Abstract
Simulations of the sky dome color shifts of a cloudless sky have been performed, assuming three urban-industrial tropospheric aerosol cases. Each of these aerosols is represented by the bimodal lognormal volume distribution (CitationDubovik et al. 2002). A total of ten parameters are used to control the aerosol characteristics. These ten parameters are perturbed and the resulting sky color shifts are estimated. All simulations have been performed relative to the reference cases that represent the time-averaged state of each aerosol case. We have simulated the sensitivity of the theoretical CIE (Commission Internationale de l′ Eclairage) standard human observer to discriminate sky color changes, due to changes of the aerosol control parameters. A Digital color Camera Model (DCM) has also been incorporated in the simulation scheme. The DCM simulates the performance of a camera-colorimeter created specifically to measure sky color. The DCM has been used to investigate whether a color digital camera can replicate the behavior of the CIE standard observer, with respect to sky color shifts. The standard observer is most sensitive to perturbations of the parameters of the aerosol fine mode, which are particles with diameters less than 0.6 μ m. However his sensitivity is highly variable, depending on the parameter varied, on the aerosol case and on the direction of the observation. The DCM was in excellent agreement with the behavior of the CIE standard observer. The camera simulated sky color measurement accuracy was high. The results show that the camera sensitivity in discriminating color differences is much better than that of the observer.
1. INTRODUCTION
Atmospheric aerosols affect visibility (Seinfeld and Pandis 1998) by scattering and absorbing radiation within the atmosphere. The net result of these processes can be to degrade visibility—the impairment of atmospheric clarity or the ability to perceive form, texture, and color (CitationTrijonis et al. 1990). The radiance of the sun-illuminated atmosphere, traversing through layers of air, forms the stimulus responsible for the sensation of the observed color. The interaction of radiation with aerosol particles alters the observed color of the sky dome (CitationAdams et al. 1974; CitationDave 1980; CitationDave 1981; CitationTsay et al. 1991; CitationDogras et al. 2004). The concept of visibility is mostly reflected by visual range (CitationTrijonis et al. 1990). However, visual range represents the overall visual experience of an observer when viewing a scene. Scenic beauty and visual air quality, apart from being sensitive to visual range, are also dependent on sky color (CitationLatimer et al. 1981; CitationWatson 2002). Visibility when related to visual range is a well-studied subject (EPA 1979; CitationTrijonis et al. 1990; CitationPitchford and Malm 1994; CitationHenry 2002), while the effect of atmospheric pollution and specifically, that of tropospheric aerosols, on the sky or skylight color has not been fully addressed yet.
Dave, almost four decades ago, published a series of articles that are considered fundamental to the transfer of visible radiation in the atmosphere, in which he describes how he simulated the chromaticities of the clear skies (CitationDave and Mateer 1968; CitationDave 1978, Citation1980, Citation1981). In his papers, Dave stresses the importance of atmospheric aerosols in determining sky color and the variability of that color for various viewing directions relative to the sun.
The importance of stratospheric ozone, especially on the color of twilight, was also reported by early studies (CitationDave and Mateer 1968; CitationAdams et al. 1974). Realistic rendering of the earth's atmosphere and the colors of the sky dome requires the inclusion of ozone and aerosols in the simulations (CitationJackèl and Walter 1997; CitationSloup 2002; CitationHaber et al. 2005). The aerosol models used in the above articles are too simplified to meet the needs of current environmental research. CitationDogras et al. (2004) have recently presented a study on the discoloration of the sky over cities that emphasize the role of urban aerosols. Because they have investigated the problem of daylight radiation that passes through a haze layer and not that of skylight, the geometry of the problem is greatly simplified and their method is unsuitable for specifically simulating skylight color.
In 1980, MacAdam published an article that introduced colorimetric theories in relation to visual air quality studies (MacAdam 1980). Although characterization of visibility of landscapes has progressed since then, and newer concepts like the deciview unit (CitationPitchford and Malm 1994; CitationWatson 2002; CitationHenry 2002) are now available, many of his suggestions are valuable when applied to sky color. Since we want to investigate the sensitivity of the human perception to sky color changes, due to variations of the aerosol properties, the concept of colorimetrically defined Just Noticeable Differences (JNDs) is very useful.
Color is the human sensation stimulated by the visual electromagnetic radiation. Therefore there is no color without an observer (CitationSharma 2003). Since it is important only for human vision it can only be defined by reference to visual functions (MacAdam 1980). Unfortunately perception of color is variable and subjective (Wyszecki and Stiles 1971; CitationPratt 1978) and only rough unreliable descriptions of color memories can be obtained by observers a short time after observing a particular color (CitationBodrogi and Tarczali 2001). Alteration of the characteristics of the scattering and absorbing atmospheric particulate matter is perceived by humans as shifts of sky color, relative to their memory of the previously observed atmospheric state. A system that consistently over time records and measures sky color is valuable and can overcome the human observer uncertainty.
In this study, we present a method for evaluating the effects of aerosols on the color of the sky and, at the same time, the sensitivity of the observer to these changes. Instead of simulating various radiation fields of the sky dome, followed by application of colorimetric formulas to estimate color coordinates, we take the novice approach to include the measuring procedure in our simulation. This is accomplished by incorporating a camera model in our simulation schematic. We believe that it is important to know not only the sensitivity of sky color to aerosol loading but also the limitations of the instrumentation used to record color, as well. This approach allows us to study the reaction of the theoretical “standard human observer”Footnote 1 (CitationBroadbent 2008; CitationPratt 1978) to the skylight stimulus and at the same time the response of the camera device to that same stimulus. This is of particular interest, since the camera is a much more feasible and realistic approach than the theoretical observer in quantifying sky color shifts.
The presented method and the results focus on the effect of urban-industrial aerosols on the color of the sky. These aerosols have an impact on the large populations living in cities all around the world. We are interested in the sky color shifts imposed by variations of the size distributions and optical properties of urban-industrial aerosols. However, other types of aerosols (e.g., continental or oceanic) are the subject of on going research as the method we use can easily utilize other types of aerosols. Humans living in the cities comprehend visual air quality deterioration due to atmospheric pollution, from the color of the sky and less through visibility deterioration, as most of the times nearby buildings put out of sight distant objects. Since different urban environments result in different aerosol characteristics, we have used three aerosol cases to describe particulate matter in the atmosphere. These are extracted from real case studies at various regions of the world (CitationDubovik et al. 2002). A detailed description is given in Section 2.1.
When rendering the sky color relative to atmospheric aerosols, various approaches are available. In computer graphics, color images of rendered outdoor scenes at a variety of aerosol types were presented (CitationJackèl and Walter 1997; CitationHaber et al. 2005). In some cases color characteristics of the sky are calculated for various mixtures of pure and composite particles (CitationTsay et al. 1991; CitationDogras et al. 2004). It is also common to simulate sky color for a pure Rayleigh scattering atmosphere, relative to various aerosol containing atmospheres (CitationAdams et al. 1974; CitationDave 1980, Citation1981). Our method is different. We use the concept of the “reference case” to describe the time averaged or common characteristics of the aerosol for the particular aerosol case. We then perturb each of the aerosol control parameters relative to the reference case and estimate the shift of the sky color. This is a much more realistic approach since cities are constant sources of particulate matter and pristine visual quality conditions are not representative of the actual conditions.
The use of camera systems in environmental research is common. Cameras have been used in the past in experimental studies on scenic beauty appreciation, when related to visual air quality (CitationLatimer et al. 1981). A very common application is the monitoring and simulation of visibility reduction (CitationMalm et al. 1983; CitationLarson et al. 1988; CitationTrijonis et al. 1990; CitationMolenar et al. 1994; CitationEldering et al. 1993, Citation1996). Recorded color photographs of outdoor scenes, form the basis for producing synthetic ones that simulate the effects of atmospheric haze. In all cases, portions of the sky are included in both the real and the simulated images. However these studies focus on the reduction of visibility of distant objects as seen through atmospheric haze and not on the faithful reproduction or estimation of the color of the sky. Photographic methods are also used for estimating the transmittance of the atmosphere (CitationRichards et al. 1989). Digital cameras combined with the appropriate image processing algorithms have been utilized as instruments for measuring visual range (CitationLuo 2005; CitationBäumer et al. 2007). A photographic technique has also been used for plume detection against the sky background (CitationDu et al. 2007).
When an accurate color measurement of the sky is sought, spectroradiometers (CitationHernández-Andrès et al. 1999; CitationHernández-Andrès and Romero 2001) are used. On the other hand cameras can be used to systematically record visual pollution incidents for qualitative assessments. Another highly desirable feature of any camera is the high angular and temporal resolution (CitationHernández-Andrès et al. 2003), which results in a vast number of sky color measurements delivered at a fraction of time and cost, when compared against spectroradiometers. The photographs of the sky dome recorded by a camera are of great archival value. However, in order for a camera to accurately measure sky color, it has to be calibrated specifically for the sky targets. The accuracy of its measurements has to be known beforehand as well. This type of camera is more a scientific instrument and less a photographic equipment. In the film era the construction of such a system was too complicated and the measurements were time consuming. Nowadays, digital cameras simplify such a task. Combinations of camera and spectroradiometers are used to record sky color (CitationLee 1994; CitationLee and Hernández-Andrès 2003) and to investigate color and luminance asymmetries of the sky dome (CitationHernández-Andrès et al. 2003). A study for designing a camera system for spectral imaging of the skylight has been presented recently (CitationLòpez-Álvarez et al. 2005).
In our previous work (CitationHaralabidis and Pilinis 2005) we have studied the desired features of a camera specifically designed to accurately record the color of the sky dome. We have shown that a color camera, equipped with a consumer digital image sensor, and combined with the appropriate imaging pipeline, can be used as a tristimulus colorimeter that is specifically intended to measure sky color. Part of our study was to build a Digital Camera Model (DCM) to simulate the performance of the real camera-colorimeter, by including color retrieval accuracy and measuring limitations. The camera output is the color coordinates of skylight from a specific point of the sky dome and the accuracy of that measurement. The DCM was included in our model to simulate the measuring procedure.
It is evident that a flexible model which simulates the sky color for a variety of aerosols, atmospheric profiles and sun observation path geometries is a multivariable problem. When combined with instrumentation modeling and investigation of the reaction of human sensation to perceived color changes, it becomes a fundamentally challenging task. In the following sections we give a detailed description of the simulation scheme and present the results from the application of the model.
2. THE SIMULATION PROCEDURE
The flow diagram of the system we have created in order to simulate sky colors and the measuring procedure is depicted in . A feature of our simulation scheme is the modular architecture. The main components are the Aerosol Optical Properties Module (AOPM), the Radiative Transfer Module (RTM) and the DCM (CitationAdams et al. 1974; CitationDave 1980; Citation1981; CitationTsay et al. 1991; CitationSloup 2002; CitationLee and Hernández-Andrès 2003; CitationHaber et al. 2005).
In order to simulate sky color it is necessary to simulate the radiation field of the sky. To do so we first have to estimate the optical properties of the aerosol populations. The module that calculates the optical properties of the aerosols is AOPM and includes a Mie scattering code. Results from AOPM are forwarded to the RTM module () in order to parameterize the atmosphere through which radiation traverses. RTM consists of two parts. The radiative transfer code itself and an interface for the code. All radiative transfer calculations were performed using the MODTRAN code (CitationBerk et al. 2003), combined with an appropriate control interface engineered using the Mathematica software. RTM output are the sky spectral radiances. Calculated radiances are redirected to the DCM module in order to simulate color recording by the camera. The DCM also estimates the theoretical values of that color, as well as the color recording accuracy of the camera, for each measurement. Appart from the MODTRAN code all modules were created using the Mathematica software.
2.1. The Aerosol Cases
In our simulations, we have included three urban-industrial aerosols, Mexico City, Creteil, Paris, and Maldives. The aerosol properties are derived from ground based indirect remote sensing measurements of the Aerosol Robotic Network (AERONET) (CitationHolben et al. 1998). These three urban aerosols correspond to three completely different areas of the world and they are the result of different emissions, chemical reactions, and geophysical-meteorological characteristics (CitationDubovik et al. 2002). A detail description of these aerosol cases is given by CitationDubovik et al. (2002). Mexico City exhibits the higher pollution levels among the three reference cases. Creteil, Paris aerosol exhibits the lowest real part of the refractive index (), usually associated with higher relative humidity and resultant hygroscopic growth. The pollution of Creteil, Paris is closer to water-soluble aerosols than the Maldives and Mexico City aerosol cases. Emission controls of car and industrial pollution is more advanced in France than in Mexico and India (CitationDubovik et al. 2002). Aerosol from Maldives has contributions from sea salt and from long-distance transport of airborne soil dust (CitationEck et al. 2001). We want to investigate how an observer will respond to sky color shifts resulting from variations of the aerosol characteristics in these different locations. We utilized aerosol characteristics created from the AERONET data, since their measuring procedure is similar to the simulated sun-observer path geometry of our study (). Aerosol size distributions derived by AERONET are well suited to our simulation scheme. The inversion procedure of CitationDubovik and King (2000) retrieves the “optical” size distribution from the light scattered and absorbed by particles. In this study we use these “optical” sizes to calculate the scattering and absorption of light by particles.
FIG. 2 Simulation geometry and the sky dome. The star shape marks the position of the sun and v marks the observer or camera viewing path.
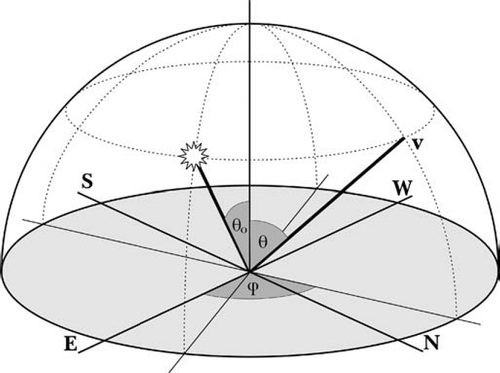
TABLE 1 Summary of the three urban–industrial aerosol models used in this study
The effect of aerosol particles on radiative transfer depends on their size distribution and chemical composition (Pilinis and Li 1998; CitationMolnár and Mészáros 2001). In practice, aerosols are a collection of discrete sized particles with a number concentration that depends on the aerosol size. Such a polydispersion can be described as the sum of log-normal distributions (Seinfeld and Pandis 1998). We have used the bimodal lognormal function of the volume distribution (CitationDubovik et al. 2002):
This study focuses on the volume concentration of the aerosol and as a result the performed perturbation of some of the parameters affects the total number and the number distribution of either the fine or the coarse mode of the aerosol. The effect of the above is analytically discussed in Section 3.
Since aerosol volume distributions dV(r)/dln r are reported (CitationDubovik et al. 2002) as the total column aerosol content (μ m3 μ m−2), we have to use optical thickness scaling factors to convert them into (μ m3 m−3). In our case the scaling factors were calculated by iterating MODTRAN with the reference case aerosol distribution shape, in order to converge to the observed aerosol optical thickness of CitationDubovik et al. (2002). A similar approach has been followed by CitationRemer et al. (1997). presents the volume size distribution for each of the reference cases after scaling.
2.2. Absorption and Scattering of Light by Atmospheric Aerosols
We have developed our own Mie scattering code using the Mathematica package (). We assumed spherical homogeneous particles characterized by a complex refractive index (CitationDubovik et al. 2002). The assumption of sphericity is consistent with the previously described aerosol cases (CitationDubovik and King 2000; CitationDubovik et al. 2002). Module inputs are the ten aerosol control parameters described in Section 2.1. Module outputs are the wavelength dependent aerosol extinction coefficients b ext (λ) (km−1), absorption coefficient b abs (λ) (km−1), aerosol asymmetry parameters g(λ), surface meteorological visibility range VIS (km), and the aerosol phase functions P(θ aer ,λ). Since we are interested in the visible portion of radiation, light wavelengths λ, are considered between λmin = 360 nm to λmax = 830 nm. The above quantities are required by MODTRAN.
2.3. Radiative Transfer Calculations
For simulating atmospheric radiance fields we use MODTRAN, which offers extensive parameterization to allow custom user control. The outputs of the radiative transfer code are the sky spectral radiances L(λ). These radiances are forwarded to the DCM module in order to estimate the skylight color.
presents the geometry of the simulation. Let θo being the sun zenith angle, θ being the observation line of sight and φ being the azimuth angle between the sun to observer path and the line of sight. To minimize possible uncertainties due to aerosol vertical variations (CitationDubovik and King 2000), we focus our analysis on simulating sky radiances at the solar almucantar (zenith angle of observations is equal to the solar zenith angle). All simulated measurements are performed for sun zenith angle of 72.5°, corresponding to morning and late afternoon hours.
Multiple scattering and refraction of light rays through the atmosphere are considered in our calculations. The effects of scattering by air molecules and by a reflecting surface are also included in the software parameterization. We used the mid-latitude standard atmosphere, a cloudless sky and surface albedo termed “urban” as defined in MODTRAN.
2.4. The Digital Camera Model
We have developed a DCM with the purpose of simulating the performance of a sky radiance colorimeter, based on a single-sensor camera. A detailed description of the DCM, as well as a presentation of its colorimetric performance is presented elsewhere (CitationHaralabidis and Pilinis 2005). The DCM simulates the performance of the Kodak KAF-5101CE CCD sensor (CitationEastman Kodak 2003), equipped with a three color filter mosaic (Red, Green, and Blue, RGB). Analog to digital conversion is performed at 12-bit of accuracy. To achieve accurate color recording, we utilized a least squares color characterization method, using sky specific radiance training sets. The method we developed especially for this purpose is termed as Sky Radiance Sky Point Preserving Least Squares (SkyRadSPPLS) and it is a variation of the constrained least square methods (CitationFinlayson and Drew 1997). The accuracy of the SkyRadSPPLS method was tested using 912 sky spectral radiance samples generated by MODTRAN. These samples represented a variety of atmospheric conditions and viewing directions. The SkyRadSPPLS method had an excellent performance with 73.5% of the samples recorded at an accuracy of less than 1/10 of the CIE-94 unit, while all of the samples were less than ½ of the CIE-94 unit (details of the CIE-94 unit are given in the next section). The DCM also simulates the uncertainties introduced during the measuring procedure due to various sources of noise. DCM input is radiance. The outputs of the DCM are the tristimulus XYZ color values based on the 2° 1931 CIE standard observer, coupled with the uncertainty of these measurements. A short description of the colorimetric terms and terminology used in this study is given in the next section.
2.5. Colorimetry
In this study we calculate the sky spectral radiances L(λ) in order to simulate sky color. In the trichromatic color system, color is described in terms of tristimulus values. Let be the 2° 1931 CIE Standard Observer color matching functions. The tabular form of these functions is provided by many authors at 5 nm intervals (e.g., CitationSharma 2003). Straightforward estimation of the CIE 1931 tristimulus values X, Y, Z is given by:
From the above equations we can estimate the theoretical values X th , Y th , and Z th of color from a particular point of the sky dome.
A desirable characteristic of any color coordinate system is its perceptual uniformity. That means that equal changes of colors of that system should be perceived as equivalently noticeable color shifts to an observer. Although the CIE XYZ color system is used to unambiguously represent color, it is perceptually nonuniform (CitationPratt 1978). Uniform color spaces are a field of active research. CIE has recommended a uniform color space called the CIE 1976 L*a*b* system, which is widely used in color imaging and easily derived from the CIE XYZ system (CitationSharma 2003). In the L*a*b* coordinates, L* is the lightness component of the color. The Euclidean distance between two color stimuli of coordinates L 1*, a 1*, b 1* and L 2*, a 2*, b 2*, respectively, represents the color difference and is given by:
It is reported that a ΔE Eu * value of around 2.3 corresponds to a JND (CitationMahy et al. 1994). Unfortunately uniformity of the L*a*b* color system has been proven to be limited (CitationSharma 2003). Alternative color difference formulae have therefore been proposed. In 1994 CIE proposed a formula, termed as CIE-94 color difference formula, based on the L*a*b* color system. A detailed description of this formula can be found elsewhere (e.g., CitationSharma 2003).
Color difference ΔE94* has units smaller than ΔE Eu *. Although in many practical cases a ΔE94* unit value is assumed to correspond to a JND, there is no definite statement in the scientific literature that this is the case. Therefore it is preferable to use the term one ΔE94* unit instead of a JND. CIE has defined the reference conditions under which the CIE-94 metric performs well (CIE 1995). One of the conditions states that color specimens whose perceptual color difference is evaluated must be viewed by the assessor when placed side by side. Since in our case sky color shifts due to variation of atmospheric optics occur at different times of the day and they are as a result cannot be observed simultaneously, it is reasonable to expect that the color difference of one ΔE94* unit is much lower than the actual color difference perception of the observer (CitationBodrogi and Tarczali 2001). In our study we used the one ΔE94* unit threshold as a worst case scenario. In actual situations this limit is expected to be much higher.
3. RESULTS AND DISCUSSION
3.1. Model Operation
The simulations were performed according to Section 2. All three aerosols of the simulations are controlled by 10 different parameters and each one is independently varied relative to the reference case. The sky color also changes relative to the sun-observer and observation path geometry. Since observations are restricted to the solar almucadar, sky color geometry is controlled only by the azimuth angle ϕ. We have performed our simulations for three different ϕ values, 30°, 90°, and 120°. Consequently thirty sets of simulations were performed for each aerosol scenario. Each set consists of a variable number of synthetic photographs. Every photograph was produced from a unique combination of aerosol parameters, azimuth angles, and aerosol scenarios.
We have produced each set of synthetic photographs, related to variation of a single control parameter, while keeping camera exposure steady. Exposure was controlled by adjusting exposure time. We wanted to avoid saturating the sensor red, green, or blue color channels. Hence camera exposure was defined to raise a maximum of 87.5% of the saturation signal for any of the channels. In order to take advantage of the camera dynamic range, we attributed the above maximum to the radiance that raised the higher signal for the camera channels. All other photographs of the same set were produced under lower lighting conditions. As a result, the lightness parameter L* of the recorded color, changes during the variation of an aerosol control parameter. Accordingly, observer color differences and camera performance results, which are presented in the following sections, also take into account changes of the lightness of the color. Because of these changes of lightness, the camera noise performance is not stable. Darker colors are related to lower signal levels at the sensor and therefore have poorer signal-to-noise ratios than lighter colors. Camera evaluation is performed for two different criteria. We investigate the ability of the camera to replicate the behavior of the standard observer, relevant to detection of sky color shifts (section 3.3) and we study camera sensitivity to positively detect sky color changes related to perturbation of the aerosol parameters (section 3.4).
3.2. Observer Sensitivity to Variations of the Aerosol Parameters
We have simulated the perception of the standard observer to sky color shifts due to changes of the aerosol control parameters. We have also evaluated the reliability of the camera to approach the behavior of the standard observer. and illustrate the results from implementing the CIE-94 formula to the theoretically calculated X th , Y th , Z th tristimulus values together with the simulated X rec , Y rec , Z rec measurements of the camera for Mexico City and Creteil, Paris aerosol. The behavior of the Maldives (INDOEX) aerosol is similar and therefore not presented. It is shown that observer sensitivity on color shifts greatly depends on the varied aerosol parameter. A more detailed description is presented in . We estimated the minimum–maximum percentage range of aerosol control parameter variation, relative to sky color shifts, which remain unperceivable by the observer. was calculated using the theoretical tristimulus values. All % changes of the aerosol control parameters presented result in color differences lower than the threshold of one ΔE94* unit. According to and in all cases, the observer is more sensitive to variations of the fine mode aerosol distribution control parameters than he is to the corresponding changes of the coarse mode. This is due to the fact that for the spectral range under consideration (360 nm–830 nm) light is scattered much more efficiently by fine mode (< 0.6 μ m) particles (Seinfeld and Pandis 1998; CitationDubovik et al. 2002).
FIG. 4 CIE-94 color difference for the Mexico City aerosol case. Estimated theoretical values (solid lines) and camera measurements (dashed lines). Plates (a), (c), and (e) present the fine mode parameters. Plates (b), (d), and (f) show the coarse mode parameters. The dashed black line intercepts color difference lines at a value of 1 ΔE94* unit. The n f curve corresponds to the top x axis and all other curves to the bottom x axis. (Figure provided in color online.)
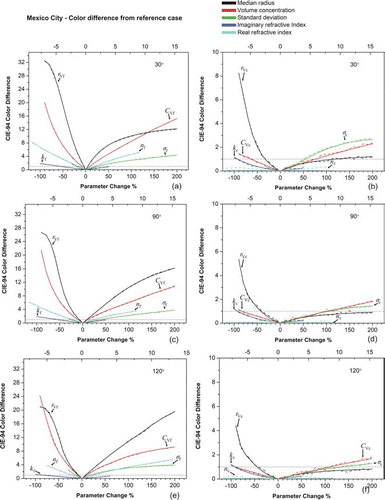
FIG. 5 CIE-94 color difference for the Creteil, Paris aerosol case. Estimated theoretical values (solid lines) and camera measurements (dashed lines). Plates (a), (c), and (e) present the fine mode parameters. Plates (b), (d), and (f) show the coarse mode parameters. The dashed black line intercepts color difference lines at a value of 1 ΔE94* unit. The n f curve corresponds to the top x axis and all other curves to the bottom x axis. (Figure provided in color online.)
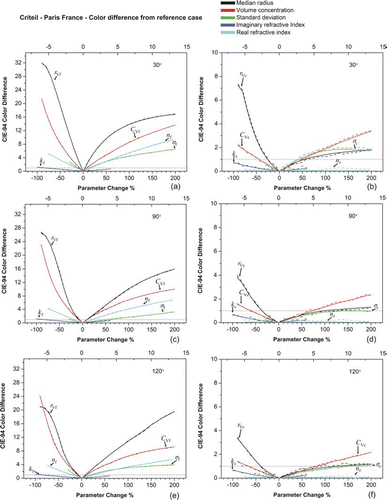
TABLE 2 Summary of the observer sensitivity to the variations of the aerosol parameters
3.2.1. Variation of the Median Radius r Vf and r Vc
From and it is evident that both sky color and the observer perception of this color are very sensitive to changes of the median radius of the fine mode r Vf for all aerosols. When decreasing the value of r Vf from the reference case, color differences are more abrupt than when increasing the value (and , , ). This is the case for all aerosols and all azimuth angles ϕ. For larger angles, however, this behavior is less noticeable. According to the scattering coefficient varies less when r Vf changes towards larger diameters than when it changes towards the smaller ones. Decreasing r Vf, while keeping C Vf constant, results in an increase of the population of the particles for that mode. On the other hand, as r Vf decreases, fine mode particles are becoming inefficient scatterers. The combination of the above two competing factors results in an overall decrease of the scattering coefficient of the aerosols (, solid black line). Increasing r Vf, initially increases the scattering ability for the majority of the fine mode particles up to a peak value. A further increase of r Vf results in a decrease of its scattering ability. In addition, increasing r Vf results in a decrease of the aerosol number population. The combination of the above has less abrupt color difference effect than when decreasing r Vf.
FIG. 6 Scattering coefficients at 550 nm as a function of the variation of the control parameters. The units are in km−1.
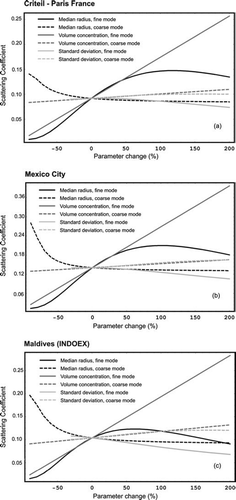
At the lower limit of the r Vf variation (−90%) aerosol scattering is small. As a result ΔE94* is very high (more than 30 units at φ = 30°, , ) and the sky colors are closer to those of an optically clearer atmosphere. The stiff slops of the negative per cent changes of the r Vf curves reveal that the observer of the reference case better comprehends sky color differences between the aerosol polluted atmospheres relative to an optically clearer than relative to more optically polluted ones.
shows that the observer is less sensitive to r Vc. As r Vc decreases from the reference case, more coarse particles enter the fine mode regime and become more efficient scatterers. At the same time the population of the particles increases, further reinforcing coarse mode scattering (). The overall result is the rise of the r Vc related Δ E94* curve of , (b, d, f) at the lower parameter limit. Aerosol scattering and correspondingly sky color exhibit slight changes for higher than the reference case r Vc values. Therefore the sensitivity of the observer is limited (). According to the observer is still capable to detect color changes for large positive percent variations. The slope of r Vc curve of and confirms that if the JND limit is higher than one ΔE94* unit, then, doubling or tripling this threshold will make color shifts undetectable.
We have also found a strong dependence of both r Vf and r Vc on azimuth angle ( and ). Observer sensitivity decreases from 30° to 90°. Since azimuth angle is directly related to the scattering angle of the single scatter radiation, this dependence can be attributed to the shape of the aerosol phase function.
3.2.2. Variation of the Volume Concentration C Vf and C Vc
We have noted in the previous section that the observer appears to be more sensitive to sky color shifts, when these shifts are driven from diminishing optical pollution, with respect to the reference case. This type of behavior is more evident in the case of C Vf and C Vc variations ( and ). Very low values of volume concentration are related directly to an aerosol free atmosphere. Increasing the volume concentration, while keeping the particles radius fixed, is directly related to increasing the population of the particles and therefore increasing air pollution due to aerosols. As expected from Mie theory, the relation between the aerosol ability to scatter light and the C Vf, C Vc parameters is linear (). Although at the upper limit of both C Vf and C Vc variation, the aerosol population is tripled, it is evident from and that the observer is still more sensitive to color variations due to decreasing the aerosol population. Increasing C Vc does not affect the scattering ability of the aerosol substantially, since the latter is dominated by the scattering ability of the fine aerosol. This results in the low magnitude ΔE94* curves of and . Despite this, the observer, as it is shown in , is capable to detect color changes when the ΔE94* unit threshold equals one. Once again increasing the ΔE94* unit threshold makes color shifts undetectable.
According to , the observer ability to detect color change due to variation of the volume concentration is less affected by the azimuth angle, than in the cases of the r Vf and r Vc variation. This is due to the fact that the variation of the volume concentration does not alter the shape of the aerosol phase function. However, when ϕ increases, the Δ E94* curves of tend to rise for very low C Vc values and to decrease for very high C Vc values. This is due to the fact that particles of the fine mode regime and especially those of the coarse regime tend to scatter more radiation in the forward (ϕ = 30°) than in the backward (ϕ = 120°) directions (Seinfeld and Pandis 1998).
3.2.3. Variation of the Standard Deviation σ f and σ c
The ΔE94* curves of and depict that the observer is not very sensitive to changes of σf and especially to those of σc. According to the aerosol scattering ability is almost unaffected by changes of σf. indicates that σf variations as small as −1.9%, while +14% can be detected by the observer. An investigation however of the shape of the curves in both and makes apparent that increasing the ΔE94* unit threshold will drastically diminish the observer's ability to discriminate color, especially in the case of φ = 90° (, ). Color variations relevant to changes of σc exhibit a dependence on the direction of observation and therefore on the aerosol phase function. A Paris aerosol observer at φ = 30° is more sensitive to σf changes than the Maldives and Mexico City observers, while at φ = 120° the Maldives observer is the most sensitive. Increasing or decreasing σf values result in widening or narrowing the particle fine mode band. Increasing σf, while keeping C Vf and r Vf fixed, also increases the number of small particles and decreases the number of larger particles of the fine mode. Thus it moves the number distribution of the fine mode towards smaller radiuses that is less efficient in scattering. This results in the limited decrease of the aerosol scattering coefficient (). On the other hand, though, the previously described changes of the aerosol distribution have stronger influence on the phase function.
3.2.4. Variation of the Real Part of the Refractive Index n f and n c
The lower per cent limit for n f and n c variations was set, so that each of the aerosol modes acquires the refractive index of water (n = 1.33) (Seinfeld and Pandis 1998). The most sudden color changes are perceived when we perturb the real part of the aerosol fine mode n f (, , , ), while the observer is the least sensitive to variations of n c . For the same changes, aerosols scattering coefficients exhibit large variations for the fine mode and are left invariant for the coarse mode ().
FIG. 7 Scattering coefficients at 550 nm as a function of the variation of the fine mode (solid lines) and coarse mode (dashed lines) refractive indices. The units are in km−1.
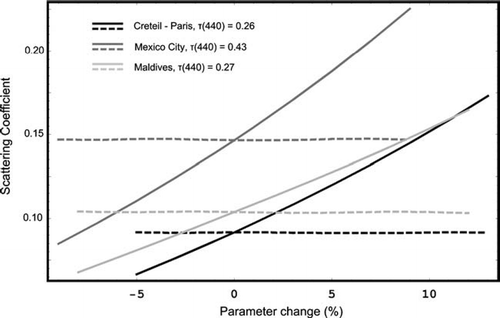
Among the three aerosol cases, the Maldives observer is the least sensitive to n f changes, since, in this case, C Vf has the lowest value (). The Paris aerosol observer is the most sensitive, while the Mexico City observer is in between (). These results are in agreement with the scattering coefficients of . We can also observe a strong dependence of n f to changes of the azimuth angle. Observations in the forward direction give better color discrimination than observations in the backward direction. Once more this is attributable to the shape of the aerosol phase function.
3.2.5. Variation of the Imaginary Part of the Refractive Index k f and k c
Observers are insensitive to variations of the imaginary part of the refractive index for both aerosol modes, k f and k c . These parameters are responsible for the absorption properties of the aerosol (CitationPilinis and Pandis 1995). Mexico and Maldives aerosols are considered strong absorbing with single scattering albedos ω o (550 nm), of 0.89 and 0.90 and with reference case k f and k c at 0.014 and 0.011, respectively. Absorption of Creteil, Paris aerosol is moderate with ω o (550 nm) at 0.94 (CitationDubovik et al. 2002) and reference case values of k f , k c equal to 0.009. For the case of Creteil, Paris aerosol changes remain undetected for the full range of variation of k c for any angle ϕ, while in all other cases, color differences remain undetected, except when k c approaches a value of zero (non-absorbing aerosol). The observer of Mexico City aerosol appears to be more sensitive to variations of k f , but is not accurate. The higher reference case values for both k f and k c result in larger deviations from the reference case for the same percent change. For an angle ϕ of 90° we have calculated the magnitude of the k f decrease needed, in order to reach the 1 ΔE94* unit threshold. For the Creteil, Paris, Mexico City, and Maldives cases k f must decrease by 0.0016, 0.0064, and 0.0029, respectively, in order for the observer to detect color shifts. This means that the Paris observer is the most sensitive to changes of k f .
3.3. Camera Behavior Evaluation
presents the statistical evaluation of the agreement between the digital camera and the behavior of the standard observer, with respect to sky color changes. According to the presented results, the camera is in excellent agreement with the behavior of the standard observer, as presented in Section 3.2. The case corresponding to the perturbations of the n c parameter has not been considered. We have already mentioned that the observer is incapable to discriminate color changes due to variations of n c . Further analysis of the simulations revealed that n c changes result in a very limited shift of sky color. In other words, the sky color practically remains constant. Consequently, the statistics in for the n c parameter are meaningless.
TABLE 3 Summary of the camera agreement to the theoretical estimated values of the observer sensitivity
3.4. Camera Sensitivity to Variations of the Aerosol Parameters
Camera sensor consists of a two dimensional array of photosites. Each element of the final image (pixel) is estimated by using information from three photosites (color channels), red, green, and blue. Our model simulates the camera performance. The properties of every image element are the final tristimulus XYZ values calculated from the original sensor color channels recorded values. Since sensors consist of millions of photosites, we can assume that many pixels of the final image practically record the same sky color, especially when using narrow field of view lenses (telephoto lenses). Since our model also incorporates sensor noise sources, each measured X rec , Y rec , Z rec value is paired with the measurement standard deviations σX, σ Y , and σZ. Let X rec,bc , Y rec,bc , and Z rec,bc be the sky color reference case tristimulus values measured by the camera and σX,bc , σ Y,bc , and σZ,bc their uncertainties. For a single pixel we define the Signal-to-Noise Ratio (SNR) relative to the reference case for the X color coordinate:
⟨X rec ⟩ and ⟨X rec,bc ⟩ being the average values of m p pixels. SNR Y and SNR Z are defined accordingly. There are various ways to choose the SNR that one can use in order to examine whether the camera can detect sky color shifts. For reasons of simplicity we have defined the camera SNR as the average of the XYZ SNRs:
Camera can positively detect a color change if the above SNR has a value above one unit. This practically means that the recorded data participating in the estimation of |⟨X rec ⟩ −⟨X rec,bc ⟩ | are stronger than the inherent noise of these data. The results presented below are similar regardless of the choice of the SNR.
presents the results of the camera sensitivity relative to the variations of the ten aerosol control parameters. was constructed in a similar way to , e.g., by assuming a threshold of 1 SNR unit. We used 500 image elements to estimate camera SNRs (m p = 500). The camera is far more sensitive than the human observer. Once more, color shifts caused by changing the parameters of the fine mode are more easily detected than changes of the coarse mode. The camera, however, is also very sensitive to changes of the coarse mode. It is interesting to note that the camera appears more sensitive to r Vf than the n f parameter. This contradicts the results of . It appears that the actual color is more sensitive to changes of r Vf but, due to the properties of the human vision, the observer mostly understands changes of n f . According to the camera sensitivity is dependent on the type of urban-industrial aerosol and on the azimuth angle φ. In many cases the values in are highly correlated to those of . Since almost no color shifts occur when changes of n c take place, SNR values were very low and no color difference could be identified by the camera measurements.
TABLE 4 Summary of the camera sensitivity to the variations of the aerosol parameters
4. SUMMARY AND CONCLUSIONS
The sensitivity of the human observer to shifts of the sky color relative to changes of various urban-industrial aerosol parameters has been studied. Detail knowledge of the human observer reactions is useful in understanding the importance of the various aerosol properties, when related to visibility and visual air quality. Results from the three urban-industrial aerosols used, reveal that the observer is more sensitive to changes of the fine than the aerosol coarse mode. The observer is most sensitive to changes of the fine mode real part of the refractive index, n f , and of the median radius, r Vf following by changes of the volume concentration C Vf, and of the mode standard deviation, σf. On the contrary, changes of coarse mode real part of the refractive index, n c are not detectable, while changes of that modes k c , and σc are hard to detect. The results of this analysis can be used to explain how the observer will respond, when physico-chemical changes of the atmospheric aerosols occur over a city. Consider, for example the scenario of an aerosol population undergoing growth, due to an increase of the ambient relative humidity. Hygroscopic growth is always associated with shifting the aerosol fine mode distribution towards the coarse mode (CitationPilinis and Pandis 1995) and increasing the volume concentration (CitationDubovik et al. 2002). This also results in lower values of n f due to water ptake of the aerosol particles. According to , in this scenario, the observer is capable of detecting changes of n f as low as of ± 1%, in the case of r Vf as low as ± 4.5% and in the case of C Vf as low as ± 8.5% in magnitude. Obviously all changes contribute to the perception of color shifts, but the reference case observer mostly understands changes due to n f perturbation and secondly to r Vf and C Vf.
A specialized camera system equipped with the appropriate software that converts tristimulus XYZ values to CIE-94 color difference estimations can closely replicate the behavior of the standard observer. We have proven that the camera, can replicate the behavior of the observer to sky color changes. This study shows that such a camera system could to be used to investigate further the sensitivity of humans to sky color shifts, when these shifts are related to the aerosol characteristics. The same system is also useful for predicting the observer reaction to these color changes.
We have also shown that the camera is more sensitive to color shifts than the human observer. This implies that the camera could be used as a monitoring system. Since camera is more sensitive than the observer in detecting color differences such a device can be used to study and to monitor sky color changes that result from pollution episodes.
Acknowledgments
This work was supported by funding from EUCAARI (European Integrated project on Aerosol Cloud Climate and Air Quality Interactions) Contract number: 36833.
Notes
1In this article the “standard human observer” is an observer with normal color vision that is compatible with the CIE (Commission Internationale de l'Eclairage) standards of color especially when referring to the Just Noticeable Difference Concept (JND). CIE is the primary organization responsible for standardization of color metrics and terminology (CitationSharma 2003).
aAerosol average optical thickness values at 440 nm used to estimate base cases (CitationDubovik et al. 2002).
bBoth fine and coarse modes of the base cases are considered to have the same n and k.
aSlope m, is calculated by m = (∑ i = 1 n O i P i − 1/n∑ i = 1 n O i ∑ i = 1 n P i)/(∑ i = 1 n O i P i − 1/n∑ i = 1 n O i ∑ i = 1 n P i ) (∑ i = 1 n O i 2 − 1/n (∑ i = 1 n O i )2). (∑ i = 1 n O i 2 − 1/n(∑ i = 1 n O i 2)), O i are the estimated CIE-94 color differences based on the camera measurement while P i are the theoretically predicted color differences. N is the population of O i or P i.
bCorrelation coefficient ρ is calculated by ρ = m s o /s o s p . sp, s o and sp being the standard deviations of O i and P i , respectively.
REFERENCES
- Adams , C. N. , Plass , G. N. and Kattawar , G. W. 1974 . The Influence of Ozone and Aerosols on the Brightness and Color of the Twilight Sky . J. Atmos. Sci. , 31 : 1662 – 1674 .
- Bäumer , D. , Versick , S. and Vogel , B. 2007 . Determination of the Visibility Using a Digital Panorama Camera . Atmos. Environ. , Doi: 10.1016/J.Atmosenv.2007.06.24
- Berk , A. , Anderson , G. P. , Acharya , P. K. , Hoke , M. L. , Chetwynd , J. H. , Bernstein , L. S. , Shettle , E. P. , Matthew , M. W. and Adler-Golden , S. M. 2003 . Modtran4 Version 3 Revision 1 User's Manual , Air Force Research Laboratory, Space Vehicles Directorate .
- Bodrogi , P. and Tarczali , T. 2001 . Colour Memory for Various Sky, Skin and Plant Colours: Effect of the Image Context . Col. Res. Appl. , 26 ( 4 ) : 278 – 289 .
- Broadbent , A. D. 2008 . Calculation from the Original Experimental Data of the Cie 1931 RGB Standard Observer Spectral Chromaticity Co-Ordinates and Color Matching Functions , Munsell Color Science Laboratory . Available from: www.Cis.Rit.Edu/Mcsl/Research/1931.Php
- Cie . 1995 . Industrial Colour-Difference Evaluation Cie Publication 116–1995
- Dave , J. V. 1978 . Extensive Datasets of the Diffuse Radiation in Realistic Atmospheric Models With Aerosols and Common Absorbing Gases . Solar Energy , 21 : 361 – 369 .
- Dave , J. V. 1980 . Simulation Colorimetry of the Earth–Atmosphere System . Remote Sens. Environ. , 9 : 301 – 324 .
- Dave , J. V. 1981 . Transfer of Visible Radiation in the Atmosphere . Atmos. Environ. , 15 ( 10/11 ) : 1805 – 1820 .
- Dave , J. V. and Mateer , C. L. 1968 . The Effect of Stratospheric Dust on the Color of the Twilight Sky . J. Geophys. Res. , 73 : 6895 – 6913 .
- Dogras , C. K. , Ioannidou , M. P. and Crissoulidis , D. P. 2004 . Analytical Study of the Changes in the Color of Daylight Due to Sulfate Droplets and Soot Grains in the Atmosphere . Journal of Quantitative Spectroscopy & Radiative Transfer , 84 : 223 – 238 .
- Du , K. , Rood , M. J. , Kim , B. J. , Kemme , M. R. , Franek , B. and Mattison , K. 2007 . Quantification of Plume Opacity by Digital Photography . Environ. Sci. Technol , 41 : 928 – 935 .
- Dubovik , O. , Holben , B. , Eck , T. F. , Smirnov , A. , Kaufman , Y. J. , King , M. D. , Tanré , D. and Slutsker , I. 2002 . Variability of Absorption and Optical Properties of Key Aerosol Types Observed in Worldwide Locations . J. Atm. Sci. , 59 : 590 – 608 .
- Dubovik , O. and King , M. D. 2000 . A Flexible Inversion Algorithm for Retrieval of Aerosol Optical Properties from Sun and Sky Radiance Measurements . J. Geophys. Res.—Atm. , 105 : 20673 – 20696 .
- Eastman Kodak Co . 2003 . Kaf-5101ce Image Sensor Available from: http://www.Kodak.Com/Global/En/Digital/Ccd/ Device Performance Specification
- Eck , T. F. , Holben , B. N. , Dubovik , O. , Smirnov , A. , Slutsker , I. , Lobert , J. M. and Ramanathan , V. 2001 . Column Integrated Aerosol Optical Properties Over Maldives During Ne Monsoon for 1998–2000 . J. Geophys. Res. , 106 : 28 555 – 28 566 .
- Eldering , A. , Hall , J. R. , Hussay , K. J. and Cass , G. R. 1996 . Visibility Model Based on Satellite-Generated Landscape Data . Environ. Sci. Technol , 30 ( 2 ) : 361 – 370 .
- Eldering , A. , Larson , S. M. , Hall , J. R. , Hussay , K. J. and Cass , G. R. 1993 . Development of an Improved Image Processing Based Visibility Model . Environ. Sci. Technol , 27 : 626 – 635 .
- EPA . 1979 . Protecting Visibility: An Epa Report to Congress , U.S. Environmental Protection Agency . Available using http://Vista.Cira.Colostate.Edu/Improve/Publications/Principle/Epa_Report/Epa_Report.Htm
- Finlayson , G. D. and Drew , M. S. 1997 . Constrained Least-Squares Regression in Color Spaces . J. Electronic Imaging , 6 ( 4 ) : 484 – 493 .
- Haber , J. , Magnor , M. and Seidel , H. P. 2005 . Physically-Based Simulation of Twilight Phenomena . ACM Transactions on Graphics , 24 ( 4 ) : 1353 – 1373 .
- Haralabidis , P. E. and Pilinis , C. 2005 . Linear Color Camera Model for a Skylight Colorimeter With Emphasis on the Imaging Pipeline Noise Performance . J. Electron. Imaging , 14 ( 4 ) Art. No. 043005
- Henry , R. C. 2002 . Just-Noticeable Differences in Atmospheric Haze . J. Air Waste Manag. Assoc , 52 : 1238 – 1243 .
- Hernández-Andrès , J. , Lee , R. L. and Romero , J. 1999 . Calculating Correlated Color Temperatures Across the Entire Gamut of Daylight and Skylight Chromaticities . Appl. Opt. , 38 ( 27 ) : 5703 – 5709 .
- Hernández-Andrès , J. , Lee , R. L. and Romero , J. 2003 . Color and Luminance Asymmetries in the Clear Sky . Appl. Opt. , 42 ( 3 ) : 458 – 464 .
- Hernández-Andrès , J. and Romero , J. 2001 . Colorimetric and Spectroradiometric Characteristics of Narrow-Field-of-View Clear Skylight in Granada, Spain . J. Opt. Soc. Am. , 18 ( 2 ) : 412 – 420 .
- Holben , B. N. , Eck , T. F. , Slutsker , I. , Tanré , D. , Buis , J. P. , Setzer , A. , Vermote , E. , Reagan , J. A. , Kaufman , Y. J. , Nakajima , T. , Lavenu , F. , Jankowiak , I. and Smirnov , A. 1998 . Aeronet—A Federated Instrument Network and Data Archive for Aerosol Characterization . Remote Sens. Environ. , 66 : 1 – 16 .
- Jackèl , D. and Walter , B. 1997 . Modeling and Rendering of the Atmosphere Using Mie-Scattering . Compute Graphics Forum , 16 : 201 – 210 .
- Larson , S. M. , Cass , G. R. , Hussay , J. H. and Luce , F. 1988 . Verification of Image Processing Based Visibility Models . Environ. Sci. Technol , 22 ( 6 ) : 629 – 637 .
- Latimer , D. A. , Hogo , H. and Daniel , T. C. 1981 . The Effects of Atmospheric Optical Conditions on Perceived Scenic Beauty . Atmos. Environ. , 15 ( 10/11 ) : 1865 – 1874 .
- Lee , R. L. Jr. 1994 . Twilight and Daytime Colors of the Clear Sky . Appl. Opt. , 33 ( 21 ) : 4629 – 4638 .
- Lee , R. L. Jr. and Hernández-Andrès , J. 2003 . Measuring and Modeling Twilight's Purple Light . Appl. Opt. , 42 ( 21 ) : 445 – 457 .
- Lòpez-Álvarez , M. A. , Hernández-Andrès , J. , Romero , J. and Lee , R. L. Jr. 2005 . Designing a Practical System for Spectral Imaging of Skylight . Appl. Opt. , 44 ( 27 ) : 5688 – 5695 .
- Luo , C. H. , Wen , C. Y. , Yuan , C. S. , Liaw , J. J. , Lo , C. C. and Chiu , S. H. 2005 . Investigation of Urban Atmospheric Visibility By High-Frequency Extraction: Model Development and Field Test . Atmos. Environ. , 39 : 2545 – 2552 .
- Macadam , D. L. 1981 . Perceptual Significance of Colorimetric Data for Colors of Plumes and Haze . Atmos. Environ. , 15 ( 10 ) : 1797 – 1981 .
- Mahy , M. , Eyckden , L. V. and Oosterlinck , A. 1994 . Evaluation of Uniform Color Spaces Developed After the Adoption of the Cielab and Cieluv . Color Res. Appl. , 19 ( 2 ) : 105 – 121 .
- Malm , E. C. , Molenar , J. V. and Chan , L. Y. 1983 . Photographic Simulation Techniques for Visualizing the Effect of Uniform Haze on a Scenic Resource . J. Apca , 33 : 126 – 129 .
- Mandel , J. 1984 . The Statistical Analysis of Experimental Data , 72 New York : Dover Publications .
- Molenar , J. V. , Malm , W. C. and Johnson , C. E. 1994 . Visual Air Quality Simulation Techniques . Atmos. Environ , 28 ( 5 ) : 1055 – 1063 .
- Molnár , A. and Mészáros , E. 2001 . On the Relation Between the Size and Chemical Composition of Aerosol Particles and their Optical Properties . Atmos. Environ. , 35 : 5053 – 5058 .
- Pilinis , C. and Li , X. 1988 . Particle Shape and Internal Inhomogeneity Effects on the Optical Properties of Tropospheric Aerosols of Relevance to Climate Forcing . J. Geophys. Res. , 103 : 3789 – 3800 .
- Pilinis , C. and Pandis , S. N. 1995 . “ Physical Chemical and Optical Properties of Atmospheric Aerosols ” . In The Handbook of Environmental Chemistry: Airborne Particulate Matter , Edited by: Kouimtzis , T. and Samara , C. 99 – 124 . Springer . 4(D)
- Pitchford , M. L. and Malm , W. C. 1994 . Development and Applications of a Standard Visual Index . Atmos. Environ. , 28 ( 5 ) : 1049 – 1054 .
- Pratt , W. K. 1978 . Digital Image Processing , 35 72 New York : Wiley Interscience .
- Remer , L. A. , Gassò , S. , Hegg , D. A. , Kaufman , Y. J. and Holben , B. N. 1997 . Urban/Industrial Aerosol: Ground-Based Sun/Sky Radiometer and Airborne In Situ Measurements . J. Geophys. Res.—Atmos , 102 ( D14 ) : 16849 – 16859 .
- Richards , L. W. , Stoelting , M. and Hammarstrand , R. G. M. 1989 . Photographic Method for Visibility Monitoring . Environ. Sci. Technol , 23 : 182 – 186 .
- Seinfeld , J. H. and Pandis , S. N. 1999 . Atmospheric Chemistry and Physics: from Air Pollution to Climate Change , 507 – 518 . 1118 1124 1127 1132 New York : Wiley Interscience .
- Sharma , G. 2003 . Digital Color Imaging , 5 7 15 17 – 20 . 30 33 Boca Raton : CRC Press .
- Sloup , J. A Survey of the Modeling and Rendering of the Earth's Atmosphere . Proceedings of the 18th Spring Conference on Computer Graphics . pp. 141 – 150 .
- Trijonis , J. C. , Malm , W. C. , Pitchford , M. , White , W. H. , Charison , R. and Husar , R. 1990 . Visibility: Existing and Historical Conditions—Causes and Effects . Napap Report , 24 ( Iii ) : 24/31 Available using http://Vista.Cira.Colostate.Edu/Improve/Publications/Publications.Htm
- Tsay , S. C. , Stephens , G. L. and Greenwald , T. J. 1991 . An Investigation of Aerosol Microstructure on Visual Air Quality . Atmos. Environ. , 25a ( 5/6 ) : 1039 – 1053 .
- Watson , J. G. 2002 . Visibility: Science and Regulation . J. Airwaste Manag. Assoc , 52 : 628 – 713 .
- Wyszecki , G. and Stiles , W. S. 1982 . Color Science: Concepts and Methods, Quantitative Data and Formulae, , 2nd Ed. , 486 New York : John Wiley & Sons .