Abstract
The Community Multiscale Air Quality (CMAQ) model is widely used in air quality management and scientific investigation. Numerous studies have been conducted investigating how well CMAQ simulates fine particle mass concentrations, but relatively few studies have addressed how well CMAQ simulates fine particle number distribution. Accurate simulation of particle number concentrations is important because particle number and surface area concentrations may be directly related to human health and visibility. Simulated fine particle number concentrations derived using CMAQ are compared to measurements to identify problems and to improve model performance. Evaluation is done using measured particle number concentrations in Atlanta, Georgia, from 1/1/1999 to 8/31/2000. While homogeneous binary nucleation mechanism used in CMAQ needs to be modified for better prediction of particle number concentrations, there are also other factors that affect the predicted particle level. Assumed particle size of the primary emissions in CMAQ causes number concentrations to be significantly underestimated, while particle density has a small impact. Assuming particle size distributions by three lognormal modes cannot accurately simulate particles with size less than 0.01 μ m, particularly during nucleation events. An additional mode that accounts for particles smaller than 0.01 μ m can improve the accuracy of the number concentration simulations. Though, the use of the Expectation-Maximization (EM) algorithm to estimate size distribution parameters of measured particles suggests that assumed parameters for the lognormal modes in CMAQ are generally reasonable.
1. INTRODUCTION
Elevated levels of ambient particles have a direct influence on human health; for example, they can trigger reactions ranging from coughing and wheezing to heart attacks and premature death (CitationDonaldson et al. 2001). Particles are also responsible for visibility reduction (CitationAdrian and Orloff 1977; CitationFaxvog and Roessler 1978), acid deposition, and perturbing the Earth's radiation balance. In 1997, the National Ambient Air Quality Standards (NAAQS) for fine particle mass concentrations were promulgated in response to a growing number of scientific linkages between fine particle levels and adverse health effects. In addition to studies associating particle mass concentrations with the human health (CitationBrauer et al. 2001; CitationDockery and Pope 1994; CitationDockery et al. 1993; CitationEbelt et al. 2000; CitationGold et al. 1999; CitationPope et al. 1995; Citation1999; CitationSaldiva et al. 1995; CitationVedal 1997), some studies suggest that fine particle number and surface area concentrations are more directly related to pulmonary disease than particle mass concentrations (CitationDonaldson et al. 1998; CitationPenttinen et al. 2001; CitationWoo et al. 2001b). In addition, particle mass concentrations are not always correlated with particle number concentrations, for example, when very small particles are formed by nucleation, particle mass does not significantly increase (CitationWoo et al. 2001a). Hence, if particle number or surface area concentrations are responsible for human health, strategies designed to meet the NAAQS for PM2.5 might not be as effective for protecting human health as desired (CitationWoo et al. 2001a). These studies also suggested that the particle number concentration itself was an important index in epidemiological analyses.
The physics, chemistry, and the response of particles to emissions have been studied using air quality models. One of the most comprehensive air quality modeling frameworks is Models-3, composed of the Community Multiscale Air Quality (CMAQ) model (CitationByun and Ching 1999), the Mesoscale meteorological Model version 5 (MM5) (CitationPSU/NCAR 2003), and Sparse Matrix Operator Kernel Emissions (SMOKE) (CitationUS-EPA 2004). CMAQ predicts gas-phase species as well as particle concentrations. Particles in CMAQ are divided into two groups, fine (PM2.5: particles with size less than 2.5 μ m) and coarse particles (PM10: particles with size between 2.5 and 10 μ m).
PM2.5 is treated by two interacting sub-distributions (or modes) in CMAQ. The Aitken (smaller one) mode represents fresh particles either from nucleation or from direct emissions, while the accumulation (larger one) mode represents aged particles. The production rate of new particle number, J [m− 3 sec− 1], is calculated from the nucleation of sulfuric acid vapor, based the classical binary homogeneous nucleation theory (CitationWilemski 1984) incorporating the hydration effect (CitationKulmala et al. 1998). New particle diameter in CMAQ v4.3 is 2.0 nm. The two modes interact with each other through coagulation, and particles in the Aitken mode may grow into the accumulation mode. PM2.5 chemical species treated in CMAQ are sulfate, nitrate, ammonium, water, anthropogenic and biogenic organic carbon, elemental carbon, and other unspecified material of anthropogenic origin. PM10 is treated by the coarse mode from direct emissions by natural processes such as blowing dust or sea salt particles from waves in CMAQ. PM10 includes sea salt, wind-blown dust, and other unspecified material of anthropogenic origin.
Fine particle mass concentrations simulated using CMAQ have been extensively evaluated using relatively abundant measurement data (CitationEder et al. 2003; CitationFan et al. 2005; CitationJun and Stein 2004; CitationMebust et al. 2003; CitationPark et al. 2006; CitationTonnesen 2003). Initial evaluation of the aerosol species concentrations over the eastern United States in June 1995 (CitationMebust et al. 2003) revealed that CMAQ underestimated particle mass with the normalized mean error less than 50% for most of the species. Recently, CMAQ was used to simulate fine particle concentrations in Houston, Texas, from August 24 to August 31, 2000 (CitationFan et al. 2005). Daily average concentrations of PM2.5 major species were simulated reasonably well with the normalized bias less than 25%. Those differences are likely due to the uncertainties in the calculated morning planetary boundary layer (PBL) height. Secondary organic aerosol was also underestimated. CMAQ was also used to investigate aerosol formation and transport in the Pacific Northwest during July 1–16, 1996 (CitationO'Neill et al. 2006). The mean fractional bias and error of PM2.5 mass were within 30% and 50%, respectively, and those of PM2.5 species concentrations were within the acceptable bound (CitationBoylan et al. 2006). Further, effects of spatial variability on CMAQ evaluation found that the uncertainties due to the different spatial scales between the point-observations and volume-averaged simulated concentrations did not significantly affect model errors (CitationPark et al. 2006).
In spite of these extensive studies focusing on the evaluation of the fine particle mass concentration, CMAQ's ability to reproduce aerosol number concentrations has not been addressed in such detail. A few studies have found that CMAQ under predicts aerosol number concentrations of the Aitken mode (i.e., particles with size less than 0.1 μ m) by a factor of 10 to 100, and those of the accumulation mode (i.e., less than about 2 μ m in the diameter) by a factor of 5 to 10 in the Pacific Northwest (CitationElleman et al. 2004, Citation2005). Errors in particle number concentrations were significantly larger than those of particle mass concentrations or gas-phase constituents. Thus, the authors concluded that the modeled mean particle size in CMAQ might be significantly larger than the measured mean size due to the inadequate treatment of aerosols as three lognormal modes or inaccurate processes affecting characterization of the aerosol size distribution.
Studies also suggested that the particle numbers were underestimated because CMAQ used the binary homogeneous nucleation theory (H2SO4 and water), which significantly underestimate the nucleation rates (CitationClarke et al. 1999; CitationCoffman and Hegg 1995; CitationGaydos et al. 2005; CitationKulmala et al. 2006; CitationStolzenburg et al. 2005; CitationWeber et al. 1996; Citation1999). One process that could reconcile particle production is the ternary nucleation mechanism. Among several ternary systems, the H2O-H2SO4-NH3 system can produce the most realistic particle production (CitationCoffman and Hegg 1995; CitationGaydos et al. 2005; CitationKorhonen et al. 1999). The accuracy of the nucleation rates was significantly improved at higher nucleation rates when using the H2O-H2SO4-NH3 system (CitationNapari et al. 2002). An appropriate formulation of this ternary system increased simulated nucleation rates (CitationGaydos et al. 2005). In addition to the ternary systems, the ion-mediated nucleation mechanism can produce new particles in a very low H2SO4 concentrations that are unfavorable for binary homogeneous nucleation (CitationKatz et al. 1994; CitationLaakso et al. 2002; CitationYu and Turco 2000).
While uncertainties in nucleation theory will continue to impact CMAQ, other factors also affect predicted particle concentrations, and CMAQ relies on several assumptions: assumed particle density, assumed size distribution of primary emissions, and assumed distribution of particle size. The goal of this research is to analyze the influence of those assumptions on the predicted particle number concentrations. The evaluation uses hourly measured particle number concentrations of 39 size bins from 3 nm to 3 μ m for one year and eight months (1/1/1999 to 8/31/2000) at an urban area located in Atlanta, Georgia. In addition, this paper also examines the feasibility to improve the accuracy of the simulations.
2. METHODS
Fine particle number and mass levels were monitored at the Jefferson Street (JST) monitor in urban Atlanta, Georgia () from 1/1/1999 to 8/31/2000 as a part of the Southeastern Aerosol Research and Characterization (SEARCH) study (CitationHansen et al. 2003; CitationWoo et al. 2001a). Hourly particle number concentrations were measured for 39 size bins from 3 nm to 3 μ m in diameter using a nano-scanning mobility particle sizer (NSMPS) (3 nm–50 nm), a standard scanning mobility particle sizer (SMPS) (20 nm–250 nm), and a laser particle counter (LPC) (100 nm–2 μ m) (CitationVan Loy et al. 2000; CitationWoo et al. 2001a). Hourly PM2.5 mass concentrations were measured using a Tapered Element Oscillating Microbalance (TEOM) (CitationPatashnick and Rupprecht 1991), which measures ambient particle concentrations using the oscillation frequency of the mass transducer.
FIG. 1 (a) Location of the Jefferson Street (JST) monitoring station in Atlanta, Georgia. (b) Air quality model (CMAQ) domain.
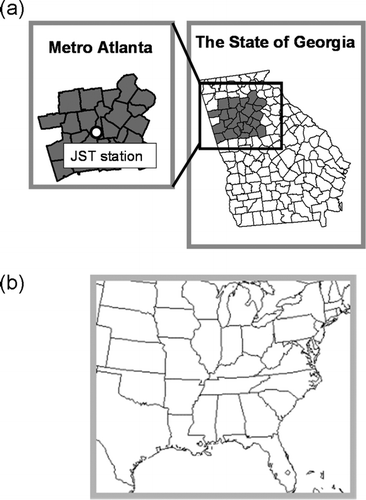
Hourly particle number and mass concentrations were simulated using EPA's Models-3 framework: SMOKE (v1.5), MM5 (v3.5.3), and CMAQ (v4.3). CMAQ was run from 1/1/1999 to 8/31/2000 over the eastern United States with 78 × 66 grids of size 36 km () for a time-series epidemiological study (CitationMarmur et al. 2006). Evaluating simulated number concentrations based on a finer modeling grid (e.g., 12 km) would have provided more spatially detailed data, and possibly improved model performance. However, comparing model results based on 36 km and 12 km grids, these differences are negligible compared to the discrepancies between observed and simulated (based on a 36 km grid) number concentrations (see “Results” section). Detailed information of the parameters used in the modeling system is available in elsewhere (CitationPark et al. 2006). Meteorological fields were evaluated using the TDL surface hourly data (CitationUCAR 2003), which were not used as an input of MM5. Mean errors (MEs) in temperature, specific humidity, and wind speed were 1.5°C, 1.8 g kg−1, and 0.8 m sec− 1, respectively. These values are within the benchmarks for the metrological model evaluation (CitationEmery et al. 2001).
Particle number concentrations are measured in each of 39 size bins, but CMAQ concentrations are simulated using three modes, which make a direct comparison cumbersome. The three modes, Aitken(i), accumulation(j), and coarse(c), follow a lognormal distribution. To compare the simulated concentrations with the measured concentrations, modal particle number concentrations from CMAQ were converted into size resolved particle number concentrations expressed in the aerodynamic diameter using PMx (Jiang 2005; CitationJiang et al. 2004; CitationJiang and Yin 2001). PMx estimates the parameters of the lognormal distribution in each mode using particle number, surface area, and mass concentrations. PMx calculates particle number concentrations in each size bin by the integral of the probability density function of particle number concentrations for each interval. Accuracy of the particle concentrations in each size bin is dependent on the simulated particle number, surface area, and mass concentrations.
Parameters of the lognormal distributions can be obtained from CMAQ's diagnostic output variables (CitationBhave and Binkowski 2005). Different parameters in the lognormal distributions lead to changes in size resolved particle number concentrations (). Number concentrations for particles with sizes between 3 to 500 nm are a slightly higher when PMx is used, and concentrations for particles with size between 0.5 μ m to 2.5 μ m are somewhat higher when CMAQ's diagnostic output variables are used. However, the differences are negligible for total particle number concentrations less than 2.5 μ m, and those differences do not significantly affect the results of the analysis performed in this paper. Also, size resolved particle number concentrations are calculated from modal concentrations from CMAQ based on the aerodynamic diameter. Size resolved concentrations differ slightly when using other kinds of diameters, such as Stokes or electric mobility diameter. Differences do not significantly influence the analysis.
TABLE 1 Geometric mean (Dg) and geometric standard deviation (σg) of lognormal distributions obtained from PMx and CMAQ's diagnostic variables from 3/23/1999 to 3/30/1999 at the JST station in Atlanta, Georgia
3. RESULTS
Simulated fine particle number concentrations were significantly lower than measured concentrations by a factor of up to 1000 (). Note that the y-axis is logarithmic. Simulated surface area concentrations were also much lower than measured values, whereas simulated volume and mass concentrations reasonably well match the measured values. In addition, CMAQ underestimated number concentrations for size less than 1 μ m, and overestimated concentrations for size larger than 1 μ m (). These results suggested that CMAQ overestimated average particle sizes. Note that measured surface area and volume concentrations are calculated using number concentrations by assuming that all particles are spheres. CMAQ also assumes that particles are in a spherical shape. The spherical assumption is not correct; for example, diesel chain agglomerates are not spherical, so there exist small errors in calculated surface area and volume concentrations associated with the particle shape (CitationMcMurry et al. 2002; CitationPark et al. 2004a). These errors were not quantified in this paper as this issue is not within the scope of this study. However, note that the correct estimation of the particle surface area is needed to accurately estimate the particle number concentrations. For example, the particle deposition velocity, which is directly related with the lifetime of particles, depends on the surface area. In addition, the surface area is important for the human health study.
FIG. 2 Daily particle number, surface area, volume, and mass concentrations for particles less than 2.5 μ m from 1/1/1999 to 8/31/2000 at the JST station in Atlanta, Georgia.
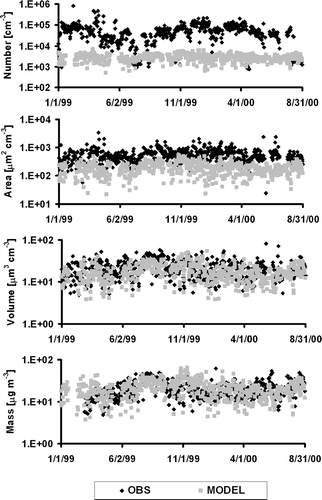
FIG. 3 Median number concentrations of particles for 39 size bins (from 3 nm to 2.5 μ m) from 1/1/1999 to 8/31/2000 at the JST station in Atlanta, Georgia.
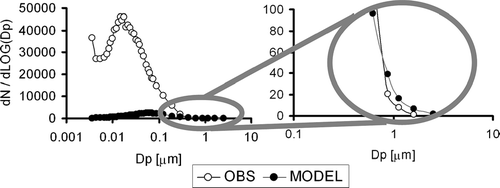
The discrepancy between measured and simulated particle number concentrations could be partly due to the different spatial scales between the observation and the model (CitationPark et al. 2006). Measured concentrations are point values at the monitoring station, whereas CMAQ simulates volume-averaged concentrations. To analyze the effect of the different spatial scales, particle number concentrations were simulated using 12 km grids as well as 36 km grids from July 1–31, 2001 in a separate study (CitationPark and Russell 2003). Number concentrations at the JST station from the 12 km grid were 17% higher than those from the 36 km. Thus, a fraction of the error in simulated number concentrations arises from the different spatial scales.
Temporally, measured particle concentrations with size less than 45 nm in the diameter were relatively higher in the winter and spring, but simulated concentrations did not have an apparent seasonal trend (). Measured concentrations had a marked weekly variation, which is not strong in the simulated concentrations. Diurnal peaks coinciding with the morning and afternoon rush hours for particles from 1 μm to 2 μm were observed in measured concentrations but not in those simulated. Thus, measured number concentrations had more apparent seasonal/daily/diurnal variations than simulated concentrations. The relatively smooth temporal variation of simulated number concentrations was consistent with that of simulated mass concentrations, which is caused in part by the smooth emission profiles used in CMAQ, the spatial averaging effect, and the spatial allocation of emissions (CitationMarmur et al. 2005; CitationPark et al. 2005) as well as the inability of the meteorological model to simulate the very fine features (CitationHogrefe et al. 2004). In summary, analysis of simulated number concentrations showed that simulated number concentrations were significantly underestimated and had less temporal variation.
4. ANALYSIS
Underestimation of particle number and surface area is due in part to the assumptions used. Here, three assumptions: particle density, size distribution of primary emissions, and the treatment of aerosols as three lognormal modes, are examined.
4.1. Particle Density
CMAQ converts particle mass concentrations into particle number concentrations using assumed particle density information () (CitationBinkowski et al. 2003). Assumed particle densities of organic carbon and elemental carbon in CMAQ appear larger than measured values. Hence, simulated number concentrations in CMAQ would be somewhat underestimated if simulated mass concentrations were reasonably well matched with the measured values, and the size distributions based on particle mass are correct as well.
TABLE 2 Assumed and measured particle densities (g cm− 3)
Influence of the inaccurate density on estimating particle number concentrations was examined by comparing the difference between measurements and simulations. Average ratios of measured to simulated number concentrations were 1.15 (mass), 1.43 (volume), 3.95 (surface area), and 29.34 (number) (). Note that PM2.5 mass measured by TEOM contains small amounts of water, although studies suggest that the amount of water would be negligible as a Nafian drier is used (CitationEatough et al. 2003; CitationGong and Demerjian 1995; CitationMeyer et al. 2000; CitationSchwab et al. 2004). Considering the possibility of water in the particles, the true ratio of observed mass to modeled mass would be slightly less than 1.15. The ratio increased from mass to volume concentrations, which is due to the higher particle densities (especially, OC and EC) used in CMAQ (see ).
TABLE 3 Summary statistics of hourly average particle number, surface area, volume, and mass concentrations for particles less than 2.5 μ m from 1/1/1999 to 8/31/2000 at the JST station in Atlanta, Georgia
Particle densities in CMAQ are changed to the measured particle densities, and particle mass and number concentrations were calculated with CMAQ (). When densities of OC and EC in CMAQ are assumed as 1.2 g cm− 3 and 2.0 g cm− 3, respectively (CitationMcMurry et al. 2002), the number concentrations increased 10.7%. When densities of OC and EC are assumed as 1.78 and 1.77, respectively (CitationPark et al. 2004a; CitationPark et al. 2004b; CitationTurpin and Lim 2001), the number concentrations increased 10.9%. However, mass concentrations changed less than 1% in both cases. Thus, CMAQ underestimated particle number concentrations about 10% due to the higher particle density, but the particle density alone cannot fully explain the large difference between measured and simulated number concentrations.
4.2. Size Distribution of Primary PM Emissions
Measured and simulated particle concentrations for different particle sizes also can be compared. CMAQ overestimated particle number concentrations for large particles (larger than 1.0 μ m), but significantly underestimated particle number concentrations for small particles (less than 1.0 μ m) ( and ). This result was expected because simulated number concentrations were significantly lower than the measured although simulated volume concentrations were predicted reasonably well (). To investigate why this might be, the influence of the size distribution of initial emissions on particle size distributions was analyzed.
The EPA emission inventory for particulate matter does not contain information about source size distributions. In CMAQ, the default assumption is that the major fraction of PM2.5 emissions are in the accumulation mode with a small fraction in the Aitken mode; i.e., 99.9% of the fine primary organic aerosol (POA) and primary elemental carbon (PEC) emissions are assumed to be in the accumulation mode, and the remaining fraction, 0.1%, are assigned to the Aitken mode (CitationBinkowski et al. 2003), and 100% of primary sulfate (PSO4), primary nitrate (PNO3), and unspecified fine PM (PMFINE) emissions are assigned to the accumulation mode. However, studies show that around 85% of primary organic carbon (POA) and elemental carbon (PEC) emissions should be assigned to the accumulation mode, and the remaining 15% of POA and PEC to the Aitken mode (CitationVenkataraman et al. 1994). If so, the assumption that 99.9% of POA and PEC emissions would be in the accumulation mode needs modification. Currently, other air quality models, for example, the European Monitoring and Evaluation Program (EMEP) unified aerosol model version, assume that 85% of POA and PEC are in the accumulation mode, and 100% of PMFINE is in the accumulation mode (CitationTsyro 2002). The size distribution of particle emissions is a hot topic of the current issue (CitationMcMurry and Woo 2002).
Sensitivity analysis of how simulated particle number concentrations respond to the ratio of the primary PM emissions in the accumulation to Aitken mode for PM2.5 emissions was performed for a limited period (3/23/1999 to 3/30/1999), which includes both regular (3/23∼ 3/28) and nucleation (3/29∼ 3/30) days, the latter being characterized by exceptionally high concentrations of particles with size less than 45 nm due to nucleation () (CitationWoo et al. 2001a). The model was run with the ratio of 0.85/0.15 (accumulation/Aitken), 0.9/0.1, and 0.99/0.01, in addition to the default ratio of 0.999/0.001 for PEC and POA emissions. When the ratio changed from 0.999/0.001 to 0.85/0.15, simulated particle number concentrations increased by a factor of 6 (), but simulated particle mass concentrations remained virtually the same (less than 1% change). Thus, as the ratio changes from 0.999/0.001 to 0.85/0.15, the simulated number concentrations approached measured concentrations for typical days, but continued to be low on days experiencing nucleation events (CitationWoo et al. 2001a). Nucleation can occur by rapid cooling of hot exhaust gases (CitationTobias et al. 2001), or oxidation of gas phase species followed by condensation (CitationWoo et al. 2001a). However, the exact physics and chemistry leading to such phenomena is still uncertain (CitationGaydos et al. 2005; CitationKulmala et al. 2004).
FIG. 5 Daily average measured particle number concentrations in JST from 3/23/1999 to 3/30/1999 at the JST station in Atlanta, Georgia.
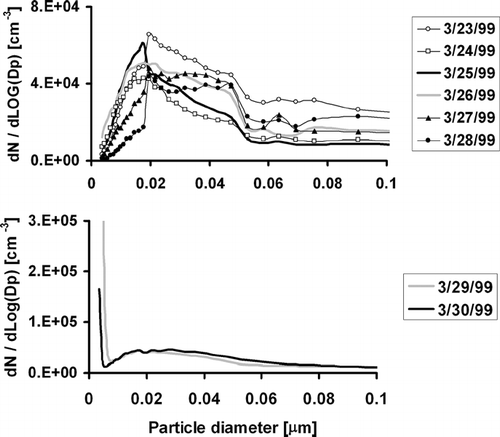
TABLE 4 Simulated fine particle number concentrations by CMAQ, as initial ratio of particle emissions in the Aitken (Ei) and accumulation (Ej) modes change from 3/23/1999 to 3/30/1999 at the JST station in Atlanta, Georgia
4.3. Treatment of Aerosol as Three Lognormal Modes
CMAQ simulates particles assuming that the particle size distribution follows three lognormal distributions: Aitken, accumulation, and coarse modes (CitationBinkowski et al. 2003; CitationWhitby 1978). Distribution parameters in CMAQ are summarized in . To evaluate the distribution parameters used, measured data were fit to lognormal distributions () using an Expectation-Maximization (EM) algorithm (see Appendix). Coarse mode parameters could not be evaluated because size measurements were not made above 3 μ m.
FIG. 6 (a) Measured median particle number, (b) surface area, and (c) volume concentrations from 1/1/1999 to 8/31/1999 at the JST station in Atlanta, Georgia, and the fitted distributions using parameters from the EM algorithm along with the number (N), surface area (S), and volume (V) concentrations, and the geometric mean (Dg), and geometric standard deviation (σ g) for the Aitken (i), and accumulation (j) modes.
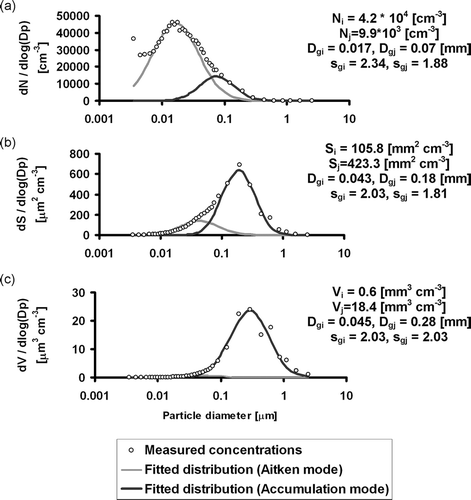
TABLE 5 Parameters of the lognormal distribution for the particle number, surface area, and volume concentrations used in CMAQ, and those based on the measurements at the JST station in Atlanta, Georgia
The distribution using the lognormal parameters from the EM algorithm fit well the measured number concentrations for particles larger than 0.01 μ m (). Parameters estimated using the measured data were similar to those assumed in CMAQ (), and also to those of typical urban aerosols (CitationWhitby 1978). However, the measured number concentrations did not fit well for particles with size less than 0.01 μ m (). This result shows that an additional mode, the geometric mean diameter of which is smaller than that of the Aitken mode, i.e., a nucleation mode, should be considered in order to better predict particles with sizes less than 0.01 mm. The appropriate shape of the new mode should be selected, and the proper treatment of the nucleation process is needed. In summary, the treatment of aerosols as three lognormal modes should simulate particles larger than 0.01 μ m reasonably well, but does not accurately simulate particles smaller than 0.01 μ m. Currently, the EMEP aerosol model version includes a nucleation mode, in addition to the Aitken, accumulation, and coarse modes (CitationTsyro 2002). EMEP aerosol model version uses MM32 as aerosol dynamic module (CitationPirjola et al. 2003; CitationTsyro et al. 2002). MM32 is another version of the multicomponent monodisperse model (MULTIMONO), which uses monodisperse approach to represent particle size distribution (CitationPirjola and Kulmala 2000). Performance of predicting particle number concentrations of the four-distribution model was significantly better than that of the mono-distribution model.
Note that the improvement in the particle prediction cannot be truly achieved without modifying the binary homogeneous nucleation mechanism used in the CMAQ. Studies suggest that the absolute rate of the particle formation used in CMAQ is also uncertain, so using relative rates of new particle formation and condensation on existing particles is less uncertain than the absolute rates. Experimental study shows that the growth of nucleated particles to a detectable size involves a competition between the coagulation loss of nucleated clusters to preexisting particles and their growth to larger sizes. This result also suggests that using the rate of formation on existing particles is reasonable (CitationMcMurry et al. 2005). Recently, a new ternary homogeneous nucleation parameterization was implemented and tested using CMAQ. The results showed that the ternary nucleation parameterization gave better performance in predicting particle number and size distributions compared with the binary nucleation parameterization (CitationZhang, Liu et al. 2005).
5. CONCLUSIONS
Fine particle mass concentrations simulated using CMAQ have been extensively evaluated, but CMAQ's ability to reproduce aerosol number concentrations is not as thoroughly demonstrated. Particle number concentrations simulated by CMAQ from 1/1/1999 to 8/31/2000 were evaluated. Simulated number concentrations were significantly underestimated up to a factor of 1000. While the nucleation mechanism, which remains an active area of research, is one uncertainty likely leading to the underestimation of particle number concentrations, other model specification affects predicted particle levels. Influences of assumptions used in CMAQ on the predicted particle number concentrations were investigated, including impacts of particle density, size distribution of the initial emissions, and the treatment of aerosols as three lognormal modes.
One cause of underestimating particle number concentrations was that the assumed particle density in CMAQ is higher than the observed values, though this had a small impact as did using larger grid sizes. Another cause of the underestimation was that the assumed size distribution of initial particle emissions in CMAQ was larger particles than observed. Currently, CMAQ assigns only 0.1% of fine particle emissions to the Aitken mode, and the remaining 99.9% of POA and PEC emissions to the accumulation mode. Measurements show that around 15% of POA and PEC emissions should be treated as being in the Aitken mode, and the remaining 85% as the accumulation mode. Sensitivity test results showed that when the fraction of initial particle emissions in the Aitken mode increased, particle number concentrations increased and approached measured values. Another cause of the underestimation was the treatment of aerosols using three lognormal distributions. The size distribution of the measured concentrations showed that three lognormal distributions could not accurately capture particles with size less than 0.01 μ m. An additional mode that accounts for the nucleated particles can improve the accuracy of the number concentration simulations.
APPENDIX
An Expectation-Maximization (EM) algorithm was employed to estimate the parameters of a mixture of lognormal distributions. The EM algorithm is based on the concept of incomplete data for optimizing likelihood functions (CitationDemster et al. 1977; CitationRedner and Walker 1984). When estimating the parameters of mixture distributions, we posit that the observed data X are incomplete and consider the existence of unobserved data, Y. This significantly facilitates optimizing the likelihood function since the optimizing process is analytically intractable in many cases without assuming Y. More precisely, let's assume the following mixture lognormal distributions.
Parameters of mixture lognormal distributions include the number of components in the mixtures, their coefficients, means, and variances denoted K, α i μ i , and σ i 2, respectively. K is usually obtained by prior knowledge. Here, we set K = 2 as the number of particles that are typically divided into two modes for size less than 2.5 μ m (CitationWhitby 1978). The EM algorithm is performed by repeating between the so-called the E-step and the M-step. In the E-step, we compute the expectation of complete log-likelihood function conditional on the initial guess of parameters and the observed data called the Q function.
In the M-step, we update the estimate of parameters to maximize the function computed in E-step.
Acknowledgments
This research was supported by the U.S. Environmental Protection Agency under Agreements RD82897602, RD83107601, and RD83096001.
Notes
*Parameters for the surface area and mass (volume) concentrations are calculated as follows.
a **Refer to
REFERENCES
- Adrian , R. J. and Orloff , K. L. 1977 . Laser Anemometer Signals—Visibility Characteristics and Application to Particle Sizing . Applied Optics , 16 : 677 – 684 . [CSA]
- Bhave , P. and Binkowski , F. S. 2005 . Private communication
- Binkowski , F. S. and Rosselle , S. J. 2003 . Models-3 Community Multiscape Air Quality (CMAQ) Model Aerosol Component 1. Model Description . J. Geophys. Res.—Atmos. , 108 ( D6 ) : 4183 doi:10.1029/2001JD001409[CROSSREF] [CSA]
- Boylan , J. , Odman , M. , Wilkinson , J. and Russell , A. 2006 . Integrated Assessment Modeling of Atmospheric Pollutants in the Southern Appalachian Mountains: Part 2. Fine Particulate Matter and Cisibility . J. Air & Waste Manag. Assoc. , 56 : 12 – 22 . [INFOTRIEVE] [CSA]
- Brauer , M. , Ebelt , S. T. , Fisher , T. V. , Brumm , J. , Petkau , A. J. and Vedal , S. 2001 . Exposure of Chronic Obstructive Pulmonary Disease Patients to Particles: Respiratory and Cardiovascular Health Effects . J. Exposure Anal. Environ. Epidemiol. , 11 : 490 – 500 . [CROSSREF] [CSA]
- Byun , D. W. and Ching , J. K. Science Algorithms of the EPA Models-3 Community Multiscale Air Qualtiy (CMAQ) Modeling System . Atmospheric modeling division, National Exposure Research Laboratory, U.S. Environmental Protection Agency . Research Triangle Park, NC. 27711
- Clarke , A. , Kapustin , V. , Eisele , F. , Weber , R. and McMurry , P. 1999 . Particle Production Near Marine Clouds: Sulfuric Acid and Predictions from Classical Binary Nucleation . Geophys. Res. Lett. , 2 : 2425 – 2428 . doi:10.1029/1999GL900438[CROSSREF] [CSA]
- Coffman , D. J. and Hegg , D. A. 1995 . A Preliminary-Study of the Effect of Ammonia on Particle Nucleation in the Marine Boundary-Layer . J. Geophy Res. Atmos. , 100 : 7147 – 7160 . doi:10.1029/2000JD000037[CROSSREF] [CSA]
- Demster , A. P. , Laird , N. M. and Rubin , D. B. 1977 . Maximum Likelihood from Incomplete Data Via the EM Algorithm (with Discussion) . J. Royal Statis. Soc. (B) , 39 : 1 – 38 . [CSA]
- Dockery , D. W. and Pope , C. A. 1994 . Acute Respiratory Effects of Particulate Air-Pollution . Ann. Rev. Public Health , 15 : 107 – 132 . [CROSSREF] [CSA]
- Dockery , D. W. , Pope , C. A. , Xu , X. P. , Spengler , J. D. , Ware , J. H. , Fay , M. E. , Ferris , B. G. and Speizer , F. E. 1993 . An Association Between Air-Pollution and Mortality in 6 United-States Cities . New England J. Medicine , 329 : 1753 – 1759 . [CROSSREF] [CSA]
- Donaldson , K. , Li , X. Y. and MacNee , W. 1998 . Ultrafine (nanometre) Particle Mediated Lung Injury . J. Aerosol Sci. , 29 : 553 – 560 . [CROSSREF] [CSA]
- Donaldson , K. , Stone , V. , Seaton , A. and MacNee , W. 2001 . Ambient Particle Inhalation and the Cardiovascular System: Potential Mechanisms . Environ. Health Perspe. , 109 : 523 – 527 . [CSA]
- Eatough , D. J. , Long , R. W. , Modey , W. K. and Eatough , N. L. 2003 . Semi-Volatile Secondary Organic Aerosol in Urban Atmospheres: Meeting a Measurement Challenge . Atmos. Environ. , 37 : 1277 – 1292 . [CROSSREF] [CSA]
- Ebelt , S. T. , Petkau , A. J. , Vedal , S. , Fisher , T. V. and Brauer , M. 2000 . Exposure of Chronic Obstructive Pulmonary Disease Patients to Particulate Matter: Relationships Between Personal and Ambient Air Concentrations . J. Air & Waste Manag. Assoc. , 50 : 1081 – 1094 . [INFOTRIEVE] [CSA]
- Eder , B. , Yu , S. and Dennis , R. An Evaluation of the Models-3 CMAQ Aerosol Module . PM 2003 Meeting—Particulate Matter: Atmospheric Sciences, Exposure and the Forth Colloquium on PM and Human Health . Pittsburgh, PA.
- Elleman , R. A. , Kotchenruther , R. A. , Covert , D. S. , Mass , C. F. and Chen , J. CMAQ Aerosol Number and Mass Evaluation for Pacific Northwest . Models-3 User's Workshop . Research Triangle Park, NC.
- Elleman , R. A. , Kotchenruther , R. A. , Covert , D. S. , Mass , C. F. and Chen , J. CMAQ Aerosol Number and Mass Evaluation for Pacific Northwest . 7th Conference on Atmospheric Chemistry, the 85th AMS Annual Meeting . San Diego, CA.
- Emery , C. , Tai , E. and Yarwood , G. Enhanced Meteorological Modeling and Performance Evaluation for Two Texas Episodes . Report to the Texas Natural Resources Conservation Commission, prepared by ENVIRON . Novato, CA : International Corp .
- Fan , J. W. , Zhang , R. Y. , Li , G. H. , Nielsen-Gammon , J. and Li , Z. Q. 2005 . Simulations of Fine Particulate Matter (PM2.5) in Houston, Texas . J. Geophys. Res. Atmos. , 110 : D16203 doi:10.1029/2005JD005805[CROSSREF] [CSA]
- Faxvog , F. R. and Roessler , D. M. 1978 . Carbon Aerosol Visibility vs Particle-Size Distribution . Appl. Optics , 17 : 2612 – 2616 . [CSA]
- Gaydos , T. M. , Stanier , C. and Pands , S. 2005 . Modeling of in Situ Ultrafine Atmospheric Particle Formation in the Eastern United States . J. Geophys. Res. Atmos. , 110 : D07S12 doi:10.1029/2004JD004683[CROSSREF] [CSA]
- Gold , D. R. , Damokosh , A. I. , Pope , C. A. , Dockery , D. W. , McDonnell , W. F. , Serrano , P. , Retama , A. and Castillejos , M. 1999 . Particulate and Ozone Pollutant Effects on the Respiratory Function of Children in Southwest Mexico City . Epidemio. , 10 : 470 – 470 . [CSA]
- Gong , Q. and Demerjian , K. L. 1995 . Hydrocarbon Losses on a Regenerated Nafion(R) Dryer . J. Air & Waste Manag. Assoc. , 45 : 490 – 493 . [INFOTRIEVE] [CSA]
- Hansen , D. A. , Edgerton , E. S. , Hartsell , B. E. , Jansen , J. J. , Kandasamy , N. , Hidy , G. M. and Blanchard , C. L. 2003 . The Southeastern Aerosol Research and Characterization Study: Part 1-Overview . J. Air & Waste Manag. Assoc. , 53 : 1460 – 1471 . [INFOTRIEVE] [CSA]
- Hogg , R. V. , Craig , A. T. and McKean , J. W. 2005 . Introduction to Mathematical Statistics , New York : Prentice Hall .
- Hogrefe , C. , Biswas , J. , Lynn , B. , Civerolo , K. , Ku , J. Y. , Rosenthal , J. , Rosenzweig , C. , Goldberg , R. and Kinney , P. L. 2004 . Simulating Regional-Scale Ozone Climatology Over the Eastern United States: Model Evaluation Results . Atmos. Environ. , 38 : 2627 – 2638 . [CROSSREF] [CSA]
- Jiang , W. , Giroux , E. , Smyth , S. , Roth , H. and Yin , D. Differences Between CMAQ Fine Mode Particle and PM2.5 Concentrations and their Impact on Model Performance Evaluation in the Lower Fraser Valley . 2004 Models-3 Conference . October 18–20 2004 , Chapel Hill, NC.
- Jiang , W. , Giroux , E. , Roth , H. and Yin , D. Evaluation of CMAQ PM Results Using Size-Resolved Field Measurement Data: The Particle Diameter Issue and its Impact on Model Performance Assessment . 27th NATO/CCMS International Technical Meeting (ITM) on Air Pollution Modelling and its Application . October 25–29 2004 , Banff, Alberta, Canada.
- Jiang , W. and Yin , D. Development and Application of the PMx Software Package for Converting CMAQ Modal Particulate Matter Results in Size-Resolved Quantities . Prepared for Program of Energy Research and Development . Natural Resources Canada . Report number: PET-1497-01S, 2001
- Jun , M. and Stein , M. L. 2004 . Statistical Comparison of Observed and CMAQ Modeled Daily Sulfate Levels . Atmos. Environ. , 38 : 4427 – 4436 . [CROSSREF] [CSA]
- Katz , J. L. , Fisk , J. A. and Chakarov , V. M. 1994 . Condensation of a Supersaturated Vapor.9. Nucleation on Ions . J. Chem. Phys. , 101 : 2309 – 2318 . [CROSSREF] [CSA]
- Korhonen , P. , Kulmala , M. , Laaksonen , A. , Viisanen , Y. , McGraw , R. and Seinfeld , J. H. 1999 . Ternary Nucleation of H2SO4, NH3, and H2O in the Atmosphere . J. Geophys. Res. Atmos. , 104 : 26349 – 26353 . doi:10.1029/1999JD900784[CROSSREF] [CSA]
- Kulmala , M. , Laaksonen , A. and Pirjola , L. 1998 . Parameterizations for Sulfuric Acid/Water Nucleation Rates . J. Geophys. Res. Atmos. , 103 : 8301 – 8308 . doi:10.1029/97JD03718[CROSSREF] [CSA]
- Kulmala , M. , Lehtinen , K. and Laaksonen , A. 2006 . Cluster Activation Theory as an Explanation of the Linear Dependence Between Formation Rate of 3 nm Particles and Sulphuric Acid Concentration . Atmo. Chem. Phys. Discuss. , 6 : 787 – 793 . [CSA]
- Kulmala , M. , Vehkamaki , H. , Petajda , T. , Dal Maso , M. , Lauri , A. , Kerminen , V. M. , Birmili , W. and McMurry , P. H. 2004 . Formation and Growth Rates of Ultrafine Atmospheric Particles: A Review of Observations . J. Aerosol Sci. , 35 : 143 – 176 . [CROSSREF] [CSA]
- Laakso , L. , Makela , J. M. , Pirjola , L. and Kulmala , M. 2002 . Model Studies on Ion-Induced Nucleation in the Atmosphere . J. Geophys. Res. Atmos. , 107 : 4427 doi:10.1029/2002JD002140[CROSSREF] [CSA]
- Marmur , A. , Park , S.-K. , Mulholland , J. and Russell , A. G. PM2.5 Source Apportionment Using Receptor and Source-oriented Models: Conceptual Differences and Implications for Health Studies . Proc. AAAR International Specialty Conference—Particulate Matter Supersites Program and Related Studies . Atlanta, Georgia.
- Marmur , A. , Park , S.-K. , Mulholland , J. , Tolbert , P. and Russell , A. 2006 . Source Apportionment of PM2.5 in the Southeastern United States Using Receptor and Emissions-Based Models: Conceptual Differences and Implications for Time-Series Health Studies . Atmos. Environ. , 40 : 2533 – 2551 . [CROSSREF] [CSA]
- McMurry , P. H. , Wang , X. , Park , K. and Ehara , K. 2002 . The Relationship Between Mass and Mobility for Atmospheric Particles-A New Technique for Measuring Particle Density . Aerosol Sci. Technol. , 36 : 227 – 238 . [CROSSREF] [CSA]
- McMurry , P. H. 1983 . New Particle Formation in the Presence of an Aerosol—Rates, Time Scales, and Sub-0.01 Mu-M Size Distributions . J. Coll. Interface Sci. , 95 : 72 – 80 . [CROSSREF] [CSA]
- McMurry , P. H. and Friedlander , S. K. 1979 . New Particle Formation in the Presence of an Aerosol . Atmos. Environ. , 13 : 1635 – 1651 . [CROSSREF] [CSA]
- McMurry , P. H. and Woo , K. S. 2002 . Size Distributions of 3–100-nm Urban Atlanta Aerosols: Measurement and Observations . J. Aerosol Med. Depos. Cleara. Effects in the Lung , 15 : 169 – 178 . [CSA]
- McMurry , P. H. , Fink , M. , Sakurai , H. , Stolzenburg , M. R. , Mauldin , R. L. III , Smith , J. , Eisele , F. , Moore , K. , Sjostedt , S. , Tanner , D. , Huey , L. G. , Nowak , J. B. , Edgerton , E. and Voisin , D. 2005 . A Criterion for New Particle Formation in the Sulfur-Rich Atlanta Atmosphere . J. Geophy. Res. Atmos. , 110 : D22S02 doi:10.1029/2005JD005901[CROSSREF] [CSA]
- Mebust , M. R. , Eder , B. K. , Binkowski , F. S. and Roselle , S. J. 2003 . Models-3 Community Multiscale Air Quality (CMAQ) Model Aerosol Component-2. Model Evaluation . J. Geophys. Res. Atmos. , 108 : 4184 doi:10.1029/2001JD001410[CROSSREF] [CSA]
- Meyer , M. B. , Patashnick , H. , Ambs , J. L. and Rupprecht , E. 2000 . Development of a Sample Equilibration System for the TEOM Continuous PM Monitor . J. Air & Waste Manag. Assoc. , 50 : 1345 – 1349 . [INFOTRIEVE] [CSA]
- Napari , I. , Noppel , M. , Vehkamaki , H. and Kulmala , M. 2002 . Parametrization of Ternary Nucleation Rates for H2SO4-NH3-H2O Vapors . J. Geophys. Res. Atmos. , 107 : 4381 doi:10.1029/2002JD002132[CROSSREF] [CSA]
- O'Neill , S. , Lamb , B. , Chen , J. , Claiborn , C. , Finn , D. , Otterson , S. , Figueroa , C. , Bowman , C. , Boyer , M. , Wilson , R. , Arnold , J. , Aalbers , S. , Stocum , J. , Swab , C. , Stoll , M. , Dubois , M. and Anderson , M. 2006 . Modeling Ozone and Aerosol Formation and Transport in the Pacific Northwest with the Community Multi-Scale Air Quality (CMAQ) Modeling System . Environ. Sci. Technol. , 40 : 1286 – 1299 . [INFOTRIEVE] [CROSSREF] [CSA]
- Park , K. , Kittelson , D. B. and McMurry , P. H. 2004a . Structural Properties of Diesel Exhaust Particles Measured by Transmission Electron Microscopy (TEM): Relationships to Particle Mass and Mobility . Aerosol Sci. Technol. , 38 : 881 – 889 . [CROSSREF] [CSA]
- Park , K. , Kittelson , D. B. , Zachariah , M. R. and McMurry , P. H. 2004b . Measurement of Inherent Material Density of Nanoparticle Agglomerates . J. Nanoparticle Res. , 6 : 267 – 272 . [CROSSREF] [CSA]
- Park , S.-K. , Cobb , C. E. , Wade , K. , Mulholland , J. , Hu , Y. and Russell , A. 2006 . Uncertainty in Air Qualiy Model Evaluation for Particulate Matter due to Spatial Variation in Pollutant Concentrations . Atmospheric Environment , in press[CSA]
- Park , S.-K. , Ke , L. , Ke , B. , Ke , Y. , Ke , G. , Ke , R. A. and Zheng , M. Source apportionment of PM2.5 using a three-dimensional air quality model and a receptor model . Proceedings of an AAAR International Specialty Cconference—Particulate Matter Supersites Program and Related Studies . Atlanta, Georgia.
- Park , S.-K. and Russell , A. G. Sensitivity of PM 2.5 to emissions in the Southeast . Proceedings of the Models-3 User's Workshop . Research Triangle Park, NC.
- Patashnick , H. and Rupprecht , E. G. 1991 . Continuous Pm-10 Measurements Using the Tapered Element Oscillating Microbalance . J. Air & Waste Manag. Assoc. , 41 : 1079 – 1083 . [INFOTRIEVE] [CSA]
- Penttinen , P. , Timonen , K. L. , Tittanen , P. , Mirme , A. , Ruuskanen , J. and Pekkanen , J. 2001 . Number Concentration and Size of Particles in Urban Air: Effects on Spirometric Lung Function in Adult Asthmatic Subjects . Environ. Health Perspec. , 109 : 319 – 323 . [CSA]
- Pirjola , L. and Kulmala , M. 2000 . Aerosol Dynamical Model MULTIMONO . Boreal Environ. Res. , 5 : 361 – 374 . [CSA]
- Pirjola , L. , Tsyro , S. , Tarrason , L. and Kulmala , M. 2003 . A Monodisperse Aerosol Dynamics Module, a Promising Candidate for Use in Long-Range Transport Models: Box Model Tests . J. Geophys. Res.-Atmos. , 108 ( D9 ) : 4258 doi:10.1029/2002JD002867[CROSSREF] [CSA]
- Pope , C. A. , Thun , M. J. , Namboodiri , M. M. , Dockery , D. W. , Evans , J. S. , Speizer , F. E. and Heath , C. W. 1995 . Particulate Air-Pollution as a Predictor of Mortality in a Prospective-Study of US Adults . Amer. J. Respir. Crit. Care Med. , 151 : 669 – 674 . [CSA]
- Pope , C. A. , Verrier , R. L. , Lovett , E. G. , Larson , A. C. , Raizenne , M. E. , Kanner , R. E. , Schwartz , J. , Villegas , M. , Gold , D. R. and Dockery , D. W. 1999 . Heart Rate Variability Associated with Particulate Air Pollution . Amer. Heart J. , 138 : 890 – 899 . [INFOTRIEVE] [CROSSREF] [CSA]
- PSU/NCAR . 2003 . “ PSU/NCAR Mesoscale Modeling System Tutorial Class Notes and User's Guide: MM5 Modeling System Version 3 ” . In Mesoscale and Microscale Meteorology Division , National Center for Atmospheric Research .
- Redner , A. R. and Walker , H. F. 1984 . Mixture Densities, Maximum Likelihood and the EM Algorithm . SIAM Review , 26 : 195 – 239 . [CROSSREF] [CSA]
- Saldiva , P. H. N. , Pope , C. A. , Schwartz , J. , Dockery , D. W. , Lichtenfels , A. J. , Salge , J. M. , Barone , I. and Bohm , G. M. 1995 . Air-Pollution and Mortality in Elderly People—A Time-Series Study in Sao-Paulo, Brazil . Arch. Environ. Health , 50 : 159 – 163 . [INFOTRIEVE] [CSA]
- Schwab , J. J. , Hogrefe , O. , Demerjian , K. L. and Ambs , J. L. 2004 . Laboratory Characterizaton of Modified Tapered Element Oscillating Microbalance Samplers . J. Air & Waste Manag. Associ. , 54 : 1254 – 1263 . [INFOTRIEVE] [CSA]
- Stolzenburg , M. , McMurry , P. , Sakurai , H. , Smith , J. , Mauldin , R. , Eisele , F. and Clement , C. 2005 . Growth Rates of Freshly Nucleated Atmospheric Particles in Atlanta . J. Geophys. Res.-Atmos. , 110 : D22S05 doi:10.1029/ 2005JD005935[CROSSREF] [CSA]
- Tobias , H. J. , Beving , D. E. , Ziemann , P. J. , Sakurai , H. , Zuk , M. , McMurry , P. H. , Zarling , D. , Waytulonis , R. and Kittelson , D. B. 2001 . Chemical Analysis of Diesel Engine Nanoparticles Using a Nano-DMA/Thermal Desorption Particle Beam Mass Spectrometer . Environ. Sci. Technol. , 35 : 2233 – 2243 . [INFOTRIEVE] [CSA]
- Tonnesen , G. S. Regional Haze Modeling: Recent Modeling Results for VISTAS and WRAP . Models-3 User's workshop . Research Triangle Park, North Carolina.
- Tsyro , S. 2002 . “ First Estimates of the Effects of Aerosol Dynamics in the Calculation of PM10 and PM2.5 ” . EMEP/MSC-W, http://emep.int/publ/reports/2002/mscw_note_4_2002.pdf
- Tsyro , S. , Pirjola , L. , Kulmala , M. , Simpson , D. and Tarrasón , L. Initial Results from Long-Range Transport of Particulate Matter in Europe . Air Pollution modeling and Simulations. Proceedings. Second conference on Air pollution Modelling and Simulation, APMS'01 . April 9–12 2001 , Champs-sur-Marne. Edited by: Sportisse , B.
- Turpin , B. J. and Lim , H. J. 2001 . Species Contributions to PM2.5 Mass Concentrations: Revisiting Common Assumptions for Estimating Organic Mass . Aerosol Sci. Technol. , 35 : 602 – 610 . [CSA]
- UCAR . 2003 . TDL U.S. and Canada Surface Hourly Observations http://dss.ucar.edu/datasets/ds472.0/
- US-EPA . 2004 . SMOKE User's manual http://cf.unc.edu/cep/empd/products/smoke
- Van Loy , M. , Bahadori , T. and Wyzga , R. 2000 . The Aerosol Research and Inhalation Epidemiology Study (ARIES): PM2.5 Mass and Aerosol Component Concentrations and Sampler Intercomparisons . J. Air and Waster Manag. Associ. , 50 : 1446 – 1458 . [CSA]
- Vedal , S. 1997 . Ambient Particles and Health: Lines That Divide . J. Air & Waste Manag. Associ. , 47 : 551 – 581 . [INFOTRIEVE] [CSA]
- Venkataraman , C. , Lyons , J. M. and Friedlander , S. K. 1994 . Sire Distributions of Polycyclic Aromatic-Hydrocarbons and Elemental Carbon.1. Sampling, Measurement Methods, and Source Characterization . Environ. Sci. Technol. , 28 : 555 – 562 . [CROSSREF] [CSA]
- Weber , R. , Marti , J. , McMurry , P. , Eisele , F. , Tanner , D. and Jefferson , A. 1996 . Measured Atmospheric New Particle Formation Rates: Implications for Nucleation Mechanisms . Chem. Engineer. Comm. , 151 : 53 – 64 . [CSA]
- Weber , R. , McMurry , P. , Mauldin , R. , Tanner , D. E. , Tanner , F L , Clarke , A. and Kapustin , V. 1999 . New Particle Formation in the Remote Troposphere: A Comparison of Observations at Various Sites . Geophys. Res. Lett. , 26 : 307 – 310 . doi:10.1029/1998GL900308, [CROSSREF] [CSA]
- Whitby , K. T. 1978 . The Physical Characteristics of Sulfur Aerosol . Atmos. Environ. , 12 : 135 – 159 . [CROSSREF] [CSA]
- Wilemski , G. 1984 . Composition of the Critical Nucleus in Multicomponent Vapor Nucleation . J. Chemi. Phys. , 80 : 1370 – 1372 . [CROSSREF] [CSA]
- Woo , K. S. , Chen , D. R. , Pui , D. Y. H. and McMurry , P. H. 2001a . Measurement of Atlanta Aerosol Size Distributions: Observations of Ultrafine Particle Events . Aerosol Sci. Technol. , 34 : 75 – 87 . [CSA]
- Woo , K. S. , Chen , D. R. , Pui , D. Y. H. and Wilson , W. E. 2001b . Use of Continuous Measurements of Integral Aerosol Parameters to Estimate Particle Surface Area . Aerosol Sci. Technol. , 34 : 57 – 65 . [CROSSREF] [CSA]
- Yu , F. and Turco , R. 2000 . Ultrafine Aerosol Formation Via Ion-Mediated Nucleation . Geophys. Res. Lett. , 27 : 883 – 886 . doi:10.1029/1999GL011151[CROSSREF] [CSA]
- Zhang , Q. , Canagaratna , M. R. , Jayne , J. T. , Worsnop , D. R. and Jimenez , J. L. 2005 . Time- and Size-Resolved Chemical Composition of Submicron Particles in Pittsburgh: Implications for Aerosol Sources and Processes . J. Geophys. Res.-Atmos. , 110 : D07S09 doi:10.1029/2004JD004649[CROSSREF] [CSA]
- Zhang , Y. , Liu , P. , Wang , K. and Jacobson , M. Predicting Aerosol Number and Size Distribution with CMAQ: Homogeneous Nucleation Algorithms and Process Analysis . Models-3 User's workshop . Research Triangle Park, NC.