Abstract
The succession of phytoplankton functional groups and environmental variables were investigated from January to December 2018 in a shallow subtropical lake, East Taihu Lake, located in the Yangtze Delta of China. Altogether, 125 genera, 21 functional groups, and 7 predominant functional groups, D (Fragilaria acus, Synedra acus, and Nitzschia spp.), P (Fragilaria capucina and Aulacoseira granulate), MP (Oscillatoria spp., Diploneis ovalis, Gomphonema angustatum, Nitzschia palea, and Achnanthes spp.), Y (Cryptomonas ovata, Cryptomonas erosa, Chroomonas acuta, and Ceratium spp.), H2 (Anabaena spp.), M (Microcystis spp.), and W1 (Euglenoids spp.), were identified, and these predominant functional groups exhibited strong seasonal variations. Groups Y and W1 dominated from winter to spring when temperature and light were low. The external pollution load and high levels of nutrients entered the lake in June, allowing group M to become dominant, even ultimately triggering Microcystis blooming. Meanwhile, the abundance of aquatic plants in summer, combined with high levels of transparency and light availability, resulted in Group M being replaced by Group P and then Groups M and H2 rebounding in autumn. The redundancy analysis revealed that water temperature, nutrients, and light availability (Zeu/Zmix) were the key factors promoting phytoplankton succession. The ecological assessment results demonstrated a good ecological status based on the assemblage index (Q average = 3.0). This work emphasizes the fact that temperature, nutrients, and light were the driving factors for phytoplankton dynamics, and aquatic plants and external pollution were important external factors for the succession of phytoplankton.
Introduction
Lakes are complex ecosystems that bear important functions of shipping transport, drinking water source and aquaculture. In recent years, because of global warming, the decline of submerged plants and the input of nutrients may be affecting the succession of phytoplankton, lead to algae blooms, and threaten the health of the lake and drinking water supply (Zhang et al. Citation2011; Wilken et al. Citation2018). Phytoplankton are the primary producers in aquatic ecosystem (Tian et al. Citation2015; Strock et al. Citation2019) and their composition, community structure, and changes in abundance directly affect water quality, energy flow, material flow (Li et al. Citation2013), and changes in the biological resources of the system (Wu et al. Citation2014). However, excessive algal blooms may reduce the transparency and stress on aquatic plants, putting lakes at risk of transition from grassy to algal turbidity, which will reduce water quality (Knight Citation2000). Therefore, it is important to understand the structure, succession, and driving factors of phytoplankton communities.
Phytoplankton growth in aquatic ecosystems is limited by various factors, such as water temperature (WT), nutrients, pH, light availability, and grazing pressure (Lewis and Wurtsbaugh Citation2008; Xia et al. Citation2011; Jiang et al. Citation2014; Cao et al. Citation2018). Many studies have reported that WT can directly affect the growth rate of phytoplankton (Pan et al. Citation2014), and different phytoplankton have different tolerance ranges to WT. For example, the abundance of filamentous cyanobacteria was found to respond positively to temperature increase (Sylwia et al. Citation2019). It is generally considered that cyanobacteria are adapted for living at high temperatures, but research in recent years has found that the growth cycle of cyanobacteria is prolonged, and there is an outbreak risk even in winter (Ho et al. Citation2019). Some reports have suggested that nutrients are the limiting factors that affect phytoplankton (Zhao et al. Citation2019), and the occurrence of many cyanobacteria blooms is mainly caused by an increase of nutrients (Paerl et al. Citation2011). A study of Taihu Lake found that phosphorus limits existed in winter and spring, while nitrogen limits were shown in summer and autumn (Xu et al. Citation2010), indicating the importance of controlling nutrients in lake management.
In addition, macrophytes are also considered to be a core factor controlling phytoplankton. Studies have shown that macrophytes could influence phytoplankton dynamics by competing for nutrients (Pinto and O'Farrell Citation2014), harboring zooplankton, (Gebrehiwot et al. Citation2017) and though allelopathy (Sabine and Elisabeth Citation2008). Scheffer and Carpenter (Citation2003) have suggested that a multi-stable state is possible in shallow lake ecosystems. Under low nutrient conditions, the lake was in the steady state of clear water dominated by submerged plants, whereas it was in the steady state of turbid water dominated by algae under high nutrient conditions, and these two states coexisted under mesotrophic conditions. However, the factors that drive the composition and distribution of phytoplankton communities are not yet well understood for lakes in a state of grass and algae dynamic equilibrium.
In recent years, the concept of phytoplankton functional groups has been increasingly used in the study of algal ecology (Xu et al. Citation2010; Birk et al. Citation2012). Because the main basis for the division of functional groups is by the similarity of phytoplankton, including the morphological and habitat adaptation characteristics, this not only simplifies the study of the phytoplankton community but also accurately demonstrates the relationship between phytoplankton and environmental factors (Becker et al. Citation2009). Judit et al. (Citation2006) applied phytoplankton functional groups to the evaluation of water environmental quality and proposed the Q index that was based on the influence factors of functional groups in different water types, determined the values (between 0 and 5) that each functional group represents, and then calculated the total value of all the coexisting functional groups by weighted average. This method of evaluating water quality based on the Q index has been widely used, both in the temperate regions (Tian et al. Citation2012), in which it was originally developed, and the subtropical (Santana et al. Citation2017) and tropical (Gebrehiwot et al. Citation2017; Rodrigues et al. Citation2018) regions.
East Taihu Lake is a long and narrow lake in the southeast of Taihu Lake that has maintained good water quality due to a rich aquatic plant community. In recent years, the harvesting of aquatic plants has resulted in a significant reduction of these aquatic plants and in frequent algae blooms (Yang et al. Citation2020). It is in a state of transition from clear water with aquatic plants to turbid water in which there is phytoplankton (Zeng et al. Citation2018). Furthermore, the water quality of East Taihu Lake is significantly affected by the inflow water of Taihu Lake, because phytoplankton is habitually affected by external pollution. However, there have been few studies on the changes of the phytoplankton community and their driving factors in East Taihu Lake. This study is the first to reveal the dynamics of the ecological status of the lake using phytoplankton functional groups and the Q index analysis methods. We hope that this study will provide important insights into the succession process of phytoplankton species in large shallow lakes.
On the basis of previous studies on changes in phytoplankton and environmental factors, we speculate that there are succession patterns in the dynamic changes of phytoplankton communities. Nutrients and light availability are the main driving factors, and the external factors of aquatic plants and external pollution control the phytoplankton dynamics in the lake. To confirm our hypothesis, we conducted a one-year survey of the environmental factors and the changes in the phytoplankton community. This study aims (1) to explore environmental changes such as water quality impact factors in different functional areas of East Taihu Lake, (2) to reveal the variations in phytoplankton groups in response to different environmental stressors, and (3) to evaluate the ecological status in East Taihu Lake using the functional groups approach and the Q index.
Materials and methods
Study area
East Taihu Lake is a large, shallow lake bay in the southeast of Taihu Lake (30°58′–31°07′ N; 120°25′–120°35′ E), which is located in the Yangtze Delta of China; it has an area of approximately 130 km2, accounting for 5.5% of the total area of Taihu Lake. The total length of the lake is 27.5 km, and the maximum width is 9.0 km. The average depth of East Taihu Lake is 1.2–1.3 m, and the water storage capacity is 1.18 × 108 m3. East Taihu Lake is connected to West Taihu Lake by a narrow lake and has important functions such as water storage, flood drainage, and water supply. It has become the main source of water for Suzhou and other areas.
Ten sampling sites were chosen according to the functions of East Taihu Lake and different utilization modes (). The entrance areas (S1, S2) consist of the water inlet of East Taihu Lake that is connected to West Taihu Lake; these have the characteristics of large flow and large external pollution. There are few aquatic plants in the aquatic plant areas (S3, S4) and almost no aquatic plants in the anhydrous plant areas (S5, S6); aquaculture is one of the important functions of East Taihu Lake. There are approximately 225 hectares of culture areas in the lake, the aquaculture areas (S7, S8) are rich in aquatic plants, with high transparency and small water flow; the feeding of bait is through the nutrient input of East Taihu Lake. The wetland and reservoir areas (S9, S10) have good water quality, with aquatic plant coverage reaching 75%–100% (Yang et al. Citation2020). The characteristics of each of the functional areas are shown in .
Figure 1. Sampling sites and location of East Taihu Lake. The picture above is a processed Landsat 8 remote sensing image. The blue color represents the water, and the yellow color represents the floating leaves or emergent macrophytes.
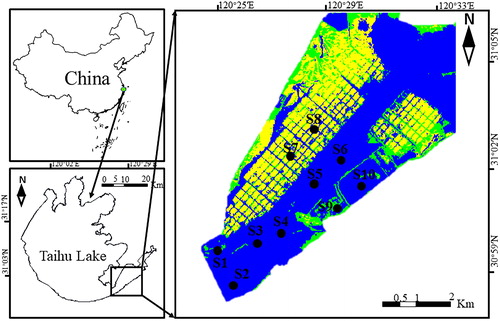
Table 1. Definition of the functional areas and characteristics in East Taihu Lake.
Sample and analysis
Surface water samples (0.5 m) were collected in 500 mL plastic containers 12 times from January to December in 2018 and were transferred to the laboratory for analysis within 24 h. Standard methods were used to analyze the total phosphorous (TP) and total nitrogen (TN) in the raw water. Nitrate (NO3−), phosphate (PO43 and ammonium (NH4+) were analyzed using samples filtered through 0.45 μm glass microfiber filters (Whatman GF/C). All the nutrients were measured using a UV–VIS spectrophotometer (UV-2450, Shimadzu, Japan). Chlorophyll a (Chl-a) was measured using a Phyto-PAM (pulse amplitude modulated fluorometer) and analyzed with Win-Control software (Heinz Walz GmbH, Effeltrich, Germany), which was calibrated with a standard configuration file, the measurement frequency being 32 (Gera et al. Citation2012; Frenken et al. Citation2016).
We performed in situ measurements on the WT, pH, dissolved oxygen (DO), and electrical conductivity (EC), using a HACH multiparameter meter (HQ40D, Hach, USA). The transparency was measured using a standard Secchi disk, also described as a Secchi disk depth (SD). The turbidity was measured using a nephelometer instrument (1900 C, Hach, USA).
Phytoplankton analysis
Surface water samples (0.5 m) were collected every month for identification and analysis of the phytoplankton at 10 sampling sites in East Taihu Lake. The phytoplankton were repeatedly collected in an “∞” shape on the water surface with a 64 μm-pore-size net and were stabilized in situ with Lugol's iodine solution (1.5% v/v) for qualitative analysis. The 500 mL surface water samples were collected using a water sampler for quantitative analysis and were stabilized in situ with Lugol's iodine solution (1.5% v/v), transferred to the laboratory, and concentrated by standing to 50 mL. The algae were enumerated using a microscope (CX21, OLYMPUS, Japan) with a 0.1 mL counting box containing 100 horizons at 10 × 40 magnification. The taxa were classified according to (Edlund Citation2003).
Statistical analysis
Principal components analysis (PCA) was used to analyze the chemical and physical changes on spatial and temporal levels; the WT, DO, EC, pH, TN, TP, NO3−, NH4+, PO43−, and Chl-a were included. Variance analysis (one-way ANOVA) was used to compare the means. Non-parametric correlation (Spearman) analyses were used to test the relationships among the environmental variables, and Bonferroni's method was used for multiple comparison correction. The statistical analysis was performed using the SPSS 22.0 statistical software package, and the level of significance used was P < 0.05 for all tests.
According to the method of Jensen et al. (Citation1994), the ratio between the eutectic depth and the mixing depth (Zeu/Zmix) was used as a measure of light utilization. The euphotic zone (Zeu) was calculated from the Secchi depth (Zeu = 1.7 Secchi depth) (Jensen et al. Citation1994). The mixing zone (Zmix) was defined as the water column mean depth where there was no temperature gradient and as the zone from the surface to the depth where the temperature gradient exceeded 1 °C/m3 in the lake.
The algae cell volume was determined using the geometry configurations, and the biomass was calculated on the basis of the cell density. The species with a total biomass >5% were combined into functional groups (Reynolds et al. Citation2002). The Q index was used to evaluate the ecological status of the lake, and the F factor of each functional group was based on previous studies conducted in Taihu Lake (Wang et al. Citation2011). Redundancy analysis (RDA) was performed by multivariate ordination using CANOCO version 5.0, following Terbraak (Citation1986), to examine the relationship between the physical/chemical parameters and the structure of the phytoplankton assemblage.
Results
Spatial and temporal patterns in the environmental factors
The means and ranges of the environmental variables at the 10 sampling sites in East Taihu Lake are presented in . The WT showed similar seasonal patterns in the whole lake, with a maximum temperature of 32.25 °C in August and a minimum temperature of 7.05 °C in January. The water from all the sampling sites was typically alkaline, with pH values varying from 7.44 to 9.32. The EC and DO concentrations ranged from 336 to 500 μS/cm and from 3.3 to 12.2 mg/L, respectively. The SD was relatively low in spring and winter, and the SD, at sites P4 and P5, was significantly higher than in other areas (ANOVA, P < 0.05).
Table 2. Means and ranges of environmental variables at the 10 sampling sites in East Taihu Lake (Jan–Dec 2018, n = 120).
With regard to the trophic condition of East Taihu Lake, the water quality of Taihu Lake greatly affected the nutrient level of the lake. The TN and TP concentrations covered a wide range (). The TN concentration reached its lowest value in autumn (0.19 mg/L) and its highest value in spring (2.92 mg/L); the TN showed significant differences in different seasons (ANOVA, P < 0.05), but the difference was not obvious in space. The NO3− and TN showed similar seasonal variations; the average concentrations of NO3− were higher in the spring (1.259 mg/L). The TP was significantly lower at sites P4 and P5 than in other areas, and there was a significant difference in different seasons, with the order of spring > winter > autumn > summer (). The concentration of PO43− ranged from 0.001 to 0.049 mg/L, with a mean value of 0.018 mg/L, and the seasonal trend was obvious where the PO43− concentration reached its lowest value in spring and its highest value in winter. For NH4+, the value was lower in spring and summer and increased markedly in autumn and winter. During the study period, the ratio of nitrogen to phosphorus in East Taihu Lake varied widely. The ratio reached its maximum in spring (TN/TP = 29.4) and its minimum in winter (TN/TP = 4.0). The nutrients were positively correlated with the DO, TSS, Chl-a, and WT, and negatively correlated with the Zeu ().
Figure 2. Seasonal variation of nutrients and Chl-a concentration in five functional areas of East Taihu Lake.
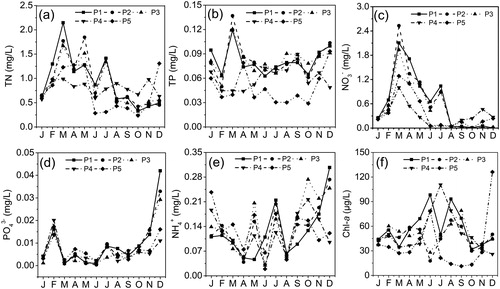
Table 3. Significant Spearman correlations among 12 environmental variables in the studied area (n = 120).
The Chl-a concentration is a very important indicator of phytoplankton biomass and distribution. In our study, Chl-a showed significant differences between different seasons (ANOVA, P < 0.05). The concentration of Chl-a increased from spring to summer, and the average concentration of Chl-a in spring was 45.49 μg/L. The concentration at site P1 showed a gradual upward trend and reached the highest value in summer (mean value = 55.50 μg/L), and high Chl-a concentrations were observed at site P1 (98.21 μg/L) and site P4 (110.6 μg/L). In autumn, Chl-a showed a decreasing trend., especially in at site P5, where it reached the lowest value; then, the Chl-a at site P5 increased rapidly, reaching a peak in December (126.06 μg/L), whereas other areas showed a decreasing trend. The Chl-a concentration was negatively correlated with the Zeu and was positively correlated with the TP ().
According to the Zmix changes, East Taihu Lake is in a mixed state throughout the year. The availability of light is an important indicator of phytoplankton selection. We used the Zeu/Zmix ratio to describe this. The euphotic zone (Zeu) values ranged from 0.37 to 1.74 m, with the highest value in autumn and the lowest value in spring (). In the study period, light reached 37%–68% of the mixing zone; the availability of light increased from spring to early summer, reaching 68% in October and then decreasing to 40% in winter (). The SD varied greatly in different functional areas; the SD at sites P4 and P5 were significantly high, especially in summer and autumn. However, the differences in other areas were not significant, and the overall transparency was low (SD < 0.5 m). These results indicate that the lighting conditions in different functional areas of East Taihu Lake alter significantly. The Zeu was negatively correlated with the DO and the nutrients and positively correlated with the WT ().
Figure 3. Seasonal variation of the mixing zone (Zmix), euphotic zone (Zeu), and the Zeu/Zmix ratio in East Taihu Lake.
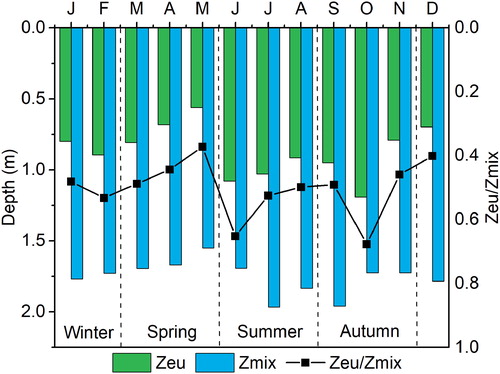
In this study, PCA was performed to explain the variance of the physical and chemical variables; 11 variables were used for East Taihu Lake. The result showed that four principles accounted for 73.1% of the data variability. Specifically, PC1 accounted for 33.5% of the data variability, and the TP (0.73), SD (−0.78), NTU (0.92), and the TSS (0.93) were the most important variables related to this principal component. PC2 accounted for 16.8% of the data variability, and pH (0.63), WT (−0.82), and DO (0.89) were the most important variables. PC3 accounted for 12.8% of the data variability, and the TN (0.75) and the NH4+ (0.86) were the most important variables. PC4 accounted for 10.1% of the data variability, and the EC (0.69) was the most important variable (). The PCA results illustrated that PC1 reflected the eutrophic state, which was largely determined by the TP, SD, and Chl-a, and a positive value in PC1 represents a higher TP and Chl-a, and a lower SD. PC2 reflected the influence of the seasonal gradient on the water environment.
Phytoplankton functional groups characteristics
In this study, the 125 taxa identified were members of seven major taxonomic categories, whereas Chlorophyta were found to have the highest number of taxa (46), followed by Bacillariophyta (35), Cyanophyta (32), Euglenophyta (4) and Cryptophyta (4), Pyrrophyta (3), and Chrysophyta (1). According to the Mcnaughton dominance index (Y index) calculation, the dominant species of phytoplankton were as follows: Microcystis spp. (Y = 0.40), Anabaena spp. (Y = 0.11), Pseudanabaena spp. (Y = 0.07), Chroomonas acuta (Y = 0.02), and Aulacoseira granulata (Y = 0.02).
Referring to the phytoplankton functional groups proposed by Reynolds et al. (Citation2002), the species appearing in the 10 samples collected in East Taihu Lake were grouped into 21 functional groups (), namely, A, C, D, P, MP, T, S1, SN, X2, X1, Y, E, F, G, J, K, H1, H2, L0, M, and W1. The species with a relative biomass >5% in each sample were defined as the dominant functional groups, and seven dominant groups were respectively identified: D, P, MP, Y, H2, M, and W1.
Table 4. Phytoplankton taxa and their functional groups in East Taihu Lake.
shows the seasonal changes of the seven dominant functional groups in different functional areas in East Taihu Lake. The single-peak pattern of the total biomass of the phytoplankton biomass in the one-year cycle is clear. The average biomass was 6.37 mg/L, reaching a maximum of 69.43 mg/L in August and September, and the lowest biomass of 2.91 mg/L appeared in December (). In spring, Groups W1, H2, MP, and M were the main functional groups, and the relative abundances of biomass were 28.71%, 21.93%, 17.1%, and 13.12%, respectively. In summer, with the increasing temperature, the biomass of East Taihu Lake reached its peak in various functional areas. Groups M and P rapidly increased to become dominant functional groups, and the relative abundance of the biomass reached 24.02% and 27.3%. The composition of the functional group at site P1 was relatively single (), the relative abundance of Group M reached 97.45% in June, and then Group P replaced Group M, and the relative abundance reached 75.8%. The high biomass of Group P was observed in other regions, and the high biomass of Group W1 was found at site P5 (), the functional group consisted of Groups P, MP, M, and H2, and the relative abundances of the biomass were 21.33%, 11.4%, 13.14%, and 18.16% at site P4 (), respectively. In the early autumn, Group P remained in a dominant position at sites P1, P2, and P3, subsequently, the phytoplankton biomass decreased rapidly, and Groups Y and D showed an upward trend at sites P3 and P4. The biomass of the phytoplankton at site P4 maintained a high level in the autumn, Group M once again dominated with the relative abundance of the biomass reaching 63.5%, and the high biomass of Group H2 was found at site P5. In winter, the biomass of the phytoplankton was habitually low; Group Y gradually became the main functional group, especially at site P4, with the relative abundance of the biomass reaching 63.18% and the high biomass of Groups MP and W1 being found at site P1. The biomass of the phytoplankton was very low at sites P2, P4, and P5.
Water quality assessment
According to Carlson's Trophic State Index (TSI), the comprehensive eutrophication index indicates the following: 0–30 oligotrophic, 30–50 mesotrophic, 50–60 light eutrophic, 60–70 middle eutrophic, and 70–100 hypereutrophic. The TSI value showed a significant regional difference that was significantly lower at site P5 than in other areas. The TSI showed as mesotrophic in early autumn, with a mean value of 45.6 (), but the eutrophication was higher in winter and spring, reaching light eutrophic, which may be related to aquatic plant decline. Although there were large numbers of aquatic plants at site P4, its eutrophication level was light eutrophic, which may have been related to the increase of nutrients caused by the aquaculture. At sites P1, P2, and P3, the level of eutrophication was high, even reaching middle eutrophic; this was inextricably linked to the influence of the external water and loss of aquatic plants.
Figure 6. Changes of the Trophic State Index (TSI) value (a) and the Q index (b) in different parts of East Taihu Lake.
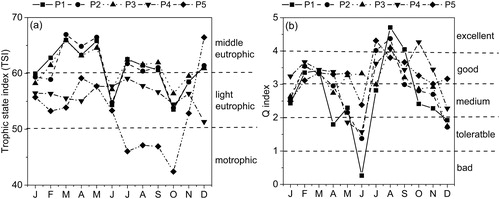
The Q index has been used to assess the trophic status of lakes and reservoirs (Judit et al. Citation2006; Padisák et al. Citation2009). The Q index (0–5) indicates the following: 0–1 bad, 1–2 tolerance, 2–3 medium, 3–4 good, and 4–5 excellent. Generally, the smaller the Q index, the higher the eutrophication of the water. We used the Q index method to evaluate the ecological condition of East Taihu Lake. The results showed that the highest Q index (4.71) occurred in autumn, the lowest Q index (0.26) occurred at site P1 (in June), and there was a mean value of 3.0, indicating medium conditions in East Taihu Lake ().
Discussions
Ecological conditions and succession of phytoplankton functional groups in East Taihu Lake
Seasonal succession of phytoplankton composition in lakes shows some patterns. Sommer et al. (Citation2012) developed a plankton ecology group (PEG) model that describes the seasonal succession of phytoplankton assemblages in lakes. According to the model, the seasonal succession of the phytoplankton community is probably from cryptophytes and bacillariophytes in winter and spring to chlorophytes in summer; cyanobacteria dominate in late summer and early autumn, and the number of diatoms rises again in autumn; the model mainly reflects the situation of deep-water lakes with the mesotrophic level. East Taihu Lake is a large, shallow lake with aquatic plants; the seasonal succession of the phytoplankton showed similar patterns to the PEG model but was not completely consistent.
In this study, Groups Y and D were the main functional groups in winter. According to the PEG model, bacillariophyta and cryptophytes dominate when nutrients are available, with overwintering leading to low temperatures. The RDA showed a negative correlation between Groups Y and D and Group W1. The study found that Group Y favored water with high inorganic turbidity (Wang et al. Citation2018). The dominance of bacillariophyta and cryptophytes in winter should therefore be attributed to the low temperatures and high turbidity. The current results are consistent with studies (Xu et al. Citation2011; Zeng et al. Citation2018) that were conducted in Chinese lakes and reservoirs. In spring, with the increasing temperature and zooplankton grazing pressure, large euglenophytes, such as Group W1, replaced Group Y, and it was notable that the biomass of Group H2 accounted for 53.1% at site P4, which may have been due to the high DO. The biomass of chlorophytes is very low in East Taihu Lake, differing from the PEG model. In the early summer, with the outbreak of cyanobacteria in Taihu Lake (Deng et al. Citation2019) and under the influence of hydrological conditions, cyanobacteria enter East Taihu Lake and gather in large numbers, affecting the water quality of East Taihu Lake, especially at site P1. Group M occupied an absolute dominant position; the absolute advantage of Group M had limited the growth of other functional groups. In summer and autumn, these seasonal variations of the phytoplankton functional groups observed in this study agree well with the PEG model of freshwater systems. Under strong light and at high temperatures in summer, environmentally friendly functional groups were selected, such as Groups MP and P (Cao et al. Citation2018). As the temperature decreased and the nutrients rebounded again in autumn, Groups M and H2 became the main functional groups again.
The Functional Group Assemblage Index (Q index) was originally based on the Water Framework Directive (Richter et al. Citation2013) proposed by the European Community to assess the ecological status of different types of lakes. Compared with the TSI, the Q index has a higher resolution and a better biological explanation and can better evaluate the environmental quality of a lake. The method for evaluating water quality based on the phytoplankton functional group Q index has been well applied in both the initially developed temperate regions (Tian et al. Citation2012) and subtropical (Santana et al. Citation2017) and tropical (Gebrehiwot et al. Citation2017; Rodrigues et al. Citation2018) regions, proving that it is a useful tool in the evaluation process. In this study, we evaluated the nutritional status of East Taihu Lake. Throughout the 2018 study period, eutrophication appeared to be moving in a good direction, and the TSI results indicated that the nutritional status was light eutrophic. The evaluation of the Q index based on the phytoplankton functional groups indicates that the nutritional status of East Taihu Lake was medium. However, the ecological status at site P1 in summer (June) was bad, and this may have been due to the large amount of cyanobacteria brought by the water from Taihu Lake; the contribution of Group M caused the ecological environment to deteriorate.
Driving factors of phytoplankton succession
In lake ecosystems, the hydrologic regime is considered as one of the main environmental forces that affect phytoplankton dynamics (Zhu et al. Citation2013), and the availability of resources for algae, such as light, nutrients, and WT, are the main variables in determining phytoplankton assemblages (Becker et al. Citation2010; Crossetti et al. Citation2013). The hydrologic regime affects the algal dynamics mainly across the longitudinal gradient (Cao et al. Citation2018). A mixed water column was observed in East Taihu Lake throughout the year, indicating that hydrological conditions had little effect on the algae across the longitudinal gradient. Furthermore, East Taihu Lake is a sack-shaped lake that is rich in aquatic plants and connected to Taihu Lake; the inflow of water from Taihu Lake and the competition of aquatic plants might be the key factors affecting the dynamics of the phytoplankton functional groups in East Taihu Lake.
In the study, there were significant spatial and temporal differences in the nutrients of East Taihu Lake. The TN and TP concentrations were significantly higher in spring than in other seasons, and, because of rainfall in summer, the nutrients decreased significantly. Additionally, the TN/TP ratios are also widely used to explain the nutrient limitations of total phytoplankton growth, where N is limiting when TNTP < 9, and P is limiting when TNTP > 22 (Bulgakov and Levich Citation1999; Sondergaard et al. Citation2013). East Taihu Lake showed an N limit only in autumn (TNTP mean = 7.75), a strong P limit in spring at site P5 (TNTP = 27.67), and an N limit at sites P1, P2, and P3 in autumn and winter (TNTP = 6.15). Although the ratios are a consequence of the uptake and not the drivers of changes in phytoplankton composition, the results indicate the interaction between the phytoplankton and nutrients.
The phytoplankton biomass of East Taihu Lake presented a single-peak pattern, reaching its peak in August and September and its lowest in winter; this was similar to the trend of temperature change, indicating that temperature played an important role in phytoplankton changes in East Taihu Lake. Many studies have shown that WT has a great effect on the abundance succession of phytoplankton and is one of the most important environmental factors in determining phytoplankton composition (Berger et al. Citation2014; Tian et al. Citation2018). The reports of Xu et al. (Citation2011) on Taihu Lake showed that WT plays a leading role in the dynamics of phytoplankton, and studies on other lakes have also shown that temperature is a key factor driving changes in phytoplankton community composition (Leitão et al. Citation2003; Tian et al. Citation2012). In our study, the results of the RDA indicated that WT was the most important factor affecting the phytoplankton changes in East Taihu Lake, with the dominant functional Groups M, MP, and H2 showing a positive correlation with the temperature ().
Figure 7. Ordination diagram from the RDA of the relationship between the environmental variables (hollow arrows) and the biomass of phytoplankton functional groups (solid arrows).
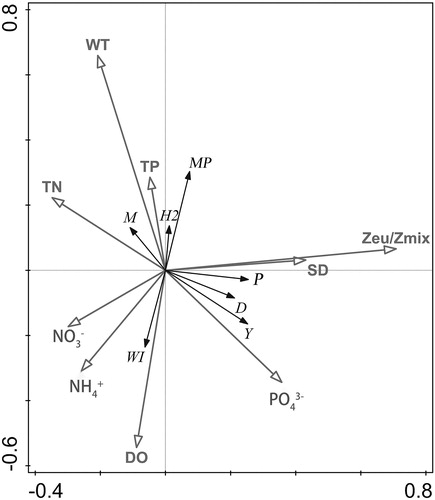
Group M (Microcystis spp.) was the most important functional group in Cyanophyta and is characterized by being found in small- to medium-sized lakes that are eutrophic to hypertrophic; it easily forms blooms in eutrophic water bodies (Harke et al. Citation2016). Studies have shown that high temperatures, increased phosphorus concentrations, and light and water exchange are responsible for the dominance of Group M (Yoshinaga et al. Citation2006). In our study, Group M was found in summer and autumn, reaching the highest relative biomass at site P1 in June. The RDA results showed that Group M was closely related to the WT and the TN (), but the TN concentration was low, and there was no nutrient limitation in June, suggesting that the TN was not the cause of the Group M outbreak in East Taihu Lake. These results proved that WT was the main factor affecting Group M.
Group MP mainly includes Oscillatoria spp., Diploneis ovalis, Gomphonema angustatum, Nitzschia palea, and Achnanthes spp., which are characterized by the occurrence of frequent agitation and inorganic turbid shallow lakes (Padisák et al. Citation2009). Studies have shown that the distribution of Group MP is related to high and low temperature periods (Tian et al. Citation2018). It had a high relative biomass at high temperatures, mainly including cyanobacteria (Oscillatoria spp.), and, at low temperatures, it has diatoms (Diploneis ovalis, Gomphonema angustatum, Nitzschia palea, and Achnanthes spp.). This was consistent with our findings. Group MP occurred mainly in early spring and summer, and there was no nutrient limitation in East Taihu Lake. In spring, low temperatures and high nutrients made Group MP of diatoms become a major functional group. As the temperature increased and the nutrient concentration decreased, Group MP changed from diatoms to cyanobacteria. The presence of Group MP was also reported in Luoma Lake (Tian et al. Citation2018) and Taihu Lake (Yingfei et al. Citation2019).
Group H2's (Anabaena spp.) habitat is a shallow lake with moderate nutrition and good light conditions. Group H2 was reported to be tolerant of low N concentrations and was sensitive to mixed, low light, and low P concentrations (Padisák et al. Citation2009). Group H2 accounted for only a small part of the total biomass, but it easily formed blooms in aquatic ecosystems and produced algal toxins, and their ecological effects could not be ignored. The results of the RDA showed that Group H2 had a positive correlation with the TP and WT and a negative correlation with the DO in East Taihu Lake. The low DO in summer was also caused by the high WT. Combined with the high WT and high TP, Group H2 was popular in spring and autumn. With the heavy rainfall in summer, the nutrients decreased rapidly, and the growth of Group H2 was restricted. In autumn, the biomass of Group H2 rebounded, which may have been related to the decline of aquatic plants and the decrease in rainfall. Despite the high TP concentration in winter, the low WT restricted the growth of Group H2. These results indicated that the WT and TP were limiting factors for the phytoplankton in the lake.
The RDA results also revealed the importance of other environmental changes to phytoplankton growth, such as the availability of light. Light availability, described by the Zeu/Zmix ratio, was very high in East Taihu Lake, especially at sites P4 and P5. Although the nutrients were much lower at site P4 than in other areas, the phytoplankton abundance and Chl-a were much higher, indicating that light was a limited resource in the lake. Many studies have shown that the availability of light plays an important role in the succession of seasonal phytoplankton (Béchet et al. Citation2013; Singh and Singh Citation2015). The correlation analysis showed that seasonal variation and particulate matter in the water column might strongly affect the Zeu value () and thus affect the light utilization of phytoplankton functional groups. This provided useful insights for the importance of TSS settlement to the availability of light in drinking water systems.
In our study, the availability of light might have become a limiting factor for certain phytoplankton functional groups. It was believed that under low grazing pressure, functional groups that can tolerate low temperatures and low light in winter were selected, such as Group Y. The advantage of Group Y well explained the maximum biomass observed in January. With the increase in temperature and the increase of available light in summer, functional groups suitable for high light intensity were selected, for example, Group P. The RDA showed that East Taihu Lake's Group P was positively correlated with the SD and Zeu/Zmix. Group P includes diatoms (Fragilaria capucina and Aulacoseira granulata) that are usually found in low-latitude lakes and can live in eutrophic water with moderate light (Reynolds et al. Citation2002; Padisák et al. Citation2009). Some studies have shown that transparency and radiation are the main environmental factors that affect Group P (Tian et al. Citation2018; Wilken et al. Citation2018; Varol Citation2019), which was, highly consistent with our RDA results. The transparency of East Taihu Lake was significantly high, and the Zeu/Zmix reached two peaks in summer and autumn, which may have been due to the contribution of the large-scale growth of aquatic plants to the transparency. Studies have shown that aquatic plants have a positive effect on transparency (Hestir et al. Citation2016; Gebrehiwot et al. Citation2017). Many small-scale experiments have shown that aquatic plants can reduce turbidity by decreasing sediment resuspension and water movement (Austin et al. Citation2017), which is consistent with our results. These results highlighted the importance of light availability to the phytoplankton community composition and structure.
The RDA results showed that the DO was also an important factor affecting the phytoplankton, and Group W1 was positively correlated with the DO (). Group W1 mainly included euglenophyta that can tolerate high biological oxygen demand but are sensitive to grazing (Padisák et al. Citation2009). Previous investigations have reported that euglenophyta can tolerate a low DO (Oliveira and Calheiros Citation2000). Our results show that Group W1 mainly occurred in spring, and the correlation analysis showed that temperature was negatively correlated with the DO (), indicating that temperature was a key factor affecting the phytoplankton community; the increase of DO concentration in spring promoted the growth of Group W1.
Conclusions
To summarize, the study of water environmental conditions and phytoplankton dynamics in a shallow lake with an area dominated by either macrophytes or phytoplankton provides insight into the understanding of phytoplankton's response to environmental changes. The study demonstrated that significant differences of water quality resulted from the functional use of the lake. Water quality in East Taihu Lake was largely constrained by the raw water condition from Taihu Lake. The establishment of macrophytes plays an important role in improving the water quality, such as reducing nutrients, improving water transparency, and controlling the growth of phytoplankton in East Taihu Lake. The phytoplankton analysis revealed that Microcystis spp. were the most dominant taxa belonging to Cyanophyta. Seasonal trends of phytoplankton composition patterns and abundance were different among the five functional areas. The RDA indicated that WT and light were the most important factors for phytoplankton succession in this lake, followed by the TP and the TN. The functional groups explained the water condition well, and the assemblage index indicated that the ecological status was good for most of the year. The information provided in this study could be useful for drinking water source protection and shallow lake restoration against eutrophication.
Author contributions
Jing Nan and Changtao Yang contributed the central idea and analyzed most of the data. Jing Nan wrote the dissertation. Jianhua Li gave guidance in experimental design and writing. Huaiyong Yu assisted the experiment and data analysis.
Disclosure statement
No potential conflict of interest was reported by the author(s).
Additional information
Funding
Notes on contributors
Jing Nan
Jing Nan is a Ph. D student from College of Environmental Science and Engineering, Tongji University, and focus on the aquatic ecology and phytoplankton in lake.
Jianhua Li
Jianhua Li is a professor in Environmental Science and Engineering, Tongji University. He is working on the ecological health of lakes and rivers, his interests include aquatic biology, cyanobacteria blooms, and health evaluation.
Changtao Yang
Changtao Yang is a Ph. D student from College of Environmental Science and Engineering, Tongji University, working on the aquatic plants in lake.
Huaiyong Yu
Huaiyong Yu is a MS student from College of Environmental Science and Engineering, Tongji University, focus on Dissolved Organic Matter (DOM) in lake.
References
- Austin ÅN, Hansen JP, Serena D, Eklöf JS, Arthur LS. 2017. Relationships between aquatic vegetation and water turbidity: A field survey across seasons and spatial scales. Plos One. 12(8):e0181419.
- Béchet Q, Shilton A, Guieysse B. 2013. Modeling the effects of light and temperature on algae growth: State of the art and critical assessment for productivity prediction during outdoor cultivation. Biotechnol Adv. 31(8):1648–1663.
- Becker V, Caputo L, Ordóñez J, Marcé R, Armengol J, Crossetti LO, Huszar VLM. 2010. Driving factors of the phytoplankton functional groups in a deep Mediterranean reservoir. Water Res. 44(11):3345–3354.
- Becker V, Huszar VLM, Crossetti LO. 2009. Responses of phytoplankton functional groups to the mixing regime in a deep subtropical reservoir. Hydrobiologia. 628(1):137–151.
- Berger SA, Diehl S, Stibor H, Sebastian P, Scherz A. 2014. Separating effects of climatic drivers and biotic feedbacks on seasonal plankton dynamics: No sign of trophic mismatch. Freshw Biol. 59(10):2204–2220.
- Birk S, Bonne W, Borja A, Brucet S, Courrat A, Poikane S, Solimini A, van de Bund W, Zampoukas N, Hering D, et al. 2012. Three hundred ways to assess Europe's surface waters: An almost complete overview of biological methods to implement the Water Framework Directive. Ecol Indic. 18:31–41.
- Bulgakov NG, Levich AP. 1999. The nitrogen: phosphorus ratio as a factor regulating phytoplankton community structure. fal. 146(1):3–22.
- Cao J, Hou Z, Li Z, Chu Z, Yang P, Zheng B. 2018. Succession of phytoplankton functional groups and their driving factors in a subtropical plateau lake. Sci Total Environ. 631–632:1127–1137.
- Crossetti LO, Becker V, Cardoso L. d S, Rodrigues LR, da Costa LS, da Motta-Marques D. 2013. Is phytoplankton functional classification a suitable tool to investigate spatial heterogeneity in a subtropical shallow lake? Limnologica. 43(3):157–163.
- Deng J, Salmaso N, Jeppesen E, Qin B, Zhang Y. 2019. The relative importance of weather and nutrients determining phytoplankton assemblages differs between seasons in large Lake Taihu. Aquat Sci. 81(3):48.
- Edlund MB. 2003. Freshwater algae of North America. Ecology and classification. J Phycol. 39(3):624–625.
- Frenken T, Velthuis M, de Senerpont Domis LN, Stephan S, Aben R, Kosten S, van Donk E, Van de Waal DB. 2016. Warming accelerates termination of a phytoplankton spring bloom by fungal parasites. Glob Chang Biol. 22(1):299–309.
- Gebrehiwot M, Kifle D, Stiers I, Triest L. 2017. Phytoplankton functional dynamics in a shallow polymictic tropical lake: the influence of emergent macrophytes. Hydrobiologia. 797(1):69–18.
- Gera A, Alcoverro T, Mascaró O, Pérez M, Romero J. 2012. Exploring the utility of Posidonia oceanica chlorophyll fluorescence as an indicator of water quality within the European Water Framework Directive. Environ Monit Assess. 184(6):3675–3686.
- Harke MJ, Steffen MM, Gobler CJ, Otten TG, Wilhelm SW, Wood SA, Paerl HW. 2016. A review of the global ecology, genomics, and biogeography of the toxic cyanobacterium, Microcystis spp. Harmful Algae. 54:4–20.
- Hestir EL, Schoellhamer DH, Greenberg J, Morgan-King T, Ustin SL. 2016. The effect of submerged aquatic vegetation expansion on a declining turbidity trend in the Sacramento-San Joaquin River Delta. Estuar Coast. 39(4):1100–1112.
- Ho JC, Michalak AM, Pahlevan N. 2019. Widespread global increase in intense lake phytoplankton blooms since the 1980s. Nature. 574(7780):667–670.
- Jensen JP, Jeppesen E, Olrik K, Kristensen P. 1994. Impact of nutrients and physical factors on the shift from cyanobacterial to chlorophyte dominance in shallow Danish lakes. Can J Fish Aquat Sci. 51(8):1692–1699.
- Jiang Y-J, He W, Liu W-X, Qin N, Ouyang H-L, Wang Q-M, Kong X-Z, He Q-S, Yang C, Yang B, et al. 2014. The seasonal and spatial variations of phytoplankton community and their correlation with environmental factors in a large eutrophic Chinese lake (Lake Chaohu). Ecol Indic. 40(5):58–67.
- Judit P, Borics G, Grigorszky I, Soróczki-Pintér É. 2006. Use of phytoplankton assemblages for monitoring ecological status of lakes within the Water Framework Directive: The assemblage index. Hydrobiologia. 553(1):1–14.
- Knight T. 2000. Biology of lakes and ponds. Ecology. 81(1):286–287.2.0.CO;2]
- Leitão M, Morata SM, Rodriguez S, Vergon JP. 2003. The Effect of perturbations on phytoplankton assemblages in a deep reservoir (Vouglans, France). Hydrobiologia. 502(1–3):73–83.
- Lewis WM, Jr., Wurtsbaugh WA. 2008. Control of lacustrine phytoplankton by nutrients: Erosion of the phosphorus paradigm. Internat Rev Hydrobiol. 93(4–5):446–465.
- Li j, Qiu Y, Fei T, Liu Y, Wu G. 2013. Using remotely sensed suspended sediment concentration variation to improve management of Poyang Lake, China. Lake Reserv Manage. 29(1):47–60.
- Oliveira MDD, Calheiros DF. 2000. Flood pulse influence on phytoplankton communities of the south Pantanal floodplain, Brazil. Hydrobiologia. 427(1):101–112.
- Padisák J, Crossetti LO, Naselli-Flores L. 2009. Use and misuse in the application of the phytoplankton functional classification: a critical review with updates. Hydrobiologia. 621(1):1–19.
- Paerl HW, Xu H, McCarthy MJ, Zhu G, Qin B, Li Y, Gardner WS. 2011. Controlling harmful cyanobacterial blooms in a hyper-eutrophic lake (Lake Taihu, China): The need for a dual nutrient (N & P) management strategy. Water Res. 45(5):1973–1983.
- Pan B, Wang H, Wang H. 2014. A floodplain-scale lake classification based on characteristics of macroinvertebrate assemblages and corresponding environmental properties. Limnologica. 49:10–17.
- Pinto P, O'Farrell I. 2014. Regime shifts between free-floating plants and phytoplankton: a review. Hydrobiologia. 740(1):13–24.
- Reynolds CS, Huszar V, Kruk C, Naselli-Flores L, Melo S. 2002. Towards a functional classification of the freshwater phytoplankton. J Plankton Res. 24(5):417–428.
- Richter S, Völker J, Borchardt D, Mohaupt V. 2013. The Water Framework Directive as an approach for Integrated Water Resources Management: Results from the experiences in Germany on implementation, and future perspectives. Environ Earth Sci. 69(2):719–728.
- Rodrigues LC, Pivato BM, Vieira LCG, Bovo-Scomparin VM, Bortolini JC, Pineda A, Train S. 2018. Use of phytoplankton functional groups as a model of spatial and temporal patterns in reservoirs: a case study in a reservoir of central Brazil. Hydrobiologia. 805(1):147–161.
- Sabine H, Elisabeth MG. 2008. Can allelopathically active submerged macrophytes stabilise clear-water states in shallow lakes? Basic Appl Ecol. 9(4):422–432.
- Santana LM, Crossetti LO, Ferragut C. 2017. Ecological status assessment of tropical reservoirs through the assemblage index of phytoplankton functional groups. Braz J Bot. 40(3):695–704.
- Scheffer M, Carpenter SR. 2003. Catastrophic regime shifts in ecosystems: linking theory to observation. Trends Ecol Evol. 18(12):648–656.
- Singh SP, Singh P. 2015. Effect of temperature and light on the growth of algae species: A review. Renew Sust Energy Rev. 50:431–444.
- Sommer U, Adrian R, De Senerpont Domis L, Elser JJ, Gaedke U, Ibelings B, Jeppesen E, Lürling M, Molinero JC, Mooij WM, et al. 2012. Beyond the Plankton Ecology Group (PEG) model: Mechanisms driving plankton succession. Annu Rev Ecol Evol Syst. 43(1):429–448.
- Sondergaard M, Bjerring R, Jeppesen E. 2013. Persistent internal phosphorus loading during summer in shallow eutrophic lakes. Hydrobiologia. 710(1):95–107.
- Strock KE, Saros JE, McGowan S, Edlund MB, Engstrom DR. 2019. Response of boreal lakes to changing wind strength: Coherent physical changes across two large lakes but varying effects on primary producers over the 20(th) century. Limnol Oceanogr. 64(5):2237–2251.
- Sylwia SW, Agata C, Marta K, Jakub M, Adam L. 2019. Environmental drivers of bloom-forming cyanobacteria in the Baltic Sea: Effects of salinity, temperature, and irradiance. Estuarine Coastal Shelf Sci. 219:139–150.
- Terbraak CJF. 1986. Canonical correspondence analysis: A new eigenvector technique for multivariate direct gradient analysis. Ecology. 67(5):1167–1179.
- Tian C, Hao D, Pei H, Doblin MA, Ren Y, Wei J, Feng Y. 2018. Phytoplankton functional groups variation and influencing factors in a shallow temperate lake. Water Environ Res. 90(6):510–519.
- Tian C, Pei H, Hu W, Hao D, Doblin MA, Ren Y, Wei J, Feng Y. 2015. Variation of phytoplankton functional groups modulated by hydraulic controls in Hongze Lake, China. Environ Sci Pollut Res Int. 22(22):18163–18175.
- Tian C, Pei H, Hu W, Xie J. 2012. Variation of cyanobacteria with different environmental conditions in Nansi Lake, China. J Environ Sci-China. 24(8):1394–1402.
- Varol M. 2019. Phytoplankton functional groups in a monomictic reservoir: seasonal succession, ecological preferences, and relationships with environmental variables. Environ Sci Pollut Res Int. 26(20):20439–20453.
- Wang L, Cai Q, Tan L, Kong L. 2011. Phytoplankton development and ecological status during a cyanobacterial bloom in a tributary bay of the Three Gorges Reservoir, China. Sci Total Environ. 409(19):3820–3828.
- Wang N, Xiong J, Wang XC, Zhang Y, Liu H, Zhou B, Pan P, Liu Y, Ding F. 2018. Relationship between phytoplankton community and environmental factors in landscape water with high salinity in a coastal city of China. Environ Sci Pollut Res. 25(28):28460–28470.
- Wilken S, Soares M, Urrutia-Cordero P, Ratcovich J, Ekvall MK, Van Donk E, Hansson L-A. 2018. Primary producers or consumers? Increasing phytoplankton bacterivory along a gradient of lake warming and browning. Limnol Oceanogr. 63(S1):S142–S155.
- Wu Z, He H, Cai Y, Zhang L, Chen Y. 2014. Spatial distribution of chlorophyllaand its relationship with the environment during summer in Lake Poyang: a Yangtze-connected lake. Hydrobiologia. 732(1):61–70.
- Xia L, Lu X, Chen Y. 2011. The effects of temperature and nutrient ratios on Microcystis blooms in Lake Taihu, China: An 11-year investigation. Harmful Algae. 10(3):337–343.
- Xu H, Paerl HW, Qin B, Zhu G, Gaoa G. 2010. Nitrogen and phosphorus inputs control phytoplankton growth in eutrophic Lake Taihu. Limnol. Oceanogr. 55(1):420–432.
- Xu Y, Cai Q, Lin Y, Shao M. 2011. Asynchrony of spring phytoplankton response to temperature driver within a spatial heterogeneity bay of Three-Gorges Reservoir, China. Limnologica. 41(3):174–180.
- Yang C, Nan J, Yu H, Li J. 2020. Embedded reservoir and constructed wetland for drinking water source protection: Effects on nutrient removal and phytoplankton succession. J Environ Sci (China). 87:260–271.
- Yingfei X, Xiaodong H, Jixiong X, Yiping L, Sushu W, Peipei W. 2019. Seasonal succession of phytoplankton functional group and assessment of water quality in Lake Taihu. J Lake Sci-China. 31(1):134–146.
- Yoshinaga I, Hitomi T, Miura A, Shiratani E, Miyazaki T. 2006. Cyanobacterium Microcystis bloom in a eutrophicated regulating reservoir. JARQ. 40(3):283–289.
- Zeng Y, Zhu J, Wang Y, Hu W. 2018. Changes of water temperature and heat flux at water-sediment interface, East Lake Taihu. J Lake Sci-China. 30(6):1599–1609.
- Zhang Y, Lin S, Qian X, Wang Q, Qian Y, Liu J, Ge Y. 2011. Temporal and spatial variability of chlorophyllaconcentration in Lake Taihu using MODIS time-series data. Hydrobiologia. 661(1):235–250.
- Zhao Q, Wang J, Wang J, Wang JXL. 2019. Seasonal dependency of controlling factors on the phytoplankton production in Taihu Lake, China. J Environ Sci (China). 76(2):278–288.
- Zhu K, Bi Y, Hu Z. 2013. Responses of phytoplankton functional groups to the hydrologic regime in the Daning River, a tributary of Three Gorges Reservoir. Sci Total Environ. 450–451:169–177.