Abstract
Objective
To investigate the knowledge and perceptions of physicians on the role of modeling studies in asthma, using a modified Delphi procedure.
Methods
Group opinions among a panel of respiratory experts were obtained using two online questionnaires and a virtual scientific workshop. A consensus was pre-defined as agreement by >75% of participants.
Results
From 26 experts who agreed to participate, 22 completed both surveys. At the end of the process, the panel rated their own understanding of modeling as good (77%) but that among physicians in general as poor (77%). Participants agreed that data from modeling studies should be used, at least sometimes, to inform treatment guidelines (91%) and could be useful for guiding clinical decisions (100%). Perceived barriers to using modeling studies were ‘A lack of understanding’ (81%) and ‘A lack of standardized methodology’ (82%). Based on data from two modeling studies, no consensus was reached on physicians recommending regular inhaled corticosteroids (ICS) versus as-needed therapy for patients with mild asthma, whereas 77% agreed that they would recommend regular ICS over maintenance and reliever therapy for ≥80% of their patients with moderate asthma. No consensus was reached on the value of modeling data in relation to empirical data.
Conclusion
There is overall support among respiratory experts for the usefulness of modeling data to guide asthma treatment guidelines and clinical decision making. More publications on modeling data using robust models and accessible terminology will aid the understanding of physicians in general and help clarify the evidence-based value of modeling studies.
Introduction
In the past decade, clinical prediction models or drug-disease models have become increasingly recognized as a tool for evidence synthesis to inform public health policy and for clinical decision-making (Citation1,Citation2). These models have been used in communicable or non-communicable diseases to identify those most at risk of developing cardiovascular disease (Citation3), in cancer screening (Citation4) and to aid decision-making in cancer control (Citation5), for the development of antiretroviral therapy guidelines (Citation6,Citation7), and most recently for the prognosis of patients with COVID-19 (Citation8) and for investigating the emergence of new variants (Citation9). The World Health Organization (WHO) recommends that findings from mathematical modeling studies can be used to provide evidence of the impact of one or several interventions (comparative effectiveness), specifically in the absence of empirical data (Citation1).
In the respiratory field, the use of modeling studies to compare the efficacy and safety effects of different treatment regimens is still relatively new. In one recent study, a pharmacokinetic (PK)/pharmacodynamic (PD) model was developed to compare the bronchoprotective and benefit/risk profiles of various inhaled corticosteroid (ICS) dosing regimens and scenarios of adherence, in mild asthma (Citation10). The study showed that all ICS regimens provide sustained bronchoprotective efficacy when dosed regularly with high adherence; however, scenarios of poor adherence or ICS use 3–4 times per week (infrequent/as needed dosing) were associated with reduced levels of bronchoprotection. Another study used similar methodology to simulate the airway efficacy and systemic activity for different ICS dosing regimens used in the management of asthma (Citation11). The results showed that regular daily ICS or ICS/long-acting beta2-agonist (LABA) dosing regimens with budesonide or fluticasone propionate, had higher airway efficacy with similarly low systemic activity compared with as-needed budesonide/formoterol in mild asthma, or with maintenance and reliever therapy (MART) dosing in moderate and moderate-to-severe asthma.
The Global Initiative for Asthma (GINA) recommends two tracks for the treatment of asthma (Citation12). One recommends the use of inhaled ICS-formoterol, either as-needed for mild asthma or as MART for moderate or moderate-to-severe asthma, particularly for patients who are likely to be poorly adherent with daily controller treatment. The other proposes ICS to be taken whenever short-acting beta agonist (SABA) is taken (mild asthma) or proactive regular doses of ICS or ICS/LABA treatment plus SABA rescue medication for treatment steps 2 to 4. Not all national treatment guidelines have adopted the same approach with several, including the British thoracic Society, Japanese, Canadian, Spanish, and Thai guidelines, still recommending regular dosing with ICS plus SABA as rescue medication, for the preferred treatment of mild asthma (Citation13–17). This has led to differing opinions within the respiratory scientific community with respect to the evidence for the safety and efficacy of these approaches (Citation18–20).
In real-world clinical practice, treatment approaches in asthma often mis-align with guideline recommendations (Citation21,Citation22). One of the issues for clinicians, when deciding on the most appropriate treatment for patients with asthma, is the scarcity of clinical trial data that compare the efficacy and safety of different treatment strategies based on airway efficacy and systemic activity profiles (Citation10,Citation11). To conduct full scale trials of this nature would be both costly and time consuming, and in some instances unethical; whereas a modeling simulation approach would be well-suited to investigate such comparisons.
The extent of the understanding among respiratory physicians about modeling studies and how they may guide clinical decision-making is widely unknown. To investigate their knowledge and perceptions on the role of modeling studies in asthma, a modified Delphi procedure, incorporating two rounds of online surveys and a scientific workshop, was conducted among a panel of respiratory experts. The procedure also sought physician opinions about asthma controller medication treatment, considered in the context of modeling study data, and the role of as-needed SABA in treating asthma.
Methods
Participants
A group of global respiratory physicians with clinical experience from 15 countries (Argentina, Brazil, Columbia, Costa Rica, Guatemala, Kingdom of Saudi Arabia, Kuwait, Lebanon, Malaysia, Mexico, Philippines, South Africa, Thailand, Trinidad & Tobago, and Vietnam) were invited to participate. Physicians were selected due to their expert knowledge of asthma and their years of experience in the field. Informed consent was obtained prior to completion of the anonymized Delphi surveys.
The industry sponsors facilitated the conduct of the scientific workshop but did not complete the Delphi surveys or contribute to any consensus statements.
Study design
The Delphi procedure uses structured questionnaires to reach a group consensus on a specific topic among a panel of selected experts (Citation23–25). The process is facilitated through anonymity and controlled group feedback. In this study, two 10-min online surveys were completed by the panel of asthma experts and an on-line virtual scientific workshop was also conducted which involved discussion between the experts (). The first survey was sent out prior to the scientific workshop discussion and the second survey was distributed after the workshop. The aim was for the entire panel to be present at the workshop but this was not a requirement. The minutes from the workshop and the results of the first survey were circulated to all participants, and anyone who had not attended the workshop was asked to review them before completing the second survey.
An independent specialist team at Evoke Mind + Matter, Macclesfield, UK, administered the surveys. The team developed the questionnaires, hosted the online surveys using Decipher software, and analyzed the data. All information provided by panelists was kept confidential and anonymous. The research complied with UK Data Protection law (GDPR), with the British Healthcare Business Intelligence Association’s (BHBIA) Legal & Ethical Guidelines, and with the European Pharmaceutical Market Research Association’s (EphMRA) code of conduct. No formal ethical approval was required as this non-interventional physician survey fell outside the remit of the Research Government Framework.
Delphi procedure
Questions for the first survey were developed following a review of the literature that included the search terms “modelling”, “asthma”, “therapeutic index”, “benefit/risk ratio” and “drug-dose model”. In addition, international and national asthma guidelines were reviewed to check for any information regarding modeling studies, and to develop questions with respect to treatment and adherence (Citation12–17). Questions included in the second survey were based on participant group responses from survey one.
The first online survey consisted of 18 questions (11 close-ended, Likert style questions and 7 questions incorporating both close-ended and open-ended elements), covering the understanding of modeling studies, their role in developing treatment guidelines and in clinical decision-making, and perceived barriers to their use (Supplementary materials, Appendix 1). Questions about asthma controller treatment and adherence, in relation to two recently published modeling studies (Citation10,Citation11), and the role of as-needed SABA in treating asthma, were also covered in this questionnaire. For survey 1, the participants were informed of the references for the two published studies but no further information was given.
Experts who participated in the round 1 Delphi survey were invited to an on-line virtual scientific workshop for presentations and discussions about the potential role of modeling studies in asthma management and clinical decision-making, and the appropriate use of SABA in asthma management. Prior to the workshop, the participants were sent copies of the two published modeling studies (Citation10,Citation11) as a pre-read and the studies were discussed at the workshop. The second online survey, e-mailed to participants after the workshop, consisted of 19 questions − 7 close-ended Likert style questions, 1 open ended question and 11 questions incorporating both close-ended and open-ended elements (Supplementary materials, Appendix 2). The objective of the post-workshop survey was to assess the level of consensus on key topics following survey 1 results and workshop discussions, specifically, the potential role of modeling studies in asthma management and clinical decision-making.
Data analysis
Data were analyzed descriptively. A content analysis of group responses from survey 1 enabled the identification and classification of common elements, and for the development of survey 2. A data analyst at Evoke Mind + Matter checked all responses to ensure consistency and quality of response. Full feedback on the results of survey 1, presented as anonymous grouped responses, was shared with the participants before survey 2 was sent out.
For the results of each survey, a consensus was pre-defined as agreement by >75% of participants who answered each question, in line with previous Delphi studies (Citation19,Citation26–28). A descriptive analysis was used to look at differences in responses between the two surveys. Open-ended questions were analyzed descriptively, with duplicate points being grouped together. The full list of verbatim open-ended responses was made available to the workshop participants.
Results
Participants
From 30 invited respiratory expert physicians 26 accepted and completed the first Delphi survey. The experts all had experience in treating adults with asthma (the main focus of this study), had a median of 22 years of clinical experience (Range: 5–40 years) and were responsible for caring for an average of 50 patients with asthma per month (Range: 10–200+ per month) (Table S1). Twenty participants attended the online scientific workshop and 22 of those who accepted the initial invitation also completed the second survey (response rate 85%).
Table 1. Summary of scientific workshop presentations and discussions.
Delphi survey 1 results
Understanding of modeling
Although the understanding of modeling was rated by the expert respiratory participants as generally poor among primary care or general physicians (poor understanding: 42%-52%) (), it was higher among participants themselves (poor understanding: 4%) who reached a consensus (> 80%) that data from modeling studies should be used to inform treatment guidelines at least some of the time (). Nearly all participants (96%) also agreed that modeling studies could be somewhat or very useful for guiding decision-making in clinical practice () with a consensus reached on them being useful to ‘Compare the effectiveness of different treatments’ (81%) and to ‘Assess the long-term effectiveness or cost-effectiveness of a treatment’ (77%) (). The most frequent reason reported as a barrier to using modeling studies was ‘A lack of understanding’ (Supplementary Figure S1a) and most participants agreed that additional educational support and training are needed to understand the concept, application and interpretation of modeling studies (≥ 81%) (Supplementary Figure S1b). When asked about two recently published modeling studies in mild (Citation10) and moderate-to-severe asthma (Citation11), it was thought that only 31% or 42% of physicians in general, would have a good or excellent understanding of these studies, respectively. Although drug-disease models were ranked fifth in the hierarchy of clinical evidence after meta-analyses, randomized controlled trials (RCTs), systematic reviews and observational cohort studies, 81% of participants agreed, somewhat or strongly, that modeling studies should drive clinical recommendations in the absence of empirical or clinical trial data ().
Figure 2. Level of understanding about modeling in a) Survey 1 and b) Survey 2.
A total not adding to 100% is due to rounding.
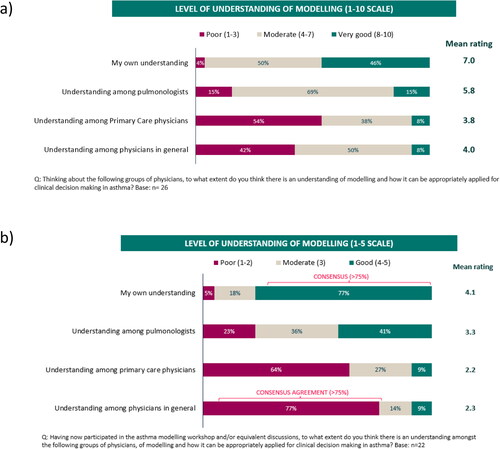
Figure 3. Use of modeling data to inform asthma treatment guidelines: a) Survey 1 and b) Survey 2.
A total not adding to 100% is due to rounding.
RCTs: Randomized controlled trials
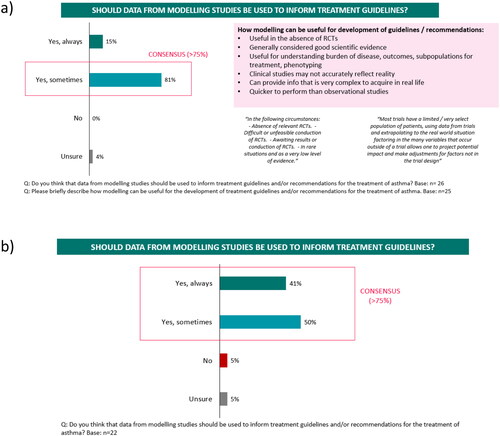
Figure 4. Usefulness of modeling data to guide decision-making in clinical practice: a) Survey 1 and b) Survey 2.

Asthma treatment
When considering the results of two modeling studies (Citation10,Citation11), participants indicated that they would recommend a proactive regular ICS dosing regimen for approximately two thirds of their patients with mild asthma (versus as-needed ICS-formoterol; ) or moderate asthma (versus a maintenance and reliever (MART) treatment regimen; ). For patients with mild asthma, some physicians indicated that as-needed treatment could be an option for patients with very infrequent symptoms and/or poor adherence to therapy. Similarly, for patients with moderate asthma, the importance of regular treatment to control the underlying disease was emphasized and the choice of MART regimen was often driven by patient factors e.g. patient understanding or adherence. All experts agreed on the importance of treatment adherence and indicated that adherence was managed through ongoing education (on what asthma is, self-management and inhaler technique), using patient reminders and via regular patient reviews. When choosing a controller treatment, 77% of participants were concerned about cortisol suppression and only 23% were not.
Figure 7. Treatment recommendations in asthma based on modeling study results (Survey 1) in a) mild asthma and b) moderate asthma.
A total not adding to 100% is due to rounding.
PRD: Proactive regular dosing; PRN: As-needed; MART: maintenance and reliever therapy
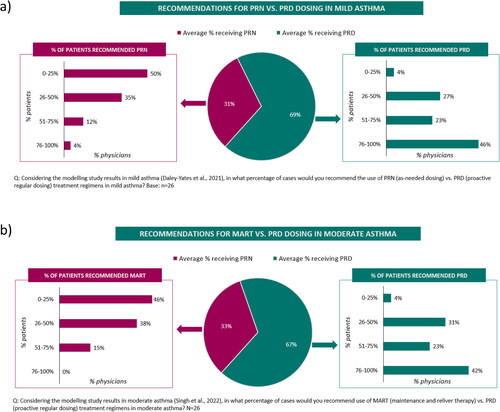
When asked about the role of SABA in the treatment of asthma, 92% of participants would recommend them to treat an acute asthma attack, 76% as a reliever medication and 72% to treat exercise-induced bronchoconstriction. No participants suggested that SABA should be used as a monotherapy to treat asthma. A consensus was not reached on the level of confidence in the efficacy or safety profile of as-needed SABA in the treatment of patients with moderate-to-severe asthma, with 62% and 54%, respectively, expressing a high level of confidence in the efficacy or safety of SABA (Supplementary Figures S2a and S2b). With respect to an acceptable frequency of SABA usage, participants most commonly indicated once or twice weekly (52%) or once monthly (32%).
Scientific workshop summary
A summary of the scientific workshop presentations and discussions is shown in . The role of modeling studies in asthma management was discussed, including how such studies can provide high quality evidence to fill in the data gaps not provided by empirical evidence. Several participants highlighted the importance of using a credible and validated model with clear justification and transparency about the data input for the model - this could be achieved through literature searches to identify input variables. There was an acknowledgement that any model would be required to make some assumptions due to the number of variables associated with asthma. There were also discussions about how modeling studies could help clinical decision-making in terms of comparing different treatment scenarios, could provide important information for policy makers and could reduce research costs. The need to simplify the terminology and jargon associated with modeling studies to enable easier interpretation, was highlighted.
An overview of the role of SABA in asthma treatment was presented with a focus on appropriate, as-needed SABA use with ICS-based maintenance treatment.
Delphi survey 2 results
Understanding of modeling
Following the scientific workshop, the level of understanding about modeling increased among participants, with 77% stating that their understanding was good, whilst their perception of the understanding among physicians in general decreased, with 77% of participants now rating the understanding amongst physicians in general as poor (). Similar to survey 1, participants agreed that data from modeling studies should be used to inform treatment guidelines at least some of the time (91%), but the proportion of participants stating that these data should always inform guidelines increased from 15% in survey 1 to 41% in survey 2 (). Similarly, participants still agreed that modeling data are somewhat or very useful for guiding clinical decisions (100%), with an increase shown in those who thought they are very useful, from 48% in survey 1 to 73% in survey 2 (). Similar to findings in survey 1, in survey 2, a consensus was reached on modeling studies being useful for ‘Assessing the efficacy of a drug’ (82%) (). However, additionally in survey 2, participants also agreed on their usefulness for ‘Drug-disease modeling’ (77%) and for ‘Determining the direct and indirect effects of an intervention at the population level’ (77%) (). Whilst, 78% of participants somewhat or strongly agreed in survey 2 that modeling studies should be routinely considered when making clinical treatment decisions (compared with 68% in survey 1), no consensus was reached in agreeing that modeling studies should carry the same weight as empirical research studies (50% somewhat or strongly agreed), similar to findings in survey 1 (). In addition to the barriers identified in survey 1, 82% of participants identified ‘A lack of a standardized method for how to integrate modeling approaches to inform guidelines’ as a further barrier to using modeling studies. Most participants (91%) also agreed that greater publication/availability of reproducible models and case study examples of how modeling can be applied to asthma are needed.
Asthma treatment
In survey 2, 55% of participants stated that they would recommend proactive regular ICS treatment versus as-needed therapy for at least 80% of patients with mild asthma, based on a recent modeling study (Supplementary Figure S3a). For patients with moderate asthma, 77% of participants said they would recommend regular treatment over MART for at least 80% of their patients (Supplementary Figure S3b).
Similar to survey 1, there was no consensus reached among the panel on cortisol suppression being a safety concern when deciding treatment for moderate-severe asthma patients. However, all participants agreed that they would prioritize asthma control over cortisol suppression when making treatment decisions for asthma patients.
Discussion
This study used a modified Delphi procedure to investigate the current knowledge and understanding of modeling studies among a panel of respiratory experts. The process demonstrated that, whilst the understanding of modeling is good among the participating experts, it is thought to be poor among physicians in general. The panel agreed that modeling studies could provide useful data to inform treatment guidelines and to guide clinical decisions with respect to comparative clinical efficacy, long-term efficacy and the cost-effectiveness of drugs. The main barriers or challenges to using data from modeling studies were identified as a lack of understanding about modeling studies and a lack of standardized methodology for integrating evidence from modeling studies into treatment guidelines. The panel did not reach a consensus on the position of modeling studies in terms of the evidence-based pyramid.
Although this study was conducted in a small sample of physicians, the results suggest there is a need for greater education and awareness amongst physicians in general to fully understand modeling study methodology and data interpretation. Even among the participating experts, some views on modeling changed following the workshop presentations and discussions, demonstrating the value of sharing such information. For example, after the workshop a consensus was reached (in survey 2) in recommending regular ICS dosing over MART for patients with moderate asthma, based on modeling study data (Citation11). On the other hand, there was no overall agreement on the treatment of mild asthma with regular ICS versus as-needed therapy, based on an earlier modeling study (Citation10). The reasons for this are not clear; it is possible that there was an increased learning between the earlier versus later-published modeling papers or that the more detailed clinical interpretation discussed in the second paper may have influenced physician opinions. The availability of more publications on good-quality modeling studies, as well as case study examples should all enhance this field of knowledge. It is also anticipated that this Delphi study will raise awareness and be helpful for future education about modeling in clinical medicine in general, and in the management of mild to moderate asthma in particular.
Data from modeling studies have been used in other areas of healthcare to inform treatment guidelines and aid in clinical decision-making. In infectious diseases, mathematical models have been used in the development of WHO antiretroviral therapy guidelines (Citation6,Citation7). Clinical prediction models have also been used in cardiovascular disease for both diagnosis and prognosis (Citation3,Citation29). In the cancer field, the OncoSim microsimulation model platform, which consists of a suite of specific cancer models, provides a powerful tool to support population-level policy- and decision-making (Citation5). In the recent COVID-19 pandemic, many prognostic prediction models were developed in relation to diagnosis and prognosis (Citation8) and for investigating new variants (Citation9). Similar to the challenges identified in our study, several other studies have highlighted the requirement for best practice in developing robust models that have credibility, good quality input data and that are well-validated (Citation8,Citation29–32). In the current study, no consensus was reached on the position of modeling studies in relation to other types of empirical evidence. The evidence-based hierarchy pyramid is a system which ranks different types of research based on the deemed quality of the evidence (Citation33). Although evidence from modeling data is gaining recognition from the WHO (Citation1) and the Food and Drug Administration (Citation34), our findings and those of other authors (Citation8,Citation29–32,Citation35) suggest that these data are currently viewed as adjunct rather than standalone evidence, and their position on the pyramid is yet to be clarified (Citation35). The OncoSim microsimulation model platform has undergone extensive validation (Citation5,Citation30) and was recently shown to accurately replicate the results of four RCTs of sigmoidoscopy (Citation36). Implementation of these types of best practice, as well as increased education and awareness initiatives, should raise the profile of modeling studies and help determine their assigned level in the evidence-based hierarchy pyramid (Citation35). The acceptance of meta-analyses as appearing at the top of the evidence-based pyramid came after their first appearance in the literature in the late 1970s followed by an exponential growth in publications through the 1990s onwards (Citation37). It’s conceivable that as more publications on modeling studies become available and their application is more widely understood, that modeling studies could follow a similar trajectory.
Although in this Delphi study, no consensus was reached in recommending regular dosing with ICS versus symptom-driven as-needed dosing in mild asthma, based on model predictions of bronchoprotection, another Delphi study on the management of mild asthma, did recommend regular daily ICS over as-needed ICS-formoterol determined from the clinical evidence for better symptom control and robust long-term clinical data (Citation19). Similar to our group, Domingo et al. also highlighted that treatment in mild asthma is often driven by patient factors such as non-adherence, and a call for ongoing patient education to encourage treatment adherence was recommended. Periods with sub-optimal bronchoprotection increase the risk of exacerbations and lung function decline (Citation38–41) and therefore continued efforts to encourage adherence are paramount. All experts on the panel agreed on the importance of adherence and, in line with other reports (Citation42,Citation43), suggested that adherence should be managed with regular reviews to check a patient’s adherence and inhaler technique, along with education tailored to the individual patient to ensure they understand that asthma is a chronic disease characterized by a variable course, hence the importance of adhering to regular treatment.
With respect to the role of as-needed SABA in the treatment of asthma, the panel agreed that it has a role in treating an acute asthma attack and as a reliever medication. However, no consensus was reached on the level of confidence felt in the efficacy or safety profile of as-needed SABA, and this appears to reflect a similar lack of consensus on the role of as-needed SABA in current treatment guidelines (Citation12–17) and among the respiratory community (Citation44,Citation45). This does highlight the importance of re-iterating the role of appropriate SABA use. In our survey, no participants suggested that SABA should be used as a monotherapy to treat asthma, in line with the potential risk of excessive SABA use in the absence of ICS. An overuse of SABA reflects poorly controlled asthma and an underuse of ICS and this underuse of ICS is linked to an increased risk of exacerbations and asthma-related deaths, rather than to SABA use per se (Citation40,Citation46,Citation47). A recent meta-analysis investigating SABA safety data from RCTs over the past 25 years, reported that, when used appropriately as a reliever therapy, SABA does not contribute to excess rates of mortality or serious adverse events (Citation48). Busse et al. reported that an attention to patterns of increased use of SABA may indicate an impending asthma exacerbation, alerting patients/clinicians to implement their action plan and acting as a reminder to take regular treatment (Citation49). During the COVID-19 pandemic, a shortage of SABA inhalers strengthened the importance and well-established role of SABA in the current management of asthma (Citation44). Medication costs and access to medications are also important considerations, particularly in low to middle-income countries where patient affordability greatly influences a physician’s choice of asthma treatment (Citation22,Citation50).
The Delphi procedure used in the current study has some strengths and limitations. The Delphi procedure is well documented as a robust and useful technique for obtaining a group consensus on a specific topic, particularly where prior evidence is unavailable or lacking (Citation23–25). A strength of this study was the inclusion of respiratory specialists with extensive experience in asthma management across different healthcare systems, cultures, and languages and thus their individual responses and opinions likely reflect current knowledge and perceptions within the field of asthma. Another strength of the study was the good response rate, with 85% of participants completing both questionnaires and 77% of invited experts participating in the workshop. The inclusion of ≥ 20 participants at each stage of the process were regarded as a satisfactory number to reach a meaningful consensus, based on previous studies in asthma and COPD (Citation19,Citation51–53). A limitation of this study is that it does not directly represent the views of general practitioners or physicians generally. A parallel survey of how the “general physician community” understands modeling study methodology and data interpretation could further identify gaps that could be addressed by future studies in this field. Another limitation is that the results from this Delphi may not be reproducible with another group of experts (Citation23), meaning these data should be interpreted accordingly. Finally, although conducting the procedure via an online virtual platform may not be as engaging as in-person interviews, the e-Delphi has the advantage of obtaining the opinions of a diverse group (geographically) in a time and cost-effective approach (Citation54).
In conclusion, this Delphi study aimed to gain an understanding of the role of modeling studies in asthma management and clinical decision-making, as perceived by a group of expert respiratory physicians. Amongst the participants, there was overall support for the usefulness of modeling data to guide asthma treatment guidelines and clinical decision-making. Modeling data can be used to explore clinical problems that cannot be solved by clinical trials. The availability of more publications on modeling data using robust, reproducible models and accessible terminology, will aid the understanding of physicians in general and help clarify the position of modeling studies in the evidence-based pyramid.
Author contributions
Conceived and designed the study: Bhumika Aggarwal, Abhay Phansalkar, Terence Seemungal
Data acquisition and/or data interpretation
All authors
Data analyses
Bhumika Aggarwal, Abhay Phansalkar, supported by Evoke Mind + Matter
All authors contributed to drafting, reviewing, and critically revising the paper, gave final approval of the version to be published, and agreed to be accountable for all aspects of the work.
Supplemental Material
Download MS Word (312.2 KB)Acknowledgements
The authors thank all participants who completed the surveys and contributed to discussions in the scientific workshop. Authors also thank Rachel Butcher and Rebecca Mills of Evoke Mind + Matter for their help in preparing and administering the surveys, and in analyzing and presenting the data. Editorial support was provided by Kate Hollingworth of Continuous Improvement Ltd and was funded by GSK.
Declaration of interest
Thitiwat Sriprasart has no competing interests to declare. Ma Bella Siasoco has received consulting fees from GSK and for acting as a senior Technical Adviser for the USAID FHI360 Epic Project, Philippines; and has received honoraria for speaking on webinars/at symposia for Getz Pharma, GSK and Novartis. Giap Vu Van has received honoraria for lectures, presentations, speakers’ bureaus or educational events, from Abbott, AstraZeneca, Boehringer Ingelheim, GSK, MSD, Novartis, Sandoz and Sanofi. Mark Cohen has received honoraria for participating in advisory boards from AstraZeneca, Boehringer Ingelheim, GSK and Sanofi; honoraria for presentations, manuscript writing or educational events from of AstraZeneca, Boehringer Ingelheim, Chiesi, GSK and Pfizer; and support for travel/attending meetings from AstraZeneca. Terence Seemungal has received honoraria for participating in advisory boards from AstraZeneca and GSK; honoraria for lectures from AstraZeneca, Boehringer Ingelheim and GSK; and support for travel/attending meetings from AstraZeneca, Boehringer Ingelheim and GSK. Marcia Pizzichini has received consulting fees from Boehringer Ingelheim, GSK and Sanofi-Genzyme; and support for travel/attending meetings from Sanofi-Genzyme. Mahmoud Mokhtar has received honoraria for presentations, manuscript writing or educational events from Abbott, AstraZeneca, Boehringer Ingelheim, GSK, Novartis and Sanofi; and support for travel/attending meetings from AstraZeneca, Boehringer Ingelheim, GSK, Mundipharma and Sanofi. Bhumika Aggarwal, Gur Levy, Abhay Phansalkar and Peter Daley-Yates are GSK employees and hold GSK shares.
Data sharing statement
Information on GSK’s data sharing commitments and requesting access can be found at: https://www.clinicalstudydatarequest.com.
Additional information
Funding
References
- Egger M, Johnson L, Althaus C, Schöni A, Salanti G, Low N, Norris SL. Developing WHO guidelines: time to formally include evidence from mathematical modelling studies. F1000Res. 2017;6:1584. doi:10.12688/f1000research.12367.1.
- Porgo TV, Norris SL, Salanti G, Johnson LF, Simpson JA, Low N, Egger M, Althaus CL. The use of mathematical modeling studies for evidence synthesis and guideline development: a glossary. Res Synth Methods. 2019;10(1):125–133. doi:10.1002/jrsm.1333.
- Allan S, Olaiya R, Burhan R. Reviewing the use and quality of machine learning in developing clinical prediction models for cardiovascular disease. Postgrad Med J. 2022;98(1161):551–558. doi:10.1136/postgradmedj-2020-139352.
- WHO guideline for screening and treatment of cervical pre-cancer lesions for cervical cancer prevention. 2nd ed. Geneva: World Health Organization, 2021.
- Gauvreau CL, Fitzgerald NR, Memon S, Flanagan WM, Nadeau C, Asakawa K, Garner R, Miller AB, Evans WK, Popadiuk CM, et al. The OncoSim model: development and use for better decision-making in Canadian cancer control. Curr Oncol. 2017;24(6):401–406. doi:10.3747/co.24.3850.
- Easterbrook PJ, Doherty MC, Perriëns JH, Barcarolo JL, Hirnschall GO. The role of mathematical modelling in the development of recommendations in the 2013 WHO consolidated antiretroviral therapy guidelines. AIDS. 2014;28(Supplement 1):S85–S92. doi:10.1097/QAD.0000000000000111.
- Consolidated guidelines on HIV prevention, testing, treatment, service delivery and monitoring: recommendations for a public health approach. Geneva: World Health Organization; 2021.
- Wynants L, Van Calster B, Collins GS, Riley RD, Heinze G, Schuit E, Bonten MMJ, Dahly DL, Damen JAA, Debray TPA, et al. Prediction models for diagnosis and prognosis of covid-19: systematic review and critical appraisal. BMJ. 2020;369:m1328.
- Ghafari M, Liu Q, Dhillon A, Katzourakis A, Weissman DB. Investigating the evolutionary origins of the first three SARS-CoV-2 variants of concern. Front Virol. 2022;2:942555.
- Daley-Yates P, Aggarwal B, Lulic Z, Fulmali S, Cruz AA, Singh D. Pharmacology versus convenience: a benefit/risk analysis of regular maintenance versus infrequent or as-needed inhaled corticosteroid use in mild asthma. Adv Ther. 2022;39(1):706–726. doi:10.1007/s12325-021-01976-4.
- Singh D, Garcia G, Maneechotesuwan K, Daley-Yates P, Irusen E, Aggarwal B, Boucot I, Berend N. New versus old: the impact of changing patterns of inhaled corticosteroid prescribing and dosing regimens in asthma management. Adv Ther. 2022;39(5):1895–1914. doi:10.1007/s12325-022-02092-7.
- Global Initiative for Asthma. Global strategy for asthma management and prevention. Global Initiative for Asthma (GINA); 2022. https://ginasthma.org/gina-reports/.
- British Thoracic Society, Scottish Intercollegiate Guidelines Network. British guideline on the management of asthma; 2019. https://www.brit-thoracic.org.uk/standards-of-care/guidelines/btssign-british-guideline-on-the-management-of-asthma/
- Nakamura Y, Tamaoki J, Nagase H, Yamaguchi M, Horiguchi T, Hozawa S, Ichinose M, Iwanaga T, Kondo R, Nagata M, et al. Japanese guidelines for adult asthma 2020. Allergol Int. 2020;69(4):519–548. doi:10.1016/j.alit.2020.08.001.
- Yang CL, Hicks EA, Mitchell P, Licskai C, Dell SD, Rowe BH, Fitzgerald M, Leigh R, Watson W, Boulet LP. 2021 Canadian Thoracic Society Guideline – a focused update on the management of very mild and mild asthma. Can J Respir Crit Care Sleep Med. 2021;5(4):205–245. doi:10.1080/24745332.2021.1877043.
- Plaza V, Alobid I, Alvarez C, Blanco M, Ferreira J, García G, Gómez-Outes A, Gómez F, Hidalgo A, Korta J, et al. Spanish Asthma Management Guidelines (GEMA) v.5.1. Highlights and controversies. Arch Bronconeumol. 2022;58(2):T150–T158. doi:10.1016/j.arbres.2021.05.032.
- Kawamatawong T, Sangasapaviriya A, Saiphoklang N, Oer-Areemitr N, Sriprasart T, Kamalaporn H, Amnuaypattanapon K, Rerkpattanapipat T, Chirakalwasan N, Kulpraneet M, et al. Guidelines for the management of asthma in adults: evidence and recommendations. Asian Pac J Allergy Immunol. 2022;40(1):1–21.
- Domingo C, Rello J, Sogo A. As-needed ICS-LABA in mild asthma: what does the evidence say? Drugs. 2019;79(16):1729–1737. doi:10.1007/s40265-019-01202-0.
- Domingo C, Garcia G, Gemicioglu B, Van GV, Larenas-Linnemann D, Neffen H, Poachanukoon O, Sagara H, Berend N, Pizzichini E, et al. Consensus on mild asthma management: results of a modified Delphi study. J Asthma. 2022. doi:10.1080/02770903.2022.2034850.
- Gauthier M, Wenzel SE. As-needed β agonist-inhaled corticosteroid in mild asthma. Lancet. 2019;394(10202):897–898. doi:10.1016/S0140-6736(19)31994-4.
- Chapman KR, Canonica GW, Lavoie KL, Nenasheva N, Garcia G, Bosnic-Anticevich S, Bourdin A, Cano MCD, Abhijith PG, Aggarwal B. Patients’ and physicians’ perspectives on the burden and management of asthma: results from the APPaRENT 2 study. Respir Med. 2022;201:106948. doi:10.1016/j.rmed.2022.106948.
- Aggarwal B, Shantakumar S, Hinds D, Mulgirigama A. Asia-Pacific Survey of Physicians on Asthma and Allergic Rhinitis (ASPAIR): physician beliefs and practices about diagnosis, assessment, and treatment of coexistent disease. J Asthma Allergy. 2018;11:293–307. doi:10.2147/JAA.S180657.
- Hasson F, Keeney S, McKenna H. Research guidelines for the Delphi survey technique. J Adv Nurs. 2000;32(4):1008–1015.
- Boulkedid R, Abdoul H, Loustau M, Sibony O, Alberti C. Using and reporting the Delphi method for selecting healthcare quality indicators: a systematic review. PLoS One. 2011;6(6):e20476. doi:10.1371/journal.pone.0020476.
- Thompson M. Considering the implication of variations within Delphi research. Fam Pract. 2009;26(5):420–424. doi:10.1093/fampra/cmp051.
- Slade SC, Dionne CE, Underwood M, Buchbinder R. Standardised method for reporting exercise programmes: protocol for a modified Delphi study. BMJ Open. 2014;4(12):e006682. doi:10.1136/bmjopen-2014-006682.
- Diamond IR, Grant RC, Feldman BM, Pencharz PB, Ling SC, Moore AM, Wales PW. Defining consensus: a systematic review recommends methodologic criteria for reporting of Delphi studies. J Clin Epidemiol. 2014;67(4):401–409. doi:10.1016/j.jclinepi.2013.12.002.
- Vogel C, Zwolinsky S, Griffiths C, Hobbs M, Henderson E, Wilkins E. A Delphi study to build consensus on the definition and use of big data in obesity research. Int J Obes (Lond). 2019;43(12):2573–2586. doi:10.1038/s41366-018-0313-9.
- Wessler BS, Lai Yh L, Kramer W, Cangelosi M, Raman G, Lutz JS, Kent DM. Clinical prediction models for cardiovascular disease: tufts predictive analytics and comparative effectiveness clinical prediction model database. Circ Cardiovasc Qual Outcomes. 2015;8(4):368–375. doi:10.1161/CIRCOUTCOMES.115.001693.
- Rutter CM, Knudsen AB, Marsh TL, Doria-Rose VP, Johnson E, Pabiniak C, Kuntz KM, van Ballegooijen M, Zauber AG, Lansdorp-Vogelaar I. Validation of models used to inform colorectal cancer screening guidelines: accuracy and Implications. Med Decis Making. 2016;36(5):604–614. doi:10.1177/0272989X15622642.
- Bernard A. Clinical prediction models: a fashion or a necessity in medicine? J Thorac Dis. 2017;9(10):3456–3457. doi:10.21037/jtd.2017.09.42.
- Lo NC, Andrejko K, Shukla P, Baker T, Sawin V, Norris S, Lewnard J. Contribution and quality of mathematical modeling evidence in World Health Organization guidelines: a systematic review. Epidemics. 2022;39:100570. doi:10.1016/j.epidem.2022.100570.
- OCEBM Levels of Evidence Working Group. The Oxford 2011 levels of evidence. Oxford Centre for Evidence-Based Medicine. [accessed 2023 Jan 29]. Available from: https://www.cebm.ox.ac.uk/resources/levels-of-evidence/ocebm-levels-of-evidence
- Food and Drug Administration. Exposure-response relationships—study design, data analysis, and regulatory applications. Guidance for industry; 2003. Available from: https://www.fda.gov/regulatory-information/search-fda-guidance-documents/exposure-response-relationships-study-design-data-analysis-and-regulatory-applications
- Younis T, Thana M, Skedgel C. Evidence in medicine: math versus biology! Curr Oncol. 2017;24(6):349–351. doi:10.3747/co.24.3970.
- Coldman A, Pader J, Gauvreau C, Memon S, Fitzgerald N, Flanagan W, Nadeau C, Earle C, Wolfson M, Miller A, et al. simulating results from trials of sigmoidoscopy screening using the OncoSim microsimulation model. J Cancer Policy. 2018;15:52–58. doi:10.1016/j.jcpo.2017.12.006.
- Haidich AB. Meta-analysis in medical research. Hippokratia. 2010;14(1):29–37.
- Boulet LP. Asymptomatic airway hyperresponsiveness: a curiosity or an opportunity to prevent asthma? Am J Respir Crit Care Med. 2003;167(3):371–378. doi:10.1164/rccm.200111-084PP.
- Woolcock AJ. What are the important questions in the treatment of asthma? Clin Exp Allergy Rev. 2001;1(2):62–64. _doi:10.1046/j.1472-9725.2001.00007.x.
- Gibbons DC, Aggarwal B, Fairburn-Beech J, Hinds D, Fletcher M, Bosnic-Anticevich S, Price D. Treatment patterns among non-active users of maintenance asthma medication in the United Kingdom: a retrospective cohort study in the Clinical Practice Research Datalink. J Asthma. 2021;58(6):793–804. doi:10.1080/02770903.2020.1728767.
- Sears MR. Lung function decline in asthma. Eur Respir J. 2007;30(3):411–413. doi:10.1183/09031936.00080007.
- Eakin MN, Rand CS. Improving patient adherence with asthma self-management practices: what works? Ann Allergy Asthma Immunol. 2012;109(2):90–92. doi:10.1016/j.anai.2012.06.009.
- Dhruve H. Management of asthma: adherence, inhaler technique and self-management. Pract Nurs. 2018;29(10):465–468. doi:10.12968/pnur.2018.29.10.465.
- Amirav I, Newhouse MT. Asthma and COVID-19: in defense of evidence-based SABA. J Asthma Allergy. 2020;13:505–508. doi:10.2147/JAA.S279061.
- Charriot J, Gaga M, Suehs C, Bourdin A. Asthma rescue treatments, time to reboot. Eur Respir J. 2020;55(4):2000542. doi:10.1183/13993003.00542-2020.
- Royal College of Physicians. Why asthma still kills: the National Review of Asthma Deaths (NRAD) Confidential Enquiry report. http://www.rcplondon.ac.uk/sites/default/files/why-asthma-still-kills-full-report.pdf. 2014.
- Fletcher M, van der Molen T, Lenney W, Boucot I, Aggarwal B, Pizzichini E. Primary care management of asthma exacerbations or attacks: impact of the COVID-19 pandemic. Adv Ther. 2022;39(4):1457–1473. doi:10.1007/s12325-022-02056-x.
- Sriprasart T, Waterer G, Garcia G, Rubin A, Andrade MAL, Roguska A, Phansalkar A, Fulmali S, Martin A, Mittal L, et al. Safety of SABA monotherapy in asthma management: a systematic review and meta-analysis. Adv Ther. 2023;40(1):133–158. doi:10.1007/s12325-022-02356-2.
- Aggarwal B, Jones PW, Yunus F, Lan LTT, Boonsawat W, Ismaila A, Ascioglu S. Direct healthcare costs associated with management of asthma: comparison of two treatment regimens in Indonesia, Thailand and Vietnam. J Asthma. 2022;59(6):1213–1220. doi:10.1080/02770903.2021.1903915.
- Busse W, Stempel D, Aggarwal B, Boucot I, Forth R, Raphiou I, Rabe KF, Reddel HK. Insights from the AUSTRI study on reliever use before and after asthma exacerbations. J Allergy Clin Immunol Pract. 2022;10(7):1916–1918.e2. doi:10.1016/j.jaip.2022.03.029.
- Bousquet J, Winchester C, Papi A, Virchow JC, Haughney J, Costa D, Usmani O, Bjermer L, Price D. Inhaled corticosteroid/long-acting? ?-Agonist combination therapy for asthma: attitudes of specialists in Europe. Int Arch Allergy Immunol. 2012;157(3):303–310. doi:10.1159/000329519.
- Harding G, Leidy NK, Meddis D, Kleinman L, Wagner S, O’Brien CD. Interpreting clinical trial results of patient-perceived onset of effect in asthma: methods and results of a Delphi panel. Curr Med Res Opin. 2009;25(6):1563–1571. doi:10.1185/03007990902914403.
- Sheikh A, Major P, Holgate ST. Developing consensus on national respiratory research priorities: key findings from the UK Respiratory Research Collaborative’s e-Delphi exercise. Respir Med. 2008;102(8):1089–1092. doi:10.1016/j.rmed.2008.03.006.
- Fink-Hafner D, Dagen T, Doušak M, Novak M, Hafner-Fink M. Delphi method: strengths and weaknesses. Adv Meth Stat. 2019;16(2):1–19. doi:10.51936/fcfm6982.