Abstract
The Colorado Coarse Rural Urban Sources and Health study (CCRUSH) is an ongoing study of the relationship between coarse particulate mass concentrations (PM10–2.5, particulate matter with diameter between 2.5 and 10 μm) and selected health effects. For two urban monitoring sites in Denver, CO, and two comparatively rural sites in Greeley, CO, hourly mass concentrations of PM10–2.5 and fine particulate matter (PM2.5, diameter less than 2.5 μm) have been measured by using dichotomous tapered element oscillating microbalances (TEOMs) with Filter Dynamics Measurement Systems (FDMS). This paper presents air quality results from just over a year of PM2.5 and PM10–2.5 measurements. Average PM2.5 concentrations ranged from 7.7 to 9.2 μg m−3 across the four sites with higher concentrations in Denver than Greeley. Average PM10–2.5 concentrations ranged from 9.0 to 15.5 μg m−3 with the highest values at the site in northeast Denver. Temporal variability in PM10–2.5 was higher than that in PM2.5 concentrations at all four sites. The two Greeley sites displayed moderate spatial correlation for PM2.5 and high correlation for PM10–2.5, whereas the two Denver sites showed lower spatial correlation for both PM sizes. PM10–2.5 concentrations in Denver were highest with winds from the direction of the city's urban core. PM10–2.5 concentrations in Greeley were moderately elevated with winds from the southwest to the northwest, coming from Denver and other large Front Range communities. Wind speed regressions for PM10–2.5 at the Denver sites primarily exhibited resuspension effects, while PM10–2.5 concentrations in Greeley showed relatively complex wind speed dependence.
Copyright 2012 American Association for Aerosol Research
1. INTRODUCTION
Coarse particulate matter in the size range from 2.5 to 10 μm (PM10–2.5) is believed to be important for human health because particles in this size range are capable of penetrating the thoracic region of the lungs when inhaled (Chan and Lippmann Citation1980). Size-resolved and chemically speciated data indicate that compared to particulate matter less than 2.5 μm in diameter (PM2.5), PM10–2.5 is more likely to contain crustal elements such as aluminum, iron, and calcium, but may also contain ions, transition metals, organic, and biological material (Milford and Davidson Citation1985, Citation1987; Boreson et al. Citation2004; Hueglin et al. Citation2005). PM10–2.5 is commonly derived from abrasive mechanical processes, including construction and agricultural activities, resuspended road dust, vegetative debris, and sea spray (Duce et al. Citation1976; Patterson and Gillette Citation1977), with emissions from many of these processes depending strongly on the wind speed (Harrison et al. Citation2001). It is also produced from incomplete combustion of solid fuels such as coal and biomass (U.S. EPA Citation1995).
Brunekreef and Forsberg (Citation2005) reviewed nearly 60 studies that evaluated health effects of short-term exposure to PM10–2.5 and concluded that for some endpoints, including chronic obstructive pulmonary disease, asthma, and respiratory admissions, PM10–2.5 could have as strong or a stronger effect than PM2.5. Short-term increases in PM10–2.5 have also been positively associated with mortality in several studies (Castillejos et al. Citation2000; Mar et al. Citation2000; Ostro et al. Citation2000; Villeneuve et al. Citation2003; Zanobetti and Schwartz Citation2009). In a recent review of studies of the health effects associated with short-term exposure to ambient PM10–2.5, the U.S. Environmental Protection Agency (EPA) concluded that existing evidence is suggestive of a causal relationship between exposures and mortality, cardiovascular effects, and respiratory effects (U.S. EPA Citation2009). EPA also recognized several critical uncertainties in epidemiology studies of PM10–2.5 impacts, including relatively high exposure error compared to PM2.5, due to greater expected spatial variability in PM10–2.5 concentrations, and limitations in the characterization of spatial distributions. Many epidemiologic studies published to date have used differences between PM10 and PM2.5 concentrations measured at colocated monitors and in some cases, monitors located at different sites within the same county to estimate PM10–2.5, which contributes further uncertainty in exposure estimation. Furthermore, epidemiologic studies of PM10–2.5 have mostly focused on urban areas, where large populations result in greater power for detecting statistically significant effects. Because sources of PM10–2.5 may be different in urban compared to rural regions, the associations with health effects may also be different in smaller communities or rural areas.
Because of their size, coarse particles are removed from the atmosphere more quickly than fine particles. As a consequence of both deposition velocity and the intermittent nature of many source processes, concentrations of PM10–2.5 are expected to be more spatially and temporally variable than PM2.5 concentrations. Wilson et al. (Citation2005) reviewed prior studies and found that reported correlation coefficients between sites within several cities ranged from 0.14 to 0.60 for 24-h average PM10–2.5 concentrations; these values are generally lower than those observed for PM2.5 or PM10. Chen et al. (Citation2007) found an average correlation coefficient of 0.75 between 24-h average PM10–2.5 concentrations measured for about 70 days at a central monitor in Chapel Hill, NC, and monitors placed at residences within about a 60 km radius. With a year of weekly monitoring at 10 sites across the Los Angeles basin, Pakbin et al. (Citation2010) found pairwise correlations ranging from 0.04 for 24-h average PM10–2.5 concentrations from an industrial site in Long Beach and concentrations at 2 suburban monitors located about 30 and 70 km away, to 0.80 for PM10–2.5 concentrations at a pair of coastal sites located within a few kilometers of each other.
Most studies of seasonal variability in PM10–2.5 concentrations have observed the highest concentrations in summer, but exceptions occur due to specific source activity patterns (Thornburg et al. Citation2009; Pakbin et al. Citation2010). Harrison et al. (Citation2001) measured PM10–2.5 continuously at five sites in England over a 3-year period. They observed higher PM10–2.5 concentrations on weekdays than on weekends and found that the fraction of PM10 contributed by PM10–2.5 was highest in the spring and summer. Moore et al. (Citation2010) reported correlation coefficients of 0.1–0.4 for continuous hourly PM10–2.5 concentrations measured at 3 sites across the Los Angeles basin. In their study, the most pronounced diurnal variation in PM10–2.5 concentrations was observed at a site near Riverside, CA, with less diurnal variability in concentrations measured near downtown Los Angeles and at a desert location about 110 km NW of downtown. Daytime or evening maxima were observed at all three locations.
This paper presents just over a year of mass concentration data from continuous PM10–2.5 and PM2.5 sampling conducted in Denver and Greeley, CO, as part of the Colorado Coarse Rural Urban Sources and Health (CCRUSH) study. CCRUSH is a multiyear study of the relationship between PM10–2.5 mass concentrations and adverse health effects, including cardiopulmonary emergency department visits and adverse birth outcomes. Denver and Greeley were selected for the study to allow comparison of the composition and relative health effects of coarse PM in urban and rural communities. For two sites in Denver and two sites in Greeley, continuous hourly mass concentrations of PM10–2.5 and PM2.5 were measured using dichotomous tapered element oscillating microbalances (TEOMs). Sampling began in January 2009 and will continue for three years. At the end of the sampling period, the mass concentration data will be analyzed with local data on birth outcomes and emergency department visits to assess and compare the associations between the two communities.
This paper examines spatial and temporal variations in hourly and 24-h average concentration values for PM10–2.5 and PM2.5. It also examines the influence of hourly wind speed and wind direction on the mass concentrations. Nonparametric regression (NPR; Henry et al. Citation2002, Citation2009; Kim and Hopke Citation2004; Yu et al. Citation2004) was used to characterize the wind speed and wind direction relationships, and help understand differences in mass concentrations across sampling locations.
2. METHODS
2.1 Sampling Locations
Continuous particulate mass concentrations were measured at two locations in Denver and two in Greeley, CO. Greeley has a population of 92,625 (U.S. Census Citation2009b) and an area of 77.7 km2, and is located within Weld County. Weld County has a population of 254,759 (U.S. Census Citation2009a) and an area of 10,417 km2. Agriculture and oil and gas extraction are among the county's leading economic activities. In contrast, the City and County of Denver has a population of 610,345 (U.S. Census Citation2009c) and an area of 401.3 km2, with a highly mixed economy (the urban areaFootnote 1 of Denver–Aurora has a population of 1.98 million and an area of 1291.9 km2). Denver is transected by major interstate highways and experiences much greater traffic volumes than Greeley. Correspondingly, PM10–2.5 concentrations in Denver are expected to be dominated by resuspended urban road dust, while agricultural activities (e.g., feedlots, soil preparation, and ditch burning) are expected to be relatively important sources in Greeley.
Monitors were located on the roofs of two elementary schools in Denver: Alsup and Edison, which are 11.1 km apart. Monitors were located in Greeley on the roof of Maplewood elementary school and in the heating, ventilation, and air conditioning (HVAC) system enclosure at McAuliffe elementary school. The two Greeley schools are 4.5 km apart. The city of Greeley is roughly 80 km northeast of Denver. includes site descriptions, sampling periods, and completeness statistics for the CCRUSH data sets considered.
TABLE 1 Monitoring site descriptions, sampling periods, and completeness statistics for the CCRUSH study
2.2 Particulate Matter Monitoring Methods
TEOM, model 1405-DF (ThermoFisher Scientific, Waltham, MA) ambient PM monitors were located at each site. Three monitors (Alsup, Edison, and Maplewood) were located outside on roofs and housed in enclosures (Complete Outdoor Enclosure for TEOM Series 1405, ThermoFisher Scientific, Waltham, MA) designed to maintain appropriate instrument conditions. At extreme high and low ambient temperatures, the enclosures failed to maintain appropriate instrument operating conditions, which resulted in data removal. The monitor located at McAuliffe was located just below the roof in an HVAC system crawl space and was equipped with an in-house designed foam enclosure equipped with a commercial air conditioner/heater unit set to maintain a temperature of 21.1°C.
The TEOM 1405-DF is equipped with a Filter Dynamic Measurement System (FDMS) to correct for semivolatile species evaporation from mass measurement filter surfaces. After a 16.7 L/min PM10 impactor inlet removes the particles larger than 10 μm from the sample stream, a round-nozzle virtual impactor is used to separate the PM2.5 and PM10–2.5 size fractions into dual measurement channels. Virtual impactors separate size fractions using particle inertia similar to a traditional inertial impactor. By replacing the impaction surface with a vertical collection probe with a low flow rate, virtual impactors separate larger particles that have sufficient inertia to impact the “virtual surface” of the cross-section of the collection probe inlet. Flow through the collection probe is referred to as the minor flow. The major flow, containing small particles, diverts away from the collection probe and has a collection efficiency that is less than unity due to a fraction of the smaller particles penetrating into the collection probe. This fraction is defined by the ratio of minor to inlet flow rates. Virtual impactor design and flow characteristics are described in detail elsewhere (Marple and Chien Citation1980; Loo and Cork Citation1988). The TEOM 1405-DF uses a 2.5-μm cut point virtual impactor with inlet, major (PM2.5), and minor (PM10–2.5) volumetric flow rates of 16.7, 15, and 1.67 L/min, respectively. Mass concentration corrections for penetration of PM2.5 mass into the PM10–2.5 channel are described in the data processing section below. The mass measurements are made by dual vertical oscillating tapered glass elements, one for each PM channel, with Pallflex TX-40 TEOM filters placed on the ends. Particulate mass is deposited as aerosol passes through the filter, which changes the natural oscillating frequency of the tapered glass element. The frequency change is related to filter mass change by simple vibration theory. The ambient mass concentrations are calculated by the change in mass and volumetric airflow rates.
With the FDMS system, the instrument operates by sampling in two modes that alternate every 6 min. In the “Base” measurement mode, the sample stream is held at 30°C with the aerosol passing directly to the mass measurement filter. The effect of water is reduced in the TEOM 1405-DF by the use of a Nafion™ membrane diffusion dryer in each particulate channel. In the Base mode, mass can be either lost or gained from the filter, depending on the amount of semivolatile material present. In the “Reference” mode, after the dryer, the sample is diverted through the cooled FDMS filter, a 47 mm Pallflex TX-40 filter held at 4°C. This filter removes the particles and semivolatile material that will condense at 4°C or below. This filtered air stream is then directed through the TEOM filter and the mass change on the filter recorded. Reference mode values are commonly negative due to mass loss from the TEOM filter, but adsorption or absorption of organic gases may also occur, resulting in mass gain (Green et al. Citation2009). The mass change during the Reference mode due to evaporation and gas-phase sampling artifacts is assumed to be equal to the artifact contribution to the mass change that occurred during the previous Base measurement. The time series of Reference mass concentrations are thus subtracted from the Base measurements, correcting for sampling artifacts and approximating the true aerosol mass concentration. This provides a total mass concentration for each 12-min time step, with the first 6 min providing the Base and the second 6 min providing the Reference concentration. The instruments were operated at flow rates prescribed by the manufacturer: 1.67 L/min (PM10–2.5), 3 L/min (PM2.5), and 12 L/min (bypass). For this study, TEOMs were set up to record the raw mass concentration data (Base and Reference) for the PM2.5 and PM10–2.5 channels, along with ambient temperature, relative humidity, and various instrument conditions, at 6 min intervals. Raw mass concentration data are not smoothed by between-measurement averaging for unmeasured channels and do not include a correction for the penetration of PM2.5 mass into the PM10–2.5 channel from the virtual impactor separation. This correction was made during subsequent data processing. Flow control was set to active, and actual flow rates were used to calculate the mass concentrations.
TEOM instrument maintenance was performed monthly at each site and consists of changing TEOM and FDMS filters; cleaning the PM10 inlet, virtual impactor, and FDMS valve; checking for seal leaks in the mass transducer, FDMS valve, and FDMS filter holder; flow audit and calibration; and an instrument leak check. Operators ensured that the instrument was operating properly before leaving the site. Other regular maintenance was performed as needed and included exchanging Nafion diffusion dryers, pump maintenance, and replacing mass transducer, FDMS valve, and FDMS filter holder seals. Ball valves were installed between the virtual impactor and diffusion dryers to increase the ease of access to sample lines for flow audits, which were performed at a higher frequency than prescribed by the manufacturer. A single external filter on the bypass flow line was used to extend pump life.
To assure the highest quality data were used for analysis, extensive quality assurance protocols were developed. Upon arriving at a monitoring site, an instrument status log, maintenance log, comment log, and flow audit/leak check log were completed. The status log was filled out before and after maintenance to assure that the instrument conditions did not change due to operator intervention. The TEOM data were downloaded manually each month prior to instrument maintenance. The discrete section of data from the last site visit to the current visit was downloaded via the available universal serial bus (USB) port on the front of the instrument. This process closed the previous section of data before the operator interfered with instrument operation. By using the ePort software provided by Thermo Scientific, the entire TEOM database was also downloaded. The data were transferred from a field laptop or flash drive to a desktop computer immediately upon arriving back at the University of Colorado.
Discrete data sections downloaded via USB flash drive were processed by a code developed in-house. Log files for each data section were created that specified data filenames, whether maintenance occurred, whether to output hourly averages, saved data interval, number of hours to remove after maintenance occurred, and number of hours to shift the time stamp into Mountain Standard Time (MST). Rows were flagged as missing data if the status code reported the following errors: power failure, database failure, FDMS valve failure, mass transducer failures, any channel flow deviating from the set flow rate (L/min) by more than 10%, either channel reading filter loading above 90%, or heater tube temperatures (°C) deviating from set temperature by more than 2%. Instrument problems were flagged as well and included: vacuum pressures above 40.5 kPa, cooler temperatures deviating more than 0.5°C from the specified set point, or if channel relative humidity was above 98%. Data corresponding to instrument problem flags were inspected manually.
Equations (Equation1)–(Equation3) were applied to the 6-min mass concentration data to correct for PM2.5 mass depositing in the PM10–2.5 channel due to the virtual impactor. In the following equations, Q represents the volumetric flow rate through the indicated channel. PM represents the mass concentration, with the TEOM label indicating raw TEOM data. It was assumed that both Base and Reference channels followed the same correction, i.e., that semivolatile mass loss was proportional to the amount of total mass in each channel
The hourly average and standard error (i.e., the standard deviation divided by the square root of the number of measurements in the hour) of all downloaded variables were calculated and exported, excluding data flagged as missing. Logs used to process data were accessed to compile full data sets, filling in missing sections of data between discrete data sets with missing data flags or combining same hour measurements with a weighted average based on the number of measurements made in that hour in each separate data set. Three scenarios were identified that required further data processing: major events of mass loss from filter surfaces, instances of highly variable noise due to temperature aliasing from rapid or oscillating changes of enclosure temperature or other sources, or instances of elevated standard error when a nonremoval status code had been triggered. The mass loss incidents were identified if the calculated mass concentration was less than the 1st percentile of the time series and the standard error of the measurement was above the 95th percentile. The incidents of induced highly variable noise were identified if the calculated concentration was below the 1st percentile and the subsequent measurement was greater than the 99th percentile or vice versa. The third scenario was triggered when a nonzero status code was recorded and the calculated hourly mass concentration standard error was above the 95th percentile. Each occurrence of one of these three scenarios was assessed manually to determine if data should be removed for final hourly average data sets. The data were then filtered for hours with less than 75% completeness. Daily averages were calculated from cleaned hourly average data sets; days missing more than 75% of completed hours were also removed.
The data set reported in this paper has been labeled phase 1, and resulted from cutting off the currently validated results when instruments were updated to a new version of the TEOM 1405-DF firmware. This update required exchanging a physical flash card; after the update instrument settings were unintentionally reset to defaults. The start and end dates and completeness statistics for each site's phase 1 data are listed in . Sampling began on different dates at each site, and completeness varies by site based on instrument maintenance issues.
The TEOM 1405-DF is a relatively new instrument and correspondingly posed numerous challenges in our effort to produce the continuous time series of mass concentration data. Through collaboration with Thermo Scientific, solutions were found for most problems, but they nonetheless led to substantial gaps in our time series. Denver and Greeley experience significant seasonal temperature variations. The air heating and cooling systems incorporated into the Thermo Scientific TEOM 1405-DF enclosures were unable to adequately condition the space within the enclosures when ambient temperatures were very high or low. Numerous measurements from midday throughout the summer were suspect, and hence flagged for removal due to large hourly variability associated with increased TEOM mass transducer temperatures. This high measurement variability mostly originated in the Reference channel, where hourly standard errors sometimes exceeded 500 μg m−3. Cold temperature extremes were less of an issue, though the operating temperatures of the FDMS systems occasionally dropped below 4°C. These changes in FDMS operating temperature were not accompanied by significant increases in the variability of mass concentration measurements so that corresponding data were not removed. A further problem with the HVAC systems occurred at Alsup and Maplewood, where insulation near the blowers peeled off and either shredded or blocked the blowers.
Malfunction of the Nafion dryer assemblies and pumps also leads to gaps in the time series. Dryer assemblies had to be replaced every 7–10 months and the pumps rebuilt every 12 months, in each case about 6 months earlier than the manufacturer's maintenance recommendations. Premature pump failure may be partly due to low ambient atmospheric pressures in Colorado, which are typically about 85.1 kPa. In addition, the bypass flow controller of the TEOM installed at McAuliffe failed when the inlet system did not adequately dispose of water vapor in the bypass line, resulting in condensation when the air was cooled in the enclosure.
Finally, a significant gap in the McAuliffe data set occurred due to seal leaks within the FDMS valve system that were not detected through the leak check process. The problem was only identified upon later inspection of the data. In response, we modified our monthly maintenance protocol to include disassembling the FDMS valve to verify that no seals failed, and to process and examine data on-site to verify the absence of leaks.
2.3 Meteorological Data
To assist with the analysis of PM mass concentration data, hourly meteorological data (temperature, RH, wind speed, and direction) were obtained from stations located at or near each of the monitoring sites. Mass concentration data from Edison were related to meteorological data from the Carriage site (39.75 N, 105.03 W), located 1.65 km to the southeast and operated by the Colorado Department of Public Health and Environment (CDPHE). Meteorological data for Alsup were collected from a CDPHE-operated meteorological station colocated with our instrument. Meteorological data for Greeley were obtained from the National Oceanic and Atmospheric Administration's (NOAA) Weld County Airport station (40.26 N, 104.38 W), located 6.7 km west and 10.9 km west of Maplewood and McAuliffe monitoring sites, respectively. Wind roses of data used for site-specific wind speed and direction regressions from Carriage, Alsup, and the Weld County Airport are shown in the online Supplemental Information. Vector-averaged wind speed was used in the data analyses.
2.4 Data Analysis and NPR
Section 3 presents standard descriptive statistics for PM2.5 and PM10–2.5 mass concentrations, along with the coefficient of divergence (COD), which is a measure of uniformity. The results of NPR of concentrations versus wind speed and direction are also presented. All data analyses used concentration data that were error-code-filtered. No negative data filtering or replacement was performed in any of the analyses, except when calculating the COD.
The COD (Wilson et al. Citation2005) for a set of measurements, X, is defined by
The data set used in the NPR was different than that used in the other analyses, as it required wind data and mass concentrations for each hour, both of which had missing data. Additionally, any data point with a corresponding wind speed value below 1 ms−1 was excluded from the NPR analysis. Exclusion of these periods with relatively calm winds sharply reduced the number of observations used in the NPR analyses compared to the full sets of hourly mass concentration data.
NPR was used to estimate the expected concentration C i from each wind direction or wind speed i by including all observations using weighting kernels, giving less weight to observations far from the point at which the estimate is being calculated and vice versa. The Gaussian kernel
2.5 Comparison with Other PM Data Sets
As part of this study, comparisons were made to PM2.5 and PM10–2.5 data from other studies and locations. These comparisons are complicated by the use of different instruments and measurement methods. Federal reference methods (FRMs) and federal equivalence methods (FEMs) for PM have been discussed previously (U.S. EPA Citation2004, Citation2009). The FRM for PM10–2.5 is calculated as the numeric difference between concurrent and colocated PM10 and PM2.5 concentrations as measured by FRM low-volume filter samplers of the same make and model (U.S. EPA Citation2009). The TEOM 1400AB and 1405 have been designated as FEM methods for PM10. The TEOM 1405-DF has been designated as an FEM method for PM2.5, but not (to date) as an FEM for PM10–2.5 (U.S. EPA Citation2010).
As described above, the TEOM 1405-DF has been designed to minimize sampling artifacts, both positive and negative. Positive artifacts are a result of excess mass collection typically caused by gas-phase adsorption onto the collection media. Negative artifacts are a result of reduced mass collection typically caused by semivolatile species that were in the particle phase but shift to the gas phase after collection due to collection temperatures that are higher than ambient or pressures that are slightly less than ambient. For example, when PM2.5 concentrations were measured by a pair of TEOMs, one operated at 50°C and the other operated at 30°C, the TEOM held at a higher temperature yielded consistently lower concentrations, as at the higher temperature, the sensor collected less semivolatile and condensable mass (Grover et al. Citation2005; Zhu et al. Citation2006). The TEOM 1405-DF operates at 30°C and also utilizes an FDMS that adjusts for filter adsorption artifacts. The results from previous studies generally show that for PM2.5, the TEOM FDMS measures higher concentrations than the FRM, especially as the ambient temperature increases (Grover et al. Citation2005; Schwab et al. Citation2006; Zhu et al. Citation2006), the FRM does not adjust for adsorption artifacts (Solomon and Sioutas Citation2008). In the end, it is important to remember that comparison across studies that have used different measurement techniques will have slight biases associated with the technique differences. Thus, the PM10–2.5 data discussed below should be viewed as only roughly comparable across studies.
3. RESULTS AND DISCUSSION
3.1 Summary Statistics and Spatial Trends
presents the summary statistics for the 24-h average PM2.5 and PM10–2.5 concentrations measured at the four study sites. Average PM2.5 concentrations ranged from 7.7 to 9.2 μg m−3 across the four sites. Average concentrations of PM2.5 were somewhat higher at the two Denver sites than at the two sites in Greeley. Average PM10–2.5 concentrations ranged from 9.0 to 15.5 μg m−3. PM10–2.5 concentrations were sharply higher at the Alsup site in northeast Denver than at the other three locations. Temporal variability in PM10–2.5 concentrations was higher than that in PM2.5 concentrations, with COV values for 24-h average PM10–2.5 ranging from 0.6 to 0.8 and those for PM2.5 all near 0.5. Across the four sites, 95th percentile 24-h average concentrations ranged from 14.7 to 17.9 μg m−3 for PM2.5 and from 18.9 to 36.0 μg m−3 for PM10–2.5.
TABLE 2 Summary statistics for 24-h average PM2.5 and PM10–2.5 concentrations
The 2 Greeley sites, which are separated by a distance of 4.5 km, had the highest spatial correlation for 24-h average concentrations of both PM2.5 and PM10–2.5, with Pearson's R values of 0.82 for PM2.5 and 0.97 for PM10–2.5. Concentrations measured at the two Denver sites, separated by a distance of 11.1 km, showed lower correlation. For the Denver sites, the Pearson's R values were 0.64 for PM2.5 and 0.70 for PM10–2.5. The COD was calculated among all site pairs. The pair of Greeley sites displayed the most homogeneous 24-h average PM2.5 and PM10–2.5 concentrations (COD = 0.13 for PM2.5 and 0.15 for PM10–2.5), while the pair of Edison and Alsup was somewhat more heterogeneous (COD = 0.21 for PM2.5 and 0.30 for PM10–2.5). The heterogeneity of PM10–2.5 concentrations in Denver is influenced by the relatively high concentrations at Alsup. A point source close to Alsup appears to contribute to the elevated concentrations seen there, as discussed below in Section 3.3.
The finding of lower correlation and higher COD values for PM2.5 and PM10–2.5 in Denver than in Greeley is consistent with expectations, as the Denver monitors are separated by a greater distance and are located in more sharply contrasting neighborhoods. The finding of higher correlation for PM10–2.5 than for PM2.5 in both communities is unexpected, as prior studies have generally observed the opposite. The relatively low correlations for PM2.5 found in our study may be partly due to noise in the PM2.5 channel. In comparison, the PM10–2.5 channel is quite stable.
3.2 Temporal Patterns
compares the median concentrations of PM2.5 and PM10–2.5 between weekends and weekdays at each site, as well as between day (6 a.m.–6 p.m.) and night (6 p.m.–6 a.m.). Significance of differences was assessed using the Kruskal–Wallis test. For PM2.5, weekend concentrations were higher than weekday concentrations at all four sites, though the difference is not statistically significant at Edison and Alsup. This result was surprising, as traffic and industrial activity leading to emissions of PM2.5 and its precursors are expected to be higher on weekdays than weekends. In contrast to the current study, the Denver Aerosol Sources and Health study (DASH; Vedal et al. Citation2009), which performed daily PM2.5 filter sampling for 4.5 years at Palmer Elementary School in Denver, found a significantly higher weekday median (7.1 μg m−3) than weekend median (6.5 μg m−3) (unpublished statistics). We do not yet have an explanation for the discrepancy.
TABLE 3 Comparison of median 1-h average concentrations by weekday/weekend and day/night
In contrast to PM2.5, concentrations of PM10–2.5 followed the expected pattern, with weekday concentrations found to be uniformly significantly higher than weekend concentrations. Likewise, Harrison et al. (Citation2001) reported higher PM10–2.5 concentrations on weekdays than weekends for two sites in London and across all seasons. As can be shown in , daytime concentrations of PM2.5 were higher than nighttime concentrations at Edison and McAuliffe, whereas the opposite was true for Alsup. Maplewood shows no statistically significant difference between day and night concentrations, though the nighttime median is slightly larger. Daytime concentrations of PM10–2.5 were significantly higher than nighttime concentrations at all four sampling sites. The diurnal patterns underlying these results are discussed below.
shows the median hourly concentrations of PM2.5 and PM10–2.5 for each monitoring location. Across all four locations, median hourly average concentrations of PM2.5 were less variable as a function of time of day than median PM10–2.5 concentrations. PM2.5 concentrations at all sites generally increased in the morning from about 6 to 10 a.m., decreased during the afternoon, and then increased again in the evening. Relatively high PM2.5 concentrations in the morning hours are likely due to temperature inversions in addition to source activity. The Alsup site showed the strongest peak in PM2.5 concentrations. This peak occurred at 7 a.m., slightly earlier than the morning peaks at the other sites.
FIG. 1 Median mass concentrations (μg m−3) by time of day at the 4 monitoring sites for (a) PM2.5 and (b) PM10–2.5.
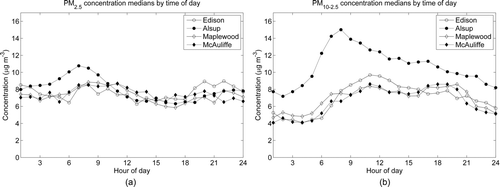
FIG. 2 Monthly median mass concentrations (μg m−3) at the 4 monitoring sites for (a) PM2.5 and (b) PM10–2.5.
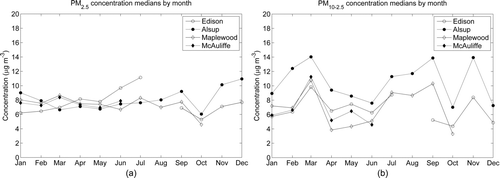
PM10–2.5 concentrations at Alsup peaked at about 8 a.m. and then declined until about 2 a.m. For both PM2.5 and PM10–2.5, the relatively pronounced morning peaks at Alsup appear to reflect the influence of relatively heavy industrial activity and traffic at this particular location. At Edison, PM10–2.5 concentrations peak at 11 a.m. and then decline relatively steadily until 4 a.m. The PM10–2.5 concentrations at the two Greeley sites were higher during the daytime hours than at night, but do not exhibit the afternoon decrease seen for PM10–2.5 in Denver and for PM2.5 at all locations. This suggests that the strength of PM10–2.5 sources affecting the Greeley monitors increases in the afternoon, roughly compensating for the enhanced dilution that occurs as the mixing layer grows. The finding that PM10–2.5 exhibits relatively strong diurnal variability compared to PM2.5 is consistent with the shorter residence time of PM10–2.5 in the atmosphere. Harrison et al. (Citation2001) and Moore et al. (Citation2010) similarly found elevated PM10–2.5 concentrations during daytime hours, at monitoring sites in London and the Los Angeles area, respectively.
shows the median concentrations of PM2.5 and PM10–2.5 plotted by month. In each size regime, the four sites showed similar monthly patterns. PM2.5 concentrations showed relatively little monthly variation compared to concentrations of PM10–2.5. The highest median concentrations of PM10–2.5 were measured during July, August, and September as well as select winter months. This is consistent with the expectation that PM10–2.5 is partly derived from resuspension processes that are enhanced under dry and windy conditions. Additional seasonal analysis will be performed when the 3-year time series of concentrations is complete.
3.3 NPR Results
NPR results showing relationships of hourly PM2.5 and PM10–2.5 concentrations with wind speed and wind direction are presented for each site in –. In each figure, the top panels show scatter plots of concentration versus wind direction and wind speed, and the bottom panels show the NPR results. The dark center line represents the values predicted by the regression (C(θ); where θ is the wind direction or speed), and the lighter lines are the 95% confidence intervals based on the assumption that predicted values are means of normal distributions at each θ value. Wind direction data are arranged clockwise from north at 0°. Note that the hourly average concentrations shown in these figures reach sharply higher values than the 24-h average concentrations summarized in . As reflected in the wider confidence limits for high wind speeds in some cases, limited data density influenced the curve shapes in these regions.
FIG. 3 NPR results for (a) PM2.5 (n = 4028) and (b) PM10–2.5 (n = 4028) mass concentrations (μg m−3) at Edison, showing relationships with wind direction (degrees clockwise from north) and wind speed (ms−1).
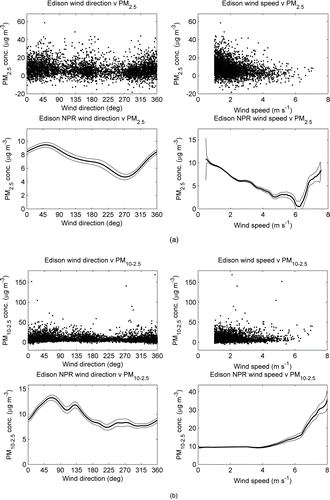
FIG. 4 NPR results for (a) PM2.5 (n = 7626) and (b) PM10–2.5 (n = 7496) mass concentrations (μg m−3) at Alsup, showing relationships with wind direction (degrees clockwise from north) and wind speed (ms−1).
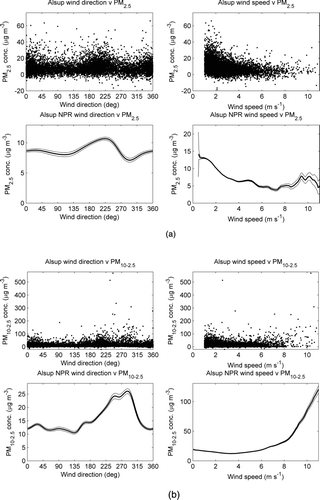
FIG. 5 NPR results for (a) PM2.5 (n = 2930) and (b) PM10–2.5 (n = 2930) mass concentrations (μg m−3) at Maplewood, showing relationships with wind direction (degrees clockwise from north) and wind speed (ms−1).
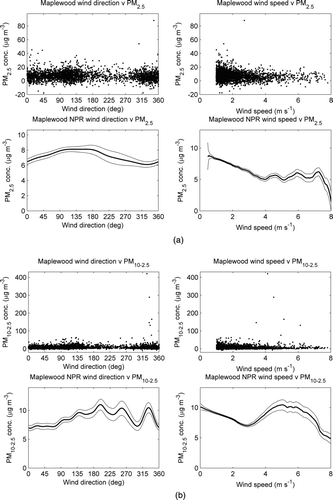
FIG. 6 NPR results for (a) PM2.5 (n = 2262) and (b) PM10–2.5 (n = 2262) mass concentrations (μg m−3) at McAuliffe, showing relationships with wind direction (degrees clockwise from north) and wind speed (ms−1).
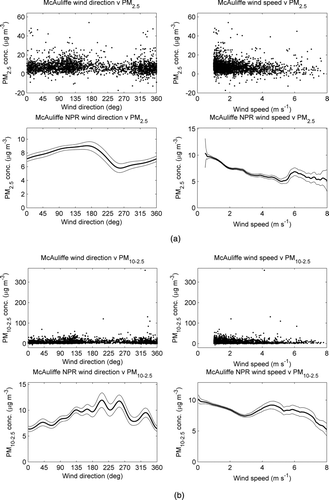
The NPR results for the Edison site () show higher concentration estimates for both size fractions when the wind is from the northeast. Estimated PM2.5 concentrations at Alsup peak with winds from the southwest (). Estimated PM10–2.5 concentrations at Alsup are markedly higher with winds from the west (). The wind direction NPR results for both pollutants at both Denver sites point toward the more densely populated, highly traveled, and industrialized core of the city as a significant source area. There are no known major point sources near the Edison site, but the westerly peak in the NPR results for PM10–2.5 at Alsup is likely influenced by a sand and gravel operation 1 km west of the monitor and a major interstate highway junction (I-76, I-270, I-25, and US-36) just west of that.
The Maplewood and McAuliffe sites have similar NPR results ( and ). The NPR results show that PM2.5 concentrations are highest with southerly winds ( and ). The NPR results for PM10–2.5 for both Greeley sites show peaks corresponding to winds from the west and southwest ( and ). A third peak corresponding to winds from the northwest is more pronounced in the results for Maplewood than those for McAuliffe. The predicted value centered on this peak is influenced by multiple data points with concentrations exceeding 100 μg m−3. When these values are omitted from the NPR, the third peak is no longer apparent for either Maplewood or McAuliffe. We have no basis for excluding these data points; the northwesterly influence appears to be a real effect on the data sets. The densely populated portion of Colorado's Northern Front Range extends from metropolitan Denver, which is south–southwest of Greeley, to Fort Collins, which is northwest of Greeley. Emissions from these source areas may account for the peaks in and . We are not aware of nearby point sources that are south, southwest, or northwest of the Greeley monitoring sites.
Across all four sites, the NPR results for PM2.5 dependence on wind speed show a general dilution effect with increasing wind speed. The NPR results for Edison and Alsup show increased PM2.5 concentrations with wind speeds above 6–7 ms−1. This could be due to resuspension processes or uncertainty associated with low data density. Additional data in this wind speed range will help in interpreting this relationship.
Results for PM10–2.5 are more complicated than those for PM2.5. The PM10–2.5 wind speed regressions for the two Denver sites ( and ) suggest resuspension effects, with concentrations increasing with wind speeds up to 8 ms−1 at Edison and 10 ms−1 at Alsup. The PM10–2.5 concentrations at the two Greeley sites ( and ) show relatively complex wind speed dependence. They decrease for wind speeds up to about 3 ms−1, increase with wind speeds between 3 and 5 ms−1, and then decrease again at higher wind speeds. In comparison, Harrison et al. (Citation2001) found a U-shaped curve for the wind speed dependence of PM10–2.5 mass concentration measurements taken near a roadway in Birmingham Hodge Hill, England, suggesting dilution at wind speeds below about 4 ms−1 and resuspension at higher wind speeds. Moore et al. (Citation2010) found positive correlation between PM10–2.5 concentration and wind speed for three Los Angeles area sites during the dry seasons, but negligible or negative correlation in winter. Once additional data are available, seasonal analysis and consideration of additional meteorological variables related to resuspension could assist in interpreting the relationship between wind speed and PM10–2.5 concentrations at our monitoring sites.
3.4 Comparison of 24-h Average Mass Concentrations and Spatial Correlation with other Locations
The State of Colorado (CDPHE) reports mass concentrations of PM2.5 and PM10 from two urban monitoring sites in Denver: the CAMP site at 2105 Broadway (lat. 39.75, long. −104.99) and the Denver Municipal Animal Shelter (DMAS) site at 678 S. Jason Street (lat. 39.70, long. −105.00). Each site houses a TEOM 1400a equipped with an FDMS unit (Series 8500 FDMS, Thermo Scientific) for continuous PM2.5 monitoring and a TEOM 1400AB without an FDMS unit for PM10 monitoring. We obtained data from 1/1/2009 to 2/28/2010 for these monitoring sites from CDPHE and estimated PM10–2.5 concentrations by subtraction. Descriptive statistics for both size ranges were calculated for comparison with results from the four CCRUSH TEOM sites. For the given time period, the mean 24-h average PM2.5 concentration at CAMP was 8.3 μg m−3, with a 95th percentile value of 16.6 μg m−3 and COV of 0.5. The mean PM2.5 concentration at DMAS was 11.1 μg m−3, with a 95th percentile value of 19.6 μg m−3 and COV of 0.4. The mean PM2.5 concentration at CAMP thus falls within the range observed at our 4 monitoring sites, while the concentration at DMAS is about 20%–40% higher than the mean concentrations we observed. The Pearson's R correlation coefficient for 24-h average PM2.5 concentrations at the two CDPHE monitors, separated by 5.1 km, was 0.82. Correlation coefficients for 24-h average PM2.5 concentrations at the CDPHE monitors paired with our Denver monitors ranged from 0.69 between Alsup and DMAS (14.7 km apart) to 0.87 between Edison and DMAS (8.8 km apart).
The mean 24-h average PM10–2.5 concentration at CAMP was 15.7 μg m−3, with a 95th percentile value of 27.5 μg m−3 and COV of 0.4. The mean PM10–2.5 concentration at DMAS was 12.9 μg m−3, with a 95th percentile value of 28.4 μg m−3 and COV of 0.7. PM10–2.5 concentrations at the CDPHE sites are thus comparable to those we observed at Alsup, and higher than those observed at our other study sites. The Pearson's R correlation coefficient for 24-h average PM10–2.5 concentrations at the two CDPHE monitors was 0.61. Correlation coefficients for PM10–2.5 concentrations at the CDPHE monitors paired with our Denver monitors range from 0.60 between Alsup and CAMP (9.7 km apart) to 0.83 between Edison and DMAS (8.8 km apart).
U.S. EPA (Citation2009) presents the distributions of 24-h average PM2.5 and PM10–2.5 mass concentrations measured from 2005 to 2007 from FRM monitors across the country that report to the agency's Air Quality System. For PM2.5, the national mean 24-h average concentration was 12 μg m−3 and the 5th and 95th percentile values were 4 and 28 μg m−3, respectively, based on nearly 350,000 observations. For Denver, U.S. EPA (Citation2009) reports a mean 24-h average PM2.5 concentration during 2005–2007 of 9 μg m−3 and 5th and 95th percentile values of 3 and 18 μg m−3, respectively, based on 4192 observations. The results from the CDPHE monitors discussed above and from the TEOM sampling conducted in this study during 2009–2010 show similar mean PM2.5 concentrations to those EPA reports for Denver, but with greater variability.
For PM10–2.5, U.S. EPA (Citation2009) reported concentrations estimated from colocated monitors using low-volume FRM filter samplers from 2005 to 2007. The national mean 24-h average PM10–2.5 concentration was 13 μg m−3 with 5th and 95th percentile values of 1 and 33 μg m−3, respectively, based on just over 12,000 observations. For PM10–2.5 in Denver, EPA reported a mean concentration of 20 μg m−3 with 5th and 95th percentile values of 4 and 42 μg m−3, based on 353 observations. In comparison to the values U.S. EPA (Citation2009) reports for Denver, the results from the CDPHE monitoring discussed above and the results from our study suggest lower mean PM10–2.5 concentrations. Differences could be due to differences in sampling methods and monitoring locations or changes in pollutant levels over time.
As mentioned in Section 1, concentrations of PM10–2.5 have generally been expected to be more variable than those of PM2.5. The results from this study indicate that 24-h average PM10–2.5 concentrations are somewhat more temporally variable than those for PM2.5, with coefficients of variation ranging from 0.6 to 0.8 for PM10–2.5 and near 0.5 for PM2.5. The data from CDPHE show a comparatively low COV for PM10–2.5 concentrations at CAMP. For both size classes, spatial correlation was relatively strong for the two monitors in Greeley, compared to the monitors located in Denver. The correlation coefficient of 0.97 for PM10–2.5 concentrations from the 2 Greeley locations, which are located 4.5 km apart, is also relatively high compared to correlation coefficients reported for pairs of PM10–2.5 monitors in other cities, including those with similar separation distances (Wilson et al. Citation2005; U.S. EPA Citation2009; Pabkin et al. 2010). The high correlation of PM10–2.5 for the Greeley monitors suggests the impact of regional sources and/or meteorological influences, rather than local sources.
uast_a_607478_sup_20667589.zip
Download Zip (42.1 KB)Acknowledgments
The CCRUSH study is funded by the National Center for Environmental Research (NCER) of the United States Environmental Protection Agency, under grant number R833744. We wish to thank this program for their support. We also thank and acknowledge assistance from Kelly Albano at the University of Colorado at Boulder; Patrick McGraw and Bradley Rink at the Colorado Department of Public Health and Environment; Debbie Bowe and Maria Johncox at Thermo Scientific; Greg Philp at Weld County School District 6; Phil Brewer at Weld Country Public Health; and all administrative and custodial staff at the elementary schools that serve as the monitoring sites for the CCRUSH study.
[Supplementary materials are available for this article. Go to the publisher's online edition of Aerosol Science and Technology to view the free supplementary files.]
Notes
An urban area consists of core census block groups or blocks that have a population density of at least 1,000 people per square mile (386 people/km2) and surrounding census blocks that have an overall density of at least 500 people per square mile (193 people/km2) (U.S. Census, Citation2000).
REFERENCES
- Boreson , J. , Dillner , A. M. and Peccia , J. 2004 . Correlating Bioaerosol Load with PM2.5 and PM10cf Concentrations: A Comparison Between Natural Desert and Urban-Fringe Aerosols . Atmos. Environ. , 38 : 6029 – 6041 .
- Brunekreef , B. and Forsberg , B. 2005 . Epidemiological Evidence of Effects of Coarse Airborne Particles on Health . Eur. Respir. J. , 26 : 309 – 318 .
- Castillejos , M. , Borja-Aburto , V. H. , Dockery , D. , Gold , D. R. and Loomis , D. 2000 . Airborne Coarse Particles and Mortality . Inhal. Toxicol. , 12 : 61 – 72 . http://informahealthcare.com/doi/abs/10.1080/089583700196392
- Chan , T. L. and Lippmann , M. 1980 . Experimental Measurements and Empirical Modelling of the Regional Deposition of Inhaled Particles in Humans . Am. Ind. Hyg. Assoc. J. , 41 : 399 – 409 .
- Chen , F. , Williams , R. , Svendsen , E. , Yeatts , K. , Creason , J. , Scott , J. , Terrell , D. and Case , M . 2007 . Coarse Particulate Matter Concentrations from Residential Outdoor Sites Associated with the North Carolina Asthma and Children's Environment Studies (NC-ACES) . Atmos. Environ. , 41 : 1200 – 1208 .
- Duce , R. A. , Hoffman , G. L. , Ray , B. J. , Fletcher , I. S. , Wallace , G. T. , Fasching , J. L. , Piotrowicz , S. R. , Walsh , P. R. , Hoffman , E. J. , Millner , J. M. and Heffter , J. L. 1976 . “ Trace Metals in the Marine Atmosphere: Sources and Fluxes ” . In Marine Pollutant Transfer , Edited by: Windom , H. L. and Duce , R. A. 77 – 119 . Lexington, MA : D.C. Heath .
- Green , D. , Fuller , G. W. and Baker , T. 2009 . Development and Validation of the Volatile Correction Model for PM10—An Empirical Method for Adjusting TEOM Measurements for Their Loss of Volatile Particulate Matter . Atmos. Environ. , 43 : 2132 – 2141 .
- Grover , B. D. , Kleinman , M. , Eatough , N. L. , Eatough , D. J. , Hopke , P. K. , Long , R. W. , Wilson , W. E. , Meyer , M. B. and Ambs , J. L. 2005 . Measurement of Total PM2.5Mass (Nonvolatile Plus Semivolatile) with the Filter Dynamic Measurement System Tapered Element Oscillating Microbalance Monitor . J.Geophys. Res. , 110 : D07S03 doi:10.1029/2004JD004995
- Harrison , R. M. , Yin , J. , Mark , D. , Stedman , J. , Appleby , R. S. , Booker , J. and Moorcroft , S. 2001 . Studies of the Coarse Particle (2.5–10 μm) Component in UK Urban Atmospheres . Atmos. Environ. , 35 : 3667 – 3679 .
- Henry , R. , Norris , G. A. , Vedantham , R. and Turner , J. R. 2009 . Source Region Identification Using Kernel Smoothing . Environ. Sci. Technol. , 43 : 4090 – 4097 .
- Henry , R. C. , Chang , Y. and Spiegelman , C. H. 2002 . Locating Nearby Sources of Air Pollution by Nonparametric Regression of Atmospheric Concentrations on Wind Direction . Atmos. Environ. , 36 : 2237 – 2244 .
- Hueglin , C. , Gehrig , R. , Baltensperger , U. , Gysel , M. , Monn , C. and Vonmont , H. 2005 . Chemical Characterization of PM2.5, PM10 and Coarse Particles at Urban, Near-City and Rural Sites in Switzerland . Atmos. Environ. , 39 : 637 – 651 .
- Kim , E. and Hopke , P. H. 2004 . Comparison Between Conditional Probability Function and Nonparametric Regression for Fine Particle Source Directions . Atmos. Environ. , 38 : 4667 – 4673 .
- Loo , B. W. and Cork , C. P. 1988 . Development of High Efficiency Virtual Impactors . Aerosol Sci. Technol. , 9 : 167 – 176 .
- Mar , T. F. , Norris , G. A. , Koenig , J. Q. and Larson , T. V. 2000 . Associations Between Air Pollution and Mortality in Phoenix . Environ. Health Perspect. , 108 : 1995 – 1997 . 347 – 353 .
- Marple , V. A. and Chien , C. M. 1980 . Virtual Impactors: A Theoretical Study . Environ. Sci. Technol. , 14 : 976 – 985 .
- Milford , J. B. and Davidson , C. I. 1985 . The Sizes of Particulate Trace Elements in the Atmosphere—A Review . J. Air Pollut. Control Assoc. , 35 : 1249 – 1260 .
- Milford , J. B. and Davidson , C. I. 1987 . The Sizes of Particulate Sulfate and Nitrate in the Atmosphere—A Review . J. Air Pollut. Control Assoc. , 37 : 125 – 134 .
- Moore , K. F. , Verma , V. , Minguillon , M. C. and Sioutas , C. 2010 . Inter- and Intra-Community Variability in Continuous Coarse Particulate Matter (PM10-2.5) Concentrations in the Los Angeles Area . Aerosol Sci. Technol. , 44 : 526 – 540 .
- Ostro , B. D. , Broadwin , R. and Lipsett , M. J. 2000 . Coarse and Fine Particles and Daily Mortality in the Coachella Valley, California: A Follow-Up Study . J. Expo. Anal. Environ. Epidemiol. , 10 : 412 – 419 .
- Pakbin , P. , Hudda , N. , Cheung , K. L. , Moore , K. F. and Sioutas , C. 2010 . Spatial and Temporal Variability of Coarse (PM10-2.5) Particulate Matter Concentrations in the Los Angeles Area . Aerosol Sci. Technol. , 44 : 514 – 525 .
- Patterson , E. and Gillette , D. 1977 . Commonalities in Measured Size Distributions for Aerosols Having a Soil-Derived Component . J. Geophys. Res. , 82 : 2074 – 2082 .
- Schwab , J. J. , Felton , H. D. , Rattigan , O. V. and Demerjian , K. L. 2006 . New York State Urban and Rural Measurements of Continuous PM2.5 Mass by FDMS, TEOM, and BAM . J. Air Waste Manage. Assoc. , 56 : 372 – 383 .
- Solomon , P. A. and Sioutas , C. 2008 . Continuous and Semicontinuous Monitoring Techniques for Particulate Matter Mass and Chemical Components: A Synthesis of Findings from EPA's Particulate Matter Supersites Program and Related Studies . J. Air Waste Manage. Assoc. , 58 : 164 – 195 .
- Thornburg , J. , Rodes , C. E. , Lawless , P. A. and Williams , R. 2009 . Spatial and Temporal Variability of Outdoor Coarse Particulate Matter Mass Concentrations Measured with a New Coarse Particle Sampler During the Detroit Exposure and Aerosol Research Study . Atmos. Environ. , 43 : 4251 – 4258 .
- Census , U. S. 2000 . United States Census 2000, Urban and Rural Classification . http://www.census.gov/geo/www/ua/ua_2k.html, accessed December 27, 2010
- U.S. Census . 2009a . Table 1. Annual Estimates of the Resident Population for Counties of Colorado: April 1, 2000 to July 1, 2009 . 2009 Population Estimates. United States Census Bureau, Population Division. http://www.census.gov/popest/counties/counties.html, accessed December 27, 2010
- U.S. Census . 2009b . Table 4. Annual Estimates of the Resident Population for Incorporated Places in Colorado: April 1, 2000 to July 1, 2009 . 2009 Population Estimates. United States Census Bureau, Population Division. http://www.census.gov/popest/cities/SUB-EST2009-states.html, accessed December 27, 2010
- U.S. Census . 2009c . Table 1. Annual Estimates of the Resident Population for Incorporated Places Over 100,000, Ranked by July 1, 2009 Population: April 1, 2000 to July 1, 2009 . 2009 Population Estimates. United States Census Bureau, Population Division. http://www.census.gov/popest/cities/SUB-EST2009.html, accessed December 27, 2010
- EPA , U. S. 1995 . AP 42 Fifth Edition: Compilation of Air Pollutant Emission Factors, Vol. 1: Stationary Point and Area Sources , Research Triangle Park , NC : U.S. Environmental Protection Agency, Office of Air Quality Planning and Standards .
- U.S. EPA . 2004 . Air Quality Criteria for Particulate Matter. EPA/600/P-99/002aF-bF , Research Triangle Park , NC : U.S. Environmental Protection Agency .
- EPA , U. S. 2009 . Integrated Science Assessment for Particulate Matter. EPA/600/R-08/139F , Washington, DC : U.S. Environmental Protection Agency .
- U.S. EPA . 2010 . List of Designated Reference and Equivalence Methods, U.S. Environmental Protection Agency, Research Triangle Park, NC . Available online at: http://www.epa.gov/ttn/amtic/files/ambient/criteria/reference-equivalent-methods-list.pdf, accessed December 29, 2010
- Vedal , S. , Hannigan , M. P. , Dutton , S. J. , Miller , S. L. , Milford , J. B. , Rabinovitch , N. , Kim , S.-Y. and Sheppard , L. 2009 . The Denver Aerosol Sources and Health (DASH) Study: Overview and Early Findings . Atmos. Environ. , 43 : 1666 – 1673 .
- Villeneuve , P. J. , Burnett , R. T. , Shi , Y. , Krewski , D. , Goldberg , M. S. , Hertzman , C. , Chen , Y. and Brook , J. 2003 . A Time-Series Study of Air Pollution, Socioeconomic Status, and Mortality in Vancouver, Canada . J. Expo. Anal. Environ. Epidemiol. , 13 : 427 – 435 .
- Wilson , J. G. , Kingham , S. , Pearce , J. and Sturman , A. P. 2005 . A Review of IntraurbanVariations in Particulate Air Pollution: Implications for Epidemiological Research . Atmos. Environ. , 39 : 6444 – 6462 .
- Yu , K. N. , Cheung , Y. P. , Cheung , T. and Henry , R. C. 2004 . Identifying the Impact of Large Urban Airports on Local Air Quality by Nonparametric Regression . Atmos. Environ. , 38 : 4501 – 4507 .
- Zanobetti , A. and Schwartz , J. 2009 . The Effect of Fine and Coarse Particulate Air Pollution on Mortality: A National Analysis . Environ. Health Perspect. , 117 : 898 – 903 .
- Zhu , K. , Zhang , J. and Lioy , P. J. 2006 . Evaluation and Comparison of Continuous Fine Particulate Matter Monitors for Measurement of Ambient Aerosols . J. Air Waste Manage. Assoc. , 57 : 1499 – 1506 .