Ambient PM 2.5 composition data in Pittsburgh, PA have been used with Positive Matrix Factorization (PMF) to determine the major sources of PM 2.5 sampled. This paper describes the use of the potential source contribution function (PSCF) with the PMF-modeled source contributions to locate the sources in a grid of 0.1° × 0.1° cells. The domain extends from the Pittsburgh Supersite at 40.44°N, 79.94°W over the range 35°–50° north latitude and 75°–90° west longitude. Six-hour back trajectories have been obtained from HYSPLIT four times each day for the 13 months of the study for use with PSCF. Using the results, higher probability locations are compared with known locations of specific source types, based on information from the EPA Toxic Release Inventory (TRI) and the EPA AIRS Database. PSCF results for several sources are compared to the conditional probability function (CPF) analysis, which uses 15-minute wind direction data to determine the most probable direction of a source. Using PSCF and CPF together aids in interpretation of potential source regions. The selenium and sulfate factor source locations are regional, while the lead, cadmium, and specialty steel factor source locations are local. The gallium-rich and Fe, Mn, and Zn factor source locations are potentially both local and regional. The nitrate, vehicle emissions and road dust, wood combustion, vegetative detritus and cooking, and crustal material factor CPF and PSCF results were inconclusive as sources of these factors exist in all directions from the site and therefore one would not expect a clear probability field in any one direction.
INTRODUCTION
The concentrations of atmospheric fine particulate matter in Pittsburgh, Pennsylvania are largely affected by regional transport of PM2.5 as well as local source emissions (CitationTang et al. 2004). Positive Matrix Factorization (PMF), a source-receptor model, was used with data collected during the Pittsburgh Air Quality Study (PAQS) to determine the most significant sources of PM2.5 in the Pittsburgh region. Details of PMF use with these data are reported in CitationPekney et al. (2005a). The current paper uses the PMF-modeled source contributions with the potential source contribution function (PSCF) and conditional probability function (CPF) to locate the sources and to examine each source as being regional or local.
From July 2001 through July 2002, daily 24-hour filter-based samples were collected at the Pittsburgh Supersite, located in a park near the Carnegie Mellon campus approximately 6 km east of downtown Pittsburgh (CitationWittig et al. 2004). Filters were analyzed for PM2.5 sulfate, nitrate, organic carbon (OC), elemental carbon (EC), and trace elements. Samples collected on Teflon filters were analyzed by IC analysis for sulfate and nitrate concentrations (CitationTakahama et al. 2004). OC and EC were determined by the Thermal Optical Transmittance method and the NIOSH thermal evolution protocol, which used quartz filters for sample collection (CitationNIOSH 1996; CitationCabada et al. 2004). A multiplier of 1.8 was used to approximate total organic carbon mass from the OC measurements (CitationPolidori et al. 2005; CitationTurpin and Lim 2001). Samples collected on cellulose filters using a high volume sampler were analyzed for trace elements by ICP-MS after microwave-assisted acid digestion (CitationPekney and Davidson 2005). A TEOM recorded continuous total PM2.5 mass concentrations, which were averaged over 24 hours for use in this study. To determine the specific chemical components of OC, a cyclone/filterpack sampler collected 24-hour samples on a quartz filter followed by a polyurethane foam (PUF) plug. This sampler operated on a one-in-six day schedule for speciated OC analysis. Several of the OC species were used in the PMF analysis, including hopanes, resin acids, PAHs, syringols, and n-alkanes.
Positive Matrix Factorization
PMF is a source-receptor model that solves the equation:
With the Pittsburgh Supersite data, the PMF-modeled factors were identified as vehicle emissions and road dust; sulfate; nitrate; selenium; cadmium; lead; wood combustion, vegetative detritus and cooking; gallium-rich; Fe, Mn, and Zn; specialty steel production and processing; and crustal material. Source compositions and contributions to ambient PM2.5 levels are given and discussed in CitationPekney et al. (2005a).
The sulfate, nitrate, crustal material, vehicle emissions and road dust, and wood combustion, vegetative detritus and cooking factors all describe regional sources. The sulfate factor contributes the most to PM2.5 on an annual average, 33%. This factor has high concentrations of sulfate as well as a fraction of the OC. This is a secondary source of PM2.5, with particle formation from reactions of gases, with higher concentrations in summer due to an increase in photochemistry. The nitrate, crustal material, and vehicle emissions and road dust factors make smaller contributions to PM2.5, with 8%, 12%, and 11% annual average contributions, respectively. The nitrate factor, with high concentrations of nitrate and some OC, shows lower concentrations in summer due to the temperature sensitivity of nitrate to volatilization. The crustal material contribution, with calcium and titanium tracers, is slightly higher during the first three months of the study with high variability. The vehicle emissions and road dust factor (total OC, EC, hopanes, PAHs, and n-alkanes as tracers) is also highly variable but with no seasonal trend. The wood combustion, vegetative detritus, and cooking factor, with total OC, EC, n-alkanes, syringols, levoglucosan, and resin acid tracers, shows lower contributions during the first three months, with increases in contribution during winter when there is increased wood burning. This factor is approximately 4% of the PM2.5 mass concentration on an annual average.
The gallium-rich factor contributions correlate very strongly with ambient gallium concentrations, with significant contributions to this factor from arsenic, vanadium, nickel, and copper. While gallium is infrequently used in source-receptor modeling studies and known sources of gallium are few, the latter four elements can be emitted from a variety of sources. However, all of these elements present in trace amounts in coal and therefore this factor could represent primary emissions from coal combustion.
The Fe, Mn, and Zn, specialty steel production and processing, selenium, cadmium, and lead factors are probably local sources rather than regional. These factors are minor in their contribution to PM2.5, 1–3% on an annual average. Despite the minor contribution, importance of these factors can be explained by the possibility of adverse health effects due to PM2.5 composition rather than PM2.5 mass (CitationCarter et al. 1997; CitationMonn and Becker 1999). The source contributions are distinguished by low concentrations for much of the study with peaks on some days. Iron, manganese, and zinc tracers mark the steel production factor. Specialty steel production and processing tracers are molybdenum and chromium, which are both alloying elements in steel. Selenium, cadmium, and lead, are all factors with a unique elemental tracer. Selenium is associated with coal combustion, but this factor is identified by PMF as separate from the sulfate factor as it represents primary and not secondary emissions. Cadmium and lead can be emitted from a variety of industrial activities.
Conditional Probability Function
The Conditional Probability Function (CPF) calculates the probability that a source is located within a particular wind direction sector, Δ Θ, or:
Potential Source Contribution Function
PSCF calculates the probability that a source is located at latitude i and longitude j. The basis of PSCF is that if a source is located at (i, j), an air parcel back trajectory passing through that location indicates that material from the source can be collected and transported along the trajectory to the receptor site. PSCF solves
The Hybrid Single-Particle Lagrangian Integrated Trajectory (HYSPLIT) model was used to calculate air mass back-trajectories in 1-hour sequential increments ending at the receptor site at 40.44°N latitude and 79.94°W longitude (CitationDraxler 1999). HYPLIT was run four times a day for every day of the study, or every six hours starting at midnight EST from July 11, 2001 to July 31, 2002. A starting height of 500 m AGL was used. Six-hour back trajectories were selected because this time is sufficient to determine probable locations of both local and regional sources, and increasing the time of trajectories also increases the uncertainty, resulting in unrealistic source locations. However, this trajectory time is not sufficient to locate very distant sources that may be hundreds of kilometers away.
The trajectory domain extends from 35°–50° north latitude and 75°–90° west longitude, and is divided into 0.1° × 0.1° cells, resulting in 22,500 total cells. The maximum n ij is 1570, although many cells on the outer edges of the grid have n ij less than 10. These outer-edge cells could have misleading large PSCF values if a trajectory passed through them during a source contribution peak, resulting in mistaken attribution of a source to an area. To reduce the effect of spurious large ratios in grid cells with low n ij , a weighting function W ij is multiplied by the PSCF value:
RESULTS AND DISCUSSION
Wind direction in the Pittsburgh region is primarily from the southwest to northwest. shows the frequency of trajectories passing through the grid cells in the PSCF grid domain, or n ij . There are relatively few occurrences of wind direction from the east during the study period. The results of the PSCF analysis for seven of the eleven PMF-odeled source contributions are shown in , , , , , , . Cells are shaded according to the PSCF value, with the darkest cells indicating the highest probability of a source in that location. CPF results for six of the eleven sources are shown in , , , , , , and .
FIG. 1 The values of n ij from Equation (Equation4) for application of PSCF to this study.
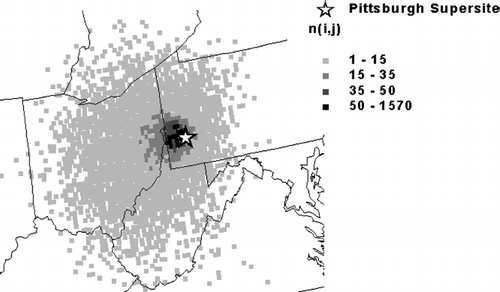
FIG. 2 PSCF and CPF results for the PMF-modeled sulfate source contributions. The top 25% of source contributions were used for m Δ θ and m ij . Sulfur dioxide sources are from the AIRS Database (CitationEPA 1999) given in tons emitted in 1997.
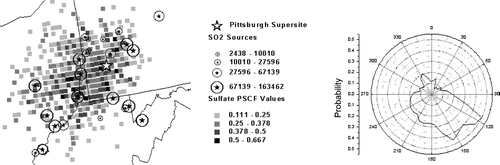
FIG. 3 PSCF and CPF results for the PMF-modeled selenium source contributions. The top 25% of source contributions were used for m Δ θ and m ij .
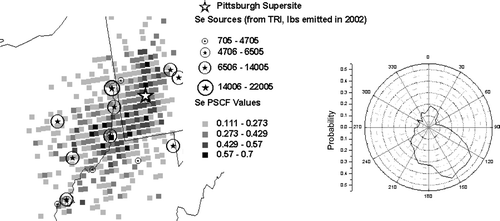
FIG. 4 PSCF and CPF results for the PMF-modeled specialty steel source contributions. The top 25% of source contributions were used for m Δ θ and the top 10% were used for m ij . Symbols for the sizes of the sources are based on the sum of Mo and Cr emissions given in the TRI.
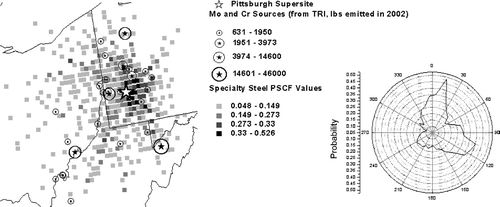
FIG. 5 PSCF and CPF results for the PMF-modeled lead source contributions. The top 25% of source contributions were used for m Δ θ and m ij .
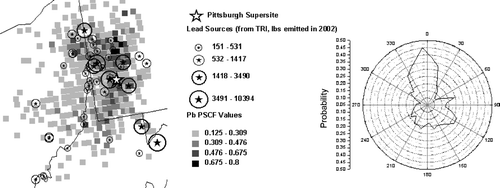
FIG. 6 PSCF results for the PMF-modeled cadmium source contributions. The top 25% of source contributions were used for m Δ θ and the top 10% were used for m ij .
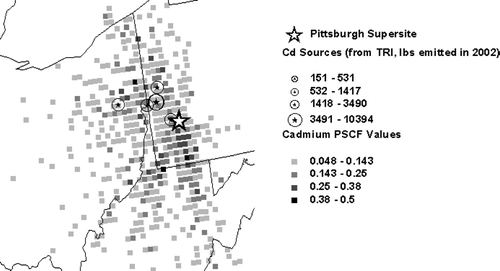
FIG. 7 PSCF and CPF results for the PMF-modeled gallium-rich source contributions. The top 25% of source contributions were used for m Δ θ and m ij . Symbols for the sizes of the sources are based on the sum of Ni, V, Cu, and As emissions given in the TRI.
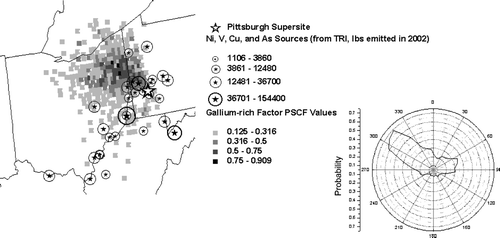
FIG. 8 PSCF and CPF results for the PMF-modeled Fe, Mn, and Zn source contributions. The top 25% of source contributions were used for m Δ θ and m ij . Symbols for the sizes of the sources are based on the sum of Zn and Mn emissions given in the TRI.
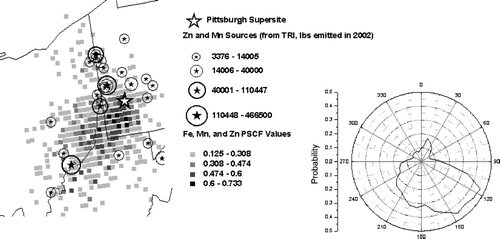
To compare the PSCF results with actual source locations in the region, the EPA Toxics Release Inventory (TRI) is used to identify locations of facilities that emit various elements, specifically the tracer elements for each source category, when available (CitationEPA 2002). The TRI lists toxic chemical releases and other waste management activities reported annually by certain industry groups and federal facilities in a public database. In this application, reported fugitive and point source releases to the air in 2002 are used. Source locations are shown in , , , , and , with symbols sized according to the emissions reported.
Sulfate is a major component of PM2.5 in Pittsburgh, comprising 38% on an annual average (CitationRees et al. 2004). The main source of sulfate in the area is from oxidation of the sulfur dioxide emitted from coal-fired power plants. The major sources of electricity in the region are from coal-fired power plants, and there are a large number of these sources in the area. The highest 25% factor contribution dates are used for determining m ij . PSCF results for the PMF-modeled sulfate factor contributions are shown in . The region of higher probability of sulfate factor locations is SW of the site in the Ohio River Valley. Sulfate is not reported in the TRI. However, sulfur dioxide is a criteria air pollutant and is listed in the AIRS Database (CitationEPA 1999) providing the source data shown in . With the exception of a petroleum and coal products facility in West Virginia, nearly all major sulfur dioxide sources are power plants, and most fall into the area of highest probability of source locations. The most probable direction of the sulfate factor as determined by CPF, also in , is to the SE of the site, rather than the Ohio River Valley. The reasons for the discrepancy are unknown. The indication that the Ohio River Valley area is a major source of secondary sulfate has been reported in other studies using PMF and PSCF (CitationPolissar et al. 2001; CitationLee et al. 2002; CitationLiu et al. 2003), influencing regions as far north as upstate New York, New Jersey, and Vermont.
Selenium factor PSCF results are shown in . Selenium in PM2.5 is typically associated with combustion of coal. Selenium is a primary pollutant released from burning coal, while sulfate is a secondary pollutant, so it is not surprising that these two factors are defined separately by the PMF analysis. Furthermore, most of the individual point sources of sulfur dioxide in are the same as those shown in for selenium. Selenium factor contributions are usually a low background with occasional high peaks, many more than the cadmium factor contributions, so the 25% highest factor contribution dates are used rather than 10% highest. The selenium factor shows highest probabilities of being located SW of the site, following the Ohio River, and to a lesser extent NE of the site. This is in good agreement with sources identified in the TRI as shown in . Highest probabilities of a selenium source in a particular cell reach 0.70. TRI emissions of selenium and selenium compounds in the region are all from electric services, known to be coal-fired power plants, as well as one flat glass production facility and one refuse system facility. Like the sulfate factor, CPF results for this factor () indicate a most probable direction to the SE rather than SW, with a maximum probability of 0.45. As for sulfate, the reasons for this discrepancy are unknown.
The specialty steel production and processing factor is marked by molybdenum and chromium tracers, as both are alloying elements in specialty steels. The highest 10% factor contribution dates are used for m ij as there are fewer dates of high concentrations for this factor. PSCF results in show highest probability of source locations clustered nearest the site, indicating that this is most likely a local source. There are many specialty steel production and processing facilities in the greater Pittsburgh region.
There are only seven known sources of molybdenum in the region (molybdenum trioxide as reported in the TRI) with industry types listed in . The molybdenum sources are located to the N and W of the site. There are many more sources of chromium in the area. Industry types include the same listed for molybdenum trioxide emissions as well as others shown in . Chromium sources are located in all directions from the Supersite. PSCF shows highest probability of specialty steel source locations immediately N, E, and S of the site. These locations correspond to many of the chromium and molybdenum sources. CPF corroborates these findings, with most probable directions to the NNE and ESE ().
TABLE 1 Summary of sources identification from PMF, CPF, and PSCF analysis. Annual average contribution each source makes to fine particle mass is given as percent of annual average PM2.5 mass
Source locations of the lead factor are difficult to locate using PSCF and CPF, because there are many small point sources of lead in this region. Resuspension of lead particles that were initially emitted during the era of leaded gasoline use can be distributed across a large area, making this source possibly regional as well as local. Industries reporting lead emissions in the TRI are listed in . In , the PSCF results show a higher probability that the lead factor is located mostly to the N and also S of the site. The highest 25% of factor contribution dates were used for m ij . The results agree reasonably well with the location of many lead point sources to the N, but reasons for the higher probabilities S of the site are less clear. CPF results are somewhat consistent with the PSCF results with N as the most probable direction ().
shows the results for the cadmium factor PSCF. Cadmium source contributions are mostly at background levels, but with a few days of concentration 10–100 times the background. These high concentrations are indicative of local sources that impact the receptor site under the right meteorological conditions. Therefore, to determine m ij , the 10% highest source contribution dates are used. The cadmium factor shows a higher probability of being located to the NW, SW, and S directions from the site. However, the locations of cadmium sources in the region according to the TRI are mostly N and NW, but none S of the site. It is possible that there are sources of cadmium in that direction, but the facilities do not report emissions to the TRI. The highest probabilities for the cadmium factor reach 0.50. Several industry types are responsible for the Cd emissions shown in ; those given in the TRI are listed in . In using CPF (results not shown), the probability of a cadmium source in any direction is less than 0.25. This low probability renders the CPF results inconclusive.
The gallium-rich factor shows surprising results with the PSCF analysis (). The highest 25% factor contribution dates were used for m ij . Gallium ambient concentrations correlate strongly with this factor, but there are also high contributions from arsenic, vanadium, nickel, and copper. Therefore, this factor was assumed to be related to coal combustion, as all of these elements are present in trace amounts in coal. Major coal-fired power plants are located W and SW of the site with some sources identical to those shown for selenium and sulfate. In contrast, PSCF results show a clear directional preference to the NW, with very high probabilities of 0.75–0.91. CPF results agree well with PSCF results for this factor, with a high probability of 0.70 that the source is located to the NW (). Gallium is not a toxic chemical and thus is not listed in the TRI; however, all of the other elements are listed. Besides being found in coal fly ash, gallium is a by-product of bauxite processing, as it is associated with aluminosilicates. Gallium and arsenic are used in the microelectronics industry to make GaAs wafers. There are several microelectronics, optics, and photonics companies in northeast Ohio that have foundries for processing aluminum and list GaAs wafers as one of their products. While there are a couple coal-fired power plants NW of the site, PMF distinguished this gallium-rich factor as separate from the sulfate and selenium factors associated with all coal-fired power plants in the region due to the influence of gallium emissions from unknown NW sources.
PSCF results for the Fe, Mn, and Zn factor, shown in , are inconclusive as the highest probability areas identified by PSCF do no coincide with zinc and manganese sources as reported in the TRI. PSCF, using the highest 25% factor contribution dates, shows highest probability of this factor being located to the SW. However, zinc sources are mostly located to the NW and manganese sources are in every direction. Iron, also a tracer species for this factor, is not a toxic chemical listed in the TRI and hence the emissions and their locations are unknown. Industry types that report emissions of zinc and manganese in the TRI are listed in . CPF results () indicate SE as the most probable direction, which further complicates determining the location of sources of this factor.
There are several factors determined by PMF that do not yield conclusive results from the PSCF or CPF analyses: nitrate; wood combustion, vegetative detritus and cooking; crustal material; and vehicle emissions and road dust. These source categories exist in all directions from the Supersite and therefore one would not expect a clear probability field in any one direction. Therefore, PSCF and CPF results are not shown for these sources. Brief summaries are listed for all factors in .
CONCLUSIONS
PSCF and CPF results for the PMF factors presented can be grouped into three different categories: regional sources, local sources, or potentially both regional and local sources. The sulfate and selenium factors represent regional sources identified as coal fired power plants located regionally. For these factors, the PSCF results show most probable source locations in agreement with TRI or AIRS Database source locations while the CPF results show a slightly offset most probable direction that does not agree well with the PSCF results. However, for the specialty steel and lead factors, which represent local sources, CPF results show clear most probable directions that agree well with both the PSCF results, which show a clustering of most probable locations nearest the site, and the known locations of molybdenum, chromium, and lead sources by TRI. Although the cadmium factor is assumed to also represent a local source or sources, the CPF results were inconclusive, with no direction showing a strongly significant probability. The gallium-rich and Fe, Mn, and Zn factors could represent either local or regional sources, or both. The gallium-rich factor PSCF and CPF results show very good agreement but as this source cannot be identified, a conclusion as to its being regional or local cannot be drawn. And while the Fe, Mn, and Zn factor PSCF and CPF results are similar to results for the sulfate and selenium factors, the difference that there are known local Mn and Zn sources is the direction indicated as most probable by CPF. Therefore, this factor can be considered both regional and local. In conclusion, PSCF appears to better predict locations of regional sources while CPF is useful in pinpointing directions of local sources.
presents a summary of the findings presented in this paper. PMF-modeled factor names are given with their tracer species, annual average contribution to PM2.5 mass concentrations in Pittsburgh, source regions as determined by the PSCF and CPF analyses, and possible sources as identified by the tracer species and the TRI. Overall, the results for several of the source categories are consistent with single particle composition data from the PAQS (CitationPekney et al. 2005b). PMF, CPF, and PSCF are powerful tools for determining the major sources of particulate pollution in an urban area like Pittsburgh, but each model has its limitations. Both models rely on counting statistics and therefore require large amounts of data such that results are statistically significant. PMF cannot distinguish between sources in the same area that impact the receptor location. This may lead to a mixing of different types of sources that makes it difficult to determine the apportionment of source contributions to PM2.5 mass concentrations. CPF can provide the probability that a source is located in a particular direction, but cannot provide any information as to the proximity of the source. PSCF, however, provides probability that a source is located in a particular grid cell, but is dependent upon the accuracy of the HYSPLIT model. HYSPLIT trajectory accuracy decreases with increasing trajectory time, and effects of the boundary layer are not considered. In an urban area like the Pittsburgh, where there are many industrial activities in and near the city as well as in the surrounding heavily industrialized region, clearly identifying source locations using any of these models is difficult. High concentrations of some pollutant species can occur for a wide range of wind directions. Furthermore, mixing height as well as wind direction can affect airborne concentrations of the tracer species, although only the latter is used in these models. When 24-hour data are used, source plumes that occur on the order of minutes are masked due to the variability of wind direction over a day. Despite these limitations, using PMF, CPF, and PSCF together determined probable locations for several of the modeled sources that have been shown to correlate with the locations of known sources as reported to the TRI. This study demonstrated that CPF and PSCF aided in determining PM source locations to significantly improve source apportionment study.
REFERENCES
- Ashbaugh , L. , Malm , W. and Sadeh , W. 1985 . A Residence Time Probability Analysis of Sulfur Concentrations at Ground Canyon National Park . Atmos. Environ. , 19 ( 8 ) : 1263 – 1270 . [CSA]
- Cabada , J. C. , Khlystov , A. , Wittig , E. , Pilinis , C. and Pandis , S. 2004 . Light Scattering by Fine Particles During the Pittsburgh Air Quality Study: Measurements and Modeling . J. Geophys. Res. , 109 : D16S03 [CROSSREF] [CSA]
- Carter , J. D. , Ghio , A. J. , Samet , J. M. and Devlin , R. B. 1997 . Cytokine Production by Human Airway Epithelial Cells After Exposure to an Air Pollution Particle Is Metal-Dependent . Toxicol. Appl. Pharmacol. , 146 : 180 – 188 . [INFOTRIEVE] [CROSSREF] [CSA]
- Draxler , R. R. 1999 . Silver Spring, MD : Air Resources Laboratory . HYSPLIT_4 User's Guide, NOAA Technical Memorandum ERL ARL-230
- Malm , W. C. , Johnson , C. E. and Bresch , J. F. 1986 . “ Application of Principal Component Analysis for Purposes of Identifying Source-Receptor Relationships ” . In Receptor Methods for Source Apportionment , Edited by: Pace , T. G. Pittsburgh, PA : Air Pollution Control Association . Publication TR-5,
- Monn , C. and Becker , S. 1999 . Cytotoxicity and Induction of Proinflammatory Cytokines from Human Monocytes Exposed to Fine (PM2.5) and Coarse Particles (PM10–2.5) in Outdoor and Indoor Air . Toxicol. Appl. Pharmacol. , 155 : 245 – 252 . [INFOTRIEVE] [CROSSREF] [CSA]
- Lee , J. H. , Yoshida , Y. , Turpin , B. Hopke . 2002 . Identification of Sources Contributing to Mid-Atlantic Regional Aerosol . J. Air and Waste Manag. Assoc. , 52 : 1186 – 1205 . [CSA]
- Liu , W. , Hopke , P. , Han , Y. Yi , S. 2003 . Appliation of Receptor Modeling to Atmospheric Constituents at Potsdam and Stockton. NY . Atmos. Environ. , 37 : 4997 – 5007 . [CROSSREF] [CSA]
- NIOSH . 1996 . “ Elemental Carbon (Diesel Exhaust) ” . In NIOSH Manual of Analytical Methods , Cincinnati, OH : National Institute of Occupational Safety and Health .
- Paatero , P. and Tapper , U. 1993 . Analysis of Different Modes of Factor Analysis as Least Square Fit Problems . Chemometrics and Intelligent Laboratory Systems. , 18 : 183 – 194 . [CROSSREF] [CSA]
- Paatero , P. and Tapper , U. 1994 . Positive Matrix Factorization: A Non-Negative Factor Model with Optimal Utilization of Error Estimates of Data Values . Environmetrics , 5 : 111 – 126 . [CSA]
- Paatero , P. 1997 . Least Squares Formulation of Robust Non-Negative Factor Analysis . Chemometrics and Intelligent Laboratory Systems , 37 : 15 – 35 . [CROSSREF] [CSA]
- Pekney , N. , Davidson , C. , Robinson , A. Zhou , L. 2005a . Identification of Major Sources of PM2.5 in Pittsburgh using PMF and Unmix . Aerosol Sci. Technol. , in press[CSA]
- Pekney , N. , Davidson , C. , Bein , K. , Wexler , A. and Johnston , M. 2005b . Identification of Sources of Atmospheric PM at the Pittsburgh Supersite Part I: Single Particle Analysis and Filter-Based Positive Matrix Factorization . Atmos. Environ. , in press[CSA]
- Pekney , N. and Davidson , C. I. 2005 . Determination of Trace Elements in Ambient Aerosol Samples . Analytica Chimica Acta. , 540 : 269 – 277 . [CROSSREF] [CSA]
- Polidori , A. , Turpin , B. , Lim , H. , Totten , L. and Davidson , C. 2005 . Polarity and Molecular Weight/Carbon Weight of the Pittsburgh Organic Aerosol Manuscript in preparation
- Polissar , A. , Hopke , P. and Poirot , R. 2001 . Atmospheric Aerosol over Vermont: Chemical Composition and Sources . Environ. Sci. Technol. , 35 : 4604 – 4621 . [INFOTRIEVE] [CROSSREF] [CSA]
- Rees , S. L. , Robinson , A. L. , Khylstov , A. , Stanier , C. O. and Pandis , S. 2004 . Mass Balance Closure and the Federal Reference Method for PM2.5 in Pittsburgh, Pennsylvania . Atmos. Environ. , 38 : 3305 – 3318 . [CROSSREF] [CSA]
- Schauer , J. J. , Rogge , W. F. , Hildermann , L. M. , Mazurek , M. A. and Cass , G. R. 1996 . Source Apportionment of Airborne Particulate Matter using Organic Compounds as Tracers . Atmos. Environ. , 30 : 3837 – 3855 . [CROSSREF] [CSA]
- Simoneit , B. R. T. 1989 . Organic Matter of the Troposphere. 5. Application of Molecular Marker Analysis to Biogenic Emissions into the Troposphere for Source Reconciliations . J. Atmos. Chem. , 8 : 251 – 275 . [CROSSREF] [CSA]
- Simoneit , B. R. T. , Rogge , W. F. , Mazurek , M. A. , Standley , L. J. , Hildemann , L. M. and Cass , G. R. 1993 . Lignin Pyrolosis Products, Lignans, and Resin Acids as Specific Tracers of Plant Classes in Emissions from Biomass Combustion . Environ. Sci. Technol. , 27 : 2533 – 2541 . [CROSSREF] [CSA]
- Simoneit , B. R. T. , Schauer , J. J. , Nolte , C. G. Oros , D. R. 1999 . Levoglucosan, A Tracer for Cellulose in Biomasa Burning and Atmospheric Particles . Atmos. Environ. , 33 : 173 – 182 . [CROSSREF] [CSA]
- Takahama , S. , Wittig , B. , Vayenas , D. Davidson , C. I. 2004 . Modeling the Diurnal Variation of Nitrate During the Pittsburgh Air Quality Study . J. Geophys. Res.—Atmos. , 109 ( D16 ) Art. No. D16S06[CSA]
- Tang , W. , Raymond , T. , Wittig , E. Davidson , C. I. 2004 . Spatial Variations in PM2.5 During the Pittsburgh Air Quality Study . Aerosol Sci. Technol. , 38 ( 1 ) : 80 – 90 . [CROSSREF] [CSA]
- Turpin , B. and Lim , H. 2001 . Species Contributions to PM2.5 Mass Concentrations: Revisiting Common Assumptions for Estimating Organic Mass . Aerosol Sci. Technol. , 35 : 602 – 610 . [CSA]
- U.S. EPA . 1999 . AirData Website (http://www.epa.gov/air/data/index.html, 1999 data used, accessed 2004
- U.S. EPA . 2002 . 2002 TRI Data Release http://www.epa.gov/tri. Accessed 2004
- Watson , J. G. , Chow , J. C. and Houck , J. E. 2001 . PM2.5 Chemical Source Profiles for Vehicle Exhaust, Vegetative Burning, Geological Material, and Coal Burning in Northwestern Colorado During 1995 . Chemosphere , 43 : 1141 – 1151 . [INFOTRIEVE] [CROSSREF] [CSA]
- Wittig , A. E. , Anderson , N. J. , Khlystov , A. Y. Pandis , S. N. 2004 . Pittsburgh Air Quality Study overview . Atmos. Environ. , 38 : 3170 – 3125 . [CSA]