Abstract
In this study, the concept of phytoplankton functional groups was used to assess phytoplankton community structure in an attempt to better understand their spatial and seasonal variation in extremely alkaline Dali Nur Lake. Samples were collected for analysis during spring, summer and autumn for a period of two years. We identified 13 phytoplankton functional groups (Lo, S1, H1, P, C, MP, X3, Y, X2, W1, J, F, and T) among 37 species out of which 7 groups Lo, H1, P, C, MP, X2, and F were dominant. The biomass of phytoplankton functional groups in the Lake exhibited a strong seasonal variation with the highest biomass value observed in autumn and lowest in spring. Functional groups MP, P, and C were dominant in spring when the temperatures were very low. Groups H1 and X2 were the dominant in summer corresponding to higher temperatures. In autumn groups Lo and X2 were the dominant corresponding to high levels of total phosphorus (TP). Our study revealed that alkalinity, pH, arsenic, chemical oxygen demand, and TP are the most important physical–chemical variables structuring the phytoplankton functional groups in Dali Nur Lake. Functional groups Lo and X2 were mainly influenced by TP while groups MP and P flourished better under high conditions of arsenic. This study reveals the importance of physical–chemical variables in the spatial and seasonal gradient, in structuring phytoplankton functional groups, and consequently in the assessment of environmental status of the lake.
Introduction
Inland alkaline lakes are characterized by high concentration of carbonates salts with a pH value ranging between 9 and 12 and a topography that limits water outflow from the lake (Hammer Citation1986). Global alkaline lakes tends to occur in semi-arid or arid areas with very high productivity compared to other aquatic systems (Melack and Kilham Citation1974; Oduor and Schagerl Citation2007a) and a confined diversity of microbial life due to high salinity and pH values (Nielsen Citation1999; Oren Citation2011). In spite of their ecological value, studies on the composition and distribution of plankton species is very scant compared to freshwater systems (Sun et al. Citation2009; Xu et al. Citation2010; Niu et al. Citation2011; Yu et al. Citation2012; Sui et al. Citation2016). Several environmental factors such as water temperature, conductivity, total phosphorus, calcium ions, magnesium ions and hydrological pattern have been considered as essential factors responsible for shaping biological composition in saline–alkaline lake (Wood and Talling Citation1988; Oduor and Schagerl Citation2007b; Schagerl and Oduor Citation2008). In regions where seasonality is very distinct, variations in environmental factors plays a major role in plankton composition and structure via the influence of light and nutrients concentrations (Yu et al. Citation2012). Notably, also is that composition and community structure of organisms in alkaline lakes seems to be controlled by the level of alkalinity and stability of other environmental factors (Sui et al. Citation2016). According to García et al. (Citation1997) in water bodies where topography limits water outflow from the lake, then seasonality is an important factor that influence the environmental variables. This could in turn affect phytoplankton composition.
Phytoplankton composition and structure have been researched by different scientists in saline–alkaline lakes in China (Luo et al. Citation2000; XU J-y et al. Citation2001; Sui et al. Citation2016). These authors have reported: (i) decrease in taxonomic diversity, genera richness and cell density with increase of saline–alkaline gradient and (ii) dominance of Melosira granulate, Melosira ambigua, and Cyclotella meneghiniana species in most of the lakes. However, despite the fact that phytoplankton forms essential component of aquatic foodweb (Tian et al. Citation2016), no studies about their composition and community structure has been conducted in Dali Nur Lake. Dali Nur Lake is situated in an endorheic basin at an ecotone of semi-arid to semi-humid in the eastern part of Inner Mongolia Plateau, North China. The lake experiences high rate of evaporation than rainfall and inflows hence its area has reduced tremendously while alkalinity increased greatly (Xiao et al. Citation2008). The mean current values of alkalinity and salinity of the lake is over 50 mmol/L and 6‰, respectively with a pH in the range of 8.25–9.6. The main objectives of this study are: (i) to assess the spatial-temporal variation of phytoplankton functional groups and their biomass in Dali Nur Lake and (ii) determine the physical–chemical variables influencing the biomass of phytoplankton functional groups in the highly alkaline Dali Nur Lake. We attempted to answer the following questions: (i) Is there any spatial variation in phytoplankton functional groups, predominantly between offshore and inshore sites in Dali Nur Lake? (ii) Is there any seasonal variation in phytoplankton functional groups in Dali Nur Lake? (iii) Which physical–chemical variables influence the spatial and seasonal variation of phytoplankton functional groups? The findings in this study are important for management of Dali Nur Lake and other aquatic systems with similar characteristics in the world.
Materials and methods
Study area and sampling sites description
Dali Nur Lake including the sampling points is shown in . Dali Nur is an inland closed-basin lake which lies at 116°25′-116°45′E, 43°13′-43°23′N eastern part of Inner Mongolia Plateau at an altitude of 1226 m above sea level. The lake surface area and maximum water depth is 238 km2 and 11 m, respectively (Xiao et al. Citation2008). In the north and west, the lake is surrounded by basaltic rocks while on the eastern side there are lacustrine plains. The lake is fed by two permanent rivers (Gongger and Salin rivers) from the northeast and two seasonal rivers (Holai and Yangsen rivers) from the southwest with no outflow. The study area is under the influence of East Asian monsoon with an average yearly temperature of 1–2 °C. Average rainfall received in the area is between 350 and 400 mm per year with more than 50% falling in June to August while evaporation reaches 1287 mm. Dali Nur Lake is an important ecosystem both local and international since it has Leuciscus waleckii a cyprinid fish which is a source of food and income to the local communities, and it is an important foraging ground for migrating birds (Zhang et al. Citation2008). Sampling sites were selected taking into consideration the viability of approaching and maximum representation of the lake. The sites included both offshore areas (1#, 3#, 5#, and 8#) which are further away from the discharge point of the river inlets and inshore sites (2#, 4#, 6#, and 7#) which are closer to discharge point of the inflowing rivers ().
Sampling collection and laboratory analysis
Samples were collected in spring (April to May), summer (July to August), and autumn (September to November) from the eight georeferenced sampling sites inside the Dali Nur Lake (). Note, however, that sampling was not conducted during the period of winter because the lake was covered by snow and ice, and also for safety reasons. Water temperature and pH readings were obtained in the field at each sampling site using a portable multi-probe (YSI 6600, YSI Inc., Yellow Springs, OH, USA) while water transparency (SD) was determined using Secchi disk. Unfiltered water for physical–chemical and phytoplankton analysis was sampled with calibrated 2.5 L van Dorn sampler at the surface and the bottom of the water column and integrated over depth for every site. Phytoplankton samples were fixed in the field with Lugol’s solution, while samples for nutrients were placed in cool box and transported to laboratory for analysis. In the laboratory total nitrogen (TN) and total phosphorus (TP) were measure by oxidation with alkaline persulfate and persulfate acid as described by Costa et al. (Citation2009). Biological oxygen demand (BOD5) and chemical oxygen demand (CODcr) were determined using the standards methods of China MEP (Ministry of Environmental Protection Citation2002). Alkalinity was determined by the acidimetric method using H2SO4 as titrant while mercury (Hg) and arsenic (As) concentrations were determined using a hydride generation-atomic fluorescence spectrometer.
The fixed samples for phytoplankton analysis were sedimented for 48 h and concentrated to 30 mL. Identification and counting of phytoplankton species was conducted with an inverted microscope at 400× magnification using several keys, illustrations and techniques from different literature (Prescott Citation1954; Utermöhl Citation1958; Komárek and Fott Citation1983; Hu Citation2006). Biovolume (mm³/L) was estimated based on the solid geometric shape (Hillebrand et al. Citation1999). Cell volumes of at least 40 algal units were estimated by approximation to the nearest solid geometric solid (Chen et al. Citation2003). Conversion of biovolume into biomass was done as 1 mm3/L = 1 mg/L (Wetzel and Likens Citation2000). The species were grouped into functional group as described by (Reynolds et al. Citation2002; Reynolds Citation2006; Padisák et al. Citation2009).
Data analysis
Statistical analysis was done using SPSS 17.0(SPSS, 2008) and Microsoft Excel window 2007. Prior to analysis, the physical–chemical variables except pH were log transformed, biomass of phytoplankton functional groups was log (1 + x) transformed to manage variance heterogeneity and ensure the data is normal distributed. The differences of physical–chemical variables and biomass of phytoplankton functional groups in different seasons and sampling sites were analyzed using one-way ANOVA. Relationships between phytoplankton functional groups and physical–chemical variables were analyzed using the detrended correspondence analysis and redundancy analysis (RDA) using CANOCO 4.5 software (Microcomputer Power, New York, USA). Monte Carlo simulations with 499 permutations were used to test the significance of the physical–chemical variables in explaining the biomass of phytoplankton functional groups data in the RDA. Pearson correlation analysis was done to confirm the significant relationships between physical–chemical variables and the biomass of phytoplankton functional groups. Figures were drawn using Microsoft excel. Unless otherwise indicated, the results are reported as mean value ± standard error (SE).
Results
Physical–chemical variables
The mean seasonal values of physical–chemical variables recorded among the sampling sites within the study period are presented in . Water temperature (WT) and water transparency (SD) showed significant differences among the three seasons (p < 0.05) with the highest values observed in summer. The average WT and SD ranged from 4.0 (summer) to 23.5 °C (spring) and 51.4 (Summer) to 74.0 cm (spring), respectively. The values of pH measured in this study were in the side of alkalinity (basic) with seasonal variations. High pH values were recorded in summer (9.51) followed by autumn (9.41) and then spring (9.28). Sites 2# and 4# located at discharge point of the permanent inflowing rivers recorded relatively high values of pH (). As one of the important environmental variable, CODcr also differs among seasons. A post hock test revealed that CODcr values observed in spring differ significantly with the other seasons. Spatial, site located inshore recorded high values of CODcr in all seasons (). Nutrient concentrations (TN and TP) differed significantly among seasons (p < 0.05). The highest concentrations of TN and TP were observed in spring and autumn respectively. Mean TP and TN ranged from 4.90 (spring) to 3.74 mg/L (summer) and 2.05 (autumn) to 1.72 mg/L (spring), respectively. Interestingly, mean TP values were low in site 2#, 3#, and 4# where CODcr values were high. Moreover, statistically differences among seasons were recorded for alkalinity (ALK), BOD5, and As, with the highest values recorded in spring. Average values of ALK ranged between 3224.43 (spring) and 2766.31 mg/L (autumn). No statistical difference was observed for Hg among seasons. However, relative high values were recorded in spring.
Figure 2. Spatial variations of selected physical-chemical variables from Dali Nur Lake in different seasons.
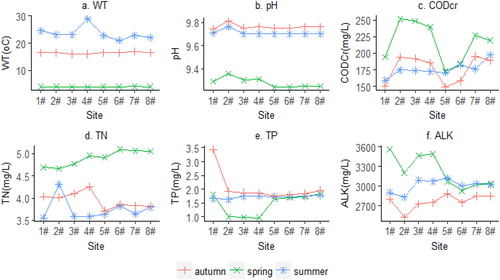
Table 1. Mean and standard error for the physical–chemical variables in the temporal dimension (Spring, Summer, and Autumn) from the eight georeferenced sampling sites inside the Dali Nur Lake.
Phytoplankton dynamics
In this study, a total of 37 phytoplankton species were found belonging to seven taxonomic classes: Chlorophyceae (16 species), Bacillariophyceae (11 species), Cyanophyceae (5 species), Euglenophyceae (2 species), Chrysophyceae (1 specie), Cryptophyceae (1 specie), and Dinophyceae (1 specie; ). The 37 phytoplankton species were categorized in 13 functional groups (Lo, S1, H1, P, C, MP, X3, Y, X2, W1, J, F, and T) as described by (Reynolds et al. Citation2002; Reynolds Citation2006; Padisák et al. Citation2009). Seven groups (Lo, H1, P, C, MP, X2, and F) were categorized as dominant groups defined by contributing a minimum of 5% of the total biomass as recommended in literature(Reynolds et al. Citation2002). The remaining six functional groups were clustered together as ‘others’ group. The seven dominant groups accounted for more than 90% of the total phytoplankton biomass hence used to analyze the composition and dynamics of phytoplankton community in the Dali Nur Lake ().
Table 2. List of phytoplankton species with their taxonomic, functional groups, and percentage contribution to their total biomass for Dali Nur Lake.
Spatial and temporal variation of phytoplankton functional groups
present the seasonal variation of the seven most dominant phytoplankton functional groups. The mean phytoplankton functional groups biomass range from 0.10 to 0.89 mg/L, with high biomass value recorded in autumn while in spring lower value was recorded. Statistically significant differences in functional group biomass was observed among seasons as determined by one-way ANOVA (p < 0.05). A post hock test showed that the biomass was statistically significant lower in spring (0.10 ± 0.01 mg/L) and summer (0.17 ± 0.09 mg/L) compared to that of autumn (0.89 ± 0.06 mg/L). In spring groups X2, C, MP, and Lo shared dominance with relative biomass levels of about 24.55%, 21.78%, 20%, and 19.41%, respectively. With exception of functional group H1, all the other six dominant functional groups were present in all sites in spring (). In summer, functional groups H1 composed of Anabaena circinalis and X2 mainly represented by Chlamydomonas globosa, Chlamydomonas ovalis, and Lobomonas granulate species were the most common accounting for 46.28% and 34.29% of the total biomass, respectively. Spatial, high biomass of group H1 was observed at site 2# corresponding to high values of TN while X2 were found at sites 1#, 2#, 3#, 5#, and 6# (). In addition, groups Lo, MP, and C were observed in summer at different sites but with low biomass values. In autumn the Merismopedia minima, Synechocystis minuscule, and Chroococcus minutes species of functional group Lo and the C. globosa, C. ovalis and L. granulate species of group X2 accounted for about 56.14% and 29.65% of the biomass respectively (). In all sites, the relative biomass of group Lo was almost similar in autumn. Moreover, group X2 showed a similar pattern to that of group Lo along the sites, though its biomass value was lower than that of Lo. Interestingly, group H1 was only observed at sites 2# and 5# only during autumn.
Relationship between physical–chemical variables and phytoplankton functional groups
The results of the RDA revealed that the physical–chemical variables explained 85.2% of the variations in phytoplankton functional groups biomass (). The first two RDA axes explained 82% (axis 1: 69.3%; axis 2: 12.7%) of the variation in phytoplankton functional groups biomass data and 96.2% (axis 1: 81.3%; axis 2: 14.9%) of the functional groups biomass–environment relationship. Axis 1 of the RDA was positively correlated with pH (0.601), TP (0.490), BOD5 (0.349), WT (0.333), SD (0.132), and Hg (0.062) and negatively to ALK (−0.719), As (−0.499), CODcr (−0.377), and TN (−0.317). Axis 2 was positively correlated with WT (0.243), pH (0.227), and TN (0.030) and negatively to BOD5 (−0.695), Hg (−0.425), As (−0.296), ALK (−0.205), SD (−0.166), CODcr (−0.151), and TP (−0.046). Monte Carlo test showed that the first RDA axis (F-ratio = 29.326, p value = 0.002) and all RDA axes (F-ratio = 7.483, p value = 0.0020) were significant.
In the Pearson correlation analysis results for the phytoplankton functional groups biomass with physical–chemical variables are presented. The biomass of functional group Lo was positively correlation with pH, TP, and BOD5 and negatively correlated with ALK and As. Group H1 had a negative correlation with BOD5. The P group was negatively correlated with WT, SD, and pH and positively correlated with TN and CODcr. Group MP was positively correlated with TN, ALK and As and negatively correlated with WT and pH. Group X2 represented by C. globosa, L. granulate, C. ovalis, and Euglena oxyuris was positively correlated with pH and TP and negatively to CODcr, ALK, and As. The F group was positively and negatively correlated with pH and ALK, respectively. The TB (of all major functional groups) was negatively correlated with CODcr, ALK, and As and positively correlated to pH and TP.
Table 3. Pearson correlation coefficients between physical–chemical variables and the biomass of phytoplankton functional groups.
Discussion
Spatial and seasonal variation of phytoplankton functional groups in Dali Nur Lake
According to Reynolds (Citation1998), phytoplankton species have undergone structural and physiological changes as a strategy for surviving in different aquatic systems. Guided by the work of Grime (Citation1979) who used the concept of adaptive ‘strategies’ to identify and characterized life-history and physiological activity of plants, Reynolds (Citation1997) described several phytoplankton functional groups that may possible dominate or co-dominate in a given environment (Reynolds Citation2006; Reynolds et al. Citation2002). In the recent past, the concept of using phytoplankton functional groups based on the morphological, physiological and ecological characteristics of the specie has proved to be reliable approach to analyze spatial and temporal variations in phytoplankton biomass (Becker et al. Citation2010; Yang et al. Citation2011; Gillett et al. Citation2015; Li et al. Citation2017).
With the current study site being an inland closed-basin lake with some sampling sites located further away from the discharge point of the river inlets (offshore areas) and others closer to discharge point of the inflowing rivers (inshore areas), we anticipated to uncover some differences in phytoplankton functional groups biomass. However, with exception of summer period, no spatial difference in phytoplankton functional groups biomass was evident in spring and autumn. We hypothesize that lack of spatial variation in phytoplankton functional groups biomass in spring and autumn could be due to the horizontally well-mixed waters in the lake. In summer, site 2# recorded high biomass values dominated by cyanobacteria (group H1) corresponding to high pH and TN values. According to Atici and Obali (Citation2006) and Okoth et al. (Citation2009) cyanobacteria can outcompete other phytoplankton species in polluted environments because they have the ability to utilize carbon from the polluted environments at high pH levels. This therefore suggests that the permanent river (Gongger river) draining into Dali Nur Lake through site 2# has some degree of pollution.
Unlike the spatial variations, phytoplankton functional groups biomass in Dali Nur Lake exhibited a strong seasonal variation. Similar to other lake in temperate regions (Yang et al. Citation2011; Gillett et al. Citation2015), phytoplankton functional groups biomass was dominated by bacillariophyta (groups MP, P, and C) and chlorophyta (X2) in spring corresponding to low temperatures (4 °C) and relatively high nutrients. Similarly, Pearson correlation analysis results confirmed that groups MP, P, and C were negatively correlated with WT (). As observed in other studies, low temperature can lead to increase in bacillariophytes (diatoms; Nowrouzi and Valavi Citation2011). The dominance of bacillariophyta in spring should therefore be attributed to the low temperatures. In summer cyanobacteria (mainly group H1) was the dominant corresponding to high temperature values. Moreover, chlorophyta (X2) accounted for about 35% of the total biomass in summer. These seasonal variations of phytoplankton functional groups observed in this study agrees well with the Plankton Ecology Group (PEG) model of freshwater systems (Sommer et al. Citation1986). According to the PEG model, bacillariophytes (diatoms), and cryptophytes dominate in spring when nutrients are available with overwintering leading to low temperatures. In early summer chlorophytes develops when nutrients are available and when temperature is high and silica is limiting cyanobacteria becomes dominate. In their study, Kosten et al. (Citation2012) and Gillett et al. (Citation2015) further verified that under warmer water temperatures, cyanobacteria often occur as the dominant phytoplankton community. Group Lo and X2 are also sensitive to water mixing; therefore, their dominance in summer could also be explained by the thermal stratification phenomena which prevent water mixing (Reynolds et al. Citation2002). Contrary to the prediction in PEG model, autumn was still dominated by cyanobacteria (mainly group Lo) and chlorophytes. Authors such as Paerl and Huisman (Citation2008), Kruk et al. (Citation2002) and Sommer et al. (Citation1986) observed that slowly variations in phytoplankton biomass from summer to autumn may be more characteristics of eutrophic environments where climate change might lengthen cyanobacterial growth season.
Interaction between phytoplankton functional groups with physical and chemical variables in Dali Nur Lake
In comparison with other water bodies, the nutrients levels (TN and TP) in this current study are remarkable higher. For instance in Xiangxi Bay, Three Gorges Reservoir China, TN values for spring, summer and autumn were 1.31, 1.58, and 1.55 mg/L, respectively and TP values were 0.46, 0.18, and 0.09 mg/L for spring, summer, and autumn, respectively (Yang et al. Citation2011). In Sau Reservoir located in northeast Spain, Becker et al. (Citation2010) recorded 2.56, 1.25, and 1.74 mg/L values of TN and 0.05, 0.04, and 0.07 mg/L values of TP for spring, summer and autumn respectively. However, although the nutrients levels were very high in Dali Nur Lake offering good conditions for high phytoplankton growth, the biomass of functional groups were hundred times lower than that of Sau Reservoir (110.8 mg/L). This implies that other physical–chemical variables essentially shape the phytoplankton functional groups in the lake. In this study, the most important physical–chemical variables structuring the biomass of phytoplankton functional groups in Dali Nur Lake are ALK, pH, As, CODcr, and TP. Alkalinity which is the measure of carbonate in aqueous solution negatively correlated with most of the biomass of phytoplankton functional groups in this study. According to Siebielec et al. (Citation2015), the most dominant cation in alkaline systems is calcium that react with phosphate leading to the formation of hydroxyapatite, octocalcium phosphate and dibasic calcium phosphate dehydrate which are insoluble and unavailable for algae growth. Similarly, as the pH rises above 7.0 in aquatic systems such as in Dali Nur Lake, most of the dissolved phosphorus reacts with calcium forming insoluble calcium phosphate species that cause phosphate to become unavailable (Esteves and Menezes Citation2011; da Silva Cerozi and Fitzsimmons Citation2016). This therefore implies that although TP occurred in high concentration, it is possible that phosphorus is a limiting nutrient for phytoplankton functional groups in Dali Nur Lake. This study reveals that species selectivity in the highly alkaline Dali Nur Lake favors groups Lo and X2, those with high surface/volume ratios and which shows wide range of nutrients concentrations adaptability (Reynolds et al. Citation2002; Yang et al. Citation2011; Li et al. Citation2017). Groups Lo and X2 were mainly comprised of minute-celled M. minima and C. globosa which can adapt to low nutrients (mainly phosphorus) levels (Laamanen Citation1997). Accordingly, in this study, groups Lo and X2 were positively influenced by TP (Pearson correlation analysis; ). Other studies have also reported positive correlation between group Lo and TP in a phosphorus limiting environment (Shen et al. Citation2011, Citation2014). In addition, groups Lo and X2 were negatively correlated with higher ALK and As conditions.
The RDA biplot diagram () shows that groups MP and P were well adapted to high As and Hg conditions. Moreover, Pearson correlation analysis reveals that groups MP and P were positively correlated with As. This is in agreement with other studies in different part of the world. While assessing the responses of diatoms communities to heavy metals in streams in Colorado, Medley and Clements (Citation1998) reported that Achnanthes minutissima and members of Fragilaria sps such as Fragilaria vaucheriae, Fragilaria brevistriata, and Fragilaria virescens were tolerant to heavy metals. Similarly, Rushforth et al. (Citation1981) observed a positive correlation between diatoms species (C. meneghiniana, Navicula exigua, Navicula secreta var. apiculata, Nitzschia palea) with heavy metals in Uintah basin of Utah, USA. The authors further concluded that these taxa are valid indicators of high levels of heavy metals. Higher biomass of phytoplankton group MP and P were found in spring coinciding with high concentrations levels of As and Hg ( and ).
Conclusion
In this study, the concept of phytoplankton functional groups was used to asses phytoplankton community structure in an attempt to better understand their spatial and seasonal variation in extremely alkaline Dali Nur Lake. In general, the biomass of phytoplankton functional groups was very low compared to other water bodies and strongly varied with season. The highest biomass value was recorded in autumn mainly dominated by cyanobacteria (group Lo) and chlorophyta (X2) which were stimulated by the relatively high phosphorus concentrations levels. The seasonal variation of phytoplankton functional groups observed in Dali Nur Lake to some extent was explained by the PEG model of freshwater ecosystems. Our study revealed bacillariophytes (groups MP, P, and C) dominance in spring when nutrients are available with overwintering leading to low temperatures, dominance of chlorophytes (group X2) in early summer and cyanobacteria (group H1 and Lo) in mid-summer when temperatures are high. Interestingly, our results further revealed that ALK, pH, As, CODcr, and TP are the most important physical–chemical variables influencing the biomass of phytoplankton functional groups in Dali Nur Lake. Functional groups Lo and X2 were mainly influenced by TP while groups MP and P flourished under high conditions of heavy metals (As and Hg). Although TN concentrations has been documented as the essential elements explaining phytoplankton variability in aquatic systems, in Dali Nur Lake TN played no significant role in structuring the phytoplankton functional groups compared to ALK, pH, CODcr, and heavy metal (As). This study reveals the importance of physical–chemical variables in the spatial and seasonal gradient, in influencing phytoplankton functional groups, and consequently in the assessment of environmental status of the lake.
Acknowledgment
We would like to acknowledge all those who contributed to accomplishment of this paper and our families for the moral support.
Disclosure statement
No potential conflict of interest was reported by the authors.
Additional information
Funding
Notes on contributors
Chengxue Ma
Dr. Chengxue Ma is a lecturer at Northeast Forestry University, China. Currently, he is doing Postdoctoral training at Northeast Forestry University, China. He research interests are freshwater plankton ecology, nutrient cycling, ecosystem dynamics and cyanobacteria. In his academic service, he have produced several research publications, and supervised the research of M. Sc. students.
Patteson Chula Mwagona
Patteson Chula Mwagona has earned his bachelor’s degree in Applied Aquatic Science from Egerton University, Kenya. He earned his M. Sc in Limnology in 2013 from Egerton University, Kenya. Currently he is a PhD student at Northeast Forestry University, China majoring in Hydrobiology. His current research focus is on nutrients removal and greenhouse gas emission from wetland ecosystems. Skills and Expertise: water quality, aquatic ecology, freshwater ecology, aquatic science, benthic ecology, aquatic macroinvertebrates, freshwater biology. In his academic service, Patteson Chula Mwagona has produced several research publications.
Hongxian Yu
Hongxian Yu is a professor at Northeast Forestry University, China. She is the program head of Hydrobiology and Wetland Scien. In her academic service, she have produced several research publications, and supervised the research of PhD. and M. Sc. students. Her research interests are fisheries resources and ecology, plankton ecology, nutrient cycling, ecosystem dynamics and cyanobacteria. Prof. Hongxian Yu is devoted in Management for quality and safety in aquaculture production, drafting management standards for aquaculture and engaged in the support management and technical system of traceability in fishery industry of China.
Xiaowen Sun
Prof. Xiaowen Sun is a research scientist at Heilongjiang River Fisheries Research Institute, China. Heilongjiang River Fisheries Research Institute is the leading institute in the research fields of fishery bioengineering breeding and cold-water fish in China. His research field Biotenogy for Aquatic Species. Prof Xiaowen Sun has produced research publications, and supervised the research of PhD. and M. Sc. students. He has received honors and awards from the progress for Science and Technology in Ministry of Agriculture of P.R. China, 1994, and from the progress for Science and Technology in Chinese Academy of Fisheries Science, 2000.
Liqun Liang
Liqun Liang is a research scientist at Heilongjiang River Fisheries Research Institute, a leading institute in the research fields of fishery bioengineering breeding and cold-water fish in China. He is responsible for the fresh-water fishery development in Heilongjiang fisheries as well as the north areas of China. His research field is Genetics and Breeding of Fisheries. Liqun Liang mainly study up artificial propagation, genetic diversity and genetic improvement of the mainly cultured freshwater fishes.
Shahid Mahboob
Prof. Shahid Mahboob has earned his M. Sc in Zoology with specialization in Limnology in 1986 from University of Agriculture, Faisalabad, Pakistan. He earned his PhD in Zoology in 1992 with specialization in freshwater nutrition from University of Agriculture, Faisalabad, Pakistan. Later he received Postdoctoral training in 2002 from the Department of Aquatic Sciences, Curtin University of Technology, Western Australia. A long academic career spanning nearly three decades with experience in the University of Pakistan, Australia and Saudi Arabia. Presently working as Professor of Zoology, Department of Zoology, King Saud University, Riyadh, Kingdom of Saudi Arabia since June 26, 2012. Previously he has been Professor/Dean/Vice Chancellor, GC University, Faisalabad, Pakistan. In 31 years of academic service, I have produced over 240 research publications, and supervised the research of 03 PhD., 25 M. Phil. and 41 M. Sc. students. Besides curriculum development at various levels, I have authored One Books Two and won research and development grants from the Higher Education commission, Pakistan Science Foundation and British Council for the up-gradation of scientific facilities at GC University, Faisalabad, Pakistan. My current research focus is on the ecotoxicology, nutrition and monitoring of fish health and quality of fish meat.
References
- Atici T, Obali O. 2006. Seasonal variation of phytoplankton and value of chlorophyll a in the Sarıyar Dam Reservoir (Ankara, Turkey). Turkish J Bot. 30(5):349–357.
- Becker V, Caputo L, Ordóñez J, Marcé R, Armengol J, Crossetti LO, Huszar VL. 2010. Driving factors of the phytoplankton functional groups in a deep Mediterranean reservoir. Water Res. 44(11):3345–3354.
- Chen Y, Qin B, Teubner K, Dokulil MT. 2003. Long-term dynamics of phytoplankton assemblages: Microcystis-domination in Lake Taihu, a large shallow lake in China.J Plankton Res. 25:445–453.
- Costa L, Huszar V, Ovalle A. 2009. Phytoplankton functional groups in a tropical estuary: hydrological control and nutrient limitation. Estuaries Coasts. 32(3):508–521.
- da Silva Cerozi B, Fitzsimmons K. 2016. The effect of pH on phosphorus availability and speciation in an aquaponics nutrient solution. Bioresour Technol. 219:778–781.
- Esteves FA, Menezes C. 2011. Papel da Água e da Limnologia na sociedade Moderna. ESTEVES. FA Fundamentos de Limnologia. 3:62–73.
- García CM, García-Ruiz R, Rendón M, Niell FX, Lucena J. 1997. Hydrological cycle and interannual variability of the aquatic community in a temporary saline lake (Fuente de Piedra. Southern Spain). Hydrobiologia. 345(2):131–141.
- Gillett ND, Luttenton MR, Steinman AD. 2015. Spatial and temporal dynamics of phytoplankton communities in a Great Lakes drowned river-mouth lake (Mona Lake, USA). J Limnol. 74(3):453–466.
- Grime J. 1979. Plant strategies and vegetation processes. Chichester: Wiley, Google Scholar.
- Hammer UT. 1986. Saline lake ecosystems of the world. Vol.59. Springer Science & Business Media. Dr W. Junk Publishers.
- Hillebrand H, Dürselen CD, Kirschtel D, Pollingher U, Zohary T. 1999. Biovolume calculation for pelagic and benthic microalgae. J Phycol. 35(2):403–424.
- Hu H. 2006. The freshwater algae of China: systematics, taxonomy and ecology. Beijing: Science Press.
- Komárek J, Fott B. 1983. Chlorophyceae (Grünalgen). Ordnung: Chlorococcales. Das Phytoplankton des Süsswaser, Systematik und biologie. Hubber-Pestalozzi, G.
- Kosten S, Huszar VLM, Bécares E, Costa LS, Donk E, Hansson L-A, Jeppesen E, Kruk C, Lacerot G, Mazzeo N, et al. 2012. Warmer climates boost cyanobacterial dominance in shallow lakes. Glob Change Biol. 18(1):118–126.,
- Kruk C, Mazzeo N, Lacerot G, Reynolds C. 2002. Classification schemes for phytoplankton: a local validation of a functional approach to the analysis of species temporal replacement. J Plankton Res. 24(9):901–912.
- Laamanen MJ. 1997. Environmental factors affecting the occurrence of different morphological forms of cyanoprokaryotes in the northern Baltic Sea. J Plankton Res. 19(10):1385–1403.
- Li L, Li Q, Chen J, Wang J, Jiao S, Chen F. 2017. Temporal and spatial distribution of phytoplankton functional groups and role of environment factors in a deep subtropical reservoir. Chinese J Oceanol Limnol. 36(3):761–771.
- Luo X, Yi F, Sun G. 2000. Characteristics of alkaline lake wetland in Songnen Plain and discussion about its agricultural development—taking Yaopao Lake of Da’an Palaeochannel Area for example. Sci Geograph Sin. 20(5):483–486.
- Medley CN, Clements WH. 1998. Responses of diatom communities to heavy metals in streams: the influence of longitudinal variation. Ecol Appl. 8(3):631–644.
- Melack JM, Kilham P. 1974. Photosynthetic rates of phytoplankton in East African alkaline, saline lakes. Limnol Oceanogr. 19(5):743–755.
- MEP (Ministry of Environmental Protection). 2002. China’s national standard: GB3838-2002: Environmental quality standards for surface water. Beijing: Ministry of Environmental Protection.
- Nielsen JM. 1999. East African magadi (trona): fluoride concentration and mineralogical composition. J African Earth Sci. 29(2):423–428.
- Niu Y, Shen H, Chen J, Xie P, Yang X, Tao M, Ma Z, Qi M. 2011. Phytoplankton community succession shaping bacterioplankton community composition in Lake Taihu, China. Water Res. 45(14):4169–4182.
- Nowrouzi S, Valavi H. 2011. Effects of environmental factors on phytoplankton abundance and diversity in Kaftar Lake. J Fisheries Aquatic Sci. 6(2):130.
- Oduor S, Schagerl M. 2007. Phytoplankton photosynthetic characteristics in three Kenyan Rift Valley saline–alkaline lakes. J Plankton Res. 29(12):1041–1050.
- Oduor S, Schagerl M. 2007. Temporal trends of ion contents and nutrients in three Kenyan Rift Valley saline–alkaline lakes and their influence on phytoplankton biomass. Hydrobiologia. 584(1):59–68.
- Okoth OE, Mucai M, Shivoga WA, Miller SN, Rasowo J, Ngugi CC. 2009. Spatial and seasonal variations in phytoplankton community structure in alkaline–saline Lake Nakuru, Kenya. Lakes Reservoir Res Manag. 14(1):57–69.
- Oren A. 2011. Thermodynamic limits to microbial life at high salt concentrations. Environ Microbiol. 13(8):1908–1923.
- Padisák J, Crossetti LO, Naselli-Flores L. 2009. Use and misuse in the application of the phytoplankton functional classification: a critical review with updates. Hydrobiologia. 621(1):1–19.
- Paerl HW, Huisman J. 2008. Climate. Blooms like it hot. Science. 320(5872):57–58.
- Prescott GW. 1954. How to know the fresh-water algae: an illustrated key for identifying the more common fresh-water algae to genus, with hundreds of species named pictured and with numerous aids for their study. Vol.1. Dubuque, Iowa: W. C. Brown Company.
- Reynolds C. 1998. What factors influence the species composition of phytoplankton in lakes of different trophic status? Hydrobiologia. 369:11–26.
- Reynolds CS. 1997. Vegetation processes in the pelagic: a model for ecosystem theory. Vol.9. Oldendorf/Luhe, Germany: Ecology Institute.
- Reynolds CS. 2006. The ecology of phytoplankton. Cambridge: Cambridge University Press.
- Reynolds CS, Huszar V, Kruk C, Naselli-Flores L, Melo S. 2002. Towards a functional classification of the freshwater phytoplankton. J Plankton Res. 24(5):417–428.
- Rushforth SR, Brotherson JD, Fungladda N, Evenson WE. 1981. The effects of dissolved heavy metals on attached diatoms in the Uintah Basin of Utah, USA. Hydrobiologia. 83(2):313–323.
- Schagerl M, Oduor S. 2008. Phytoplankton community relationship to environmental variables in three Kenyan Rift Valley saline–alkaline lakes. Mar Freshwater Res. 59(2):125–136.
- Shen H, Li B, Cai Q, Han Q, Gu Y, Qu Y. 2014. Phytoplankton functional groups in a high spatial heterogeneity subtropical reservoir in China. J Great Lakes Res. 40(4):859–869.
- Shen H, Xu Y, Wang L, Zhang M, Kong L, Cai Q. 2011. Spatial and temporal variations of phytoplankton in Danjiangkou Reservoir and its affecting factors. Plant Sci J. 29(6):683–690.
- Siebielec G, Ukalska-Jaruga A, Kidd P. 2015. Bioavailability of trace elements in soils amended with high-phosphate materials. Phosphate in Soils: Interaction with Micronutrients, Radionuclides Heavy Metals. Vol. 2. pp. 237–260. Boca Raton, FL: CRC Press.
- Sommer U, Gliwicz ZM, Lampert W, Duncan A. 1986. The PEG-model of seasonal succession of planktonic events in fresh waters. Arch Hydrobiol. 106(4):433–471.
- Sui F, Zang S, Fan Y, Ye H. 2016. Effects of different saline–alkaline conditions on the characteristics of phytoplankton communities in the lakes of Songnen Plain, China. PLoS One. 11(10):e0164734
- Sun L, Jin XC, Zhong Y, Zhi YL, Li H, Zhou QX, Zhuang YY. 2009. Seasonal dynamics of phytoplankton in relation to key aquatic habitat factors in a polluted urban small water body in Tianjin, China. Bull Environ Contam Toxicol. 82(5):543–548.
- Tian W, Zhang H, Zhao L, Xu X, Huang H. 2016. The relationship between phytoplankton evenness and copepod abundance in Lake Nansihu, China. IJERPH. 13(9):855.
- Utermöhl H. 1958. Zur Vervollkommnung der quantitativen Phytoplankton-Methodik: Mit 1 Tabelle und 15 abbildungen im Text und auf 1 Tafel. Int Vereinigung Theor Angew Limnol: Mitteilungen. 9(1):1–38.
- Wetzel R, Likens G. 2000. Limnological analysis. New York: Springer.
- Wood R, Talling J. 1988. Chemical and algal relationships in a salinity series of Ethiopian inland waters. Saline Lakes: Springer; p. 29–67.
- Xiao J, Si B, Zhai D, Itoh S, Lomtatidze Z. 2008. Hydrology of Dali lake in central-eastern Inner Mongolia and Holocene East Asian monsoon variability. J Paleolimnol. 40(1):519–528.
- Xu H, Paerl HW, Qin B, Zhu G, Gaoa G. 2010. Nitrogen and phosphorus inputs control phytoplankton growth in eutrophic Lake Taihu, China. Limnol Oceanogr. 55(1):420–432.
- J-y XU, Y-T LI, Tian Z-J, Q-S LI. 2001. Investigation and research on phytoplankton in lake and marsh of Song-Nen plain. Water Resour Hydropower Northeast China. 12:018.
- Yang M, Bi Y, Hu J, Zhu K, Zhou G, Hu Z. 2011. Seasonal variation in functional phytoplankton groups in Xiangxi Bay, Three Gorges Reservoir. Chin J Ocean Limnol. 29(5):1057.
- Yu H-X, Wu J-H, Ma C-X, Qin X-B. 2012. Seasonal dynamics of phytoplankton functional groups and its relationship with the environment in river: a case study in northeast China. J Freshwater Ecol. 27(3):429–441.
- Zhang Y, Liang L, Jiang P, Li D, Lu C, Sun X. 2008. Genome evolution trend of common carp (Cyprinus carpio L.) as revealed by the analysis of microsatellite loci in a gynogentic family. J Genet Genom. 35(2):97–103.