Abstract
Regional deposition and ventilation of particles by generation, lobe and lung during steady inhalation in a computed tomography (CT) based human airway model are investigated numerically. The airway model consists of a seven-generation human airway tree, with oral cavity, pharynx, and larynx. The turbulent flow in the upper respiratory tract is simulated by large-eddy simulation. The flow boundary conditions at the peripheral airways are derived from CT images at two lung volumes to produce physiologically realistic regional ventilation. Particles with diameters equal to or greater than 2.5 microns are selected for study because smaller particles tend to penetrate to the more distal parts of the lung. The current generational particle deposition efficiencies agree well with existing measurement data. Generational deposition efficiencies exhibit similar dependence on particle Stokes number regardless of generation, whereas deposition and ventilation efficiencies vary by lobe and lung, depending on airway morphology and airflow ventilation. In particular, regardless of particle size, the left lung receives a greater proportion of the particle bolus as compared to the right lung in spite of greater flow ventilation to the right lung. This observation is supported by the left-right lung asymmetry of particle ventilation observed in medical imaging. It is found that the particle-laden turbulent laryngeal jet flow, coupled with the unique geometrical features of the airway, causes a disproportionate amount of particles to enter the left lung.
1. INTRODUCTION
Particle deposition studies in the human airway tree are vitally important for characterizing hazards of exposure to particulate matter, developing effective drug treatment methods for chronic airway diseases, and assessing dosimetry for occupational hygiene (CitationLambert et al. 2009) and medicinal treatments. Due to asymmetry of the human airway tree and heterogeneity of ventilation, it is essential to understand regional differences in particle deposition in the lungs, such as lobar and left-right lung deposition differences. For example, CitationSubramaniam et al. (2003) studied lobar differences in particle deposition using a five-lobe symmetric lung geometry that was structurally different in each of the five lobes. They found that deposition in the lower lobes of the tracheobronchial (TB) tree was approximately twice that in the upper lobes. This ratio was similar between both the left and right lungs. For the TB tree, deposition fraction in the lower lobes was the same for both the left and right lobes. For the upper lobes, deposition fraction was the same between left and right lung as well. The right middle lobe had the lowest deposition fraction of the five lung lobes. Likewise, CitationBennett (1991) studied bolus inhalation using gamma camera imaging and observed a left-to-right (L/R) asymmetry in terms of particle distribution in the airways. For 2.0 μm sized particles inhaled at 90% total lung capacity (TLC), there was greater distribution of aerosol bolus to the left lung in spite of the larger size and greater ventilation of the right lung. CitationMöller et al. (2009) observed similar L/R asymmetry for inhalation of 100 mL boli of 100 nm diameter radiolabeled particles. They found an L/R deposition ratio of 1.85 +/– 0.30 for shallow aerosol bolus inhalation and 1.18 +/– 0.10 to 1.74 +/– 0.31 for Kr-gas inhalation. They attributed this asymmetry to non-uniform expansion at the end of inhalation between the left and right lungs. They hypothesized that the liver may restrict the right lung's expansion at the end of breath, whereas expansion of the left lung is less restricted.
A better understanding of non-uniformity of particle distribution in the lungs is of critical importance in terms of targeted drug delivery. The ability to predict and determine site-specific and lobar deposition efficiency in the airway tree can aid in the development of more efficient and cost effective pharmaceutical aerosols, as well as administration techniques. In addition, lung diseases preferentially develop in certain regions of the lung due to deposition and accumulation of toxins/irritants/carcinogens. For example, the sites of tumors occur more frequently in the upper lobes of the lung as studied in CitationParkash (1977) and CitationLulu and Lawson (1964).
Medical imaging is one of the methods for studying particle or bolus dispersion in the lungs, such as used in the studies of CitationBennett (1991) and CitationMöller et al. (2009). However, these methods may require that patients inhale a radioactive tracer gas, which can be harmful if overexposed. Alternative methods, such as those based on airway casts, mathematical dispersion models or computational fluid dynamic (CFD) techniques, to accurately represent bolus dispersion and deposition have also been adopted. Airway casts provide a realistic representation of the airway tree, which can be used experimentally to characterize particle deposition. Semi-empirical bolus dispersion models are used to examine particle penetration, but cannot resolve particle trajectories. CFD models are able to track particle trajectories, but require realistic airway geometric models and physiological boundary conditions for ventilation and are more computationally demanding than mathematical bolus dispersion models.
CitationZhou and Cheng (2005) used an airway cast made from a volunteer and an adult cadaver to analyze particle transport in the lungs. The model included an oral cavity, pharynx, larynx, trachea, and four generations of conducting airways. They were able to quantify particle deposition on an airway generation basis. CitationFresconi et al. (2003) studied particle deposition during expiratory flow in an experimental symmetric bifurcation model and found that secondary motions during expiration influence particle transport. CitationCheng et al. (1999) used an oral airway cast to quantify deposition in that region. They were able to create a best-fit correlation for oral airway deposition that has subsequently been used in some empirical models.
Mathematical models of bolus dispersion can be modified to reflect experimental trends where such data exists. CitationPark and Wexler (2007a) studied particle transport and deposition for a single breath using a semi-empirical model for the whole lung. They found significant mixing effects during expiration; however, these effects were minimal in the smaller airways due to rapid decrease in the Dean number, which represents viscous to centrifugal forces for flows in curved pipes or channels. It has been found that particle deposition increased with subsequent breaths, as the particles were able to penetrate further into the lung, CitationPark and Wexler (2007b). CitationPark and Wexler (2008) found that particle deposition was relatively low for particles in the 100 nm to 1 μm size range and it increased for particles smaller than 100 nm and larger than 1 μm; the bulk of particle deposition was in the conducting airways and pulmonary region, except for large particles in the 10-μm size range, in which case the extrathoracic region had more pronounced deposition.
CitationAsgharian et al. (2006) used a multiple-path mathematical deposition model to analyze particle losses in 30 stochastic asymmetric tracheobronchial tree structures, each supplemented with a seven-generation symmetric alveolar region. It was assumed that particles were mono-disperse and uniformly distributed; in addition, airflow in the lung airways was assumed to be proportional to the change in distal airway volume under uniform or non-uniform expansion. They found that overall particle deposition was greatest for particles smaller than 100 nm and greater than 1 μm. In addition, they found little difference in deposition between uniform and non-uniform lung expansion while breathing at rest. Despite the usefulness of mathematical models, they typically do not yield information on particle trajectories and hence cannot identify “hot spots” within the lung geometry, where harmful/toxic particulate matter may accrue and be absorbed in and transported by the lung mucous, possibly leading to ill health effects.
CFD models drastically reduce the cost and time that are associated with experimental models and have the ability to retain the realistic features of the airway tree as well as identify regions of high deposition rates. Different airway geometries have been adopted for CFD studies, ranging from symmetric cylindrical models to realistic Computed-Tomography (CT) based asymmetric geometries. CitationLongest and Vinchurkar (2007) studied respiratory aerosol deposition in a symmetric Weibel A model for the third through fifth generations (G3-G5) using laminar, standard Reynolds-Averaged Navier-Stokes (RANS) k-ω and low Reynolds number (LRN) k-ω CFD models for steady inspiratory flow. They found that an accurate representation of transitional and turbulent flows has a significant effect on particle deposition patterns. For a review of particle transport in idealized symmetric models using laminar or RANS models, please refer to CitationKleinstreuer and Zhang (2010). Particle deposition in three-dimensional (3D) oropharyngeal, 3D tracheobronchial, and two-dimensional (2D) alveolar models have shown that deposition is more uniform for nano-particles than for micron sized particles, CitationFarkas et al. (2006). In addition, extrathoracic and tracheobronchial deposition fractions of nano-particles decrease with increasing flow rate, while the opposite is true for micro-particles. CitationRusso et al. (2008) studied the effects of cartilage rings on particle deposition in a symmetrical single bifurcation model that included generations G0 and G1. They found that the tracheal cartilage rings increased deposition as compared with a smooth-walled trachea model.
CitationFarkas and Balashazy (2008) studied particle deposition in an asymmetrical five-generation tracheobronchial model and found localized deposition patterns for all particle sizes, but they were most pronounced for larger particles. They also found that deposition density in “hot spots” may be hundreds or thousands of times greater than average deposition density. CitationNowak et al. (2003) found significant differences in deposition between the Weibel A and CT based lung models, which were attributed to the different geometries. They concluded that in most cases, the Weibel A model is not adequate for prediction of particle deposition patterns. CitationZhang et al. (2002) studied micron-particle deposition in a human oral airway model based on a human cast and concluded that turbulence after oral airway constriction could increase particle deposition in the laryngeal region in the trachea. CitationMatida and Finlay (2002) employed a RANS model to examine airflow in an idealized mouth-throat geometry, which they in turn used to study particle deposition. Near-wall corrections that assumed anisotropic turbulence in the near-wall zones were utilized, without which the predicted particle deposition did not agree well with experimental data. CitationLongest et al. (2008) found that models conserving the irregular geometry of the mouth-throat were most useful for deposition prediction. CitationLi et al. (2007) studied particle deposition for transient inhalation waveforms, concluding that deposition sites are dependent on geometry and flow rate magnitude. CitationXi and Longest (2008) studied submicrometer particle transport for transient inhalation and found that using a drift flux model produced the best results when compared with experiment. CitationMa and Lutchen (2008) performed a CFD study on an airway model derived from a CT-based one-dimensional centerline airway tree using the k-ϵ turbulence model. They found that particle deposition increased with increasing particle size and that overall tracheobronchial deposition is dominated by the central airways for micrometer sized aerosol particles. CitationXi et al. (2008) studied the effects of the laryngeal jet on micro- and nano-particle transport in the upper tracheobronchial airways; they found that inclusion of the laryngeal jet has significant effects on particle deposition. CitationJayaraju et al. (2008) compared the RANS k-ω, detached eddy simulation, and large-eddy simulation in an idealized mouth-throat model. They found that the flow fields computed by the latter two models agreed well with the measurements of particle image velocimetry, and improved prediction of particle deposition considerably, in particular for particles of size below 5 μm.
Although there have been many studies utilizing CFD methods, there are three major challenges that need to be overcome to acquire data that is sensitive to lung structure (geometry) and function (flow) for addressing non-uniformity and asymmetry of particle deposition in the lungs. These challenges are the representation of realistic airway geometry, the imposition of physiological boundary conditions, and the treatment of turbulence (CitationLin et al. 2009; CitationTawhai et al. 2009). In this work, we employ a large-eddy simulation (LES) technique for accurate modeling of turbulence (CitationLin et al. 2007; CitationChoi et al. 2009, CitationChoi et al. 2010), adopt a CT-based airway model for accurate presentation of airway morphology (CitationHoffman et al. 2004), and impose image-based boundary conditions to each of the five lobes to produce physiological lobar ventilation (CitationLin et al. 2009; CitationYin et al. 2010). The goals of this study are twofold. The first goal is to quantitatively describe particle transport and deposition on steady inhalation in a realistic CT-based, seven-generation human airway tree, with oral cavity, pharynx and larynx, including determination of particle deposition and ventilation characteristics by generation, lobe and lung. Aerosols with diameter ⩾2.5 μm were selected for study because they tend to deposit in the upper respiratory tract, whereas smaller particles tend to transport to the more distal parts of the lung. The CFD-predicted data were validated against the experimental data of CitationZhou and Cheng (2005) and CitationChan and Lippmann (1980). The second goal is to examine whether the current approach is able to predict the L/R asymmetry of particle deposition that is observed in medical imaging and further explain the underlying physical mechanics for the asymmetry.
2. METHODS
2.1. Computational Fluid Dynamics Methods
The filtered continuity and Navier-Stokes equations for incompressible flow, shown in Equations (Equation1) and (Equation2) are solved using an in-house LES code, which explicitly resolves large-scale energy-containing turbulent eddies and parameterizes small-scale eddies with a sub-grid scale (SGS) model.
The governing equations are discretized, with second-order accuracy in both time and space, using an implicit characteristic-Galerkin approximation coupled with a fractional four-step algorithm (CitationLin et al. 2005). The continuity equation is enforced by solving the pressure-Poisson equation. The SGS model of CitationVreman (2004) was adopted for calculation of the eddy viscosity ν T (CitationLin et al. 2007; CitationChoi et al. 2009, CitationChoi et al. 2010). Performance of the current method including spatio-temporal convergence with a similar condition can be found in Choi et al. (2009, Choi et al. 2010).
2.2. Airway Model Geometry, CFD Mesh, and Boundary Conditions
The human airway geometry under study is shown in . It was reconstructed from CT images of a human lung measured at a lung volume of 85% TLC. The airway model consists of the extra-thoracic upper airways, i.e., the mouth cavity, the oropharynx and the larynx, and the intra-thoracic central airways of up to 7 generations from the zeroth generation (G0) of the trachea to the sixth generation (G6) of the airways. There are a total of 70 peripheral small airways.
FIG. 1 Realistic airway tree geometry acquired using a Siemens Sensation 64 multi-detector row computed tomography (MDCT) scanner housed at the Iowa Comprehensive Lung Imaging Center at the University of Iowa.
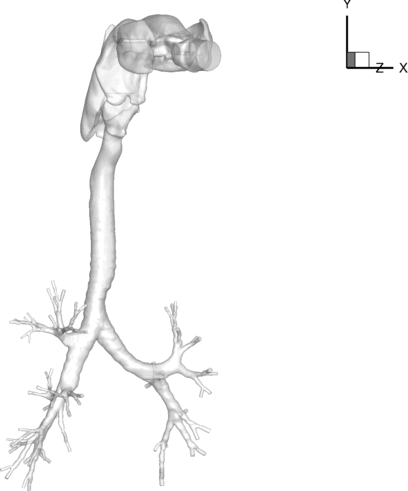
depicts the specific lung airways in the conducting tree. Airway segments are identified by name, along with an arrow for clarity. Airway generations are labeled as G0-G6, where G0 is the trachea and G6 is the sixth generation. Airway lobes are labeled as well, identified with a box around the text. The CT-lung geometry accounts for all five lung lobes: the left-upper lobe (LUL), left-lower lobe (LLL), right-upper lobe (RUL), right-middle lobe (RML), and right-lower lobe (RLL). The trachea, the left main bronchus (LMB), the right main bronchus (RMB), and the segment leading to three branches in the RUL (TriRUL) are also marked in the figure. For this geometry, the LMB has a much greater length as compared to the RMB, as well as a high degree of curvature. In terms of cross-sectional area, the LMB averages an area of 112 mm2 as compared to an area of 204 mm2 for the RMB. With the measured regional ventilation of the same subject, the air velocity in the LMB is approximately 1.78 times greater than that in the RMB. In addition, one can clearly see that the position of the aorta within the chest cavity induces curvature in the trachea near the first bifurcation. The dashed centerline in the figure shows that the majority of the trachea lies to the left of the carina of the first bifurcation.
The computational domain was sub-divided into 65 sub-volumes with volume boundaries denoted by solid lines. Two different mesh densities were constructed, referred to as original and refined mesh geometries. The original mesh geometry was comprised of 899,465 nodes and 4,644,447 tetrahedral elements. The refined mesh geometry consisted of 1,528,932 nodes and 8,063,559 tetrahedral elements. The relative differences (L1 norms) of the mean speed and the peak turbulent kinetic energy (TKE) in the jet core between the two meshes are less than 1% and 5%, respectively. All the results presented in the following sections are based on the refined mesh data unless otherwise noted. The sensitivity of particle deposition on mesh size will be discussed in section 3.2.
A steady parabolic inspiratory flow rate of 20 L/min was imposed at the mouth-piece inlet. To employ physiologically consistent boundary conditions at the peripheral airway segments, the air volumes in five lobes at two different lung volumes of the same subject were analyzed from CT images using the Pulmonary Analysis Software Suite (PASS) developed at the University of Iowa (CitationHoffman et al. 2004). The differences between the air volumes at two lung volumes in each of the five lobes were used to determine the partition of air flowing into each of the five lobes, (CitationLin et al. 2009; CitationYin et al. 2010). The resulting ventilation is physiologically consistent, as the ventilation fraction per lobe is (LUL, LLL, RUL, RML, RLL) = (0.145, 0.349, 0.130, 0.052, 0.324) and the flow division between the left and right lung is 0.494 and 0.506, respectively. The ratio of airflow to the left lung over the right lung is 0.976, which is the exact value computed by PASS. All wall surfaces were rigid and utilized the no-slip condition.
The flow simulation was conducted on 512 CPU cores of the Lonestar and Ranger clusters at the Texas Advanced Computing Center (TACC). The simulation took about ∼86 h for 320,000 time iterations to produce 100 instantaneous volume data sets.
2.3. Lagrangian Particle Tracking Algorithm
Particle tracking was done as a post-processing step after obtaining the LES solution. Each particle's motion was individually computed. Brownian motion of the particles was not considered due to the size of the particles under study. The equation of motion for spherical particles (CitationNowak et al. 2003; CitationMaxey and Riley 1983) reads

A velocity-Verlet integration scheme (CitationVattulainen et al. 2002) was employed to integrate the above Lagrangian particle tracking Equation (Equation3). As previously mentioned, the particle tracking algorithm is a post-processing step which uses the 3D velocity fields computed by the LES. Particles are initialized as a cylindrical bolus that consists of 10,000 spherical particles located at the mouthpiece inlet. A constant concentration of particles was used; however, particle velocity was initialized to be equal to fluid velocity plus a small random component. The oral region has a mouthpiece inlet attached to it; between the mouthpiece and the oral cavity the flow is constricted, causing a jet to form. Particles that enter the mouth essentially take on the profile of this jet instantaneously. The total simulation time of 2.16 s corresponds to a full inhalation cycle. A total of 46 LES data sets are used throughout the inhalation cycle, with each data set being 0.048 s apart (CitationLin et al. 2007). At each time step increment, the fluid velocity is interpolated between two of the LES data sets, whose time window contains the current time, in order to obtain an instantaneous flow field for the particle transport. The interpolation method used a second-order approach that utilizes fluid velocity at each of the four node points of the tetrahedral element containing the particle. Due to the transient and turbulent nature of the flow, eight different particle release times with an interval of 0.48 s were simulated. That is, with 150 instantaneous LES volume data sets, simulations for a given particle size were carried out beginning at the 21st, 31st, 41st, 51st, 61st, 71st, 81st, and 91st data sets, respectively, through the next 45 data sets. In the end, the results of these eight release times were used to calculate the mean and standard deviation (error bar). Deposition criteria for a particle are described below. If the shortest distance from the center of mass of the particle to the airway wall is less than the particle radius, it is considered deposited. The code also allows particles to exit the airway structure or remain active inside the domain. At the end of the inspiratory phase, the coordinates of all the particles are stored for inspection.
In the following section, Stokes number will be used in presenting deposition efficiencies for particles of various size and flow conditions in various airway generations. Stokes number is typically characterized as a ratio of the particle stopping distance to a characteristic dimension of an obstacle (CitationHinds 1999). Thus for large Stokes number, particles may deviate from fluid streamlines and impact on the obstacle surface, whereas for small Stokes number, particles tend to follow fluid streamlines. When applying the typical definition of Stokes number to an airway bifurcation, the Stokes number can be recast into (CitationFinlay 2001)
3. RESULTS
The flow characteristics are briefly described to facilitate understanding of particle transport in the human lungs. For a detailed analysis, please refer to CitationLin et al. (2007) and Choi et al. (2009, Choi et al. 2010), which studied the variability of flow fields between subjects.
shows the contours of mean speed and TKE of the flow in a vertical plane. The mean speed plot exhibits a high velocity jet in the oral region, which develops as air is inhaled through the mouth and travels through the narrow oral cavity. The turbulent laryngeal jet is formed at the glottis where the sudden constriction of the airway causes the airflow to rapidly accelerate. The TKE contours show that significant turbulence is produced in the oral cavity and the trachea; the more severe of the two is seen in the tracheal region, downstream of the glottal constriction, in association with the turbulent laryngeal jet.
3.1. Overall Deposition Efficiency
depicts the deposition locations for 2.5, 10, and 30-μm particles at the end of the inspiratory period (T = 2.16 s), i.e., at the normalized time t*(≡t/T) = 1. Deposition for 2.5 μm particles is the most uniform, whereas 30 μm particles predominantly deposit in the oral region near the tongue and throat, and additionally experience high deposition at the laryngeal constriction, the carina, and the bottom wall of the LMB. For particles in the 10 μm range, deposition is more uniform than in the 30 μm case, but high concentrations persist at the larynx constriction and bifurcation regions. Deposition at bifurcations is quite enhanced for particles with a diameter greater than 10 μm, due to impaction from inertial effects. These particles are characterized by large Stokes number and therefore cannot avoid obstacle surfaces, such as the curved airway wall and the bifurcation. These regions therefore constitute particle deposition “hot spots,” where harmful/toxic particulate matter could accumulate and be transported by the mucus layer lining the bronchial walls. As expected, 30-μm particles have the highest deposition rate, with approximately 99.7% of the particles depositing in the portion of the airway tree modeled here.
shows that deposition in the mouth-trachea region is enhanced for these larger particles, especially on the tongue and back of the mouth due to the irregular geometry and curvature of the oral cavity near the pharyngeal region. Particles with high Stokes number deviate from the fluid streamlines and impact at the back of the mouth and at the bifurcation region between the larynx and the esophagus.
FIG. 5 Oral airway deposition patterns for 2.5, 10, and 30-μm particles with respective deposition efficiencies of 3.9%, 9.5%, and 75.0% (left, middle, right).
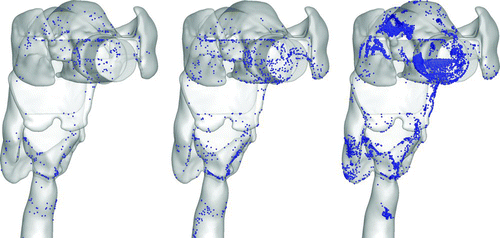
The high curvature from the oral cavity through the pharynx and larynx acts as a filter for the larger particles. This effect could be beneficial or harmful, depending on the toxicology of the particles inhaled. For coarse particulate matters, such as dust and soot, this filtering effect plays a positive role. Drug delivery methods to the lung typically consider particles of the 1–5 μm range (CitationFinlay 2001), which have sufficiently small Stokes number such that wasted drug depositions on the roof and back of the oral cavity are avoided. For the current model, the 2.5 and 5-μm particles have very low oral cavity deposition, with over 95% of particles making their way to the trachea for transport into the lung.
3.2. Grid Sensitivity Study
Figures and examine the effect of grid size on overall and oral particle deposition efficiencies. Overall particle deposition is a measure of the percentage of particles deposited in the entire CT airway tree, whereas oral particle deposition is the percentage of particles deposition in the mouth, pharynx, and larynx regions.
FIG. 6 Overall deposition efficiency vs. (a) particle size and (b) particle Stokes number for original and refined meshes.
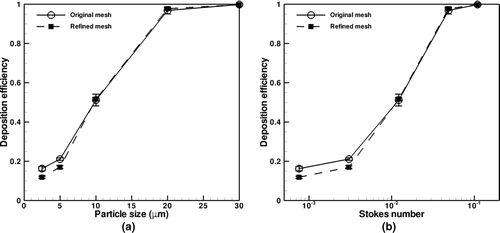
FIG. 7 Oral deposition efficiency vs. (a) particle size and (b) particle Stokes number for original and refined meshes.
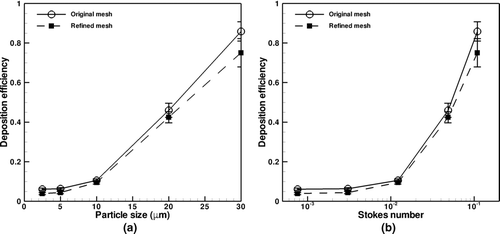
shows overall particle deposition as both a function of particle size (a) and particle Stokes number (b). Particle size is a typical variable used to characterize deposition. Particle Stokes number is used for analysis because for aerosols in which sedimentation and inertial impaction are the dominant deposition mechanisms, inertial impaction can be characterized as solely a function of Stokes number (CitationFinlay 2001). Particle Stokes number in Figures and is calculated using the mean velocity and the diameter of the mouth piece. The plots show good agreement between the original and refined meshes for particle deposition. The refined mesh predicts slightly lower deposition for 2.5 and 5-μm particles as compared to the original mesh, which is most likely due to more accurate interpolation of fluid velocity and subsequent calculation of the drag force. For all cases considered the deposition efficiency increases with increasing particle size and particle Stokes number.
For larger particles, deposition in the oral airways was enhanced due to increased inertial effects on particle trajectory. The sharp curvature of the oral airways filters larger particles out of the airstream, such that they are not able to deposit in large quantities further into the lung.
As shows, the refined mesh model predicts that for coarse particles of size 30 μm or greater, approximately 75% or more of the particles deposit in the oral region, which is less than the value of 100% reported by CitationMa and Lutchen (2008) using a RANS turbulence model. This may be due to either the use of LES, which resolves energy-containing turbulent eddies in the oral region whereas RANS only resolves mean flow, or geometric variability in the oral region, which requires further study. The deposition in the refined mesh is slightly less than the original mesh case, with the difference between them increasing with particle size and Stokes number. Despite this, the error bars for the two mesh data sets overlap, indicating consistency between the results. It is noted that the error bar for a given particle size is the standard deviation of the efficiencies for the eight particle release times to account for the effect of turbulence.
The oral deposition results are also compared to experimental results of CitationGrigic et al. (2004), as shown in . The agreement between the simulation results for oral deposition and the curve fit determined through oral airway cast experimentation is quite good, although there is slight deviation from the experimental curve at the lower end of the StkRe0.37 range. This difference was similarly found for CitationLongest et al. (2008), which used CFD to study particle deposition in a 90° bend and a mouth-throat geometry.
FIG. 8 Comparison of oral deposition results to CitationGrigic et al. (2004).
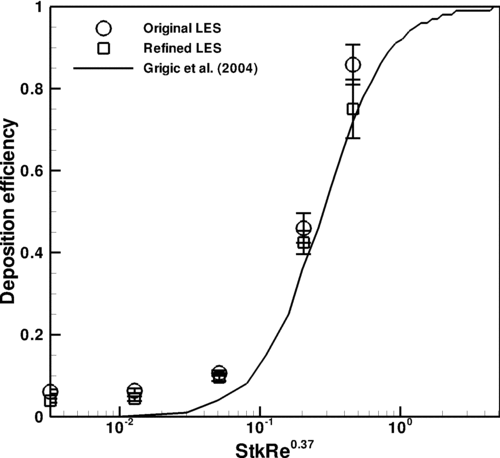
3.3. Generational Deposition Efficiency
Deposition efficiency in the first through fourth generations of the CT-based airway tree is explored for comparison with experimental data obtained by CitationZhou and Cheng (2005), who experimentally studied particle deposition for a steady flow condition in a four-generation airway replica made from an adult cadaver. Their airway replica included an oral cavity, pharynx, larynx, trachea, and four generations of bronchi. In their experiment, they removed deposited fluorescent particles from the cast and quantified deposition using a fluorescent spectrometer. In addition, the results are also compared with those of CitationChan and Lippmann (1980), who studied particle deposition in a hollow cast of the human larynx-tracheobronchial tree from the first to the sixth generations. The generational efficiency is defined as the number of deposited particles divided by the number of particles that entered the airway branch.
Figures – depict the averaged generational deposition efficiency in the first through fourth generations, respectively, for particles in the 2.5–30 μm range. The error bars depict the standard deviation of the results, the variability of which is due to transient effects. The first generation consisted of one bifurcation and its two daughter branches, the right and left main bronchus. The second generation consisted of two bifurcation regions and their subsequent daughter branches. Three third generation and five fourth generation bifurcating regions were selected for analysis. Regardless of generation, the deposition efficiency exhibits a similar dependence on the Stokes number. Overall, the generational deposition efficiencies for these generations agree well with the experimental data of both studies, thus validating the current simulation and modeling of particle transport and deposition. Although there is some deviation between the results at the smaller Stokes range in the third generation shown in , this difference is actually quite low and is visually magnified through the use of the log-scale on the y-axis. Also, from the experimental point of view, there is little difference between ∼1% and ∼0.1%. Additional simulations that included Brownian motion and increased numbers of particles yielded the same results. The results are also in agreement with the experimental data of CitationChan and Lippmann (1980), especially in the range of smaller Stokes number. It is noted that CitationZhang and Finlay (2005) observed in their experimental studies that particle deposition increases with increasing Reynolds numbers of 2276, 4553, and 6830. However, the dependence of particle deposition on Reynolds number at different flow rates is not evident from the experimental data of CitationZhou and Cheng (2005) shown in . It is perhaps due to the lower Reynolds number of the flow.
FIG. 10 Deposition efficiency in the second generation. Different symbols in the current LES denote different bifurcations.
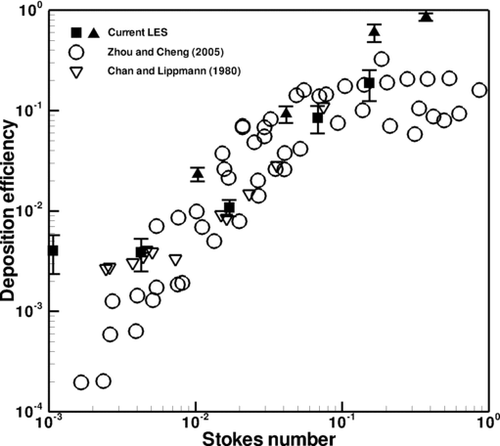
3.4. Lobar Particle Ventilation and Deposition
Particle ventilation and deposition amongst the five lung lobes cannot be assumed to be uniform due to geometrical asymmetry. Particle deposition is defined as any particles that deposit on the airway wall surfaces of the CT-geometry, whereas particle ventilation counts the number of deposited particles as well as the number of particles that are advected through the CT-geometry outlets. For lobar particle deposition and ventilation considered here, the reported fractions include all particles that deposit or ventilate to the lobar regions. This is investigated in , which shows that particle ventilation amongst the lobes for the 2.5 to 30 μm particle size range.
The left lower lobe (LLL) and right lower lobe (RLL) have very similar particle ventilation and overall receive the greatest particle fraction. The right middle lobe (RML) consistently receives the lowest fraction of particles that enter the tracheobronchial airways. The right upper lobe (RUL) receives similar particle ventilation as the left upper lobe (LUL) except for 30 μm particles, which is most likely due to the filtering effect of the long curved LMB on large particles. In terms of particle deposition in the lobar bronchi, both LLL and the RLL have the greatest fraction of deposited particles, with the left being greater than the right except for larger particles. This difference is due to the fact that approximately 80% of the 30-μm particles that enter the left lung deposit along the bottom wall of the LMB, thus never make it to the lobar bronchi. As expected, deposition in the RML is minimal for all particle sizes because the RML received a small fraction of the inspired air.
summarizes the lobar depositions for 2.5 and 5.0-μm particles, which compare well qualitatively with lobar deposition data for 4.0-μm particles from CitationSubramaniam et al. (2003). In the current data and their cases, deposition in the upper lobes is similar between the right and left lungs, and lowest in the middle lobe. However, there is a difference in predicted lower-lobe deposition, with a bias towards greater deposition in the LLL as compared with the RLL that is not present in CitationSubramaniam et al. (2003).
TABLE 1 Lobar deposition for 2.5 and 5.0-μm particles compared against 4.0-μm particles from CitationSubramaniam et al. (2003)
In addition, deposition between the upper and lower lobes is examined in , showing that the lower lobes of the lung have a greater number of deposited particles. This is most likely due to the fact that the lower lobes receive a greater fraction of the inspired air volume.
TABLE 2 Deposition ratio between upper (U) to lower lobes (L) U/L, for the right and left lungs (note that the RML is not included in the calculation; +/– denotes standard deviation)
3.5. Asymmetry between Left and Right Lung Particle Transport
The left lung receives a greater proportion of the particle bolus as compared to the right lung, as shown in , despite the fact that the right lung has slightly greater ventilation as discussed in section 2.2. The ratio of ventilated and deposited particles in the left lung to the right lung is plotted against tracheal and glottal Stokes number as shown in . For the glottal Stokes number (Stkglottis), the maximum air speed through the constriction was used, as well as the hydraulic diameter, which is defined as Dh= 4A/P, where A is the area of the constriction and P is the wetted perimeter. For particle ventilation, the L/R ranges from approximately 1.16 to 1.56, while for particle deposition, the L/R ranges from approximately 1.01 to 1.60, which is summarized in . This left to right asymmetry is most pronounced for the larger particles and decreases as particle size decreases. The L/R particle ventilation ratio decreases with decreasing Stokes number, but reaches an asymptote for particle size of less than 10 μm.
TABLE 3 Left lung (L) over right lung (R) ratio for 2.5, 5, 10, 20, and 30-μm particles, +/– denotes standard deviation
shows a snapshot of particle distribution for 2.5-μm particles with Stkglottis≪ 1, whereas shows that for 20 μm particles with Stkglottis∼ 0.4. The 2.5-μm particles are much more evenly dispersed than the 20-μm particles. This is due to the low Stokes number for 2.5-μm particles, as local turbulent vortices can influence particle motion and enhance dispersion. For 20-μm particles, due to the dominance of inertial forces the particles are less dispersed, congregating near the core of the jet, and are overall less uniform in distribution.
FIG. 16 Particle transport profiles at normalized time t* = 0.13. (a) 2.5-μm particles with Stkglottis= 0.006, (b) 20-μm particles with Stkglottis= 0.405. The unit of air speed is m/s.
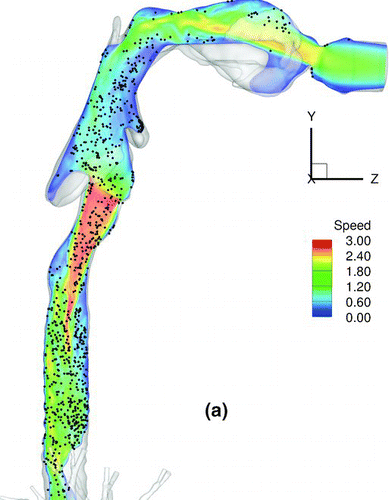
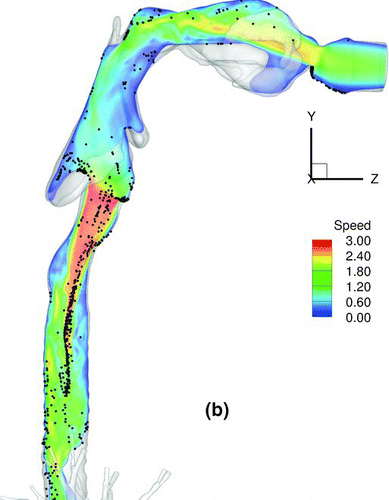
displays the iso-surfaces of the air speed in the trachea and subsequent airways. As seen in the plot, a significant portion of high-speed flow from the trachea is diverted into the LMB because the cross sectional area of the LMB is much smaller than that of the RMB. The particle transport profile overlaps very well with the velocity iso-surfaces that persist from the trachea into the LMB, suggesting that the continuation of the high-speed core of the jet from the glottis to the LMB may have a direct effect on particle bolus dispersion.
4. DISCUSSION
The results shown here for particle deposition locations in the upper airways and at airway bifurcations are expected considering the particle size. These deposition sites are prime targets for impaction of inhaled aerosols as particle inertia causes deviation from fluid streamlines. Identification of these deposition “hot spots” can be useful for targeted drug delivery, or assessing the dangers of ambient or occupational exposure to toxic particulate matter. In this study, deposition in the first through the fourth generations of the airway tree compared well quantitatively with experimental data, which showed that with increasing Stokes number the deposition efficiency of particles entering the first through fourth generations of the lobar bronchi increased. Overall, these results are encouraging because they show that accurate numerical simulation can provide good prediction for aerosol deposition in the conducting airways, which has implications in terms of targeted drug delivery. This is an important conclusion, as the use of imaging techniques or airways casts for particle tracking can be expensive and time consuming, especially if one wants to examine the effects of different parameters such as particle size, inhalation waveform, or precise geometrical features.
One benefit of utilizing CT-based geometry is the inclusion of each of the five lung lobes. Previously, many CFD studies have used simplified, symmetric lung geometries that do not account for the fact that the lung has five distinct lobes, thus these simplified models cannot speculate on the relationship between particle transport and lobar ventilation. The current study shows that particle deposition and ventilation is greatest in the lower lobes of the lung. The results also qualitatively compared well to those published in CitationSubramaniam et al. (2003), which showed that deposition favored the lower lobes. The upper lobes receive less flow and hence fewer particles.
Perhaps the most intriguing observation of this work is that the left lung receives a greater proportion of the particle bolus as compared to the right lung in spite of greater flow ventilation to the right lung. This observation is consistent with the experimental studies of Bennett et al. (1991, 1998, 1999) and CitationMöller et al. (2009). Bennett observed left to right deposition asymmetry during shallow bolus inhalation, concluding that there was more deposition activity in the left lung than the right lung for inhaled boluses at 90% TLC, and that the asymmetry increases with increasing lung volume. CitationMöller et al. (2009) used gamma camera imaging to track the inhalation of 100 mL boli of 100 nm diameter radiolabeled particles. They reported an L/R ratio of 1.69 for shallow boli inhalation, which they hypothesized could be due to greater expansion of the left lung as compared to the right lung during inhalation, as the right lung is constrained by the rigid liver. Nevertheless, the current results show that even with slight greater flow ventilation to the right lung, more particles deviate to the left lung, resulting in an L/R asymmetry for aerosol deposition. To shed light on the asymmetric particle ventilation in this study, we shall examine the geometric features of the human airway as well as the characteristics of particle-laden turbulent flow.
The human airway exhibited in Figures and has three distinct features: the glottal constriction, the smaller cross sectional area of the LMB than that of the RMB, and the location of the carina with respect to the glottis and the upper trachea. The constrictions at the glottis and the LMB lead to high-speed flows in the trachea and the LMB. As the ventilation ratio between the left and right lungs is 0.98, the persistence of higher velocity in the trachea into the LMB as opposed to the RMB becomes clear. Particles are concentrated in the core region of the laryngeal jet when passing through the glottis before entering the trachea. While being advected downstream along the trachea, they are dispersed laterally to the peripheral region of the jet and mixed with the ambient resident air. The high TKE region surrounding the high-speed jet in is associated with this entrainment and mixing process. The degree of dispersion depends on particle Stokes number. For example, shows that particles with large Stokes number are less uniformly distributed than those of small Stokes number, resulting in more asymmetry as shown in . With glottal Stokes number on the order of 0.1 or less, particles act like fluid tracers, yielding similar dispersion and distribution, and subsequently similar L/R asymmetry. Furthermore, if one follows the dashed line through the carina in , a significant portion of the trachea is to the left of the carina due to the position of the aorta. With increasing Stokes number, large particles become less sensitive to flow disturbances. Thus, particles initially concentrated in the core region of the laryngeal jet on the right-hand side of the dashed line in (i.e., to the left of the carina) tend to remain on the same side, contributing to the asymmetry. Particle dispersion in simple free shear turbulent flows has been investigated extensively for many engineering applications, such as particle-laden flows in a round jet (CitationLongmire and Eaton 1992), a plane mixing layer (CitationYang et al. 2000), a backward-facing step (CitationWang et al. 2006), a square jet (CitationLuo et al. 2006), amongst others. All of these studies corroborated the notion proposed by Crowe et al. (1985 1988) that the Stokes number, which is the ratio of the particle aerodynamic response time over the transit time for large turbulent eddies, plays a key role for particle dispersion in large scale flow structures. With Stk ≪ 1 particles essentially follow the fluid motion. With Stk ∼ 1 particles concentrate largely in the outer fringes of large scale eddies. And with Stk ≫ 1 particles are insensitive to the fluid velocity fluctuations. In spite of the complexity of the airway geometry and flow structure in the current study, the particle-laden laryngeal jet, coupled with the geometrical features of the trachea, causes a disproportionate amount of particles to enter the left lung as compared to the right. The extent of asymmetry depends on the Stokes number as well as the distribution of particles at the glottis. CitationLin et al. (2007) and CitationChoi et al. (2009) found Taylor-Görtler-like or Dean-like vortices at the glottis in human subjects of differing glottal constrictions and tracheal shapes. These vortices may rotate in clockwise or counter-clockwise direction with different characteristic time scales. Therefore, different particle release times at the mouth inlet may lead to association of particles with different states of vortices at the glottis, yielding various degrees of asymmetry in particle ventilation. This effect has been quantified with the standard deviation in calculated from the data of eight release times.
The favorable transport of the particle bolus into the left lung is an important conclusion, as it shows that bolus distribution is not uniform to both lungs, which can have implication in terms of assessing drug dosimetry or exposure to particulate hazards. This also implies that without the use of physiologically realistic airway geometry and an LES method, which accounts for turbulent fluctuations, this effect may be lost. Idealized models will not capture the specific geometry of the lung, nor its effects on airflow. Also, the RANS turbulence models tend to average out turbulent effects, thus they may not capture the oscillating behavior of the laryngeal jet and its subsequent effect on the bolus transport profile. The current results are encouraging because they show that the CFD solution using the CT model geometry gives accurate prediction of deposition efficiency in the airway tree, as evidenced by the good agreement with the experimental results of CitationZhou and Cheng (2005) and CitationChan and Lippmann (1980), as well as good agreement with experimental results for particle transport asymmetry as studied by Bennett (1991, 1998, 1999) and CitationMöller et al. (2009). This indicates that CFD modeling of pulmonary flows is a viable tool for assessing particle transport and deposition in the human airway tree. In addition, the use of the CT-model geometry provides many benefits over the more commonly used simplified and symmetric models, such as the ability to quantify lobar deposition and study asymmetries in the distribution of particles. The use of image-based boundary conditions for the airway tree is unique to this study and further enhances the quality of the solution, ensuring physiologically accurate ventilation.
5. CONCLUSIONS AND RECOMMENDATIONS
This study has demonstrated the use of an in-house Lagrangian particle tracking code for determining particle trajectories for aerosols in a realistic CT-based human airway tree, including the mouth-throat cavity. A large-eddy simulation technique was used to simulate the flow fields within the airway tree with a high degree of accuracy. The particle deposition results are in agreement with the experimental results of CitationZhou and Cheng (2005) and CitationChan and Lippmann (1980). In addition, the asymmetry in particle deposition and ventilation between the left and right lungs was supported by those found in experimental studies of Bennett et al. (1991, 1998, 1999) and CitationMöller et al. (2009). The quality agreement between the current numerical results and experimental studies shows the accuracy and robustness of the LES code coupled with the realistic CT geometry.
Particle deposition was enhanced in regions of bifurcation or high curvature due to inertial effects. Deposition increased with increasing particle size and hence Stokes number. For the largest particles, the filtering effects of the oral cavity were very pronounced, leading to low particle clearance into the lower regions of the lung. For the smaller particles, filtering effects were not significant and a large number of particles make their way into the conducting airways, as well as the deeper regions of the lung. There was a clear asymmetry between the distribution of particles to the left and right lung, which seems to be the result of airway geometry as well as the interaction between particles and free-shear jet flow which is characterized by the Stokes number.
Future studies could study the effect of breathing motions on particle trajectories. This would require transient simulations that accurately represent airway wall movement during inhalation. The modeling of lung movement could be achieved by image registration (CitationLin et al. 2009; CitationTawhai et al. 2009; CitationYin et al. 2009a, Yin et al. 2b; Yin et al. 2010). In addition, the effect of the surfactant layer of mucus lining the airway walls is not studied, which can have an important effect on particle retention and clearance (CitationMöller et al. 2004). Extension of the airway tree to the smaller generations is another area of interest; however this is difficult due to limits on scanning resolution, however methodologies to model the small airways explicitly have been explored in CitationLin et al. (2009). In addition, particle deposition in a detailed alveolar model (CitationKumar et al. 2009) would yield interesting results as well.
Acknowledgments
This work was supported in part by NIH-R01-EB-005823, NIH-R01-HL-064368, NIH-R01-HL094315, NIH-S10-RR-022421, a University of Iowa CTSA NIH/NCRR grant 1UL1RR024979 and a NIEHS/NIH Center Grant P30 ES05605. The authors would also like to thank Jiwoong Choi, Haribalan Kumar, and Youbing Yin for assistance during the course of the study, and thank Drs. Yue Zhou and Yung-Sung Cheng at the Lovelace Respiratory Research Institute for providing their experimental data (CitationZhou and Cheng 2005). The computer time was supported by TeraGrid TACC.
REFERENCES
- Asgharian , B. , Price , O. T. and Hofmann , W. 2006 . Prediction of Particle Deposition in the Human Lung using Realistic Models of Lung Ventilation . J. Aerosol Sci. , 37 : 1209 – 1221 .
- Bennett , W. D. 1991 . Targeting Respiratory Drug Delivery with Aerosol Boluses . J. Aerosol Med. , 4 : 69 – 78 .
- Bennett , W. D. , Scheuch , G. , Zeman , K. L. , Brown , J. S. , Kim , C. , Heyder , J. and Stahlhofen , W. 1998 . Bronchial Airway Deposition and Retention of Particles in Inhaled Boluses: Effect of Anatomic Dead Space . J. Appl. Physiol. , 85 : 685 – 694 .
- Bennett , W. D. , Scheuch , G. , Zeman , K. L. , Brown , J. S. , Kim , C. , Heyder , J. and Stahlhofen , W. 1999 . Regional Deposition and Retention of Particles in Shallow, Inhaled Boluses: Effect of Lung Volume . J. Appl. Physiol. , 86 : 168 – 173 .
- Chan , T. and Lippmann , M. 1980 . Experimental Measurements and Empirical Modeling of the Regional Deposition of Inhaled Particles in Humans . Am. Ind. Hyg. Assoc. J. , 41 : 399 – 409 .
- Cheng , Y. S. , Zhou , Y. and Chen , B. T. 1999 . Particle Deposition in a Cast of Human Oral Airways . Aerosol Sci. Technol. , 31 : 286 – 300 .
- Choi , J. , Tawhai , M. H. , Hoffman , E. A. and Lin , C.-L . 2009 . On Intra- and Intersubject Variabilities of Airflow in the Human Lungs . Phys. Fluids , 21 : 101901
- Choi , J. , Xia , G. , Tawhai , M. H. , Hoffman , E. A. and Lin , C.-L. 2010 . Numerical Study of High Frequency Oscillatory Air Flow and Convective Mixing in a CT-Based Human Airway Model . Annals of Biomedical Engineering , DOI:10. 1007/s10439–010–0110–7
- Crowe , C. T. , Gore , R. A. and Troutt , T. R. 1985 . Particle Dispersion by Coherent Structures in Free Shear Flows . Particul. Sci. Technol. , 3 : 149 – 158 .
- Crowe , C. T. , Chung , T. N. and Troutt , T. R. 1988 . Particle Mixing in Free Shear Flows . Prog. Energy Combust. Sci. , 14 : 171 – 194 .
- Farkas , A. , Balashazy , I. and Szocs , K. 2006 . Characterization of Regional and Local Deposition of Inhaled Aerosol Drugs in the Respiratory System by Computational Fluid and Particle Dynamics Methods . J. Aerosol Med. , 19 : 329 – 343 .
- Farkas , A. and Balashazy , I. 2008 . Quantification of Particle Deposition in Asymmetrical Tracheobronchial Model Geometry . Computers in Biology and Medicine , 38 : 508 – 518 .
- Finlay , W. H. 2001 . The Mechanics of Inhaled Pharmaceutical Aerosols , New York : Academic Press .
- Fresconi , F. E. , Wexler , A. S. and Prasad , A. K. 2003 . Expiration Flow in a Symmetric Bifurcation . Exper. Fluids , 35 : 493 – 501 .
- Grigic , B. , Finlay , W. H. , Burnell , P. K. P. and Heenan , A. F. 2004 . In Vitro Intersubject and Intrasubject Deposition Measurements in Realistic Mouth-Throat Geometries . J. Aerosol Sci. , 35 : 1025 – 1040 .
- Hinds , W. C. 1999 . Aerosol Technology:Properties, Behavior, and Measurement of Airborne Particles , 07030 – 5774 . Hoboken, NJ : John Wiley & Sons .
- Hoffman , E. A. , Clough , A. V. , Christensen , G. E. , Lin , C.-L. , McLennan , G. , Reinhardt , J. M. , Simon , B. A. , Sonka , M. , Tawhai , M. H. , van Beek , E. J. R. and Wang , G. 2004 . The Comprehensive Imaging Based Analysis of the Lung: A Forum for Team Science . Acad. Radiol. , 11 : 1370 – 1380 .
- Jayaraju , S. T. , Brouns , M. , Lacor , C. , Belkassem , B. and Verbanck , S. 2008 . Large Eddy and Detached Eddy Simulations of Fluid Flow and Particle Deposition in a Human Mouth-Throat . J. Aerosol Sci. , 39 : 862 – 875 .
- Kleinstreuer , C. and Zhang , Z. 2010 . Airflow and Particle Transport in the Human Respiratory System . Ann. Rev. Fluid Mechan. , 42 : 301 – 334 .
- Kumar , H. , Lin , C.-L. , Tawhai , M. and Hoffman , E. 2009 . The Effects of Geometry on Airflow in the Acinar Region of the Human Lung . J. Biomechan. , 42 : 1635 – 1642 .
- Lambert , A. R. , Lin , C.-L. , Mardorf , E. and O'shaughnessy , P. 2009 . CFD Simulation of Contaminant Decay for High Reynolds Flow in a Controlled Environment . Ann. Occupat. Hyg. , 54 ( 1 ) : 88 – 99 .
- Li , Z. , Kleinstreuer , C. and Zhang , Z. 2007 . Particle Deposition in the Human Tracheobronchial Airways Due to Transient Inspiratory Flow Patterns . J. Aerosol Sci. , 38 : 624 – 644 .
- Lin , C.-L. , Lee , H. , Lee , T. and Weber , L. J. 2005 . A Level Set Characteristic Galerkin Finite Element Method for Free Surface Flows . Int. J. Numer. Methods Fluid. , 49 : 521 – 548 .
- Lin , C.-L. , Tawhai , M. H. , McLennon , G. and Horrman , E. A. 2007 . Characteristics of the Turbulent Laryngeal Jet and Its Effect on Airflow in the Human Intra-Thoracic Airways . Respir. Physiol. & Neurobiol. , 157 : 295 – 309 .
- Lin , C.-L. , Tawhai , M. H. , McLennon , G. and Hoffman , E. A. 2009 . Multiscale Simulation of Gas Flow in Subject Specific Models of the Human Lung . IEEE Engineering in Medicine and Biol. , 28 : 25 – 33 .
- Longest , P. W. , Hindle , M. , Choudhuri , S. D. and Xi , J. 2008 . Comparison of Ambient and Spray Aerosol Deposition in a Standard Induction port And More Realistic Mouth–Throat Geometry . J. Aerosol Sci. , 39 : 572 – 591 .
- Longest , P. W. and Vinchurkar , S. 2007 . Validating CFD Predictions of Respiratory Aerosol Deposition: Effects of Upstream Transition and Turbulence . J. Biomech. , 40 : 305 – 316 .
- Longmire , E. K. and Eaton , J. K. 1992 . Structure of a Particle-Laden Round Jet . J. Fluid Mech , 236 : 217 – 257 .
- Lulu , D. J. and Lawson , L. J. 1964 . Carcinoma of the Lung: A Review of 321 Cases . American Medical Association Arch. Surg , 88 ( 2 ) : 213 – 217 .
- Luo , K. , Klein , M. , Fan , J.-R. and Cen , K.-F . 2006 . Effects on Particle Dispersion by Turbulent Transition in a Jet . Physics Letters A , 357 : 345 – 350 .
- Ma , B. and Lutchen , K. R. 2008 . CFD Simulation of Aerosol Deposition in an Anatomically Based Human Large-Medium Airway Model . Ann. Biomed. Engineer. , 37 : 271 – 285 .
- Matida , E. A. , Finlay , W. H. , Lange , C. F. and Grgic , B. 2002 . Improved Numerical Simulation of Aerosol Deposition in an Idealized Mouth-Throat . J. Aerosol Sci. , 35 : 1 – 19 .
- Maxey , M. R. and Riley , J. J. 1983 . Equation of Motion for a Small Rigid Sphere in a Nonuniform Flow . Phys. Fluids , 26 : 883 – 889 .
- Möller , W. , Meyer , G. , Scheuch , G. , Kreyling , W. G. and Bennett , W. D. 2009 . Left-to-Right Asymmetry of Aerosol Deposition after Shallow Bolus Inhalation Depends on Lung Ventilation . J. Aerosol Med. and Pulmonary Drug Deliv. , 22 : 1 – 7 .
- Möller , W. , HäuBinger , K. , Winkler-Heil , R. , Stahlhofen , T. M. , Hofmann , W. and Heyder , J. 2004 . Mucociliary and Long-Term Particle Clearance in the Airways of Healthy Nonsmoker Subjects . J. Appl. Physiol. , 97 : 220 – 2206 .
- Morsi , S. A. and Alexander , A. J. 1972 . An Investigation of Particle Trajectories in Two-Phase Flow Systems . J. Fluid Mechanics , 55 : 193 – 208 .
- Nowak , N. , Kakade , P. P. and Annapragada , A. V. 2003 . Computational Fluid Dynamics Simulation of Airflow and Aerosol Deposition in Human Lungs . Ann. Biomed. Engineer. , 31 : 374 – 390 .
- Park , S. S. and Wexler , A. S. 2007a . Particle Deposition in the Pulmonary Region of the Human Lung: A Semi-Empirical Model of Single Breath Transport and Deposition . J. Aerosol Sci. , 38 : 228 – 245 .
- Park , S. S. and Wexler , A. S. 2007b . Particle Deposition in the Pulmonary Region of the Human Lung: Multiple Breath Aerosol Transport and Deposition . J. Aerosol Sci. , 38 : 509 – 519 .
- Park , S. S. and Wexler , A. S. 2008 . Size-Dependent Deposition of Particles in the Human Lung at Steady-State Breathing . J. Aerosol Sci. , 39 : 266 – 276 .
- Parkash , O. 1977 . Lung Cancer: A Statistical Study Based on Autopsy Data from 1928 to 1972 . Respiration , 34 : 295 – 304 .
- Russo , J. , Robinson , R. and Oldham , M. J. 2008 . Effects of Cartilage Rings on Airflow and Particle Deposition in the Trachea and Main Bronchi . Medical Engineer. & Phys. , 30 : 581 – 589 .
- Subramaniam , R. P. , Asgharian , B. , Freijer , J. I. , Miller , F. J. and Anjilvel , S. 2003 . Analysis of Lobar Differences in Particle Deposition in the Human Lung . Inhal. Technol. , 15 : 1 – 21 .
- Tawhai , M. H. , Hoffman , E. A. and Lin , C.-L . 2009 . The Lung Physiome: Merging Imaging-Based Measures with Predictive Computational Models of Structure and Function: Wiley Interdisciplinary Reviews . Sys. Biol. Med. , 1 : 61 – 72 .
- Vattulainen , I. , Karttunen , M. , Besold , G. and Polson , J. M. 2002 . Integrating Schemes For Dissipative Particle Dynamics Simulations: From Softly Interacting Systems Towards Hybrid Models . J. Chem. Phys. , 116 : 3967 – 3979 .
- Vreman , A. 2004 . An Eddy-Viscosity Subgrid-Scale Model for Turbulent Shear Flow: Algebraic Theory and Applications . Phys. Fluids , 16 ( 10 ) : 3670 – 3681 .
- Wang , B. , Zhang , H. Q. and Wang , X. L. 2006 . Large Eddy Simulation of Particle Response to Turbulence Along Its Trajectory in a Backward-Facing Step Turbulent Flow . Intl. J. Heat and Mass Trans. , 49 : 415 – 420 .
- Xi , J. and Longest , P. W. 2008 . Evaluation of a Drift Flux Model for Simulating Submicrometer Aerosol Dynamics in Human Upper Tracheobronchial Airways . Ann. Biomed. Engineer. , 36 ( 10 ) : 1714 – 1734 .
- Xi , J. , Longest , P. W. and Martonen , T. B. 2008 . Effects of the Laryngeal Jet on Nano- and Microparticle Transport and Deposition in an Approximate Model of the Upper Tracheobronchial Airways . J. Appl. Physiol. , 104 : 1761 – 1777 .
- Yang , X. , Thomas , N. H. and Guo , L. J. 2000 . Particle Dispersion in Organized Vortex Structures Within Turbulent Free Shear Flows . Chem. Engineer. Sci. , 55 : 1305 – 1324 .
- Yin , Y. , Hoffman , E. A. and Lin , C.-L. 2009a . “ Local Tissue-Weight-Based Nonrigid Registration of Lung Images With Application to Regional Ventilation ” . In SPIE Medical Imaging’09 , Edited by: Hu , X. P. and Clough , A. V. 72620C Bellingham, WA : SPIE . 7262
- Yin , Y. , Hoffman , E. A. and Lin , C.-L . 2009b . Mass Preserving Nonrigid Registration of CT Lung Images Using Cubic B-spline . Med. Phys. 36 , 9 : 4213 – 4222 .
- Yin , Y. , Choi , J. , Hoffman , E. A. , Tawhai , M. H. and Lin , C.-L. 2010 . Simulation of Pulmonary Air Flow with a Subject Specific Boundary Condition . J. Biomechan. , doi: doi:10.1016/j.jbiomech.2010.03.048
- Zhang , Z. and Finlay , W. H. 2005 . Experimental Measurements of Particle Deposition in Three Proximal Lung Bifurcations Models with an Idealized Mouth-Throat . J. Aerosol Med. , 18 : 460 – 475 .
- Zhang , Z. , Kleinstreuer , C. and Kim , C. S. 2002 . Micro-Particle Transport and Deposition in a Human Oral Airway Model . J. Aerosol Sci. , 33 : 1635 – 1652 .
- Zhou , Y. and Cheng , Y. S. 2005 . Particle Deposition in a Cast of Human Tracheobronchial Airways . Aerosol Sci. Technol. , 39 : 492 – 500 .