecol Model. 269:1–8.
- Newcomer TA, Kaushal SS, Mayer PM, Shields AR, Canuel EA, Groffman PM. 2012. Influence of natural and novel organic carbon sources on denitrification in forest, degraded urban, and restored streams. Ecol Monogr. 82:449–466.
- Roach WJ, Grimm NB. 2009. Nutrient variation in an urban lake chain and its consequences for phytoplankton production. J Environ Qual. 38:1429–1440.
- Sato K, Sakui H, Sakai Y, Tanaka S. 2002. Long-term experimental study of the aquatic plant system for polluted river water. Water Sci Technol. 46:217–224.
- Shen JR, Dai AY, Song RX. 1979. Chinese animal record, Arthropoda, Crustacea, freshwater copepods. Beijing: Science Press.
- Sulthana A, Latha KC, Rathan R, Sridhar R, Balasubramanian S. 2014. Factor analysis and discriminant analysis of wastewater quality in Vidyaranyapuram sewage treatment plant, Mysore, India: a case study. Water Sci Technol. 69:810–818.
- Sun YX, Wu GX, Hu HY, Guo F, Wu YH, Guo YM. 2013. Assessment of effluent quality of wastewater treatment plant in Kunming based on the percentile of standard compliance method. China Environ Sci. 33:1113–1119.
- Teittinen A, Taka M, Ruth O, Soininen J. 2015. Variation in stream diatom communities in relation to water quality and catchment variables in a boreal, urbanized region. Sci Total Environ. 530–531:279–289.
- TerBraak CJF, Smilauer P. 2002. CANOCO reference manual and canocodraw for windows user's guide: software for canonical community ordination (version 4.5). Ithaca (NY): Microcomputer Power.
- Tornés E, Pérez MC, Durán C, Sabater S. 2014. Reservoirs override seasonal variability of phytoplankton communities in a regulated Mediterranean river. Sci Total Environ. 475:225–233.
- Wu XD, He DM, Yang GJ, Ye LL, Zhu CL, Jia HF, Hu JM. 2014. Seasonal variability of water quality and metazooplankton community structure in Xiaowan Reservoir of the upper Mekong River. J Limnol. 73:167–176.
- Zhao C S, Liu C M, Xia J, Zhang Y, Yu Q, Eamus D. 2012. Recognition of key regions for restoration of phytoplankton communities in the Huai River basin, China, J Hydrol. 420–421:292–300.
- Zębek E. 2015. Response of planktonic cyanobacteria and periphyton assemblages to physicochemical properties of storm water in a shallow urban lake. J Elementol. 20:231–245.
- Zhang JY, Ni WM, Zhu YM, Pan WD. 2013. Effects of different nitrogen species on sensitivity and photosynthetic stress of three common freshwater diatoms. Aquat Ecol. 47:25–35.