Abstract
In the present study, the interaction effects of different vehicle speeds and exhaust tailpipe exit velocity and temperature conditions on the three-dimensional flow structure, exhaust particle dynamic behavior, formation and evolution processes (i.e., nucleation, coagulation, condensation, and dispersion), number and volume concentration, and nucleation rate fields in the near-wake region behind the studied ground vehicle in urban road microenvironment were comprehensively simulated using large-eddy simulation (LES) with the aerosol dynamics and dispersion model based on our recently established direct quadrature method of moments (DQMOM) approach. The numerical results show that the particles are drawn up into the recirculation region and then moved toward the upper vortex by its lower vortex accordingly. The particle nucleation rate is much more associated with the distribution of the root mean square (RMS) temperature than with that of the temperature itself. Some of the sulfuric acid concentration emitted from the vehicular exhaust tailpipe are trapped by the vortex and then recirculated back to the rear end of the studied ground vehicle. As a result, the exhaust particle concentration in this region is much higher than a farther distance downstream and the nucleation rate is also enhanced in this region. The exhaust particle volume concentration is the highest in the recirculation region behind the studied ground vehicle and is diluted gradually beyond the recirculation region in the downstream.
INTRODUCTION
Since the particulate mass emissions from the diesel engines are typically ten to hundred times higher than those from spark ignition engines (CitationKittelson 1998), more recent studies have focused on the concentration of diesel exhaust particle emissions and the characteristics and dispersion of particles from a vehicular exhaust plume (CitationBessagnet and Rosset 2001; CitationKim et al. 2002; CitationWong et al. 2003; CitationJiang et al. 2005; CitationChan et al. 2006; CitationKittelson et al. 2006; CitationUhrner et al. 2007; CitationYin et al. 2007; CitationLiu et al. 2009; CitationYu et al. 2009; CitationChan et al. 2010a, Citation2010b). Over the past decades, the advancement of engine technology and improvement in fuel quality has contributed greatly to the steady decrease of the mass concentration of particles emitted from diesel vehicles. However, it is shown that the adverse health effects are more correlated with the particle number and size concentration than the particle mass concentration (CitationPope and Dockery 2006), and several studies have reported the inverse relationships between the size and health response of particulate matters, as the smaller the particles (i.e. nano to fine particles), the deeper it can penetrate into the human body via lungs (CitationDavidson et al. 2005). On the other hand, the nanoparticle number concentration is extremely high in the near-wake region behind the moving ground vehicle(s). This situation has a direct adverse impact on human health if people passing/working/living near busy roads/streets are exposed to such short-term or long-term dynamic peak levels of nano and ultrafine particle number concentrations particularly to in-cabin microenvironment for vehicle drivers and passengers, cyclists, pedestrians, people passing/working/living nearby traffic congested roads/roadsides, or waiting to use public transport (e.g., bus stops).
Consequently, a better understanding of the formation mechanism of vehicle exhaust nanoparticles is essentially required. These vehicle exhaust nanoparticles are formed from volatile precursor (i.e., sulfuric acid (H2SO4) and heavy hydrocarbons) and are then diluted in the atmosphere. However, accurate measurement of these vehicular exhaust particles is greatly affected by the sampling and dilution conditions. Small changes in the dilution process can vary the number concentration of nanoparticles formed by one or two orders of magnitude (CitationMathis et al. 2004). Kittelson and colleagues (CitationKittelson 1998; CitationKittelson et al. 2006) reported that the elapsed time needed to achieve the dilution ratio of 100 is a critical factor for the nanoparticle formation in diesel exhaust. The critical dilution ratio is usually achieved in the vehicle wake region within seconds under typical urban driving conditions. On the basis of the studies of CitationBaker (2001), the wake of a vehicle may be conveniently divided into two regions, namely, near-wake and far-wake region, respectively. In the near-wake region, the flow vortex and turbulence is very complex where the large-scale flow structures can remove the exhaust pollutants and the smaller turbulence scales can diffuse the exhaust pollutants in this region. While in the far-wake region, the pollutant behavior in atmosphere can usually be described by a Gaussian pollutant dispersion pattern. It has been clearly revealed that the wake flow structures of a ground vehicle have significant effects on the exhaust pollutant dispersion (CitationEskridge and Hunt 1979; CitationHider et al. 1997; CitationDong and Chan 2006; CitationKanda et al. 2006; CitationUhrner et al. 2007; CitationChan et al. 2008a, Citation2008b). Consequently, the vehicular exhaust turbulent mixing behavior in the near-wake region behind a moving vehicle is very important for investigating exhaust particles, and the eventual redistribution in the far-wake region is highly dependent upon the formation of particles in the near-wake region (CitationZhang and Wexler 2004).
Since the persistent recirculation and vortices in the near-wake region behind a ground vehicle make the initial vehicular pollutant dispersion no longer follow the Gaussian distribution closely, CitationHider et al. (1997) attempted to further develop the analysis of CitationEskridge and Hunt (1979) for investigating numerically the flow fields and particle dispersion in the wake of a single vehicle. CitationBessagnet and Rosset (2001) developed the general fractal aerosol model to simulate the particle evolution in a car exhaust plume during its dilution process in the ambient air. CitationKim et al. (2002) developed an Eulerian advection-diffusion equation coupled with the k-ϵ turbulence closure to predict the nucleation, coagulation, and dynamics of particulate matter in the exhaust plume from on-road heavy-duty diesel vehicle. CitationZhang and Wexler (2004) have investigated the evolution of aerosol number distributions near roadways in two distinct dilution processes (e.g., tailpipe-to-road and road-to-ambient) by using their developed aerosol dynamic models. They have clearly indicated that the dilution profile is mainly dependent on vehicle types and traffic conditions and may also affect the occurrence of nucleation. They have also suggested that further measurements and simulations of turbulent mixing behind a moving vehicle are required to elucidate this dilution process. CitationJiang et al. (2005) have also examined the effects of different flow streamlines in a vehicle exhaust plume, ambient aerosol particle size distributions (PSDs), and vehicle and wind speeds on the PSD in an exhaust plume using the one-dimensional aerosol dynamic model coupled with the standard k-ϵ turbulence closure. CitationPohjola et al. (2006) have recently analyzed the effects of different aerosol processes and simple plume dispersion on the properties of vehicular exhaust particles in a street environment. Recently, CitationKerminen et al. (2007) have investigated the development of particle number size distribution at 9 and 65 m distances from a major road in Helsinki, Finland, during an episodic inversion situation. CitationYin et al. (2007) has used the large-eddy simulation (LES) approach coupled with the nucleation and coagulation equations to study the flow structure and the formation of pollutant nanoparticles in a vehicular exhaust twin-jet plume of a moving car. CitationKeskinen and Ronkko (2010) have reviewed the studies comparing diesel exhaust PSD in real-world settings with partial dilution systems in laboratory.
However, the rate of nucleation and condensation as well as the dispersion of particles are highly dependent on the nature of flow structures (i.e., small- and large-scale fluctuations) that have to be fully coupled with the computational fluid dynamics (CFD) and aerosol model approaches. An attractive method is represented by the method of moments (MOM) (CitationMcGraw et al. 1995; CitationChan et al. 2006; CitationYu et al. 2008; CitationBae et al. 2009) where the PSD is tracked through its moments by integrating over the internal coordinate. The main advantage of MOM is that the number of scalars required is very small (i.e., usually 4–6), which makes its implementation in CFD codes more feasible. Moreover, it is found that the distributions with identical moments exhibit virtually identical physical and optimal properties and dynamics of aerosols (CitationMcGraw et al. 1998). However, this method has been scarcely applied due to the so-called closure problem (CitationDiemer and Olson 2002). By using an ad hoc quadrature formula to approximate the unclosed terms, CitationMcGraw (1997) developed the quadrature method of moments (QMOM) as an alternative method. One of the main limitations of QMOM is that the phase-average velocity of the different solid phases must be used to solve the transport equations for the moments. In order to address these issues, the direct quadrature method of moments (DQMOM) has recently been formulated and validated (CitationMarchisio and Fox 2005; CitationChan et al. 2010a). The DQMOM shows the advantage of being directly applicable to multivariate general dynamic equation (GDE) (i.e., GDE with more than one internal coordinate) and offers a powerful approach for describing polydisperse solids undergoing the segregation, growth, aggregation, and breakage processes in the context of CFD simulation. CitationUhrner et al. (2007) have recently performed the measurement of particle concentration emitted from a moving diesel vehicle. They have also developed a coupled CFD-aerosol model. Their model has taken into consideration the effects of dilution, nucleation, condensation, and coagulation on particle formation and growth in the exhaust plume of a diesel vehicle by combining a k-ϵ turbulence model with a fully coupled aerosol model based on the MOM approach in which the lognormal distribution of particle size is assumed as a precondition. However, the small-scale fluctuations are filtered by the average operation in Reynolds-averaged turbulent models (i.e., k-ϵ turbulence model) and may greatly affect the local nucleation and condensation rates of particles. It has been demonstrated that the effects of exhaust gas temperature and H2SO4 concentration variations on the new-particle formation rates are usually orders of magnitude greater than these variable themselves (CitationHousiadas et al. 2004) and turbulent fluctuations, determined experimentally, significantly reduce local maxima in the average nucleation rate of particles and broaden the region of particle formation in the shear layer (CitationLeniewski and Friedlander 1995). Consequently, the effects of small-scale fluctuations on the nucleation and condensation rate as well as the dispersion of particles in the wake region of a moving ground vehicle are also still unclear and should be further investigated through more detailed turbulent models. LES approach, in which much of the actual transport of momentum, mass, and energy are explicitly calculated while the effects of small eddies are modeled for a reasonable computational time and cost, has demonstrated to be an appealing method for providing a better understanding of the detailed dynamics of flow structures and unsteady pollutant dispersion process in the near-wake region of a moving ground vehicle (CitationYin et al. 2007; CitationChan et al. 2008b).
Recently, CitationChan et al. (2010a) have first investigated numerically the effects of the characteristics and behavior of the average diameter and the number and volume concentration of the exhaust particles in the wake of the studied ground vehicle for stationary (i.e., low idling mode) and lower vehicle speed (i.e., 10 and 30 km/h modes) conditions due to the nucleation, coagulation, and strong dilution processes by using LES with the aerosol dynamics and dispersion model based on the DQMOM approach. The results have shown that the processes of nucleation and coagulation are completed in a short distance away from the vehicular exhaust tailpipe exit of the studied ground vehicle. Furthermore, CitationChan et al. (2010b) have investigated the interaction effects of different sulfur contents, relative humidities, driving modes, and vehicular exhaust tailpipe exit conditions on the three-dimensional exhaust gas-to-nanoparticle conversion and concentration distribution in the wake region of a typical bus-shaped vehicle due to the nucleation and coagulation processes by using LES coupled with the aerosol dynamics and turbulent dispersion model. Among all the studied factors, sulfur content in diesel fuel affects the number concentration of nanoparticles significantly. A reduction of sulfur content from 500 ppm to 50 ppm will decrease the number concentration of nanoparticles more than one thousand times. However, no condensation process has been taken into consideration in their recent studies.
The aim of the present study is to perform the interaction effects of a wider range of vehicle speeds (i.e., 10, 30, 50, and 70 km/h) and exhaust tailpipe exit velocity and temperature conditions on the three-dimensional flow structure, exhaust particle dynamic behavior, formation and evolution processes (i.e., nucleation, coagulation, condensation, and dispersion), number and volume concentration fields, and nucleation rates in the near-wake region behind the studied ground vehicle were comprehensively simulated by using LES with the aerosol dynamics and dispersion model based on our recently established DQMOM approach.
NUMERICAL APPROACH
In the present study, the geometry and dimension of the studied ground vehicle were the same as used in our previously established work (CitationDong and Chan 2006; CitationChan et al. 2008b, Citation2010a) for validation purpose, and some of the boundary conditions used were from our previous experimental data in CitationChan et al. (2007). The computational domain is a rectangular box of 20 m × 6 m × 5 m in X, Y, and Z directions, respectively. The studied ground vehicle with a dimension of 4 m (L) × 2 m (W) × 1.8 m (H) is placed inside the computational domain at X = 4 m measuring from the upstream boundary (X = 0 m) as shown in . An undisturbed and uniform incoming flow profile can be obtained before reaching the frontal of the studied ground vehicle. The vehicular exhaust tailpipe exit (i.e., X = 8 m, Y = 0.525 m, and Z = 0.375 m) with a diameter of 0.05 m emitted the gaseous and particle pollutants into the real atmosphere and was located at the rear end of the studied ground vehicle, x (i.e., x/H = 0).
Large-Eddy Simulation
The three-dimensional LES computes the resolved-scale turbulent motions by directly solving a set of filtered Navier–Stokes equations and models the small subgrid-scale motions (CitationDong and Chan 2006; CitationChan et al. 2008b, Citation2010a, Citation2010b). By applying the volume-averaged operation to continuum equation and Navier–Stokes equations, the filtered equations can be expressed as follows:




The subgrid-scale stress, τij , represents the effect of the small scales that must be modeled, and the eddy-viscosity models are most commonly used subgrid model in LES (CitationSmagorinsky 1963):



The differential equation for the evolution of vapor concentrations neglecting the chemical reaction and taking into account the effects of nucleation and condensation are as follows:
DQMOM for General Dynamic Equation
The transport of the microscale or nanoscale particle dispersed in the fluid flow is governed by the aerosol GDE (CitationFriedlander 2000), which can be expressed as



The main idea behind GDE is the solution of the closure problem that is related to the evolution of the moments of the PSD by using a quadrature approximation of the order N. This corresponds to the approximation of the PSD as follows:
2N moments of the distribution can be obtained when the values of N weights and N abscissas of the quadrature approximation can be calculated by directly solving the transport equations of weight and abscissas [i.e., DQMOM which has recently been proposed by CitationMarchisio and Fox (2005)]. The general formula of the DQMOM can be expressed as follows:
In order to solve this linear system in Equation (9), the source term vectors of the moments need to be evaluated. The definition of the source term due to nucleation, coagulation, and condensation will be discussed as follows.
Nucleation Model
Nucleation is the formation of a new stable embryonic particle or droplet that is capable of growth from condensation. In order to determine the nucleation rate, it is necessary to convert the sulfur content in diesel fuel to the vapor pressure of H2SO4 in a dilution environment (CitationBaumgard and Johnson 1996; CitationKim et al. 2002). Typically, most of the sulfur content in diesel fuel transforms into sulfur dioxide (SO2) and a small part can be directly oxidized into sulfuric trioxide (SO3) when fuel combustion takes place inside the engine. Because of the abundance of water vapor in the exhaust gas, SO3 can be transformed into H2SO4 easily and then form new particles due to nucleation effect. In the present study, the sulfur content of the diesel fuel used was 500 ppm in mass basis with a H2SO4 conversion rate of 5%, which is the common condition after combustion in the engine (CitationBaumgard and Johnson 1996; CitationCasati et al. 2007). CitationVehkamaki et al. (2003) have recently presented the parameterized equations for calculating the critical nucleus compositions and homogeneous nucleation rates of sulfuric acid/water and providing high accuracy and computational efficiency in their results. These parameterized equations were also adopted in the present study. The temperature range of the parameterizations is from 300 K to 400 K; as a result, the nucleation rate of particles was neglected due to the small effect on the results of T > 400 K (CitationUhrner et al. 2007).
Coagulation Model
Coagulation is primarily caused by the Brownian motion of particles. Its effects can be written as follows:
Condensation Model
The condensation rate of i-vapor is given in the free molecule and continuum size regimes, respectively.
In the free molecular size regime, the aerosol growth law can be defined as follows (CitationPratsinis 1988):
In the continuum size regime, the aerosol rate can be defined as follows (CitationPratsinis 1988):
Initial and Boundary Conditions
The effects of four typical vehicle speed modes and exhaust tailpipe exit velocity and temperature conditions are listed in , based on our previous experimental data (CitationChan et al. 2007). In order to have a better understanding of the interaction effects of different vehicle speeds and exhaust tailpipe exit conditions on the three-dimensional flow structure, exhaust particle dynamic behavior, formation and evolution processes (i.e., nucleation, coagulation, condensation, and dispersion), and number concentration fields in the near-wake region behind the studied ground vehicle, the constant bimodal size distributions consisting of an emitted soot mode with d gn = 50 nm, N = 104/cm3, σ = 1.6 and a background accumulation mode with d gn = 120 nm, N = 2.5 × 103/cm3, σ = 1.8 used were exactly the same as used in CitationUhrner et al. (2007), where d gn, N, and σ are the geometric average diameter, number concentration of particles, and geometric standard deviation, respectively, for the present parametric study. The initial PSD is assumed to follow a lognormal distribution, so that the initial wi and vi in Equation (8) can be explicitly given. For the binary homogeneous nucleation in the present study, the ambient relative humidity used was 70%, which is a typcial local urban road condition, while the relative humidity is transported by the equivalent of Equation (5). The detailed effects of ambient relative humidity on the gas-to-nanoparticle conversion and concentration distribution can be referred to CitationChan et al. (2010b). It is worth noting that the present developed aerosol dynamics and dispersion model could further be used for future studies and new situations by using the summary of bimodal particle distributions emitted for different light duty Euro 1, 3, and 4 vehicles (CitationGiechaskiel et al. 2009).
TABLE 1 Initial exit conditions at the vehicular exhaust tailpipe of the studied ground vehicle (CitationChan et al. 2007)
Numerical Scheme
A typical and adequate grid size distribution that follows the shape of the studied ground vehicle body was used to discretize the computational domain that was similar and validated to our recently established work (CitationDong and Chan 2006; CitationChan et al. 2008b, Citation2010a). In the regions near the ground vehicle body surface and wall, a sufficient fine mesh resolution was used to capture the sharp gradients and boundary layers, and the near-wake region behind the studied ground vehicle to 3 m of distance downstream where the finest flow structure and turbulent mixing induced by the interaction between the vehicular exhaust jet plume and the vehicle wake is expected.
A control volume-based technique was used to convert the nonlinear governing equations to algebraic equations that can be solved numerically. The semi-implicit method for pressure-linked equations (SIMPLE) algorithm was applied for the pressure–velocity coupling. A segregated and implicit solver was used to solve the governing equations, and the second-order accuracy central differencing scheme was adopted to improve the accuracy of the solution. The commercial finite volume CFD code, FLUENT, was used to compute the velocity, temperature, and concentration fields, and the DQMOM was also implemented via the user-defined function. The volume fraction of particles was assumed to be small enough to have a negligible effect on the studied flow field in the present study.
RESULTS AND DISCUSSION
Ten character time scales were calculated before any numerical data were exported, where the character time scale is the time required for a fluid particle in the free stream to pass through the computational domain (CitationChan et al. 2008b). For an example, Case 1 in where the vehicle speed is taken at 10 km/h, the time step and scale used are Δt = 0.01 s and τ = 7.2 s, respectively, which corresponds to 7200 time steps being computed before the numerical data are time-averaged and then analyzed (CitationChan et al. 2008b). Typical time-averaged temperature distribution for Case 1 in the middle cross-sectional plane, ZX, with Y = 0.5 m (i.e., the centerline of the vehicular exhaust tailpipe), is shown in .
FIG. 2 Typical time-averaged temperature distribution of the studied ground vehicle in the cross-sectional plane, ZX, at Y = 0.5 m for Case 1 (i.e., 10 km/h). (Figure provided in color online.)
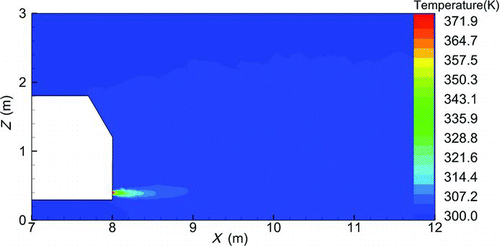
shows the time-averaged flow streamlines of the studied ground vehicle in the cross-sectional plane, ZX, at Y = 0 m. A two-tier contra-rotating vortex structure is clearly visible. The lower vortex rotating anticlockwise is more circular in shape and taller than its counterpart, while the upper vortex is longer extending farther downstream and rotating in an anticlockwise direction. The mean recirculation length estimated from is about 1.22H (i.e., X = 10.2 m or x = 2.2 m away from the rear end of the studied ground vehicle, x). However the mean recirculation length is observed to vary with distance from the centerline of the studied ground vehicle due to a change in the relative size of the internal vortex structure. shows the time-averaged flow streamlines of the studied ground vehicle in the cross-sectional plane, ZX, at Y = 0.5 m, which corresponds to the location of vehicular exhaust exit. Hence, the jet flow structure at this wake region is very important for understanding the dispersion and concentration of gaseous and particle pollutants. shows clearly a different vortex structure than that observed in . The upper vortex of is larger and more dominant along the downstream distance from the cross-sectional plane of the studied ground vehicle at the centerline of the studied ground vehicle than that in . The change in recirculation region along the downstream distance from the centerline of the studied ground vehicle clearly shows the interaction effect between the vehicular exhaust jet plume and the wake region behind the studied vehicle.
FIG. 3 Time-averaged flow streamlines at (a) Y = 0 m and (b) Y = 0.5 m and time-averaged particle number concentration at (c) Y = 0 m and (d) Y = 0.5 m of the studied ground vehicle in the cross-sectional plane, ZX, for Case 1 (i.e., 10 km/h). (Figure provided in color online.)
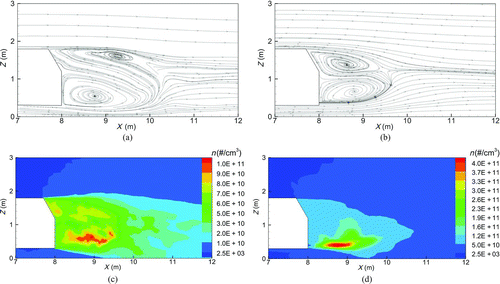
The time-averaged particle number concentrations of the studied ground vehicle in the cross-sectional planes, ZX, at Y = 0 m and Y = 0.5 m are shown in and d, respectively. Different from the gaseous pollutant concentration, which decreases along the length of the vehicular exhaust jet plume as the distance from its pollutant source at the tailpipe exit increases (CitationChan et al. 2008b), the maximum particle number concentration is observed at about 0.8 m away from the vehicular exhaust tailpipe exit in and d. This is because the nucleation affects the particle number concentration mostly and is mainly due to the cooling and dilution of the exhaust from the tailpipe to ambient air. Then, the particle number concentration decreases along the downstream distance due to the coagulation and dilution process. The overall distribution of particle number concentration shows a general upward dispersion where the particles are drawn up into the recirculation region through the lower vortex. With reference to the vortex structure at Y = 0.5 m in , it is clearly demonstrated that the location of X = 9.26 m (i.e., x/H = 0.7) behind the studied ground vehicle is a primary pick-up point for the particles emitted from vehicular exhaust plume and formed by nucleation process. The particles are drawn up into the recirculation region by the anticlockwise rotation of the lower vortex and then recirculated back by the lower vortex toward the rear end of the studied ground vehicle and into the upper vortex.
The detailed formation and characteristics of the streamwise longitudinal vortices in the near-wake region behind the similar studied ground vehicle can be referred to our previous work of CitationChan et al. (2008b) in that Figure 12 for the time-averaged velocity vector distribution in the cross-sectional, YZ, plane. It has been clearly demonstrated that the downstream development of the streamwise longitudinal vortices is shown by the increased rotation within the flow field. For X ≤ 10 m, there is only a weak rotation in this cross-sectional plane, ZY, due to the dominance of the streamwise rotation within the recirculation region. The magnitude of these vortex pairs becomes stronger along the downstream distance (e.g., 12 m ≤ X ≤ 16 m) because the dominant effect of the recirculation region lessens (i.e., the vortex begins to grow around the trailing edge of the recirculation region). From X = 10 to 12 m, the core of this vortex pair moves inwards and downwards the ground along the downstream distance. Similar flow behaviors are also observed (CitationDong and Chan 2006; CitationChan et al. 2008a, Citation2008b). It is likely that the increased rotation in the flow due to this vortex growth is responsible for the change in the recirculation region as shown in and b. In addition, the increased rotational strength of the vortex along the downstream distance may have the effect of stretching the recirculation region, thus resulting in the longer recirculation length in this recirculation region (i.e., Y = 0.5 m).
–d show the time-averaged particle number concentration behind the studied ground vehicle in the cross-sectional plane, YZ, at x / H = 0, 1, 2, and 5 for Case 1 (i.e., 10 km/h). Compared with the time-averaged flow streamlines at Y = 0 m and Y = 0.5 m in and b, it could be observed that x/H = 1 falls within the recirculation region, while x/H = 2 and 5 fall outside as shown in and d. At x/H = 1, there is picking up of particles by the lower vortex, and the particle number concentration fields are primarily at this location due to the recirculation of particles moving from the lower vortex into the upper vortex. The overall distributions of particle number concentration at x/H = 2 and 5 are wider spread due to the change in the relative vortex sizes of the streamwise longitudinal vortices along the downstream distance from the rear end of the studied ground vehicle.
FIG. 4 Time-averaged particle number concentration behind the studied ground vehicle in the cross-sectional plane, ZY, at (a) x/H = 0 (X = 8 m), (b) x/H = 1 (X = 9.8 m), (c) x/H = 2 (X = 11.6 m), and (d) x/H = 5 (X = 17 m) for Case 1 (i.e., 10 km/h). (Figure provided in color online.)
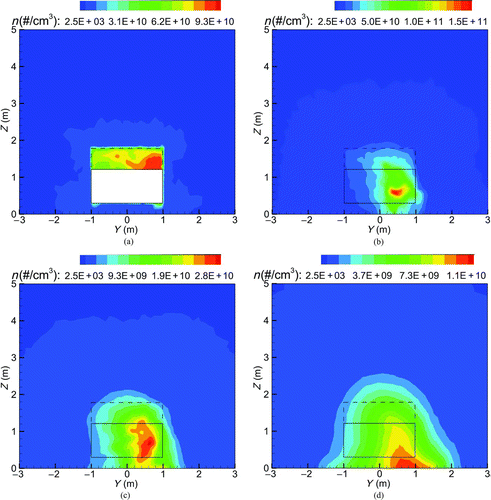
shows the time-averaged nucleation rate of the studied ground vehicle in the cross-sectional plane, ZX, with Y = 0.5 m (i.e., the centerline of the vehicular exhaust tailpipe exit) for Case 1. Recently, CitationKittelson et al. (2006) have shown that the nucleation process takes place mainly by the cooling and dilution of the vehicular exhaust jet plume to ambient air, and the driving force for the nucleation of nanoparticles during dilution is saturation ratio, which is highly temperature dependent. CitationHousiadas et al. (2004) have also experimentally demonstrated that the effect of root mean square (RMS) temperature on nucleation rate is usually even greater than the temperature itself, which can also be observed in the present computational study by comparing with and , respectively. shows the RMS temperature of the studied ground vehicle in the cross-sectional plane, ZX, with Y = 0.5 m for Case 1. The nucleation rate is much more correlated with the distribution of RMS temperature than with that of the temperature itself.
FIG. 5 Time-averaged particle nucleation rate of the studied ground vehicle in the cross-sectional plane, ZX, at Y = 0.5 m for Case 1 (i.e., 10 km/h). (Figure provided in color online.)
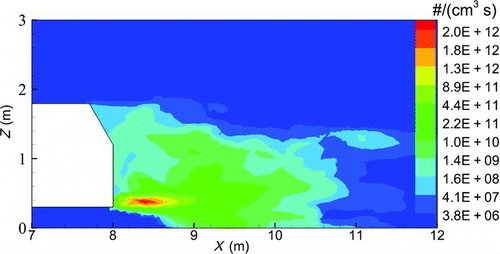
FIG. 6 RMS temperature of the studied ground vehicle in the cross-sectional plane, ZX, at Y = 0.5 m for Case 1 (i.e., 10 km/h). (Figure provided in color online.)
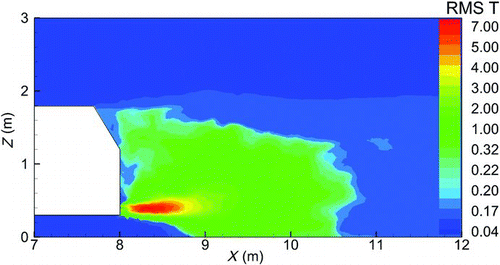
The time-averaged particle number concentration contours of the studied ground vehicle in the cross-sectional plane, ZX, with Y = 0.5 m for different vehicle speeds (i.e., Cases 2–4) are shown in . A lower particle number concentration behind the studied ground vehicle is observed for a higher vehicle speed because of the rapidly increasing dilution ratio for higher vehicle speed. From , the maximum particle number concentration in Case 2 (i.e., 30 km/h) is 9 × 109/cm3 that is almost fifty times higher than that in Case 4 (i.e., 70 km/h). The time-averaged nucleation rate, which contributes most to the maximum particle concentration at this dilution stage (i.e., “tailpipe-to-road” process) (CitationZhang and Wexler 2004), in the cross-sectional plane, ZX, with Y = 0.5 m for different vehicle speeds is shown in . The relatively lower maximum nucleation rate in turbulent mixing flow is found in the near-wake region of the studied vehicle with the higher vehicle speed (i.e., corresponding to the higher exhaust velocity and temperature at the tailpipe exit) based on these four studied vehicle driving cases as listed in .
FIG. 7 Time-averaged particle number concentration of the studied ground vehicle in the cross-sectional plane, ZX, at Y = 0.5 m for (a) Case 2 (i.e., 30 km/h), (b) Case 3 (i.e., 50 km/h), and (c) Case 4 (i.e., 70 km/h). (Figure provided in color online.)
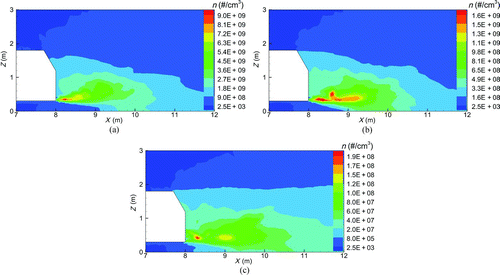
FIG. 8 Time-averaged particle nucleation rate of the studied ground vehicle in the cross-sectional plane, ZX, at Y = 0.5 m for (a) Case 2 (i.e., 30 km/h), (b) Case 3 (i.e., 50 km/h), and (c) Case 4 (i.e., 70 km/h). (Figure provided in color online.)
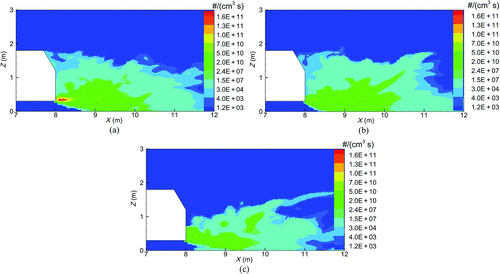
shows the time-averaged particle volume concentration of the studied ground vehicle in the cross-sectional plane, ZX, with Y = 0.5 m for different vehicle speeds. Owing to the flow recirculation region in the near-wake region behind the studied ground vehicle, some of the exhaust H2SO4 from the tailpipe is trapped by the vortex and recirculated back to the rear end of the studied ground vehicle as shown in and b. Therefore, the exhaust particle concentration in this wake region is much higher than farther downwind. As a result, the saturation ratio may become high enough to induce the nucleation. In the meantime, the H2SO4 may also condense quickly on the particles due to the existence of large surface areas of particles in the exhaust jet plume which may suppress nucleation and increase the particles volume concentration. In addition, the coagulation rate in this region is also increased because the large particle number concentration has enhanced the effect of condensation in reducing nucleation by increasing the surface area and decreasing the particle number concentration. The particle volume concentration beyond the recirculation region is diluted gradually. The higher the vehicle speed is, the lower the particle volume concentration occurs.
FIG. 9 Time-averaged particle volume concentration of the studied ground vehicle in the cross-sectional plane, ZX, at Y = 0.5 m for (a) Case 1 (i.e., 10 km/h), (b) Case 2 (i.e., 30 km/h), (c) Case 3 (i.e., 50 km/h), and (d) Case 4 (i.e., 70 km/h). (Figure provided in color online.)
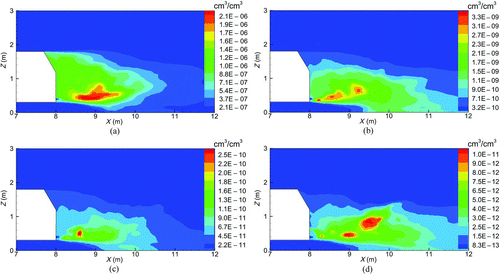
Since the nucleation rate is highly dependent on the temperature, relative humidity and vapor concentration, and these sensitive parameters will change with the flow field condition instantaneously, hence the present study demonstrates that formation and evolution of vehicle exhaust particle number concentrations are very complex to be predicted and simulated in the wake region of a moving vehicle. However, it is worth noting that the sensitivity of influential parameters (i.e., sulfur fuel content, mass and initial diameter, geometric average diameter and standard deviation, number concentration and size distribution of emitted soot particles, mass flow rate and hot gas temperature at the tailpipe exit conditions, the background soot mode, ambient humidity and temperature, vehicle speeds, turbulent mixing, etc.) on the exhaust particle dynamic behavior, formation and evolution processes, and number concentration fields in the wake region of moving vehicle(s) poses a major challenge for future studies and new situations.
CONCLUSION
In the present study, the interaction effects of different vehicle speeds, and exhaust tailpipe exit velocity and temperature conditions on the three-dimensional flow structure, exhaust particle dynamic behavior, formation and evolution processes (i.e., nucleation, coagulation, condensation, and dispersion), number and volume concentration, and nucleation rate fields in the near-wake region behind the studied ground vehicle in urban road microenvironment were comprehensively simulated by using LES with the aerosol dynamics and dispersion model based on our recently established DQMOM approach. The maximum particle number concentration is observed at about 0.8 m away from the vehicular tailpipe exit in the cross-sectional plane, ZX, with Y = 0.5 m. The overall distribution of particle number concentration shows that the particles are drawn up into the recirculation region and then moved toward the upper vortex by its lower vortex accordingly. Owing to the change in the relative vortex sizes of the longitudinal streamwise vortices, the distribution of particle number concentration in the cross-sectional plane, YZ, at x/H = 2 and 5 is wider spread than x/H = 1. The particle nucleation rate is much more associated with the distribution of RMS temperature than with that of the temperature itself. Some of the emitted H2SO4 concentration from the vehicular exhaust tailpipe are trapped by the vortex and then recirculated back to the rear end of the studied ground vehicle. As a result, the exhaust particle concentration in this region is much higher than farther distance downstream and the nucleation rate is also enhanced in this region. The exhaust particle volume concentration is the highest in the recirculation region behind the studied ground vehicle and is diluted gradually beyond the recirculation region in the downstream.
Acknowledgments
This work was supported by the grants from the Departmental and Central Research Grants of The Hong Kong Polytechnic University (project nos. G-U738 and G-U918). The authors would like to thank the valuable discussions from Dr. Mingzhou Yu, China Jiliang University, Hangzhou, China, on the present work.
REFERENCES
- Bae , S. Y. , Jung , C. H. and Kim , Y. P. 2009 . Development of an Aerosol Dynamics Model for Dry Deposition Process Using the Moment Method . Aerosol Sci. Technol. , 43 ( 6 ) : 570 – 580 .
- Baker , C. J. 2001 . Flow and Dispersion in Ground Vehicle Wakes . J. Fluid Struct. , 15 ( 7 ) : 1031 – 1060 .
- Baumgard , K. J. and Johnson , J. H. 1996 . The Effect of Fuel and Engine Design on Diesel Exhaust Particle Size Distributions SAE Technical Papers No. 960131, SAE International, Warrendale, PA, USA
- Bessagnet , B. and Rosset , R. 2001 . Fractal Modelling of Carbonaceous Aerosols—Application to Car Exhaust Plumes . Atmos. Environ. , 35 ( 8 ) : 4751 – 4762 .
- Brown , D. P. , Kauppinen , E. I. , Jokiniemi , J. K. , Rubin , S. G. and Biswas , P. 2006 . A Method of Moments Based CFD Model for Polydisperse Aerosol Flows with Strong Interphase Mass and Heat Transfer . Comput. Fluids , 35 ( 7 ) : 762 – 780 .
- Casati , R. , Scheer , V. , Vogt , R. and Benter , T. 2007 . Measurement of Nucleation and Soot Mode Particle Emission from a Diesel Passenger Car in Real World and Laboratory in Situ Dilution . Atmos. Environ. , 41 ( 10 ) : 2125 – 2135 .
- Chan , T. L. , Gosse , K. , Zhou , Y. , Lee , S. C. , Wang , X. W. and Huang , J. F. 2008a . Effect of Rear Slant Angle on Flow Structures, and Pollutant Dispersion and Concentration Fields in the Wake of the Studied Model Vehicle . Int. J. Heat Mass Tran. , 51 ( 25–26 ) : 6180 – 6193 .
- Chan , T. L. , Lin , J. Z. , Zhou , K. and Chan , C. K. 2006 . Simultaneous Numerical Simulation of Nano and Fine Particle Coagulation and Dispersion in a Round Jet . J. Aerosol Sci. , 37 ( 11 ) : 1545 – 1561 .
- Chan , T. L. , Liu , Y. H. and Chan , C. K. 2010a . Direct Quadrature Method of Moments for the Exhaust Particle Formation and Evolution in the Wake of the Studied Ground Vehicle . J. Aerosol Sci. , 41 ( 6 ) : 553 – 568 .
- Chan , T. L. , Luo , D. D. , Cheung , C. S. and Chan , C. K. 2008b . Large Eddy Simulation of Flow Structures and Pollutant Dispersion in the Near-Wake Region of the Studied Ground Vehicle for Different Driving Conditions . Atmos. Environ. , 42 ( 21 ) : 5317 – 5339 .
- Chan , T. L. , Ning , Z. , Wang , J. S. , Cheung , C. S. , Leung , C. W. and Hung , W. T. 2007 . Gaseous and Particle Emission Factors from the Selected on-Road Petrol/Gasoline, Diesel, and Liquefied Petroleum Gas Vehicles . Energ. Fuel. , 21 ( 5 ) : 2710 – 2718 .
- Chan , T. L. , Zhou , K. , Lin , J. Z. and Liu , C. -H. 2010b . Vehicular Exhaust Gas-to-Nanoparticle Conversion and Concentration Distribution in the Vehicle Wake Region . Int. J. Nonlinear Sci. Numer. Simul. , 11 ( 8 ) : 581 – 593 .
- Davidson , C. I. , Phalen , R. F. and Solomon , P. A. 2005 . Airborne Particulate Matter and Human Health: A Review . Aerosol Sci. Technol. , 39 ( 8 ) : 737 – 749 .
- Diemer , R. B. and Olson , J. H. 2002 . A Moment Methodology for Coagulation and Breakage Problems: Part 2- Moment Models and Distribution Reconstruction . Chem. Eng. Sci. , 57 ( 12 ) : 2211 – 2228 .
- Dong , G. and Chan , T. L. 2006 . Large Eddy Simulation of Flow Structures and Pollutant Dispersion in the Near-Wake Region of a Light-Duty Diesel Vehicle . Atmos. Environ. , 40 ( 6 ) : 1104 – 1116 .
- Eskridge , R. E. and Hunt , J. C. R. 1979 . Highway Modeling. 1. Prediction of Velocity and Turbulence Fields in the Wake of Vehicles . J. Appl. Meteorol. , 18 ( 4 ) : 387 – 400 .
- Friedlander , S. K. 2000 . Smoke, Dust, and Haze: Fundamentals of Aerosol Dynamics , New York : Oxford University Press .
- Germano , M. 1996 . A Statistical Formulation of the Dynamic Model . Phys. Fluid. , 8 ( 2 ) : 565 – 570 .
- Giechaskiel , B. , Alfoldy , B. and Drossinos , Y. 2009 . A Metric for Health Effects Studies of Diesel Exhaust Particles . J. Aerosol Sci. , 40 ( 8 ) : 639 – 651 .
- Hider , Z. E. , Hibberd , S. and Baker , C. J. 1997 . Modelling Particulate Dispersion in the Wake of a Vehicle . J. Wind Eng. Ind. Aerod. , 67–68 : 733 – 744 .
- Housiadas , C. , Drossinos , Y. and Lazaridis , M. 2004 . Effect of Small-Scale Turbulent Fluctuations on Rates of Particle Formation . J. Aerosol Sci. , 35 ( 5 ) : 545 – 559 .
- Jiang , P. Z. , Lignell , D. O. , Kelly , K. E. , Lighty , J. S. , Sarofim , A. F. and Montgomery , C. J. 2005 . Simulation of the Evolution of Particle Size Distributions in a Vehicle Exhaust Plume with Unconfined Dilution by Ambient Air . J. Air Waste Manage. Assoc. , 55 ( 4 ) : 437 – 445 .
- Kanda , I. , Uehara , K. , Yamao , Y. , Yoshikawa , Y. and Morikawa , T. 2006 . A Wind-Tunnel Study on Exhaust Gas Dispersion from Road Vehicles—Part I: Velocity and Concentration Fields Behind Single Vehicles . J. Wind Eng. Ind. Aerod. , 94 ( 9 ) : 639 – 658 .
- Kerminen , V. M. , Pakkanen , T. A. , Makela , T. , Hillamo , R. E. , Sillanpaa , M. , Ronkko , T. , Virtanen , A. , Keskinen , J. , Pirjola , L. , Hussein , T. and Hameri , K . 2007 . Development of Particle Number Size Distribution Near a Major Road in Helsinki During an Episodic Inversion Situation . Atmos. Environ. , 41 ( 8 ) : 1759 – 1767 .
- Keskinen , J. and Ronkko , T. 2010 . Can Real-World Diesel Exhaust Particle Size Distribution be Reproduced in the Laboratory? A Critical Review . J. Air Waste Manage. Assoc. , 60 ( 10 ) : 1245 – 1255 .
- Kim , D. H. , Gautam , M. and Gera , D. 2002 . Modeling Nucleation and Coagulation Modes in the Formation of Particulate Matter Inside a Turbulent Exhaust Plume of a Diesel Engine . J. Colloid Interface Sci. , 249 ( 1 ) : 96 – 103 .
- Kittelson , D. B. 1998 . Engines and Nanoparticles: A Review . J. Aerosol Sci. , 29 ( 5–6 ) : 575 – 588 .
- Kittelson , D. B. , Watts , W. F. and Johnson , J. P. 2006 . On-Road and Laboratory Evaluation of Combustion Aerosols—Part 1: Summary of Diesel Engine Results . J. Aerosol Sci. , 37 ( 8 ) : 913 – 930 .
- Leniewski , T. and Friedlander , S. K. 1995 . The Effect of Turbulence on Rates of Particle Formation by Homogeneous Nucleation . Aerosol Sci. Technol. , 23 ( 2 ) : 174 – 182 .
- Liu , Z. G. , Vasys , V. N. , Dettmann , M. E. , Schauer , J. J. , Kittelson , D. B. and Swanson , J. J. 2009 . Comparison of Strategies for the Measurement of Mass Emissions from Diesel Engines Emitting Ultra-Low Levels of Particulate Matter . Aerosol Sci. Technol. , 43 ( 11 ) : 1142 – 1152 .
- Marchisio , D. L. and Fox , R. O. 2005 . Solution of Population Balance Equations Using the Direct Quadrature Method of Moments . J. Aerosol Sci. , 36 ( 1 ) : 43 – 73 .
- Mathis , U. , Ristimaki , J. , Mohr , M. , Keskinen , J. , Ntziachristos , L. , Samaras , Z. and Mikkanen , P. 2004 . Sampling Conditions for the Measurement of Nucleation Mode Particles in the Exhaust of a Diesel Vehicle . Aerosol Sci. Technol. , 38 ( 12 ) : 1149 – 1160 .
- McGraw , R. 1997 . Description of Aerosol Dynamics by the Quadrature Method of Moments . Aerosol Sci. Technol. , 27 ( 2 ) : 255 – 265 .
- McGraw , R. , Huang , P. I. and Schwartz , S. E. 1995 . Optical-Properties of Atmospheric Aerosols from Moments of the Particle-Size Distribution . Geophys. Res. Lett. , 22 ( 21 ) : 2929 – 2932 .
- McGraw , R. , Nemesure , S. and Schwartz , S. E. 1998 . Properties and Evolution of Aerosols with Size Distributions having Identical Moments . J. Aerosol Sci. , 29 ( 7 ) : 761 – 772 .
- Miller , S. E. and Garrick , S. C. 2004 . Nanoparticle Coagulation in a Planar Jet . Aerosol Sci. Technol. , 38 ( 1 ) : 79 – 89 .
- Pohjola , M. A. , Pirjola , L. , Kukkonen , J. and Kulmala , M. 2006 . Correction to Modelling of the Influence of Aerosol Processes for the Dispersion of Vehicular Exhaust Plumes in Street Environment . Atmos. Environ. , 40 ( 2 ) : 311 – 314 .
- Pope , C. A. and Dockery , D. W. 2006 . Health Effects of Fine Particulate Air Pollution: Lines that Connect . J. Air Waste. Manage. Assoc. , 56 ( 6 ) : 709 – 742 .
- Pratsinis , S. E. 1988 . Simultaneous Nucleation, Condensation and Coagulation in Aerosol Reactors . J. Colloid Interf. Sci. , 124 ( 2 ) : 416 – 427 .
- Smagorinsky , J. 1963 . General Circulation Experiments with the Primitive Equations. I. The Basic Experiment . Mon. Weather Rev. , 91 : 99 – 164 .
- Uhrner , U. , von Lowis , S. , Vehkamaki , H. , Wehner , B. , Brasel , S. , Hermann , M. , Stratmann , F. , Kulmala , M. and Wiedensohler , A. 2007 . Dilution and Aerosol Dynamics within a Diesel Car Exhaust Plume—CFD Simulations of On-Road Measurement Conditions . Atmos. Environ. , 41 ( 35 ) : 7440 – 7461 .
- Vehkamaki , H. , Kulmala , M. , Lehtinen , K. E. J. and Noppel , M. 2003 . Modelling Binary Homogeneous Nucleation of Water-Sulfuric Acid Vapours: Parameterisation for High Temperature Emissions . Environ. Sci. Technol. , 37 ( 15 ) : 3392 – 3398 .
- Vouitsis , E. , Ntziachristos , L. and Samaras , Z. 2005 . Modelling of Diesel Exhaust Aerosol During Laboratory Sampling . Atmos. Environ. , 39 ( 7 ) : 1335 – 1345 .
- Wong , C. P. , Chan , T. L. and Leung , C. W. 2003 . Characterisation of Diesel Exhaust Particle Number and Size Distributions Using Mini-Dilution Tunnel and Ejector-Diluter Measurement Techniques . Atmos. Environ. , 37 ( 31 ) : 4435 – 4446 .
- Yin , Z. Q. , Lin , J. Z. , Zhou , K. and Chan , T. L. 2007 . Numerical Simulation of the Formation of Pollutant Nanoparticles in the Exhaust Twin-Jet Plume of a Moving Car . Int. J. Nonlinear Sci. Numer. Simul. , 8 ( 4 ) : 535 – 543 .
- Yu , M. Z. , Lin , J. Z. and Chan , T. L. 2008 . A New Moment Method for Solving the Coagulation Equation for Particles in Brownian Motion . Aerosol Sci. Technol. , 42 ( 9 ) : 705 – 713 .
- Yu , M. Z. , Lin , J. Z. and Chan , T. L. 2009 . Numerical Simulation for Nucleated Vehicle Exhaust Particulate Matters Via the TEMOM/LES Method . Int. J. Mod. Phys. C , 20 ( 3 ) : 399 – 421 .
- Zhang , K. M. and Wexler , A. S. 2004 . Evolution of Particle Number Distribution Near Roadways—Part I: Analysis of Aerosol Dynamics and Its Implications for Engine Emission Measurement . Atmos. Environ. , 38 ( 38 ) : 6643 – 6653 .