Abstract
A nodal aerosol dynamics model TUTEAM (Tampere University of Technology Exhaust Aerosol Model) was developed to study the number and mass evolution of exhaust particles in a dilution system. The model includes processes such as nucleation, condensation, coagulation, and wall losses and it takes into account the dilution and temperature cooling profiles. The model considers nucleation and soot modes separately. For soot particles the fractal structure was implemented in the model to take the density and size, which continuously vary due to condensation, into account accurately. The model was compared with a set of dynamometer measurements of a heavy-duty diesel vehicle with different lubricant oils and fuels, and with a sectional aerosol dynamics model AEROFOR. The trends of nucleation mode geometric median diameter and number concentration as a function of fuel and lubricant sulphur contents were reproduced well. Also the predicted particle composition was in agreement with the measurements of CitationSchneider et al. (2005).
1. INTRODUCTION
During the last decade, the health effects of urban aerosols (e.g., CitationDockery et al. 1993) have become a major concern for the society. At the same time, the radiation forcing by the natural and human-related aerosol particles has become a pressing concern when predicting global climate-change. In urban areas of developed countries, traffic is the largest single source of aerosol. Diesel engines produce significantly more particulate matter both in terms of number and mass concentration than other combustion engines used in vehicles. In terms of mass, the most important component of the diesel aerosol is the soot mode composed of non-volatile particles in the range of 20 nm to few hundreds of nanometers (e.g., Kittelson 1998). During the recent years, the properties and development of this mode have become well known (e.g., Kittelson 1998, CitationVirtanen et al. 2002). The soot mode size distribution can be described by a log-normal distribution with a geometric standard deviation of circa 1.8 (CitationHarris and Maricq 2002). The soot mode particles are fractal-like agglomerates of carbonaceous primary particles.
Semi-volatile compounds in the exhaust gas may get adsorbed onto the soot particles. During dilution and cooling of the exhaust, these compounds may condense on the particles (e.g., CitationCanagaratna et al. 2004; CitationSchneider et al. 2005) In this respect, the structure of the soot particles is particularly interesting. As the particles are fractal-like, they have a density which decreases as a function of size, a phenomenon experimentally detected by CitationSkillas et al. (1998). The empty space between the primary particles of the agglomerate means that substantial mass transfer to the particle may take place without significant mobility diameter growth, a point experimentally validated by CitationRistimäki et al. (2007).
Besides soot particles, the diesel exhaust features in some cases a second mode consisting of ultrafine particles (Kittelson 1998; CitationKhalek et al. 2003; CitationVogt et al. 2003; CitationVaaraslahti et al. 2004; CitationMathis et al. 2004; CitationVaaraslahti et al. 2005; CitationGiechaskiel et al. 2005; CitationRönkkö et al. 2006). These particles are usually thought to be formed and grown during the dilution and cooling occurring in the exhaust after exiting the tailpipe (e.g., CitationZhang and Wexler 2004). Because of the mass proportional sensitivity of the measurements, the experimental composition data mostly relates to the larger grown nucleation mode particles. The compounds found on these particles are the same as those transferred onto the soot particles, namely long alkanes with 24–32 carbon atoms per molecule (CitationTobias et al. 2001; CitationLee et al. 2006), sulphuric acid and water (CitationSchneider et al. 2005).
There is not much direct evidence about the composition of the actual nucleating species. Indirectly, this has been supported by experimental evidence linking nucleation mode formation to high sulphur content in the fuel, the lubricant, or the exhaust gas (e.g., CitationLepperhoff 2001; CitationMaricq et al. 2002; CitationVaaraslahti et al. 2005; CitationArnold et al. 2006). CitationVaaraslahti et al. (2004) proposed two processes for the formation of nucleation mode. In case of high engine loads and with Continuously Regenerating Trap (CRT) exhaust after-treatment technology, the nucleation mode formation is sulphur-driven. Without after-treatment, at low loads, the mode formation was not correlated to the amount of sulphur, but to the hydrocarbon compound content of the exhaust. There is recent evidence that in this case the nucleation mode may be formed onto solid nuclei particles of a few nm in diameter (CitationRönkkö et al. 2006).
The first modelling studies of nucleation in diesel exhaust were carried out by CitationBaumgard and Johnson (1996) and by CitationShi and Harrison (1999). They both considered only the nucleation rates in diesel exhaust, using classical nucleation theory for sulphuric acid nucleation. More comprehensive aerosol dynamic models for the development of diesel exhaust have been proposed by CitationKim et al. (2002) and CitationVouitsis et al. (2005). Kim et al. (2003) studied diesel exhaust nucleation process by applying a nodal model with water-sulphur acid nucleation to a real flow field behind a truck. This approach, although mathematically rigorous, neglects the different chemical properties of the soot mode and the nucleation modes. CitationVouitsis et al. (2005) took these into account in their model involving two modes but their model considers only two monodisperse particle modes. Uhrner et al. (2007) study the diesel aerosol using a realistic flow field, but their study is also limited to a model involving two monodisperse modes. None of the above mentioned models used in diesel exhaust studies, considers the effects of the fractal-like nature of the soot particles.
In this article, a diesel exhaust aerosol model Tampere University of Technology Exhaust Aerosol Model (TUTEAM) involving two particle modes and a nodal approach to particle sizes, was developed to study evolution of exhaust particles in an ageing chamber. The model incorporates the fractal-like nature of soot and considers two externally mixed polydisperse particle modes. In this way, the approach used by Kim et al. (2003) and Uhrner et al. (2007) with monodisperse modes is expanded into a nodal model.
The main problems in applying modelling for diesel exhaust aerosol are: (1) The nucleating species and its concentration are unknown or there may be a solid nucleus, (2) particles are grown by heavy hydrocarbon compounds, whose concentration and thermodynamic properties are unknown, (3) the complex flow field after the tailpipe make it difficult to estimate the dilution and cooling path. We therefore test the model for a specific case of a heavy-duty diesel engine equipped with an oxiding CRT. In this case, (1) the nucleation is thought to be sulphur driven (CitationVaaraslahti et al. 2004, Citation2005), (2) the effect of the heavy hydrocarbons on the particles is minimized because the hydrocarbon content of the exhaust is relatively low (CitationVaaraslahti et al. 2004). The third problem is tackled by using the simple dilution model proposed by CitationLemmetty et al. (2006) and described later in this article.
In this particular case the growth of the fractal soot mode particles through hydrocarbon condensation is not expected to be dominant. It is therefore expected that the atmospheric aerosol models developed for the study of vehicle exhaust evolution in the vicinity of road (e.g., CitationPohjola et al. 2003, Citation2006; CitationZhang and Wexler 2004; CitationDu and Yu 2006) could be applied as well. Although the condensable gas concentrations and the time scales differ by several orders of magnitude, the basic chemistry and physics of aerosol evolution in diesel exhaust are similar to those of the atmospheric aerosols. TUTEAM is therefore compared with the existing updated sectional atmospheric chemistry and aerosol dynamics model AEROFOR (CitationPirjola 1999; CitationPirjola and Kulmala 2001).
The study focuses on the development of the nucleation mode as a function of lubricant and fuel sulphur content. The nucleation is considered to happen through the binary homogeneous H2O–H2SO4-nucleation. In addition, we study the effects of the different dilution schemes, hydrocarbon concentration and the quantum mechanical correction factor introduced by CitationTammet and Kulmala (1995).
2. MODEL DESCRIPTION
2.1. Nodal Exhaust Aerosol Model TUTEAM
The aerosol dynamics model TUTEAM (Tampere University of Technology Exhaust Aerosol Model) involves three major phenomena concerning the exhaust aerosol: condensation, coagulation, and nucleation. The development of the aerosol can be modelled with differential equations involving the conservation of mass and development of number concentration. From these, we solve the particle number size distributions and the size-resolved particle compositions. In the following, all equations are expressed in the SI system, unless otherwise stated.
The molecular concentration C j of vapor j in gas phase is described by equation
Here R c,m,ij is the condensation rate of vapor i on the m-mode particles of size d j . N m,j is their number concentration. R w, i is the loss of the component on the dilution system walls, J is the nucleation rate, n* is the number of molecules in a single stable nucleated cluster, and x i * is the mole fraction of component i in critical nuclei. D i (t) is the rate of change of vapour concentration due to dilution.
The particles are divided into two modes: nucleation mode and soot mode. Inside these modes, the particles are further divided by their size into 230 size-bins (nodes), each of which has its particle size d m,i , number concentration N m,i , density ρ m,i. Unlike the nodal models of CitationPrakash et al. (2003) and CitationMukherjee et al. (2006), which have the nodes located logarithmically evenly with regard to volume, TUTEAM has the particle size bins located logarithmically evenly in regard to diameter. This allows covering a wider range of particle sizes with a smaller number of nodes. In our notation, the index m denotes the mode, i denotes the size bin number and j the chemical component in question. In TUTEAM, the smallest size bin has a diameter of 0.8 nm, and the largest has the diameter of 10 μ m. As the nucleation clusters usually have a size of circa 1 nm, and the (numerical) majority of soot mode particles remain under 1 μ m, the TUTEAM covers the size range of particles present in diesel exhaust. The particles are assumed to consist of four components: water (w), sulphuric acid (ac), organic volatile component (ov), and soot (s). The amounts of substance of the component species j in a single particle of mode m and size bin i are stored in the mole number n m,i,j . These relate to the masses of components in a single particle by
The density ρ m,i of the particles in a mode m and in a size bin i is calculated according to Equation (Equation2), which takes into account the mole fractions of sulphuric acid, water, and organic volatile compound
For soot particles, their fractal nature must be taken into account. This is especially important to account for their condensational growth properties. The effective density of the soot particles in a size bin i (ρ S,i ) may be written in form (CitationSkillas et al. 1998; CitationVirtanen et al. 2004)
2.1.1. Basic Equations
As mentioned above, the volume change of soot particles is not linearly dependent on the mass change. In some cases, the particle mass may even change without any change in particle size. This causes practical problems in evaluating the general dynamic equation in the form given by, e.g., Seinfeld and Pandis (1998, pp. 682–683). Particularly evaluating the coagulation integral over particle sizes which result in particles of size bin i is no longer straightforward. Neither is it straightforward to calculate the particle growth rate due to condensation from the condensation rate as it is in the discussion of Seinfeld and Pandis (1998, pp. 649–650). However, the discussion by CitationGelbard and Seinfeld (1980) takes into account only the conservation of mass, so it remains valid, although it must be expanded to take into account intra-mode coagulation.
For each size section in both modes, TUTEAM tracks particle number concentrations N m,k and the component masses m m,k,j . Here the index m refers to a mode (N = nucleation, S = Soot), I to a size bin and j to a chemical component. During each time-step, the particles in a size bin i grow to a size y m, i. The number concentration of particles is decreased due to coagulation and wall losses. Each collision between two particles results in a new particle. In TUTEAM we consider that the resulting particle is counted to the larger size bin. The particles resulting from collisions between a nucleation mode and soot mode particles are counted into the soot mode.
The change of the mass concentration of the component species j in a size section i during a time step Δ t is described by the equations
If we were to calculate the size change of m-mode, i-bin particles from Equation (Equation7) without taking into account the fact that not only mass concentration, but also the number concentration is changing, we would underestimate the particle mass. This happens because coagulation and wall deposition decrease the particle mass concentration by removing particles from the bin. However, this removal does not change the mass of the particles that remain in the mode. Thus, we must discuss the mole numbers of components in a single particle instead of the composite mass concentrations of a certain size bin.
Let us consider a single particle that is not scavenged by a larger particle during the time step. This particle gains chemical substances from two processes: from condensation, and from coagulation with smaller and equally sized particles. The addition of component j on a single, surviving particle in size bin i is
Here, one must note that the nucleation and the soot modes are treated separately. As any size bin may receive contributions from several other size bins, the particle concentrations of the next time step will be
The chemical composition of the particles is tracked using an equation similar to Equation (Equation10). Now, the new mole numbers of the compounds are calculated from Equation (Equation8). The mole numbers of the particles in a size bin i and in a mode m is simply the weighed average of the composition of particles contributing to that particle size bin according to the Equation (Equation10). The freshly nucleated particles are considered to have the critical cluster composition:
2.1.2. Nucleation
In this article, we assume that the nucleation observed in the measurements is caused by homogeneous nucleation of sulphuric acid and water. For calculation of the nucleation rate, the parameterization of CitationVehkamäki et al. (2003) is used. The parameterization gives the composition and the size of the stable nucleation cluster in addition to the nucleation rate. A major property of the Vehkamäki parameterization is its sensitivity to the temperature and concentrations of sulphuric acid and water. A relatively small change in any of these may cause the nucleation rate to change several orders of magnitude. The sensitivity of the parameterization of CitationVehkamäki et al. (2003) to changes in the dilution model described later and to the component species has been studied in detail by CitationLemmetty et al. (2006). On the basis of discussion by CitationLemmetty et al. (2007), we conclude that the use of ternary H2SO4–H2O–NH3 system would not change our results significantly. The study of the quasi-unary nucleation model of Yu (2006) is outside the scope of this article.
In addition to the nucleation model parameterization of CitationVehkamäki et al. (2003), we have used the barrierless nucleation model (CitationClement and Ford 1999) as applied by CitationVouitsis et al. (2005). They used a quadratic expression J = A[H2SO4]2 for nucleation rate. This comes from the simplified assumption the [H2SO4]2 cluster lifetime is so long that all clusters survive. Although this assumption is somewhat unphysical, CitationVouitsis et al. (2005) have used the expression with some success for a smaller range of sulphuric acid concentrations. We used it to study whether a simpler, computationally more efficient model is capable of reproducing the measurements. For the kinetic coefficient A, we used the fit of CitationVouitsis et al. (2005), which was made on the basis of experimental diesel nucleation data. As in their study, the properties of stable clusters are calculated from the classical parameterization of CitationVehkamäki et al. (2003). A similar fitting of a barrierless model has been undertaken by CitationKulmala et al. (2006), using experimental atmospheric data.
2.1.3. Condensation
Condensation is governed by the traditional equation
The quantum mechanical considerations applying to the condensation of molecules on clusters coagulation also apply, although to more limited extent, to the coagulation of small clusters with each other. Such process is not conceptually different from the condensation of molecules on particles, as nanometer sized clusters are essentially in the same size range as molecules. When two small clusters coagulate, the kinetic energy of the new particle is smaller than the kinetic energy of the preceding particles. The difference must be absorbed as the rotational and vibrational energy of the cluster. Thus, for coagulation, the quantum mechanical correction was used to calculate the sticking coefficient αas described by CitationTammet and Kulmala (2005). In calculating α, the diameter of the particle was selected to be the larger of the two coagulating particles. Because of this, the correction had importance only in the coagulation of clusters below size 2 nm.
Most of the other terms in Equation (Equation12) follow the approach of CitationVouitsis (2005) and the references therein, but the activity coefficient and surface tension require a closer scrutiny. These terms require the knowledge of thermodynamical properties which are not easily found in the literature, i.e., they must be, more or less, guessed. We have used the model of Taleb et al. (1998) used, for example, by CitationKulmala et al. (1998) to parameterize the activities and surface tensions for water–sulphuric acid system.
TABLE 1 Values of thermodynamic parameters in pseudobinary assumption (CitationKulmala et al. 2004)
The actual activities of water and sulphuric acid are obtained by multiplying Equations 10 by Γ pb :
The soot in the pre-existing aerosol has been considered non-volatile, insoluble, and inert in all processes of the particle, so its activity is not needed. The surface tension is calculated also using an equation used to fit surface tensions of aqueous carboxylic acid solutions by Alvarez et al. (1997) and for aerosol modelling by CitationKulmala et al. (2004).
The surface tension of the sulphuric acid–water system, σ pb , is calculated using the approach of CitationVehkamäki et al. (2003).
The concentration of water in the exhaust is at all times so high that the particle is virtually in equilibrium with the surrounding water vapor. The algorithm, however, is capable of identifying situations where this approximation is no longer valid. In these cases, the condensation and evaporation of water is handled similarly to the case of other condensable components. Otherwise, the particles have the equilibrium water content.
2.1.4. Cooling and Dilution Profiles
The cooling and dilution conditions after the tailpipe are dependent on the vehicle model, tailpipe design, vehicle speed, and ambient wind. A rigorous, vehicle-dependent model would require the consideration of all these and has been made for gas components, for example, by CitationWang et al. (2006). If there is no component i in the dilution air, the local instantaneous dilution can be quantified by the dilution ratio
However, many important phenomena can be studied with a much simpler model, and we use the ad hoc–description proposed by CitationLemmetty et al. (2006) in which the temperature and the instantaneous dilution ratio of the gas are assumed to follow simple exponential functions.
2.2. Sectional Aerosol Dynamics Model AEROFOR
Model simulations were also performed by an atmospheric chemistry and aerosol dynamics box model AEROFOR (CitationPirjola 1999; CitationPirjola and Kulmala 2001). The model includes gas phase chemistry, formation of thermodynamically stable clusters by homogeneous H2SO4–H2O nucleation (CitationVehkamäki et al. 2003), condensation of H2SO4, H2O, and an organic vapor onto particles (Fuchs and Sutugin 1970), Brownian coagulation of particles (CitationFuchs 1964), temperature and cooling profiles Equations (15–16) (CitationLemmetty et al. 2006), wall losses Equation (Equation17) as well as mixing with the particle-free dry diluted air. To minimize the effect of numerical diffusion, 200 size sections were used in this work. The same organic vapor properties as explained in section 2.1 were also implemented into AEROFOR. Also used was the quantum rebound correction factor Equations (11) by CitationTammet and Kulmala (2005). The set of stiff differential equations describing the time evolution of the particle number concentrations in each section as well as the vapor concentrations was solved using Numerical Algorithms Group, Ltd. library FORTRAN-routine D02EJF [1990].
AEROFOR uses a more sophisticated method for solving the general dynamic equation than TUTEAM; however, the benefit of TUTEAM in this work is that it enables handling of nucleation and soot mode particles separately along with fractal structure of soot particles.
3. EXPERIMENTAL BASIS OF THE SIMULATION
The models were used to examine one driving situation from the measurement campaign of CitationVaaraslahti et al. (2005). In the measurement, a EURO II–level heavy duty diesel vehicle (Volvo DH 10A-285) was running at 184 kW and 980 Nm (88% of maximum torque, mode 10 of European Standard Cycle) on fuel with 1 ppm fuel sulphur content (FSC). The lubricating oil was varied, the tested sulphur contents are listed in . Oil 1 represents fairly standard commercial synthetic lubricant oil, while the oils with lowest sulphur content were products in development. In the last measurement, the oil number 1 was used and 45 ppm sulphur was added into the fuel. This situation represents a “low-sulphur” fuel used in many countries. No exhaust gas recirculation was used and the exhaust was after-treated with a continuously regenerating trap (CRT). The exhaust was sampled with a two-stage modified “Particulates” dilution, depicted in . In this dilution set-up, the exhaust sample first undergoes a primary dilution, with a dilution ratio of circa 12. After the dilution, the sample resides in an ageing chamber for circa 2 s, allowing the sample to mature. After this, the exhaust sample undergoes a secondary dilution, with a dilution ratio of circa 8, before measured by an ELPI (CitationKeskinen et al. 1992) and two Scanning Mobility Particle Sizers (SMPS): one with a long DMA, the other with a nano-DMA (CitationKnutson and Whitby 1975; CitationWang and Flagan 1990) The hydrocarbon (HC) concentration of the exhaust was measured with a flame ionization detector (FID). The measurement setup has been described in detail by CitationVaaraslahti et al. (2005) and the dilution setup reviewed by CitationNtziachristos et al. (2004). The dilution system used in the measurement has been shown to reproduce on-road measurements satisfactorily by CitationRönkkö et al. (2006).
FIG. 1 Experimental setup, after CitationVaaraslahti et al. (2004).
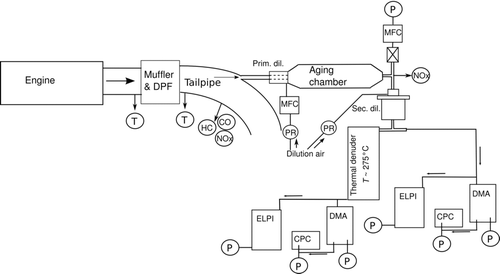
TABLE 2 Data on oils used in the measurement
From the CO2 concentration of the raw exhaust, the stoichiometric air-to-fuel ratio at this measurement point could be calculated to be 1.24. From the statistical information concerning the long-term oil consumption of this engine type we could calculate the oil consumption to be 38.5 g/h, while the fuel consumption was circa 42.5 kg/h. This rough estimate allowed us to calculate the effective fuel sulphur content which takes into account the different lubricant oil sulphur contents (listed in ). In the load point studied in this article, the CRT was hot and, we can approximate the SO2-to-H2SO4 conversion ratio with 90%, on the basis of Gieschaskiel et al. (2007). Although this is only an approximation, we get the right magnitude for the conversion factor and the calculations are more straightforward.
The concentrations of the gas components were calculated stoichiometrically (e.g., CitationBaukal 2004, p. 84), using air-to-fuel ratio chosen to give the measured CO2 concentrations. The molecular number concentration of water in the raw exhaust was 1.53 · 1024 m–3 at a temperature of 400 K. The calculated number concentrations of raw exhaust H2SO4 in 400 K are listed in . The dry, hot soot mode was described using an SMPS measurement made with oil 4 after treating the sample with a thermodenuder, thus removing the volatile component from the soot. This measurement was used to depict the initial, dry soot mode. The distribution of the initial soot mode is depicted in . In AEROFOR, the lognormal parameters for the soot mode used were N tot = 2.4 · 104 cm−3, d = 40 nm and GSD = 2.
FIG. 2 The initial aerosol concentration is taken to be the soot mode measured through a thermal denuder. The initial nucleation mode is set to zero. (Figure is provided in color online.)
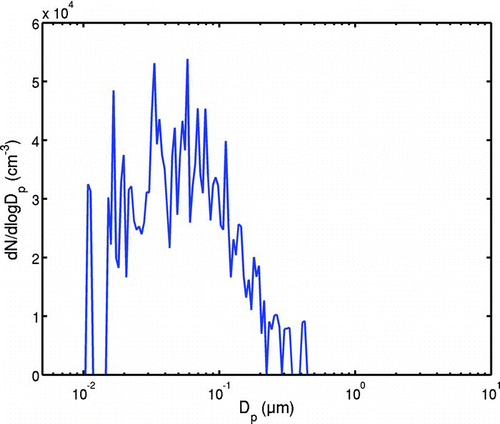
The choice of temperature and dilution profile experienced by the exhaust is extremely important for the results of the simulation. We select the dilution time constant to be τ d = 0.4 s, which is sensible in the light of the results of CitationRönkkö et al. (2006). The final dilution ratio after the primary dilution is D R = 12, as in the experiments of CitationVaaraslahti et al. (2005). The cooling time constant τ c is used as a free parameter to fit the results. The initial temperature T i was 400 K and the final one, T f , 310 K, to make the simulations compatible with the study of CitationLemmetty et al. (2006) The simulation ran over a total time of 2 s, which corresponded to the residence time in the ageing chamber of the measurement setup (CitationVaaraslahti 2006, p. 18). Finally, as the particles undergo a secondary dilution with a dilution ratio of 8, they evaporate slightly. This was modelled as an instantaneous dilution to the final dilution, after which the particles in both modes were given a sufficient time (0.1 s) to reach their equilibrium compositions, corresponding to the gas composition in the measurement devices.
In the measurements of CitationVaaraslahti et al. (2005), there was a pronounced storage effect in the nucleation mode particle number concentration. When the measurement runs were made first with a high-sulphur lubricant, the number concentration of the nucleation mode in subsequent measurements with lower-sulphur lubricant oil was consequently much larger than in the measurements with the same oil, where even lower-sulphur lubricant was used first. CitationVaaraslahti et al. (2005) conclude that this is caused by the sulphur storage in the CRT. To minimize the effect of the sulphur storage on the gas concentrations, we use the measurement runs made with an increasing fuel and lubricant sulphur content ( of CitationVaaraslahti et al., 2005). However, we are compelled to use the measurement with lubricant 2, which is made in the decreasing order of sulphur content, as there was no measurement with this lubricant in the other series. As the H2SO4 concentration is dominated by the sulphur oxidization and storage in the CRT, the eventual H2SO4 losses on the exhaust pipe walls may be neglected.
FIG. 4 Measurements and simulation results with oil 1 and 45 ppm fuel sulphur. Solid line with errorbars, measurements. Dotted red line, AEROFOR results, blue line, TUTEAM soot mode, red line, TUTEAM nucleation mode results. Compared to situation of , the H2SO4 concentration in this case is circa 10 times higher.
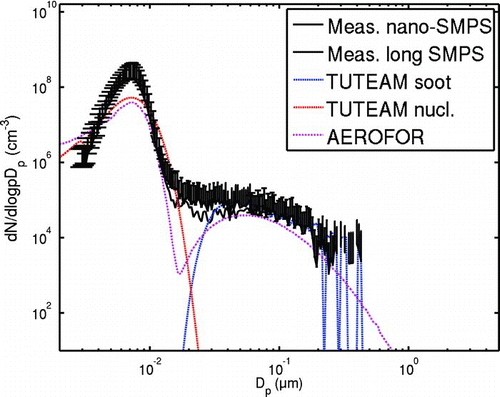
4. RESULTS AND DISCUSSION
The measurement situation was studied by the TUTEAM and AEROFOR models by using the gas and particle concentrations described above. The behavior of the simulation was studied as a function of the cooling time constant τ c and the organic vapor concentration.
The selection of the cooling and dilution time constants is extremely important for the simulation. As CitationLemmetty et al. (2006) showed, the nucleation rate is mostly affected by the ratio τ c /τ d . This means that one of the two time constants may be held fixed, while the other changes. The dilution time constant τ d was given the value 0.4 s, corresponding to the experimentally observed aerosol formation time of CitationRönkkö et al. (2006), while the cooling time constant was selected to fit the geometrical count mean diameter GMD and the nucleation mode number concentration to the measurement made on oil 2. The measurement and the model results are given in . For the TUTEAM, this resulted in value τ c = 0.10 s, which was also used for the AEROFOR. As the dilution system and the flow rates were constant in all the measurements, the time constants fitted for oil 2 were used, respectively, in the other simulation runs presented in this article.
FIG. 3 Measurements and simulation results with oil 2. Solid line with errorbars, measurements. Dotted red line, AEROFOR results, blue line, TUTEAM soot mode, red line, TUTEAM nucleation mode results. This measurement was used to fit the τ c for all other measurements.
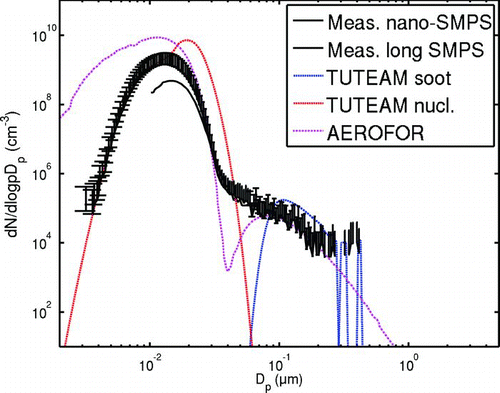
Following the convention of diesel exhaust studies, the particle number concentrations are reduced to the undiluted raw exhaust values at 400 K. The soot mode is typically given in raw exhaust conditions or as an emission factor. Following this practice, even nucleation mode concentrations have been given in raw exhaust conditions (e.g., CitationVaaraslahti et al. 2005, Citation2004; CitationRönkkö et al. 2006), when soot mode coexists with the nucleation mode, although no nucleation mode exists in the raw exhaust. We feel that even though philosophically problematic, this approach is usable.
illustrates the results of TUTEAM and AEROFOR models with oil 2. In the figure, the experimental results with the two sets of SMPS are given as solid lines with errorbars. The result distributions of the models TUTEAM and AEROFOR are given as dotted, colored lines. The measurement depicted in was used to fit the parameter τ c . As the engine load and the flow conditions in dilution system remained constant with different oils, we used the same τ c value for all other fuel and lubricant sulphur levels. Examples of results obtained for these cases are given in (oil 1 and a fuel with 45 ppm sulphur) and 5 (oil 4). Each figure represents the same load point, with the same experimental dilution conditions, but with different fuel and lubricant sulphur levels.
In all situations except with oil 4 (), TUTEAM slightly overestimates the GMD of the nucleation mode. However, the trend perceived is similar to the measurements, as can be seen from . The particle number concentration is estimated reasonably well, as can be seen in . Only with the lowest sulphuric acid concentration, when oil 4 is used, the particle number concentration is underestimated severely by both models. This is not necessarily a problem in the models but can also be a storage effect, because immediately before the run with oil 4, the engine had been used with oils 1–3, in decreasing order of sulphur content. This may have left a sulphur residue into the CRT. As the lubricant oil 4 and the fuel have a very low sulphur level, even a small storage offloading might increase the H2SO4 concentration significantly. The slight deviation between TUTEAM and AEROFOR results is due to different approaches used in solving the equilibrium concentration of water inside the particles.
FIG. 5 Measurements and simulation results with oil 4. Solid line with errorbars, measurements. Dotted red line, AEROFOR results, blue line, TUTEAM soot mode, red line, TUTEAM nucleation mode results. The gross underestimation of the nucleation mode is most likely a result of the wrong gas-phase sulphuric acid concentration estimate.
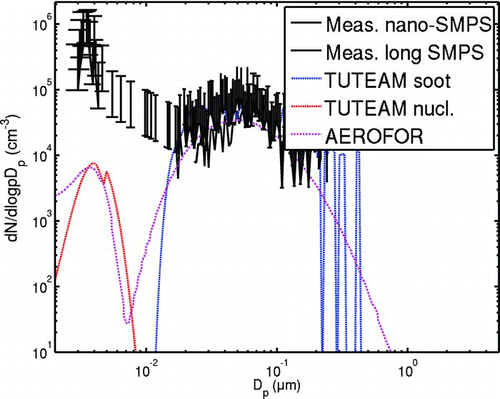
FIG. 6 The nucleation mode GMD as a function of estimated gas-phase H2SO4 concentration. The barrierless nucleation case has been calculated using TUTEAM and the nucleation model of CitationVouitsis et al. (2005).
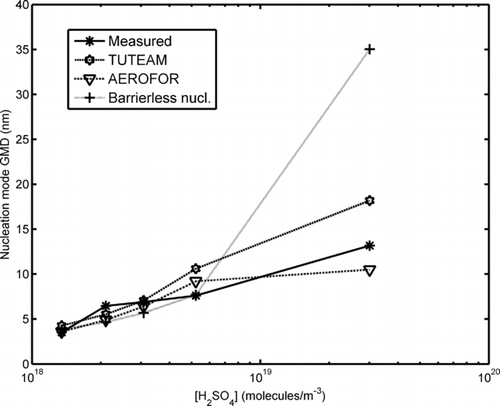
FIG. 7 The nucleation mode particle concentration as a function of estimated gas phase H2SO4 concentration. The barrierless nucleation case has been calculated using TUTEAM and the nucleation model of CitationVouitsis et al. (2005). The errorbars represent the approximate error of the H2SO4 concentration estimate.
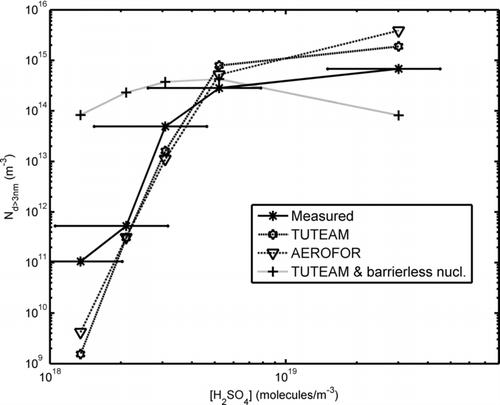
Between the cases of oil 1 with the base fuel and oil 1 with a fuel with 45 ppm sulphur content, the sulphur concentration increases about five-fold. In principle, this might cause an increase of several orders of magnitude in the nucleation rate as CitationVehkamäki et al. (2003) point out, resulting from the extreme sensitivity of the nucleation rate to the gas concentrations. However, the actual increase in the nucleation mode number concentration is much smaller, only about an order of magnitude. This is due to the fact that the nucleation mode itself (nucleated particles and larger particles due to intramode coagulation) causes a condensation sink limiting the growth of total number concentration. Coagulation, on the other hand, has a much smaller influence on the total number concentration, as the time scale of the modelled system is only 2 s. While coagulation remains important, the dominating feature of the model is the condensational growth.
The sensitivity of the models to the uncertainty in the H2SO4 concentration may be inferred from and . Any error made in the estimation would cause the measurement points to shift on the [H2SO4] axis. The most important error sources for this estimation are the uncertainties in the SO2-to-SO3 conversion factor and in the lubricant oil consumption. For the un-doped fuel points the latter uncertainty is believed to be dominant. For simplicity, we have used 50% error estimate for the H2SO4 concentration. This is shown as horizontal error bars for the experimental data points in .
The particle composition is followed by TUTEAM for each particle size in both modes separately. The model predicts nucleation mode particles consisting mainly of water and sulphuric acid. It was found that the model was insensitive to organic vapor concentrations below 1 · 1018 m− 3 and the measurements could be fairly well explained using only the cooling time constant as a free parameter. This behavior is mostly due to the fact that the organic compound has a high molar mass and a fairly large Kelvin effect, decreasing its condensation on the nucleation mode particles. The organic vapor concentration had no significant effect on the size distributions unless it was an order of magnitude over the concentrations measured with the FID. Increasing the organic vapor concentration to such concentrations increases the GMDs of both soot and nucleation modes. These high organic vapor concentrations might well be observed when no oxidizing after-treatment is used.
If we choose a compound with lower molecular mass, the increase in the saturation vapor pressure will cancel out most of the effect of the decrease of the Kelvin effect. Thus, the model behaved similarly when we used the molar masses and calculated vapor pressures of hexadecane or triacontane. Naturally, changing the thermochemical constants of would affect the organic compound behavior greatly. However, a thorough study describing the thermochemical properties of an organic compound which could condense on the particle at the same conditions as H2SO4 is outside the scope of this article.
With a high engine load point and an oxidizing after-treatment system, the hydrocarbon concentration of the exhaust is relatively low. In this case, the process is dominated by H2O–H2SO4 chemistry and can be explained without the organic component of the type used in the atmospheric aerosol modelling of CitationKulmala et al. (2004). This is clearly in conflict with the measurements of CitationTobias et al. (2001) who observed nanoparticles consisting mainly of organic compounds. However, they sampled non-aftertreated exhaust from a diesel engine running at low loads. At such loads, the organic vapor concentration is much higher, as the results of CitationVaaraslahti et al. (2006) show. On the other hand, CitationSchneider et al. (2005) have measured diesel nanoparticles whose chief components were sulphuric acid and water, when the diesel engine load has been high and an oxidation catalyst has been used. Naturally, the particles in the soot mode also have a significant amount of water and sulphuric acid condensed on them, but the change in the actual size distribution is minimal, due to the large size and fractal-like nature of the particles. The change in chemical composition is reasonable in the light of the results of CitationDeMott (2007) who has observed diesel soot acting as hydrophilic ice nucleus when the soot has undergone a cooling–dilution process similar to the one described in this article. In any case however, the current study shows that even a quite simple chemical model may reproduce the observed nucleation mode particle size distribution quite handsomely.
To study the sensitivity of the models to the changes in dilution model, both AEROFOR and TUTEAM were run at numerous different time constants τ c and τ d to test the sensitivity of the models for the changes in dilution parameterization. The model is mainly sensitive to changes in the ratio τ c /τ d . If this ratio is changed, the nucleation mode number concentration and the nucleation mode GMD both change rapidly. A change of five percent in the ratio τ c /τ d changes the nucleation mode GMD by several nanometers and the nucleation mode number concentration by a factor of two or more. On the other hand, for each choice of τ d we can find a value of τ c with which we obtain results very similar to the results presented above. Similarly, most systematic errors in the H2SO4 concentration estimate are lost in the fitting.
4.0.1. Comparison with Barrierless Nucleation Model
and show the TUTEAM results obtained when the barrierless nucleation model of CitationVouitsis et al. (2005) was used instead of the classical parameterization of CitationVehkamäki et al. (2003). In these runs, all other parameters and inputs were kept the same as in the corresponding runs using the standard TUTEAM model. It can be seen that the model of CitationVouitsis et al. (2005) returns mostly about the same nucleation mode GMD as the models using the parameterization of CitationVehkamäki et al. (2003). This is not a wonder, as the stable cluster size and composition in the model of Voutsis et al. (2005) is obtained using the parameterization of Vehkamäki et al. (2005) and the particle growth due to condensation is calculated using the same models as in the base case. However, the nucleation mode particle number concentration as a function of gas H2SO4 follows the measurements poorly. As mentioned earlier, the barrierless model used by CitationVouitsis et al. (2005) uses a square function (or a polynomial function of second order) to depict the nucleation rate as a function of H2SO4 concentration, but the actual dependency of the nucleation rate on the gas concentrations is a much steeper function. Thus, a barrierless model cannot account for the nucleation mode number concentrations over a large spectrum of gas concentrations.
In the case of oil 1 and added fuel sulphur, the barrierless model returns a much too high nucleation mode GMD, as can be seen from . This is due to the fact that the model of CitationVouitsis et al. (2005) takes the stable cluster size from the classical parameterization. With the high H2SO4 concentration present in the input, the barrierless nucleation gives high nucleation rates in situations where the classical stable cluster size is over 10 nm. This unrealistic feature of the model leads to an overly large nucleation mode GMD.
4.0.2. Effect of Quantum Rebound Correction Factor
The quantum mechanical correction factor (Equation [8]), introduced for nucleated clusters by CitationTammet and Kulmala (2005), accounts for the increased number of elastic, i.e., non-sticking, collisions of condensable vapor molecules and the particle that are due to the limited number of energy levels capable of adsorbing the kinetic energy that would be freed in an inelastic collision. For particle diameters above 2 nm, the Tammet factor is negligible and does not affect the condensation rates. For the nucleated clusters, the Tammet factor is of major importance: ψ = 0.02 for d p = 1.2 nm using the estimate of CitationTammet and Kulmala (2005). However, the parameter T 0 should be fitted with the measurements. Due to the lack of this information we assumed T 0 = 0 in the above calculations. Changing the value of T 0 drastically changes the rate at which the nucleated clusters grow into measurable particles. This, in its turn, affects the speed at which the nucleation mode matures. We found that for T 0 = 1000 K the factor ψ of Equation (Equation11) was almost unity already for particles with diameters around 1.6 nm and corresponded the case when no quantum mechanical correction was taken into account. As a comparison, shows an example of the effect of not including the Tammet rebound factor into the model. The particle size distributions in these cases were calculated by TUTEAM with τ c = 0.10 s and τ d = 0.4 s for the case of Oil 2. (All other runs presented in this study were made using the Tammet factor in both AEROFOR and TUTEAM runs.) We can see that setting rebound correction factor to unity slightly increases both the number concentration and the particle size which is reasonable: the quantum rebound slows down the nucleation mode particle growth.
FIG. 8 The nucleation mode development with and without the Tammet quantum rebound factor for oil 2. Assuming that no quantum rebound takes place has the effect of increasing the nucleation mode GMD and decreasing the mode geometric standard deviation. For the sake of clarity, the errorbars of the SMPS-measurement are not drawn.
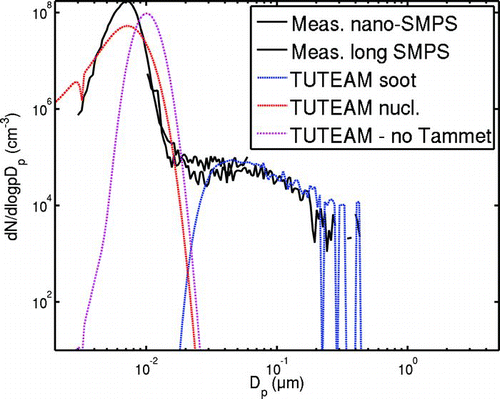
5. CONCLUSIONS
The TUTEAM model presented in this article is an aerosol dynamics model based on nodal approach. It considers the time evolution and interaction of two separate modes having different size distributions and compositions. The composition is followed separately for all size sections in both modes, and the fractal-like geometry of the soot particles is taken into account. The use of classical nucleation theory proved sufficient to explain the trends seen in the diesel nucleation mode measurements. A barrierless nucleation model proves incapable of depicting the extremely steep dependency of the nucleation rate on the gas concentrations well enough or of reproducing measurements over a wide range of initial H2SO4 concentrations, even if it might produce reasonably good fits in single cases.
In this article, the TUTEAM and the AEROFOR are used to reproduce an actual series of measurements. The models give fairly good reproduction of actual measurement data while the only fitting parameter is the cooling time constant and all other parameters were selected on the basis of literature. TUTEAM predicts nucleation particle compositions, which agree with the experimental results of CitationSchneider et al. (2005), who observed nucleation mode particles composed of water and sulphuric acid in the exhaust of an engine running with a similar after-treatment system and at similar load conditions. The organic component assumed in this article does not have an impact on the aerosol formation at measured concentrations. As the chemical and nucleation models which are used in this study are not without uncertainties, the agreement of the model results with the measurements is reasonably good.
In the case of the measurements discussed in this article, we can see that the condensation and nucleation of sulphuric acid and water can alone explain most features of the measured aerosol size distribution. However, according to the results of CitationTobias et al. (2001), CitationLemmetty et al. (2006), and CitationRönkkö et al. (2006) it is likely that some diesel nucleation phenomena are not driven by H2SO4–H2O-system but depend on organic compounds. A most typical such case would appear, if an oxidization catalyst were not in place or would be operating at low temperatures. Then, a more comprehensive chemical model would be needed to account for the organic species. In such study, accounting for the fractal-like geometry of the soot particles would also become a more significant aspect of modelling.
Acknowledgments
This work has been supported by Finnish Academy of Science and Letters, Vilho, Yrjö and KalleVäisälä Fund, by the Academy of Finland, and by Tekes, the Finnish Funding Agency for Technology and Innovation (project LIPIKA). We thank Dr. Kati Lehtovaara (née Vaaraslahti) of Ecocat Ltd (formerly of Tampere University of Technology) for measurement data and helpful advice. We gratefully acknowledge the helpful comments of the reviewers and the editor.
REFERENCES
- Arnold , F. , Pirjola , L. , Aufmhoff , H. , Schuck , T. , Lähde , T. and Hämeri , K. 2006 . First Gaseous Sulphuric acid Detection in Automobile Exhaust: Implications for Volatile Nano-Particle Formation and Health Risk . Atmos. Environ. , 40 : 7097 – 7105 .
- Baukal , C. E. 2004 . Industrial Combustion Pollution and Control , New York : Marcel Dekker .
- Baumgard , K. J. and Johnson , J. H. 1996 . The Effect of Fuel and Engine Design on Diesel Exhaust Particle Size Distributions . SAE Tech. Pap. Ser , 960131
- Birmili , W. , Stratmann , F. , Wiedensohler , A. , Covert , L. , Russell , M. and Berg , O. 1997 . Determination of Differential Mobility Analyzer Transfer Functions Using Identical Instruments in Series . Aerosol Sci. Tech. , 27 ( 2 ) : 215 – 223 .
- Canagaratna , M. R. , Jayne , J. T. , Ghertner , D. A. , Herndon , S. , Shi , Q. , Jimenez , J. L. , Silva , P. J. , Williams , P. , Lanni , T. , Drewnick , F. , Demerjian , K. L. , Kolb , C. E. and Worsnop , D. R. 2004 . Chase Studies of Particulate Emissions From in-Use New York City Vehicles . Aerosol Sci. Tech. , 38 : 555 – 573 .
- Clement , C. F. and Ford , I. J. 1999 . Gas-to-Particle Conversion in the Atmosphere: II. Analytical Models of Nucleation Bursts . Atmos. Env. , 33 : 489 – 499 .
- DeMott , P. J. Progress and Issues in Quantifying Ice Nucleation Involving Atmospheric Aerosols . Nucleation and atmospheric aerosols—17th International Conference . 2007 , Galway, Ireland. Edited by: O'Dowd , C. and Wagner , P. pp. 405 – 417 . Springer .
- Dockery , D. W. , Pope , C. A. III , Xu , X. , Spengler , J. D. , Ware , J. H. , Fay , M. E. , Ferris , B. G. Jr and Speizer , F. E. 1993 . An Association between Air Pollution and Mortality in Six U.S. Cities . New Engl. J. Med. , 329 : 1753 – 1759 . 1993
- Du , H. and Yu , F. 2006 . Role of the Binary H2SO4–H2O Homogeneous Nucleation in the Formation of Volatile Nanoparticles in the Vehicular Exhaust . Atmos. Env. , 40 : 7579 – 7588 .
- Fuchs , N. A. 1964 . The Mechanics of Aerosols , Edited by: Daisley , R. E . New York : Fuchs, and M. Dover Publications .
- Fuchs , N. A. and Sutugin , A. G. 1971 . “ High Dispersed Aerosols ” . In Topics in current aerosol research , Edited by: Hidy , G. M. and Brock , J. R. New York : Pergamon . (Part 2)
- 1990 . The NAG Workstation Library Handbook 1 , Oxford : The Numerical Algorithms Group Ltd. . FORTRAN-routine D02EAF
- Gelbard , F. and Seinfeld , J. H. 1980 . Simulation of Multicomponent Aerosol Dynamics . J. Colloid Interface Sci. , 78 ( 2 ) : 485 – 501 .
- Giechaskiel , B. , Ntziachristos , L. , Samaras , Z. , Scheer , V. , Casati , R. and Vogt , R. 2005 . Formation Potential of Vehicle Exhaust Nucleation Mode Particles on-Road and in the Laboratory . Atmos. Env. , 39 ( 18 ) : 3191 – 3198 .
- Giechaskiel , B. , Ntziachristos , L. , Samaras , Z. , Casati , R. , Scheer , V. and Vogt , R. 2007 . Effect of Speed and Speed-Transition on the Formation of Nucleation Mode Particles From a Light Duty Diesel Vehicle . SAE Tech. Pap. Ser. , 2007-01-1110
- Harris , S. J. and Maricq , M. M. 2002 . The Role of Fragmentation in Defining the Signature Size Distribution of Diesel Soot . J. Aerosol Sci. , 33 ( 6 ) : 935 – 942 .
- Keskinen , J. , Pietarinen , K. and ja Lehtimäki , M. 1992 . Electrical Low-Pressure Impactor . J. Aerosol Sci. , 23 : 353 – 360 .
- Khalek , I. A. , Spears , M. and Charmley , W. 2003 . Particle Size Distribution From a Heavy-Duty Diesel Engine: Steady State and Transient Emission Measurement Using Two Dilution Systems and Two Fuels . SAE Tech. Pap. Ser. , 2003-01-0285
- Kim , D.-H. , Gautam , M. and Gera , D. 2002 . Modeling Nucleation and Coagulation Modes in the Formation of Particulate Matter Inside a Turbulent Exhaust Plume of a Diesel Engine . J. Colloid Interface Sci. , 249 : 96 – 103 .
- Kittelson , D. B. Engines and nanoparticles: A Review . J. Aerosol Sci. , 29 ( 5/6 ) 575 – 588 . [YEAR?]
- Knutson , E. O. and Whitby , K. T. 1975 . Aerosol Classification by Electric Mobility: Apparatus, Theory and Applications . J. Aerosol Sci. , 6 : 443
- Kreith , F. , Boehm , R. F. , Raithby , G. D. , Hollands , K. G. T. Suryanarayana , N. V. “Heat and Mass Transfer.” . In The CRC Handbook of Thermal Engineering , Edited by: Kreith , Frank . Boca Raton : CRC Press .
- Kulmala , M. , Laaksonen , A. and Pirjola , L. 1998 . Parameterizations for Sulfuric Acid/Water Nucleation Rates . J. Geophys. Res , 103 ( D7 ) : 8301 – 8307 .
- Kulmala , M. , Kerminen , V.-M. , Anttila , T. , Laaksonen , A. and O'Dowd , C. 2004 . Organic Aerosol Formation via Sulphate Cluster Activation . J. Geophys. Res. , 109 : D04205
- Kulmala , M. , Lehtinen , K. E. J. and Laaksonen , A. 2006 . Cluster Activation Theory as an Explanation of the Linear Dependence Between Formation Rate of 3 nm Particles and Sulphuric Acid Concentration . Atmos. Phys. Chem. , 6 : 787 – 793 .
- Lee , D. , Miller , A. , Kittelson , D. and Zachariah , M. R. 2006 . Characterization of Metal-Bearing Diesel Nanoparticles Using Single-Particle Mass Spectrometry . J. Aerosol Sci. , 37 ( 1 ) : 88 – 110 .
- Lepperhoff , G. 2001 . Influences on The Particle Size Distribution of Diesel Particulate Emissions . Top. Catal. , 29 : 575 – 588 .
- Lemmetty , M. , Pirjola , L. , Mäkelä , J. M. , Rönkkö , T. and Keskinen , J . 2006 . Computation of Water–Sulphuric Acid Nucleation in Diesel Exhaust with Different Dilution Paths . J. Aerosol Sci , 37 ( 11 ) : 1596 – 1604 .
- Lemmetty , M. , Vehkamäki , H. , Virtanen , A. , Kulmala , M. and Keskinen , J. 2007 . Homogeneous Ternary H2SO4–NH3–H2O Nucleation and Diesel Exhaust: A Classical Approach . Aerosol and Air Quality Res. , 7 ( 4 ) : 489 – 499 .
- Lide , D. R. , ed. 2005 . “ Concentrative Properties of Aqueous Solutions: Density, Refractive Index, Freezing Point Depression, and Viscosity ” . In CRC Handbook of Chemistry and Physics , Boca Raton, FL : CRC Press . Internet version 2005, <http://www.hbcpnetbase.com>2005
- Maricq , M. , Chase , R. , Xu , N. and Laing , P. 2002 . The Effects of the Catalytic Converter and Fuel Sulfur Level on Motor Vehicle Particulate Matter Emissions: Light Duty Vehicles . Environ. Sci. Tech. , 36 : 283 – 289 .
- Mathis , U. , Mohr , M. and Zenobi , R. 2004 . Effect of Organic Compounds on Nanoparticle Formation in Diluted Diesel Exhaust . Atmos. Chem. Phys. , 4 : 609 – 620 .
- Mukherjee , D. , Prakash , A. and Zachariach , M. R. 2006 . Implementation of a Discrete Nodal Model to Probe the Effect of Size-Dependent Surface Tension on Nanoparticle Formation and Growth . J. Aerosol Sci. , 37 ( 10 ) : 1388 – 1399 .
- Ntziachristos , L. , Giechaskiel , B. , Pistikopoulos , P. , Samaras , Z. , Mathis , U. , Mohr , M. , Ristimäki , J. , Keskinen , J. , Mikkanen , P. , Casati , R. , Scheer , V. and Vogt , R. 2004 . Performance Evaluation of a Novel Sampling and Measurement System for Exhaust Particle Characterization . SAE Tech. Pap. Ser , 2004-01-1439
- Pankow , J. F. 1994 . An Adsorption Model for Gas/Particle Partitioning of Organic Compounds in the Atmosphere . Atmos. Env. , 28 : 185 – 188 .
- Pirjola , L. 1999 . Effects of the Increased UV Radiation and Biogenic VOC Emissions on Ultrafine Sulphate Aerosol Formation . J. Aerosol Sci. , 30 ( 3 ) : 355 – 367 .
- Pirjola , L. and Kulmala , M. 2001 . Aerosol Dynamical Model MULTIMONO. Development of Particle Size and Composition Distribution with a Novel Aerosol Dynamics Model . Tellus , 53B : 491 – 509 .
- Pirjola , L. , Korhonen , H. and Kulmala , M. 2002 . Condensation/Evaporation of Insoluble Organic Vapor as Functions of Source Rate and Saturation Vapor Pressure . J. Geophys. Res , 107 ( D11 ) ACH 1-1-1-9
- Pohjola , M. , Pirjola , L. , Kukkonen , J. and Kulmala , M. 2003 . Modelling of the Influence of Aerosol Processes for the Dispersion of Vehicular Exhaust Plumes in Street Environment . Atmos. Environ. , 3 : 339 – 351 .
- Pohjola , M. , Pirjola , L. , Karppinen , A. , Härkönen , J. , Ketzel , M. and Kukkonen , J. 2006 . Evaluation of a Coupled Dispersion and Aerosol Process Model Against Measurements Near a Major Road . Atmos. Chem. Phys. , 7 : 4065 – 4080 .
- Prakash , A. , Bapat , A. P. and Zacharias , M. R. 2003 . A Simple Numerical Algorithm and Software for Solution of Nucleation, Surface Growth, and Coagulation Problems . Aerosol Sci. Tech. , 37 ( 11 ) : 892 – 898 .
- Ristimäki , R. , Vaaraslahti , K. , Lappi , M. and Keskinen , J. 2007 . Hydrocarbon Condensation in Heavy-Duty Diesel Exhaust . Env. Sci. Tech. , 41 ( 18 ) : 6397 – 6402 .
- Rönkkö , T. , Virtanen , A. , Vaaraslahti , K. , Keskinen , J. , Pirjola , L. and Lappi , M. 2005 . Effect of Dilution Conditions and Driving Parameters on Nucleation Mode Particles in Diesel Exhaust: Laboratory and On-Road Study . Atmos. Env. , 40 ( 16 ) : 2893 – 2901 .
- Schneider , J. , Hock , N. , Weimer , S. , Borrmann , S. , Kirchner , U. , Vogt , R. and Scheer , V. 2005 . Nucleation Particles in Diesel Exhaust: Composition Inferred From in Situ Mass Spectrometric Analysis . Environ. Sci. Technol. , 39 : 6153 – 6161 .
- Seinfeld , J. H. and Pandis , S. M. 1998 . Atmospheric Chemistry and Physics—from Air Pollution to Climate Change , New York : John Wiley & Sons .
- Shi , J. P. and Harrison , R. M. 1999 . Investigation of Ultrafine Particle Formation During Diesel Exhaust Dilution . Env. Sci. Technol. , 33 : 3730 – 3736 .
- Skillas , G. , Künzel , S. , Burtscher , H. , Baltensberger , U. and Siegmann , K. 1998 . High Fractal-Like Dimension of Diesel Soot Agglomerates . J. Aerosol Sci. , 29 : 411 – 419 .
- Taleb , D.-E. , Ponche , J.-L. and Mirabel , P. 1996 . Vapor Pressures in The Ternary System Water-Nitric Acid-Sulfuric Acid at Low Temperature: A Reexamination . J. Geophys. Res. , 101 : 25967 – 25977 .
- Tammet , H. and Kulmala , M. 2005 . Simulation Tool for Atmospheric Aerosol Nucleation Bursts . J. Aerosol Sci. , 36 : 173 – 196 .
- Tammet , H. 1995 . Size and Mobility of Nanometer Particles, Clusters and Ions . J. Aerosol Sci. , 26 : 459 – 475 .
- Tobias , H. J. , Beving , D. E. , Ziemann , P. J. , Sakurai , H. , Zuk , M. , McMurry , P. H. , Zarling , D. , Waytulonis , R. and Kittelson , D. B. 2001 . Chemical Analysis of Diesel Engine Nanoparticles Using a Nano-DMA/Thermal Desorption Particle Beam Mass Spectrometer . Environ. Sci. Technol. , 35 : 2233 – 2243 .
- Uhrner , U. , von Löwis , S. , Vehkamäki , H. , Wehner , B. , Bräsel , S. , Hermann , M. , Stratmann , F. , Kulmala , M. and Wiedensohler , A. 2008 . Dilution and Aerosol Dynamics Within a Diesel Car Exhaust Plume—CFD Simulations of On-road Measurement Conditions . Atmos. Env. , 4 : 7440 – 7461 .
- Vaaraslahti , K. , Virtanen , A. , Ristimäki , J. and Keskinen , J. 2004 . Effect of After-Treatment Systems on Size Distribution of Heavy Duty Diesel Exhaust Aerosol . SAE Tech. Pap. Ser. , 2004-01-1980
- Vaaraslahti , K. , Keskinen , J. , Giechaskiel , B. , Solla , A. , Murtonen , T. and Vesala , H. 2005 . Effect of Lubricant on the Formation of Heavy-Duty Diesel Exhaust Nanoparticles . Environ. Sci. Technol. , 39 : 8497 – 8504 .
- Vaaraslahti , K. 2006 . Diesel exhaust particles: effects of after-treatment, fuel and lubricant , Dissertation. Publication 622 Tampere : Tampere University of Technology .
- Vehkamäki , H. , Kulmala , M. , Lehtinen , K. E. J. and Noppel , M. 2003 . Modelling Binary Homogeneous Nucleation of Water-Sulfuric Acid Vapours: Parameterisation for High Temperature Emissions . Environ Sci. Technol. , 37 : 3392 – 3398 .
- Virtanen , A. , Ristimäki , J. , Marjamäki , M. , Vaaraslahti , K. , Keskinen , J. and Lappi , M. 2002 . Effective Density of Diesel Exhaust Particles as a Function of Size . SAE Tech. Pap. Ser , 2002-01-0056
- Virtanen , A. , Ristimäki , J. and Keskinen , J. 2004 . Method for Measuring Effective Density and Fractal Dimension of Aerosol Agglomerates . Aerosol Sci Tech. , 38 : 1 – 10 .
- Vogt , R. , Scheer , V. , Casati , R. and Benter , T. 2003 . On-Road Measurement of Particle Emission in the Exhaust Plume of a Diesel Passenger Car . Environ. Sci. Tech. , 37 ( 18 ) : 4070 – 4076 .
- Vouitsis , E. , Ntziachristos , L. and Samaras , Z. 2005 . Modelling of Diesel Exhaust Aerosol During Laboratory Sampling . Atmos. Env. , 39 : 1335 – 1345 .
- Wang , J. S. , Chan , T. L. , Cheung , C. S. , Leung , C. W. and Hung , W. T. 2006 . Three-Dimensional Pollutant Concentration Dispersion of a Vehicular Exhaust Plume in the Real Atmosphere . Atmos. Env. , 40 : 484 – 497 .
- Wang , S. C. and Flagan , R. C. 1990 . Scanning Electrical Mobility Spectrometer . Aerosol Sci. Technol. , 13 : 230 – 240 .
- Wiedensohler , A. , Orsini , D. , Covert , D. S. , Coffman , D. , Cantrell , W. , Havlicek , M. , Brechtel , F. J. , Russell , L. M. , Weber , R. J. , Gras , J. , Hudson , J. G. and Litchy , M. 1997 . Intercomparison Study of the Size-Dependent Counting Efficiency of 26 Condensation Particle Counters . Aerosol Sci. Tech. , 27 : 224 – 242 .
- Zhang , K. M. and Wexler , A. S. 2004 . Evolution of Particle Number Distribution Near Roadways—Part I: Analysis of Aerosol Dynamics and its Implications for Engine Emission Measurement . Atmos. Env. , 38 : 6643 – 6653 .