The objectives of this study were to identify the sources of the PM 2.5 in Indianapolis, Indiana and estimate their contributions to the total PM 2.5 mass concentrations by analyzing the data from the samples collected at the EPA Speciation Trends Networks (STN) site in Indianapolis, Indiana. Both positive matrix factorization (PMF2) and an expanded factor analysis model were applied. The two methods obtained essentially identical source profiles and contributions, so the results of the simpler method, PMF, are described in the formal text of this paper in detail while the corresponding results provided by the expanded factor analysis model are presented in the supplemental material for this paper. The seven resolved sources are secondary sulfate (40.2%), secondary nitrate (21.9%), gasoline emission (16.6%), diesel emission (7.9%), airborne soil (5.3%), Fe-related industries (4.4%), and Cu-related industries (2.5%). The comparison between two models suggests that PMF coupled with subsequent data analysis methods (such as CPF, PSCF, seasonal variation analysis, and weekday/weekend variation analysis) yields the results that are comparable to those of the expanded factor analysis. The results suggest that such studies of STN data can be used to assist in the development of State Implementation Plans (SIPs) for PM 2.5 .
1. INTRODUCTION
Particulate matter concentrations have been found to have a relationship with human mortality and morbidity (CitationDockery et al. 1993; CitationGauderman et al. 2000). Especially in large urban areas, the mixture of regional sources and local sources makes the study of source/receptor relationships more complex. The U.S. Environmental Protection Agency established the Speciation Trends Network (STN) consisting of 54 core STN monitoring sites and about 170 other monitoring sites to characterize PM2.5 (particle matter with the aerodynamic diameter ≤2.5 μm) composition in urban areas and to provide the input data to develop emission control strategies for these locations. In this study, the ambient aerosols measured at the STN site in Indianapolis, IN were analyzed with two advanced factor analysis models, positive matrix factorization (PMF) and expanded factor analysis model. The major problems in the STN data are the organic carbon (OC) concentrations were not blank corrected and most of the measurement uncertainties were not provided (CitationKim et al. 2005a). The goals of this study included (1) to identify the sources for the ambient aerosols in the Indianapolis area and estimate the contribution of each aerosol source to the total PM2.5 mass and (2) to further test the proposed approaches to solve the measurement problems. Since the results of two factor analysis methods are essentially the same, the results discussion will be focused on the simpler model, PMF, leaving the results of the expanded model to be discussed in the supplemental file for this paper.
2. SAMPLING AND DATA PRETREATMENT
The STN site in Indianapolis (Latitude: 39.81° N, Longitude: −86.11° W, Elevation: 235 meters) is located at 3120 E. 30th ST., Washington Park, Indianapolis, IN. shows a map of the area around this sampling site. The corresponding wind data were measured at Indianapolis International Airport (Lat: 39°42′ N, Long: 86°16′ W, Elev: 794 Feet). A spiral aerosol speciation sampler (SASS) was used at this monitoring site. Integrated 24-hr samples were collected every third or sixth day during the period from 12/20/2000 to 11/14/2003. Teflon filters were analyzed by any one of five different X-Ray Fluorescence (XRF) spectrometers located in three different laboratories: Chester LabNet (770 and 771), Cooper Lab, and Research Triangle Institute (RTI1 and RTI2). Organic carbon (OC) and elemental carbon (EC) on pre-fired quartz filters were analyzed by the Thermal Optical Transmittance (TOT) method (CitationBirch and Cary 1996). The Nylon filters for anions and cations were analyzed by ion chromatography.
The OC concentrations in the STN data were not blank corrected. The corrected OC concentrations were estimated by subtracting the intercept of the regression of OC concentrations against PM2.5 concentrations (blank OC) from the original OC concentrations (CitationTolocka et al. 2001; CitationKim et al. 2005a). The blank OC concentration for this study was 2.38 μ g/m3. The uncertainties for the STN data prior to July 2003 have not been provided, so the uncertainties in this study were estimated using the procedures of CitationKim et al. (2005a). Different instruments had individual method detection limits (MDL), so in this study, the average MDL was used for estimating measurement uncertainties. For the normal measured values, the uncertainties were estimated by:
Those samples for which all of the species were missing or below the MDL values were excluded from the analysis. The samples whose corresponding total PM2.5 mass concentrations were missing were also removed. Some species had extreme values in their concentration series suggesting unusual events. Four samples corresponding to these sharp peaks were excluded. The July 4, 2002 sample was also removed from the analysis. Subsequent to the initial factor analysis, the samples corresponding to two high and uninterpretable peaks in the contribution series of the gasoline vehicle emissions source were excluded. Thus, 240 samples were retained for further analysis.
The species screening was based on two rules that signal/noise ratio (S/N) should be larger than 0.2 (The species can be considered as bad, weak, and normal, if S/N < 0.2, 0.2 ≤ S/N < 2, and S/N ≥ 2, respectively (CitationPaatero and Hopke 2003)), and the percentage of missing and BDL values should be less than 90% (at least 10% of measurement are normal). In addition, there is a strong relationship between XRF sulfur and IC sulfate, so only XRF sulfur was used. Ultimately, 28 species were retained for analysis. shows the summary statistics for these species. As to the weak species defined by S/N, a relatively large factor (5) was used to downweight their concentrations in the analysis. It was found that Na+ in Nylon filters collected between October 2001 and January 2002 were contaminated (CitationRTI, 2004), so Na+ was downweighted by a factor of 40. It may also be reasonable to exclude Na+.
TABLE 1 Summary of the PM2.5 measurements at the Indianapolis STN site
3. DATA ANALYSIS
3.1 PMF and Expanded Receptor Model
Two advanced receptor models, positive matrix factorization (PMF) and expanded receptor model, were investigated in this study. PMF provides a source profile matrix and a source contribution matrix to fit the measured concentration data as well as possible through minimizing the sum of the scaled residuals. PMF is able to handle missing and BDL values using specific weights and applies non-negative constraint to provide physically interpretable solution. The detailed principles and algorithms of PMF can be found in CitationPaatero (1997). PMF has been implemented in the program PMF2 that has been used in a number of prior studies (CitationPolissar et al. 2001a; CitationSong et al. 2001; CitationKim et al. 2004; CitationZhao and Hopke 2004). The expanded receptor model can be considered as an extension of PMF. It provides a general and flexible framework for factor analysis, so one can not only obtain the source profiles and contributions but also observe the action of environmental factors on the measured concentrations (CitationPaatero and Hopke 2002; CitationKim et al. 2003; CitationZhao et al. 2004). The expanded receptor model consists of two sets of equations. One set is called the main equation that is used for extracting source profiles and contributions and the other is called auxiliary equation that is used for estimating the actions of environmental factors on pollutant concentrations. In this study, the environmental factors included wind direction, wind speed, seasonal factor, and weekday/weekend factor. The corresponding expanded receptor model is represented as:
The objective of expanded receptor model is to resolve the above factors (F, G, D, V, W, and S) from the measured species mass concentration data through the following optimization function with the optimization tool called multi-linear engine (CitationPaatero 1999).
3.2 Potential Source Contribution Function
In order to locate regional sources, the potential source contribution functions (PSCF) (CitationAshbaugh et al. 1985; CitationHopke et al. 1995) was applied in this study. The PSCF model was calculated using the source contributions estimated from factor analysis models and backward trajectories calculated with the Hybrid Single Particle Lagrangian Integrated Trajectory (HYSPLIT) model and gridded meteorological data (CitationDraxler and Rolph 2003). Based on the assumptions that if a trajectory endpoint of the air parcel lies in a geophysical grid cell, the trajectory is assumed to collect PM2.5 emitted in this cell and the PM2.5 matter from this cell is assumed to be transported along the trajectory to the monitoring site, the PSCF is the conditional probability of a high concentration upon the arrival at the monitoring site that passed through each cell and is defined as
To minimize the effect of the cells with small np ij values that may have high uncertainties, it is suggested to use a special weight to downweight the PSCF values of the cells with the small number of end points (CitationHopke et al. 1995; CitationPolissar et al. 2001b). The arbitrary weights for this study were set to be
3.3 Conditional Probability Function
A method to locate sources (especially local sources) and investigate the wind direction effects on each source is the conditional probability function model (CitationAshbaugh et al. 1985; CitationZhou et al. 2004). CPF estimates the probability that a given source contribution from a given wind direction will exceed a predetermined threshold criterion. Supposing the wind direction range is divided into k sectors, the CPF for each sector is defined as
4. RESULTS AND DISCUSSION
Because the sources obtained through PMF and expanded receptor model are not orthogonal, a solution with more factors does not necessarily contain the same sources in a solution with fewer factors. Therefore, it is necessary to explore different numbers of sources to find a solution providing an adequate fit to the data and good physical interpretability. Generally, finding the solution can be divided into two steps, coarse selection and fine adjustment.
In the first step, it is expected to determine the proper number of sources. A plot of Q as the function of factor number can help determine the proper source number (CitationZhao and Hopke 2004). In the second step, the parameters FPEAK (for PMF) and FKEY can be used to control the rotation problem and find the optimal solution (CitationPaatero et al. 2002). In a plot of Q versus FPEAK, a proper FPEAK should lie in the range where the Q has low and stable values. In the PMF analysis of this study, the final FPEAK value was –0.2. FKEY is used to pull down species that are not expected to be or have abnormal abundances in source profiles. Careful examination of the scaled residuals as well as the ability to interpret the solution provided the bases for selecting the number of factors.
PMF and expanded receptor model both generated a reasonable solution with seven sources. This section will be focused on PMF results and the results of the expand model will be briefly discussed in the appendix section. The profiles and contributions of the resolved sources by PMF were shown in and . shows the reconstructed PM2.5 mass of PMF model is in the good agreement with the measured, which proves the resolved sources can correctly account for the particle mass.
FIG. 2 Source profiles of ambient aerosols in Indianapolis of PMF (error bars denote the uncertainty of solution).
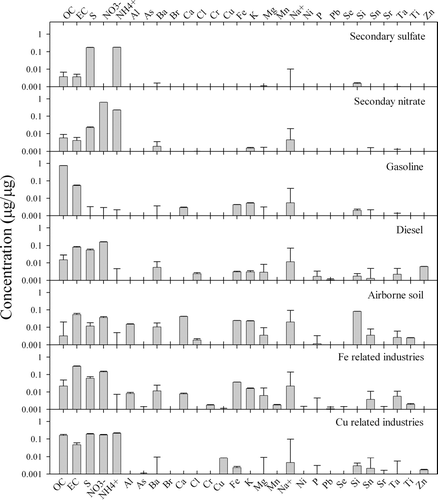
FIG. 4 Reconstructed total PM2.5 mass concentrations of PMF versus measured PM2.5 mass concentrations for the STN site in Indianapolis.
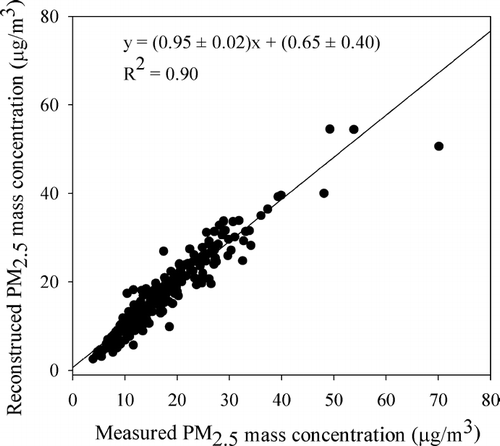
Source 1 represents secondary sulfate with high abundance of S. CitationBrook et al. (2004) using data from the CASTNET and CAPMON networks have shown that the upper mid-western U.S. and corresponding portions of southern Canada have high concentrations of particulate nitrate while the eastern coastal and southeastern regions of the U.S. have high sulfate concentrations. The regions to the south of Indianapolis have generally higher concentration of SO4 − 2. This fact agrees with the PSCF plot for secondary sulfate in showing a large contribution from the south where there are a number of coal-fired power plants in the Ohio and Tennessee River valleys. The seasonal variation plot of this source in shows a strong seasonal pattern with high contributions in the summer. The contribution time series of this source in also shows a clear seasonal pattern with the higher contribution in the summer time when the rate of photo-oxidation of SO2 to SO4 −2 was maximal (CitationHarrison and Perry 1986). As shown in , this source is the largest source in the Indianapolis area contributing 40.2% to the total PM2.5 mass. The weekday and weekend contribution variation plot () does not show a significant weekend effect.
FIG. 6 Seasonal (summer: April–September, winter: October–March) contribution variations of seven identified sources in Indianapolis (mean ± 95% confidence interval).
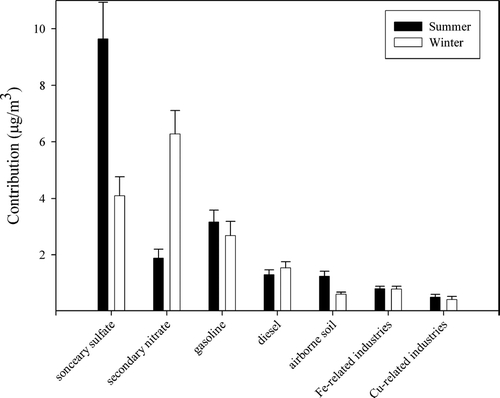
FIG. 7 Weekend/weekday contribution variations of seven identified sources in Indianapolis (mean ± 95% confidence interval).
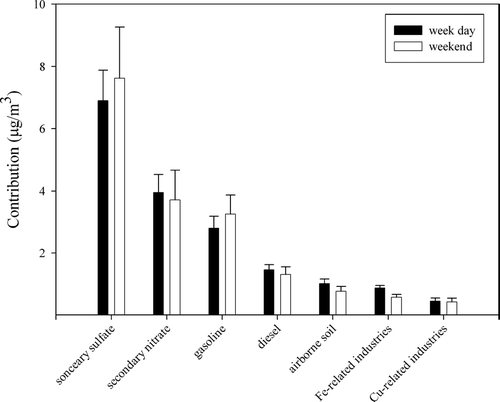
TABLE 2 Average source contributions of PMF model (CI: confidence interval)
The second source represents secondary nitrate with high concentration of nitrate. Nitrate is formed in the atmosphere predominantly through oxidation of NOx and its possible origins include mobile and stationary source emissions. The seasonal variation plot shows this source has a strong seasonal trend with high contributions in the winter. The major reason is that low temperature shifts the equilibrium system of NH3 −and HNO3 toward the particle phase, increasing the mass of NH4NO3 (CitationSeinfeld and Pandis, 1998). The PSCF plot of this source shows there are higher NO3 − probabilities to the northwest of Indianapolis. The areas with high probabilities (darker color) in the PSCF plot show areas where there are large-scale animal husbandry facilities and significant use of anhydrous ammonia as a fertilizer (CitationGoebesa et al. 2003). Thus, in this case, it may be possible that ammonia could be the determining factor for the formation of particulate nitrate. This source does not have a significant weekend effect and contributes 21.9% to the total PM2.5 mass.
Source 3 is attributed to gasoline vehicle emissions because of the high OC concentration. The included Fe and Ca may be from the catalyst-equipped gasoline vehicles (CitationSchauer et al. 2002). The CPF plot of this source in shows a large contribution from the west where there is a high density of express ways and highways. The seasonal contribution variation plot of this source shows a relatively higher contribution in the summer. Summer is the travel season and I-70 is a major east-west interstate highway. This source did not have a significant weekend () effect. Recently CitationShah et al. (2004) reported that diesel vehicles operating at very slow speed or in stop-and-go mode produced OC/EC ratios that were like typical spark-ignition emissions. Thus, this factor could be mixed with some emissions of diesel vehicles slowly moving on major roads (not expressways). The contribution of this source is 16.6% to the total PM2.5 mass.
Source 4 represents diesel emission with higher EC. The diesel emissions include some Zn, Fe, and Si. Zn is in a motor oil additive, Fe is likely to be from muffler and brake ablation, and Si may be from road dust. The CPF plot in shows a relatively isotropic pattern with a somewhat larger contribution from the west, which is in the agreement with the road distribution of that area (ring-shape express way and a little bit higher density of major roads). The PSCF plot for this source in does not clearly reflect its source locations. This source does not show a significant weekend effect in , which is different from a number of other studies in which obviously higher weekday contributions were observed for diesel vehicle emissions (CitationKim and Hopke 2005). It appears that a little fewer but still significant amount of heavy duty vehicles operated in this area on the weekends. Indiannapolis is a metropolis (traffic hub) in the central United States, so some heavy duty vehicles across the continent could pass through this area without a rest on weekends according to schedule, which means there could be more diesel vehicles running on weekends in Indiannapolis than in many other areas. This could be one reason for the relatively weak weekend effect of this source. This source does not have a significant seasonal effect and contributes 7.9% to the total PM2.5 mass.
The fifth source is assigned to be airborne soil with its high abundances of Si, Ca, and Fe. There are three sharp peaks (4/22/01, 6/4/02, 5/27/03) in the temporal contribution plot of this source. Backward trajectories for April 22, 2001 and June 4, 2002 are shown in . The peak in the airborne soil contribution on 4/22/01 can be traced back to Asia. The contribution on 6/4/02 can be tracked backward to Africa across the Atlantic Ocean. These trajectories suggest the peak contributions could be the result of Asian and Africa Sahara dust events, respectively. Similar Asian dust impacts were also observed on 4/22/01 at Mammoth Cave National Park (CitationZhao and Hopke 2005) and the Bondville IMPROVE site (CitationKim et al. 2005b).
FIG. 9 Calculated backward air parcel trajectory plots for the high peaks in the contribution series of airborne soil in Indianapolis.
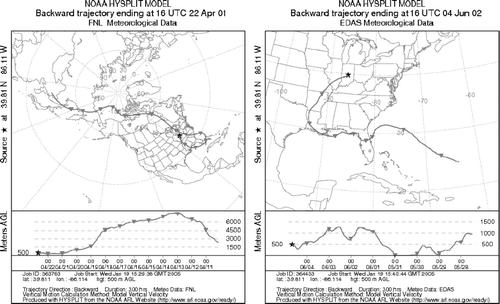
A number of studies have indicated the significant effect of the summer African dust on the air quality of Southeast and even central U.S. areas such as Illinois (CitationGatz and Prospero 1996; CitationEPA 2002) further supporting the hypothesis of Saharan dust being the origin of the soil on 6/4/02. Other summer dust storms were observed across the Midwest. The event observed on July 4, 2002 in Indianapolis was also observed across the Midwest. It was seen to have a maximum value on July 1 in St. Louis (CitationLee and Hopke 2005; CitationLee et al. 2005), but had large contributions on June 28 and July 7 as well. CitationKim et al. (2005b) observed the peak on July 1 with a substantial contribution on July 4. at Bondville, Illinois. The corresponding soil peak at Mammoth Cave National Park (CitationZhao and Hopke 2005) was observed on July 4 with a low contribution on July 1.
In addition, farm work in the spring and summer could also be a contributor to this source. This source can be considered as the combination of intercontinental dusts and local soil. The PSCF plot for this source shows a region in the southeastern United States. This southeastern area could be a major source area for this factor or simply the area through which the long range African dust passes. Additionally, this source shows a summer high pattern () with the highest contributions during the period from April to September when the Asian dusts, African dusts and the farm work occur. The weekend/weekday variation plot of this source shows the weekend contributions are a slightly lower than the weekday contributions and may be a consequence of the passage of a few of these large events on specific days. This source contributes 5.3% to the total PM2.5 mass.
The S, OC/EC, and relative high Fe suggest that source 6 might be Fe-smelters or Fe-related industries. The Indianapolis Casting Corp. and Indianapolis Engine Plant that fabricate diesel truck engines are just located to the south of the monitoring site. There is also a large coking plant to the south of the site. There are also Fe-related industries to the north of Indianapolis including a steel foundry in Attica and a gray iron foundry in New Castle. The emissions from these industries could explain the CPF plot that shows a relatively larger contribution from the south as well as a contribution from the north.
The PCSF plot of this source suggests the influence of the large Fe-related industries (steel mills) in the Chicago and Gary-Hammond area. However, their influences are likely to have been weakened during the transport to the sampling site so that local sources appear to dominate this factor. The weekday and weekend variation plot of this source shows a lower weekend factor value. The operating schedules of these Fe-related plants are not known, but the relatively low weekend contribution values follows the ratio of the average Fe concentration over weekends to that over weekdays. This source does not have a significant seasonal effect. Thus, this factor appears to be mixed local ferrous industry sources.
Source 7 shows S, OC, and some Cu, and the temporal contributions of this source have the highest correlation to Cu concentrations. This information suggests this source could be Cu-related industries. There are brass manufacturing companies to the north of Indianapolis, and copper rolling and drawing companies around Indianapolis. In particular, a copper rolling and drawing company is located to the south of the sampling site, which could explain the contribution from the south in the CPF plot. This source is thus assigned to be a combination of brass manufacturers and copper processing industries. The PSCF plot of this source does not provide any clear locations for the local Cu-related industries. This source has neither a significant weekend effect nor a seasonal effect.
Both of these sources represent relatively small contributions to the overall particulate mass concentrations, but the Fe-industries factor represents approximately 40% of the iron and 40% of the manganese variations and the Cu-industries factor produces 66% of the copper variations. Thus, they are important in identification of the sources of various trace metals in the airborne particulate matter.
The results of expanded factor analysis model are described in the supplemental material for this paper. Only slight differences between two models were observed and will be discussed in the supplementary material.
5. CONCLUSION
Seven sources for the ambient PM2.5 mass observed in Indianapolis were identified and the average contribution of each source was estimated. Secondary sulfate and nitrate were the two largest sources in the Indianapolis area. Indianapolis has significant quantities of gasoline and diesel vehicle emissions. A Fe-related source and a Cu-related source were resolved, and intercontinental transported dust episodes were observed. Regional sources like secondary nitrate, secondary sulfate, and intercontinental dust have more interpretable PSCF plots while the local sources had more interpretable CPF plots.
An expanded receptor model yielded the essentially the same results as the PMF model except that the wind direction factors for some sources of the expanded model are not in good agreement with the more interpretable CPF results. The comparison between two models suggests PMF coupled with downstream data analysis methods (such as CPF, PSCF, seasonal variation analysis, and weekday/weekend variation analysis) yields comparable results to expanded factor analysis and are simpler to employ.
This work was supported by the Lake Michigan Air Directors' Consortium (LADCO) through a contract to Clarkson University. The results presented here do not necessarily reflect the views of LADCO and no endorsement should be inferred. The authors would like to thank Donna Kenski and Michael Koerber of LADCO for their assistance in identifying the likely particle sources in Indianapolis. The authors also wish to acknowledge the NOAA Air Resources Laboratory (ARL) for the provision of the HYSPLIT transport model and READY website (http://www.arl.noaa.gov/ready.html) used in this publication.
REFERENCES
- Ashbaugh , L. L. , Malm , W. C. and Sadeh , W. Z. 1985 . A Residence Time Proability Analysis of Sulfur Concentrations at Grand Canyon National Park . Atmos. Environ. , 19 : 1263 – 1270 . [CSA]
- Begum , B. , Hopke , P. K. and Zhao , W. 2005 . Source Identification of Fine Particles in Washington DC by Expanded Factor Analysis Modeling . Environ. Sci. Technol. , 55 : 227 – 240 . [CSA]
- Birch , M. E. and Cary , R. A. 1996 . Elemental Carbon-Based Method for Monitoring Occupational Exposures to Particulate Diesel Exhaust . Aerosol Sci. Technol. , 25 : 221 – 241 . [CSA]
- Brook , J. R. , Dann , T. F. and Vet , R. 2004 . Time-Integrated Particle Measurements: Status in Canada , LA : EMEP Workshop on Particulate Matter Measurement & Modeling New Orleans .
- Draxler , R. R. and Rolph , G. D. 2003 . HYSPLIT (Hybrid Single-Particle Lagrangian Integrated Trajectory) , Silver Spring, MD : NOAA Air Resources Laboratory . Model Access Via NOAA ARL READY Website http://www.arl.noaa.gov/ready/hysplit4.html
- Dockery , D. W. , Pope , C. A. , Xu , X. P. , Spengler , J. D. , Ware , J. H. , Fay , M. E. , Ferris , B. and Speizer , F. E. 1993 . An Association Between Air-Pollution and Mortality in 6 United-States Cities . New Eng. J. Med. , 329 : 1753 – 1759 . [INFOTRIEVE] [CROSSREF] [CSA]
- EPA National Estuary Program . 2002 . Information About Estuaries and Near Coastal Waters 12 3; June 2002
- Gauderman , W. J. , McConnell , R. , Gilliland , F. , London , S. , Thomas , D. , Avol , E. , Vora , H. , Berhane , K. , Rappaport , E. B. , Lurmann , F. , Margolis , H. G. and Peters , J. 2000 . Association Between Air Pollution and Lung Function Growth in Southern California Children . Amer. J. Respir. Crit. Care Med. , 162 : 1383 – 1390 . [CSA]
- Gatz , D. F. and Prospero , J. M. 1996 . A Large Silicon-Aluminum Aerosol Plume in Central Illinois: North Africa Desert Dust? . Atmos. Environ. , 30 : 3789 – 3799 . [CROSSREF] [CSA]
- Goebesa , M. D. , Stradera , R. and Davidson , C. 2003 . An Ammonia Emission Inventory for Fertilizer Application in the United States . Atmos. Environ. , 37 : 2539 – 2550 . [CROSSREF] [CSA]
- Harrison , R. M. and Perry , R. 1986 . Handbook of Air Pollution Analysis, , 2nd ed. , Chapman and Hall .
- Hopke , P. K. , Barrie , L. A. , Li , S. -M. , Cheng , M. -D. , Li , C. and Xie , Y. 1995 . Possible Sources and Preferred Pathways for Biogenic and Non-Seasalt Sulfur for the High Arctic . J. Geophys. Res. , 100 : 16595 – 16603 . [CROSSREF] [CSA]
- Kim , E. , Hopke , P. K. , Paatero , P. and Edgerton , E. S. 2003 . Incorporation of Parametric Factors into Multilinear Receptor Model Studies of Atlanta Aerosol . Atmos. Environ. , 37 : 5009 – 5021 . [CROSSREF] [CSA]
- Kim , E. , Hopke , P. K. and Edgerton , E. S. 2004 . Improving Source Identification of Atlanta Aerosol Using Temperature Resolved Carbon Fractions in Positive Matrix Factorization . Atmos. Environ. , 38 : 3349 – 3362 . [CROSSREF] [CSA]
- Kim , E. , Hopke , P. K. and Qin , Y. 2005a . Estimation of Organic Carbon Blank Values and Error Structures of the Speciation Trends Network Data for Source Apportionment . J. Air and Waste Manag. Assoc. , 55 : 1190 – 1199 . [CSA]
- Kim , E. , Hopke , P. K. , Kenski , D. M. and Koerber , M. 2005b . Sources of in a Rural Midwestern U.S. Area . Environ. Sci. Technol. , 39 : 4953 – 4960 . [INFOTRIEVE] [CROSSREF] [CSA]
- Kim , E. and Hopke , P. K. 2005 . Improving Source Apportionment of Fine Particles in the Eastern U.S. Utilizing Temperature Resolved Carbon Fractions . J. Air Waste Manage. Assoc. , 55 : 1456 – 1463 . [CSA]
- Lee , J. H. and Hopke , P. K. 2005 . Apportioning Sources of PM2.5 in St. Louis, MO Using Speciation Trends Network Data, Atmospheric Environ. In Press
- Lee , J. H. , Hopke , P. K. and Turner , J. R. 2005 . Source Identification of Airborne PM2.5 at the St. Louis-Midwest Supersite . J. Geophys Res.—Atms. , 111 : D10S10 [CROSSREF] [CSA]
- Paatero , P. 1997 . Least Squares Formulation of Robust, Non-Negative Factor Analysis . Chemom. Intell. Lab. Syst. , 37 : 23 – 35 . [CROSSREF] [CSA]
- Paatero , P. 1999 . The Multilinear Engine—A Table-Driven Least Squares Program for Solving Multilinear Problems, Including the n-Way Parallel Factor Analysis Model . J. Comput. Graphical Stat. , 8 : 854 – 888 . [CROSSREF] [CSA]
- Paatero , P. and Hopke , P. 2002 . Utilizing Wind Direction and Wind Speed as Independent Variables in Multilinear Receptor Modeling Studies . Chemom. Intell. Lab. Syst. , 60 : 25 – 41 . [CROSSREF] [CSA]
- Paatero , P. , Hopke , P. K. , Song , X. H. and Ramadan , Z. 2002 . Understanding and Controlling Rotations in Factor Analytic Models . Chemom. Intell. Lab. Syst. , 60 : 253 – 264 . [CROSSREF] [CSA]
- Paatero , P. and Hopke , P. K. 2003 . Discarding or Downweighting High Noise Variables in Factor Analytic Models . Analytica Chimica Acta , 490 : 277 – 289 . [CROSSREF] [CSA]
- Polissar , A. V. , Hopke , P. K. , Malm , W. C. and Sisler , J. F. 1998 . Atmospheric Aerosol over Alaska: 2. Elemental Composition and Sources . J. Geophys. Res. , 103 : 19,045 – 19,057 . [CSA]
- Polissar , A. V. , Hopke , P. K. and Poirot , R. L. 2001a . Atmospheric Aerosol over Vermont Chemical Composition and Sources . Environ. Sci. Technology. , 35 : 4604 – 4621 . [CROSSREF] [CSA]
- Polissar , A. V. , Hopke , P. K. and Harris , J. M. 2001b . Source Regions for Atmospheric Aerosol Measured at Barrow, Alaska . Environ. Sci. Technol. , 35 : 4214 – 4226 . [INFOTRIEVE] [CROSSREF] [CSA]
- RTI . 2004 . “ Extended Semi-Annual Data Summary Report for the Chemical Speciation of PM2.5 Filter Samples Project ” . Research Triangle Park, NC RTI/07565/06DS
- Schauer , J. J. , Kleeman , M. J. , Cass , G. R. and Simoneit , B. R. T. 2002 . Measurement of Emissions from Air Pollution Sources. 5. C1-C32 Organic Compounds from Gasoline-Powered Motor Vehicles . Environ. Sci. Technol. , 36 : 1169 – 1180 . [INFOTRIEVE] [CROSSREF] [CSA]
- Seinfeld , J. H. and Pandis , S. N. 1998 . Atmospheric chemistry and physics, from air pollution to climate change , John Wiley and Sons, Inc .
- Shah , S. D. , Cocker , D. R. III , Wayne , J. M. and Norbeck , J. M. 2004 . Emission Rates of Particulate Matter and Elemental and Organic Carbon from In-Use Diesel Engines . Environ. Sci. Technol. , 38 : 2544 – 2550 . [INFOTRIEVE] [CROSSREF] [CSA]
- Song , X. , Polissar , A. V. and Hopke , P. K. 2001 . Sources of Fine Particle Composition in the Northeastern US . Atmos. Environ. , 35 : 5277 – 5286 . [CROSSREF] [CSA]
- Tolocka , M. P. , Solomon , P. A. , Mitchell , W. , Norris , G. A. , Gemmill , D. B. , Wiener , R. W. , Vanderpool , R. W. , Homolya , J. B. and Rice , J. 2001 . East Versus West in the US: Chemical Characteristics of PM2.5 During the Winter of 1999 . Aerosol Sci. Technol. , 34 : 88 – 96 . [CSA]
- Zhao , W. , Hopke , P. K. and Karl , T. 2004 . Source Identification of Volatile Organic Compounds in Houston, Texas . Environ. Sci. Technol. , 38 : 1338 – 1347 . [INFOTRIEVE] [CROSSREF] [CSA]
- Zhao , W. and Hopke , P. K. 2004 . Source Apportionment of Ambient Particles in the San Gorgonio Wildness . Atmos. Environ. , 38 : 5901 – 5910 . [CROSSREF] [CSA]
- Zhao , W. and Hopke , P. K. 2005 . Source Identification for Fine Aerosols in the Mammoth Cave National Park Area . Atmospheric Research , In Press[CSA]
- Zhou , L. , Kim , E. , Hopke , P. K. , Stanier , C. O. and Pandis , S. 2004 . Advanced Factor Analysis on Pittsburgh Particle Size-Distribution Data . Aerosol Sci. Technol. , 38 ( S1 ) : 118 – 132 . [CROSSREF] [CSA]