Abstract
Nanoparticle agglomerates play an essential role in the manufacturing of many nanomaterials and are commonly found in combustion products. Conventional aerosol instruments based on equivalent spheres are not directly applicable to the measurement of nanoparticle agglomerates. The increasing interest in real-time assessment of the structure of engineered nanoparticle agglomerates and the mass concentration of potentially hazardous agglomerates (e.g., diesel soot, welding fume) makes an instrument devoted to online structure and mass measurements for nanoparticle agglomerates highly desirable. A recently developed instrument, universal nanoparticle analyzer (UNPA), utilizes the close relation between agglomerate structure and unipolar charging properties and infers agglomerate structure from measurement of the average charge per agglomerate. It was used in this study to characterize in situ the structure of metal nanoparticle agglomerates generated by spark discharge, to study the effects of sintering on the structure of these agglomerates, and to make real-time assessment of their airborne mass concentration. The primary particles sizes measured by UNPA for the gold (Au), silver (Ag), and nickel (Ni) agglomerates are in reasonable agreement with the TEM (transmission electron microscopy) sizing results, d p = 7.9 ± 1.5, 11.8 ± 3.2, and 6.6 ± 1.0 nm, respectively. In addition, findings from the study of agglomerate structural change during sintering using the UNPA sensitivity coincide with results from TEM and mobility analyses. With regard to the mass concentration of silver agglomerates at room temperature, good agreement was found under our experimental conditions between results given by UNPA, the effective density, and the gravimetric measurement.
Copyright 2012 American Association for Aerosol Research
INTRODUCTION
A variety of engineered nanomaterials, such as carbon black, fumed silica, and titania, are produced in the form of nanoparticle agglomerates by combustion (Medalia and Heckman Citation1969; Pratsinis Citation1998; Stark and Pratsinis Citation2002). Nanoparticle agglomerates are also generated from the burning of biomass and diesel fuel (Park et al. Citation2004; Chen et al. Citation2005; Gwaze et al. Citation2006), and are abundant in welding fumes (Jenkins et al. Citation2005). The particles formed in these high-temperature processes are commonly found to grow from nearly spherical primary particles into fractal-like agglomerates through aggregation. Their primary particle size is often in the range of several to tens of nanometers, while the agglomerate itself may be of the order of micrometers. This has profound implications on particle properties, including light scattering, surface chemistry, transport, and potential health effects. As to the commercial manufacturing of nanomaterials, agglomerate morphology and primary particle size are of direct importance to product quality.
Consequently, characterization of agglomerate structure has received extensive attention. Light scattering is used to measure agglomerate radius of gyration, fractal dimension, and number of primary particles per agglomerate (Puri et al. Citation1993; Oh and Sorensen Citation1997). Transmission electron microscopy (TEM) proves a useful tool for examining agglomerate morphology, as discussed in the works of Samson et al. (Citation1987), Koylu et al. (Citation1995), Park et al. (Citation2004), and so forth. It can provide direct measurements of primary particle size and agglomerate 2D fractal dimension, and a complementary measurement of agglomerate 3D fractal dimension.
An increasing number of aerosol instruments and techniques are available for measurements of agglomerate concentration and size distributions. The condensation particle counter (CPC) delivers real-time measurement of particle number concentration. When it is combined with a differential mobility analyzer (DMA), the particle mobility size distribution can be established through a voltage scan; the whole system often called by the commercial name – scanning mobility particle sizer (SMPS). Other variations of the system include the electrical aerosol spectrometers (EEPS and FMPS, TSI Inc.). With respect to particle surface area, the nanoparticle surface area monitor (NSAM, TSI, Inc.) can estimate the surface area of inhaled particles deposited in the lung by measuring its unipolar charging current. The Brunauer–Emmett–Teller (BET) method determines the so-called BET surface area of a particle by adsorption of nitrogen on particle surfaces at the boiling temperature of liquid nitrogen (77 K). Lastly, inductively coupled plasma optical emission spectrometry (ICP-OES) has been applied to measure the mass concentration of agglomerated metal particles (Weber et al. Citation1996). For both metal and nonmetal particles of irregular shape, mass concentration is commonly determined through filter collection. One most important example is the assessment of diesel engine particulate matter (PM) emissions. Unfortunately, not only is the gravimetric method cumbersome and unsuitable for providing real-time results, but it also has inherent difficulties dealing with PM emissions at very low levels. The latter drawback in particular could pose a practical limitation as the regulations on diesel PM emissions become increasingly stringent (Maricq and Xu Citation2004). Therefore, a real-time and sensitive measurement technique for agglomerate mass concentration is highly desirable.
Kelly and McMurry (Citation1992), Park et al. (Citation2003), and Maricq and Xu (Citation2004) introduced “effective density” in the search of a way to perform mass measurement on a mobility spectral instrument, such as SMPS. In their studies, the effective density of given types of agglomerates was determined from the mass–mobility relationship measured by tandem DMA-ELPI (differential mobility analyzer-electrical low pressure impactor) or DMA-APM (-aerosol particle mass analyzer) methods. The mass distribution of these agglomerates can be obtained then by simple calculations based on the measured number size distribution.
An alternative to the aforementioned techniques of agglomerate structural characterization and distribution/concentration measurement is an instrumental system called universal nanoparticle analyzer (UNPA), which was recently developed by Wang and coworkers (Citation2010). The instrument is designed to evaluate in-situ agglomerate structural parameters, such as the primary particle size, from combined measurements of electrical mobility and unipolar charging efficiency. It can be used in estimating the “compactness” of agglomerates relative to spheres with the same mobility diameter, since particle unipolar charging properties were found to be morphology dependent (Oh et al. Citation2004; Shin, Wang et al. Citation2010). Moreover, real-time assessment of the mass distribution and concentration of loose agglomerates can be made using the Lall–Friedlander theory (Citation2006) after the primary particle size is determined, provided particle material density is known. As this method of mass evaluation is based on the number distribution of agglomerates, it has a good sensitivity in principle.
In the present study, UNPA was employed to characterize the structure of metal nanoparticle agglomerates formed by spark discharge (similar to agglomerates found in the welding fume) and study its changes in the sintering process. In addition, real-time measurements were conducted using UNPA for the mass concentration of loose agglomerates without sintering after production, and the results were compared with those obtained by the effective density and the filter collection methods. Two themes are pursued throughout the paper. One is aimed at a deeper understanding of the structure and the sintering of metal nanoparticle agglomerates generated by spark discharge facilitated by the use of UNPA. The second focuses on the performance evaluation of this new instrument through validating experiments, such as TEM analysis for agglomerate structure and filter collection for agglomerate mass concentration.
THEORETICAL BACKGROUND
Among all types of agglomerates, an important limiting case, agglomerates consisting of spherical primary particles of nearly uniform size, has been studied extensively by numerous investigators. Such agglomerates can be modeled as idealized agglomerates, i.e. the primary particles are rigid spheres with point contacts and constant size. Moreover, an agglomerate having fractal dimension (Forrest and Witten Citation1979; Mandelbrot Citation1982; Schmidt-Ott Citation1988), D f, less than 2 is often referred to as the loose agglomerate or the chain agglomerate, because in the extreme case, it resembles a straight chain with occasional branches. The primary particles in a loose agglomerate are “transparent” to one another and are equally exposed to the surrounding gases. Based on the free-molecule drag formula of Chan and Dahneke (Citation1981), Lall and Friedlander (Citation2006) developed a mobility model for idealized loose agglomerates with primary particle size in the free molecular regime (Knudsen number, Kn = 2λ/d p ≥ 10):
Theoretical evaluation of the unipolar and bipolar charging efficiencies for particle agglomerates is a subject of fair amount of difficulty. No completely definite theories have yet been developed. In this study, we employ the theory of Chang (Citation1981) for unipolar charging of arbitrary-shaped particles, and the theory of Wen et al. (Citation1984) for bipolar charging of chain-like agglomerates. The former is used to derive the UNPA sensitivity correlation and to compute from it the primary particle size, as discussed below; the latter is found in the calculation of agglomerate number size distribution in “Results and Discussion.”
The UNPA consists primarily of a DMA, a CPC, and an NSAM (Fissan et al. Citation2007), as illustrated by the schematic diagram in . Polydisperse test aerosols are first mobility-selected by the DMA, the exiting monodisperse particles are then sampled simultaneously by the CPC and the NSAM after proper dilution. The CPC gives readings of the particle number concentration, while the NSAM measures the electric current of the particles after they are passed through its internal unipolar diffusion charger (corona needle). The UNPA sensitivity, S, is defined as the aerosol current divided by the number concentration, S = I/n (fA-cm3). It is a measure of the average charge per particle for a fixed aerosol sampling flow rate. The assumption of same particle concentration in the CPC and the NSAM was satisfied in our experiment by having both instruments sample the same monodisperse aerosol from a tee connector using tubings of the same length and type (conductive silicone tubing: inner diameter [ID] 0.48 cm, length 25 cm). From the formula of Gormley and Kennedy (Citation1949), it can be shown that at a sampling flow rate of 1.5 lpm, the particle penetrations through the sampling tubing after diffusional loss are 99.6% and 99.8% for d m = 50 nm and 100 nm, respectively. Furthermore, Medved et al. (Citation2000) reported very high transmission efficiency of the NSAM unipolar charger, which means a marginal effect of internal particle loss on measured sensitivity.
FIG. 1 Schematic diagram of UNPA (Wang et al. Citation2010).
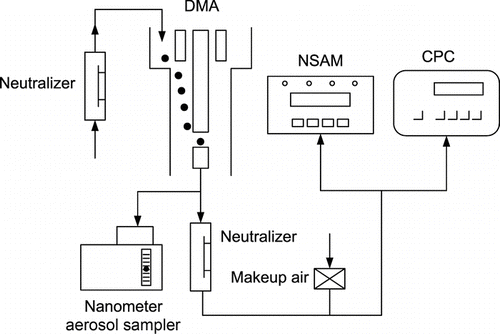
A number of investigators (Medved et al. Citation2000; Wang et al. Citation2010) have shown that the sensitivity of a spherical particle in the range of 10–1000 nm is given by the power law:
Substituting EquationEquations (1) and Equation(4) in EquationEquation (3), we can write the sensitivity of the loose agglomerate as:
If the correlation coefficients, c 1, c 2, h, and k in Equation (3) are known, the primary particle size d p is the only unknown parameter and thus can be obtained by a fitting to the sensitivity data of loose agglomerates. The calibration of UNPA, as will be discussed later, involves determination of the coefficients c 1, h from the sensitivity of spherical particles (e.g. polystyrene latex [PSL] or silver spheres), and c 2, k from that of loose agglomerates of known primary particle size.
EXPERIMENTAL
Special attention is devoted first to the spark-discharge nanoparticle generation. Nanoparticle generation by spark discharge was initially developed by Schwyn et al. (Citation1988). Basically, this technique employs periodic spark discharge to vaporize electrode materials and subsequent nucleation/condensation to form nanoparticles. Therefore, it can be regarded as an evaporation–condensation method which is different from the tube-furnace method in the way of material vaporization. Although the spark-discharge technique has not been studied as extensively as the tube-furnace method, the size and characteristics of spark-generated ultrafine carbon, metal, and metal oxide particles or particle agglomerates have been examined by mobility scanning, TEM and BET methods (Schwyn et al. Citation1988; Watters et al. Citation1989; Roth et al. Citation2004). The metal nanoparticles in our study were generated by a Palas spark generator (model GFG-1000). Its design and operating principle can be found in the paper of Helsper et al. (Citation1993). There are several advantages associated with the use of the spark generator as an ultrafine aerosol source. First, the temperature generated at the instant of spark discharge is much higher than the typical upper limit of a tube furnace. Thus, even materials with very high melting point can be used by a spark generator if they are conductive, which provides us a greater flexibility in testing our instrument. Furthermore, there need be no concern about impurities evaporated from crucibles and tube materials at elevated temperatures. The spark generator in the current study proves capable of generating nanoparticles at high mass output with stable characteristics over many hours.
A schematic diagram of the experimental setup is shown in . The following electrodes for the spark generator have been used: gold (ESPI Metals, 0.125′ diameter, 99.999%), silver (ESPI Metals, 0.125′ diameter, 99.999%) and nickel (ESPI Metals, 0.125" diameter, 99.995%). Spark frequency was set at 1 kHz. Nitrogen (99.999%) was used as the carrier gas at a flow rate of 5 lpm. The nanoparticle number concentration under these conditions was found to be of the order of 108 particles/cm3. These nanoparticles were brought to charge equilibrium by a Po-210 neutralizer before they entered the agglomeration chamber, which was 6.7 L in volume. The aerosol flow rate through the chamber was maintained at 1 lpm, providing a residence time of 6.7 min for aggregation at room temperature (RT) and standard pressure. The aerosol wall loss is roughly estimated for 100-nm silver agglomerates assuming mass per agglomerate to be 1.07 fg, using the data of Kim et al. (Citation2009). The wall loss coefficient β was found to be 4.19 × 10−5 s−1, according to the theory of Crump and Seinfeld (Citation1981) and Equation (12) in the paper of Crump et al. (Citation1983) for the coefficient of eddy diffusivity k e. For particle number concentration of 106 cm−3 in the chamber, it corresponds to a wall loss rate of 42 cm−3 s−1 and particle loss of 1.7 × 104 cm−3 in the residence time of 6.7 min. Major assumptions in the calculation include spherical vessel of equivalent volume and complete mixing of aerosol in the vessel. The 4 lpm excess aerosol exiting the spark generator was discharged into the room air via an HEPA (high-efficiency particulate air) filter. The metal nanoparticle agglomerates formed within the chamber by thermal aggregation were then passed through a sintering furnace (Lindberg/Blue M, model HTF55322A, length of heating zone = 30.5 cm) equipped with a ceramic tube (length = 89 cm, ID = 1.43 cm), whereby the morphology of the agglomerates was controlled. The residence time of the aerosol in the heating zone is 2.94 s at RT. Particles were sampled by UNPA after passing through the sintering furnace.
Two operational modes of UNPA were used in this work: (1) the scanning mode and (2) the sensitivity mode. Mobility size distributions of the metal nanoparticle agglomerates were measured in the scanning mode for a variety of sintering temperatures, with the DMA (TSI model 3081, long-column) and the CPC (TSI model 3775) in the system. This is essentially equivalent to the standard SMPS scan. In the sensitivity mode, the DMA, the CPC and the NSAM were used concurrently to measure the UNPA sensitivity S of mobility-selected agglomerates. The sampling flow rates for the scanning and the sensitivity modes were set at 0.3 lpm and 1 lpm, respectively.
In addition, filter collection experiments were conducted for silver nanoparticle agglomerates at RT (without additional sintering after production). Agglomerates were collected by Teflon membrane filters (SKC Inc., 47-mm diameter, 2.0-μm pore size) at a flow rate of 1.5 lpm. Three samples were taken, each lasted for 1 h. They were weighed on a microbalance (Cahn model C-31, to the accuracy of 1 μg); the collected mass was converted to the airborne mass concentration of the sampled agglomerates. Last but not least, complementary TEM sampling and analysis were performed for each agglomerate type and sintering condition. Particles were deposited on 3-mm-diameter copper grids (Ted Pella Inc., 400 mesh, coated with carbon film) using an electrostatic nanometer aerosol sampler (TSI model 3089). An FEI Tecnai T12 TEM (accelerating voltage: 20–120 kV, magnification: up to 700,000×) was employed to obtain the micrographs.
RESULTS AND DISCUSSION
Projected Properties of Spark-Generated Agglomerates
The projected structural properties of metal nanoparticle agglomerates were studied from the TEM images with the aid of image analysis software. shows the TEM images of polydisperse agglomerates under different sintering temperatures. The metallic agglomerates at RT, such as those in , c and g, are found to meet the criteria for loose agglomerate (i.e., D f < 2) in most cases. We selected primary particles that are clearly distinguishable in the TEM images and measured their diameters using the software, ImageJ (Java application from NIH [National Institutes of Health], version 1.42). The Ag primary particle size distribution is plotted in . It is found to be relatively well described by a Gaussian fitting. Additionally, constant primary particle size is a justified assumption in this case due to the relatively small standard deviation. The Au and Ni primary particles, whose size distributions are not shown here, have similar features. summarizes the primary particle sizes from TEM analysis for all three types of agglomerates under current investigation. The order of the primary particle size from big to small is Ag, Au, and Ni, which happens to correspond to an order of the material melting point from low to high (bulk melting points: Ag = 962°C, Au = 1064°C, Ni = 1453°C).
FIG. 3 TEM images of spark-generated metal nanoparticle agglomerates at different sintering temperatures. (a) Silver agglomerate at RT. (b) Silver agglomerates sintered at 200°C. (c) Gold agglomerates at RT. (d) Gold agglomerates sintered at 200°C. (e) Gold agglomerates sintered at 400°C. (f) Gold agglomerates sintered at 600°C. (g) Nickel agglomerates at RT. (h) Nickel agglomerates sintered at 400°C. (i) Nickel agglomerates sintered at 800°C. (j) Nickel agglomerates sintered at 1000°C. (Continued)
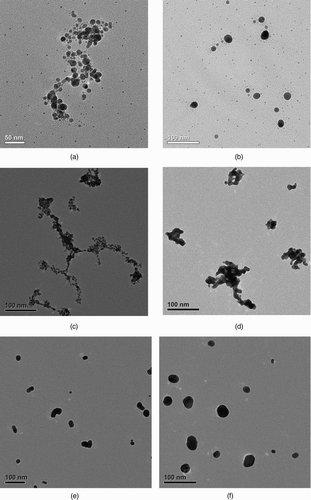
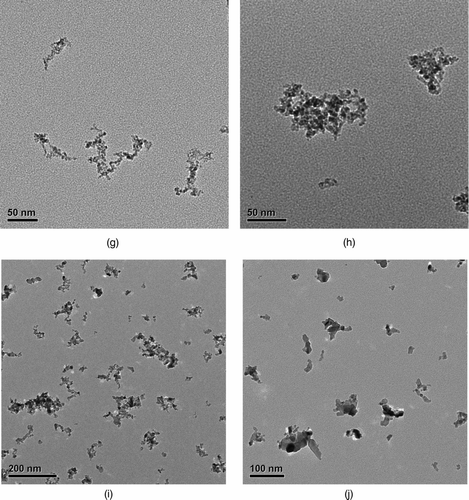
TABLE 1 Summary of primary particle sizes measured by TEM
The change in agglomerate morphology by sintering is evident from the TEM images. The sintering of metal nanoparticle agglomerates generated by spark discharge will be discussed in the next section in the context of electrical mobility and UNPA sensitivity measurements.
Online Characterization of Agglomerate Structure and Effects of Sintering
The mobility size distributions measured by UNPA under the scanning/SMPS mode are shown in for a variety of sintering temperatures. lists the total particle number concentration, the mean diameter, and the geometric standard deviation (σg) for the size distributions shown in . It is noteworthy that particle number concentration reduces with increasing sintering temperature, except for Au agglomerates from 400°C to 600°C. This general trend is most likely the result of particle loss to the tube wall by thermophoresis, which is enhanced at elevated temperatures. The cause for the exception needs to be further investigated.
TABLE 2 Statistics of mobility size distributions for metal nanoparticle agglomerates
FIG. 5 Mobility size distributions of metal nanoparticle agglomerates as a function of sintering temperature: (a) Au, (b) Ni, and (c) Ag.
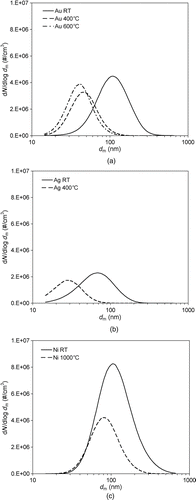
FIG. 6 UNPA sensitivity as a function of mobility diameter for different sintering temperatures: (a) Au, (b) Ag, (c) Ni, and (d) Ni, Au, and Ag at room temperature.
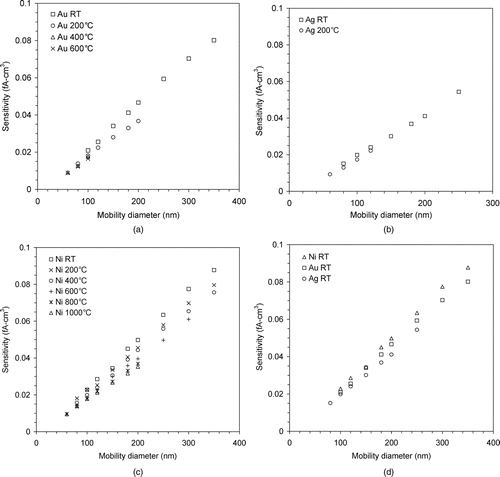
Based on the mobility size scan and the TEM analysis, a qualitative investigation of the agglomerate sintering kinetics is carried out in the first place. In the initial phase of sintering, the change in agglomerate morphology is achieved primarily through thermal restructuring. Above a certain temperature (e.g. ∼150°C for the Au agglomerates in our study), the agglomerate starts to gain enough thermal energy from the surrounding gas molecules to overcome its activation energy threshold for restructuring (Weber and Friedlander Citation1997). Its primary particles rearrange spontaneously as a means to reduce the total free energy, resulting in a more compact agglomerate structure ( and h). The mobility diameter is reduced markedly in this phase and a value for the close-packed agglomerate, d cl, is reached at the end. In the next sintering phase at elevated temperatures, grain boundary diffusion between primary particles starts to accelerate, which leads to fewer number of primary particles and severe necking between them (). Mobility diameter decreases more slowly in this stage. Finally, as the temperature keeps increasing, the elongated spheroidal particle formed in the previous stage fully coalesces into a sphere ( and f) through the rearrangement of internal molecules and the action of surface tension. The mobility diameter hardly changes in this stage. It is frequently found, as was in our case, that the sintering of nanoparticle agglomerates starts well below the material bulk melting point, and that the temperature range corresponding to each sintering phase depends on particle type, size, and surface conditions. In our experiment, complete sintering was observed at about 400°C and 600°C, respectively, for the majority of Ag and Au agglomerates. But most Ni particles shown by our TEM images were not spheres even at 1000°C.
A more careful examination of the sintering process is facilitated by UNPA. The UNPA sensitivity of mobility-classified metallic agglomerates was measured for each sintering temperature – the results plotted in as a function of mobility diameter. Each data point is the average value of three measurements, in each of which the sensitivity was recorded once per second for 30 s. Note that as the relative standard deviation of the measured sensitivity is very small (commonly of the order of 10−3), the error bars are actually shorter than the data symbols. Mobility diameters for the data points were selected after the mode of the size distribution was selected to reduce multiply charged particles. The maximum mobility size for which the sensitivity measurement was taken is constrained by both the range of the DMA and the concentration of monodisperse aerosol. It is shown in that the UNPA sensitivity universally increases with the mobility diameter for all particle types and sintering temperatures. According to the charging theory of Chang (Citation1981), the greater electrical capacitance and surface area of a bigger particle (agglomerate or sphere) allow it to acquire more charge on average than a smaller one under the same unipolar charging conditions. The increase in sensitivity with particle size is also evident in EquationEquations (2) and Equation(5).
Besides, reveals a monotonic decrease in the UNPA sensitivity with increasing sintering temperature for a given agglomerate type and mobility size. This effect mainly results from the change in particle structure. We have shown earlier that agglomerate restructuring associated with temperature increase leads to a more compact cluster. Brown and Hemingway (Citation1995) proved that a loose agglomerate has larger capacitance than a close-packed cluster comprising equal number of primary spheres. Following their approach Shin, Wang et al. (Citation2010) calculated the capacitance of agglomerates with different configurations and showed that this conclusion is valid for comparison based on the same mobility diameter too. Thus, for a given mobility diameter, agglomerates sintered at higher temperatures will have a smaller capacitance and a lower UNPA sensitivity. The sensitivity of Ni agglomerates was measured at several additional sintering temperatures besides those in the TEM sampling and the mobility scanning experiments. Based on the Ni sensitivity data, a quantitative characterization is performed for the structural change in Ni agglomerates during sintering, which is as follows.
The Ni sensitivity data presented in as a function of mobility diameter are replotted in versus the sintering temperature. It can be seen that the sensitivity of Ni agglomerates at d m = 100 nm is almost constant in the measured sintering temperature range. This observation is supported by the calculation of Shin, Wang et al. (Citation2010) showing that at d m = 100 nm, the difference in capacitance is roughly less than 15% between a sphere and a branched loose agglomerate with d p = 19.5 nm. Despite the relatively constant sensitivity of 100-nm Ni agglomerates in the tested sintering temperature range, we did observe under the microscope some morphological changes in small Ni particles from one temperature to another.
FIG. 7 UNPA sensitivity as a function of sintering temperature for Ni agglomerates of different mobility diameters.
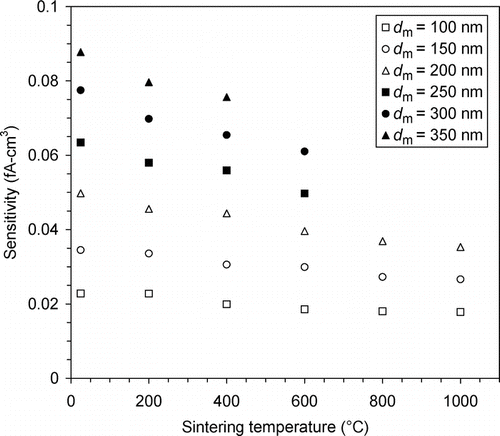
The change in Ni sensitivity between different sintering temperatures is greater for larger mobility sizes. This confirms the experimental findings by Oh et al. (Citation2004) and Shin, Wang et al. (Citation2010) with regard to silver agglomerates. It implies that the structural difference between these agglomerates becomes more pronounced as particles get larger. This point is not very difficult to understand, as a large agglomerate, such as our Ni agglomerates with d m = 300 and 350 nm, has a great number of primary particles and a much larger geometric extension than a sphere of the same mobility size, which allow charge to be more widely distributed within the particle. In contrast, for a small agglomerate, e.g., Ni agglomerates with d m = 100 nm, its capability of distributing and holding more charge than a sphere is limited.
It is interesting to relate the UNPA sensitivity measurement with other metrics of agglomerate morphology, such as the fractal dimension. We obtained the average value of 2D fractal dimension for polydisperse Ni particles sampled on TEM grids at selected sintering temperatures. A customized macro (FracLac, version 2.5, A. Karperien) for the ImageJ software was used, which determines the fractal dimension by a nested-square method similar to that in the study of Forrest and Witten (Citation1979). We ran the analysis first on test images and obtained 2D fractal dimensions of 1.0 and 2.0 for a line and a plane, respectively. The fractal dimension of any real particle image would lie between these values. The fractal dimensions of Ni agglomerates are summarized in for sintering temperatures at which TEM images are available. It can be seen that for each mobility diameter, the UNPA sensitivity diminishes with while the fractal dimension increases with rising temperature, both are indications of particle compaction during sintering. These two quantities might be implicitly related via the charging dynamics of the fractal agglomerates. Furthermore, it is tempting to build on the TEM images and UNPA sensitivity to reveal the differences in the sintering process for gold, silver, and nickel nanoparticle agglomerates. Unfortunately, with the limited TEM images and sensitivity data for silver and gold in the present work, we can only state that silver agglomerates reach complete coalescence (spherical particles and no further change in sensitivity) at the lowest temperature among all three, followed by gold and nickel. This finding corresponds to the order of their melting points, which implies that material bulk properties play a significant role in nanoscale thermodynamic processes.
TABLE 3 UNPA sensitivity of Ni agglomerates with selected mobility diameters as functions of sintering temperature and 2D fractal dimension
In sum, from our experience, the UNPA sensitivity is a sensitive measure for the morphological change in agglomerates. It can provide useful insight into subtle changes (e.g., between and i) that are not always detectable by TEM analysis due to primary particle overlapping and, sometimes, low image resolution. On top of these, UNPA delivers measurement in situ and in real time.
Instrumental Evaluation of Primary Particle Size
Before one can use UNPA to evaluate the primary particle size, the coefficients in Equation (5), c 1, h, c 2, and k, need to be determined through calibration. The UNPA calibration in the present work was carried out using loose silver agglomerates generated by a tube furnace (d p = 19.5 nm by TEM), and fully sintered silver spheres. Power fitting to the sensitivity data of spheres and loose agglomerates according to EquationEquations (2) and Equation(5), respectively, gives c 1 = 6.02 × 10−5, h = 1.216, c 2 = 0.595, and k = 0.736.
It is worthwhile to investigate how these coefficients would be affected if different particles were used in the calibration. Shin et al. (Citation2009) reported a small effect of material dielectric constant on unipolar charging of spherical nanoparticles. We also found in the present and other UNPA works that the sensitivity of spheres is relatively independent of material type. In addition, the calibration coefficients of a given UNPA should be, in principle, not affected by the primary particle size of calibrating agglomerates, because the exponent of the power fitting is mainly affected by the slope of the sensitivity data set and d p is included in the prefactor to account for differences in sensitivity magnitude. However, in practice, slightly different coefficient values are obtained for different calibrating particles (in terms of material type, generation method, or primary particle size). In the routine UNPA work in our laboratory, the calibration coefficients are determined with certain reference particles (e.g., loose silver agglomerates and sintered silver spheres by furnace method) and taken to be applicable to other particles too. It was found that this treatment does not significantly affect the calculated primary particle size.
Once the calibration coefficients are known, to evaluate the primary particle size, we can rewrite EquationEquation (5) as:
A linear fitting between S
1/(h−2k)
agg and can give d
p as the slope. The primary particle sizes for Au, Ag, and Ni agglomerates are calculated in this manner using the sensitivity data in and compared in with the values measured by TEM. Reasonable agreement is found between the two groups, with a discrepancy of about 1 standard deviation, except that for Ni agglomerates, the difference is 2.5 standard deviations. The larger difference for Ni is believed to be partly due to TEM sizing error. Because of the relatively large transmitivity of Ni to electron beam, Ni agglomerates have low-contrast TEM images in which primary particles are hard to distinguish. As a consequence, much fewer Ni primary particles could be picked and sized than for Au and Ag (), bringing a greater uncertainty to the sizing result.
TABLE 4 Comparison of primary particle sizes measured by UNPA with those by TEM
It might have been noted that the primary sizes calculated by UNPA seem to be systematically smaller than the TEM-measured values. The reason could lie in the calibration of the current UNPA unit, the non-ideal effects (e.g., less necking found in spark-generated agglomerates than in furnace-generated calibration agglomerates), or the theory. Further investigation is needed to address this concern. Its influence in the evaluation of agglomerate mass concentration will be discussed later.
Nevertheless, UNPA gave a correct estimation of the order of the primary particle sizes. It was found in that the loose agglomerate (at RT) with a smaller primary particle size has higher sensitivity for a given mobility size. Mathematically, this can be explained by the fact that the exponent h – 2k in EquationEquation (5) has a negative value in the present study (–0.256), as well as in the study of Wang et al. (Citation2010) and the unpublished UNPA work of the authors. The physical interpretation is that for a given mobility diameter, smaller primary particle size corresponds to larger capacitance and specific surface area that can accommodate more electrical charge.
Real-Time Assessment of Agglomerate Mass Concentration
After the primary particle size is determined, the mobility size distribution of loose agglomerates measured in the scanning mode of UNPA can be corrected for the actual number, surface area, and volume distributions. The correction is based on the approach introduced by Lall and Friedlander (Citation2006). It is worth noting that a few years ago, TSI introduced into the SMPS software the same Lall–Friedlander calculations of agglomerate surface area and volume distributions as described here. Here, we refer to the SMPS scan as one without such correction, i.e. particles are assumed to be spheres. For a given number of particles detected at the exit of the DMA (assuming all singly charged), the original number concentrations of spheres (n sph) and loose agglomerates (n agg) are related by:
FIG. 8 Comparison of SMPS scans based on sphere assumption with size distributions corrected for loose silver agglomerates at room temperature: (a) number, (b) surface area, and (c) volume.
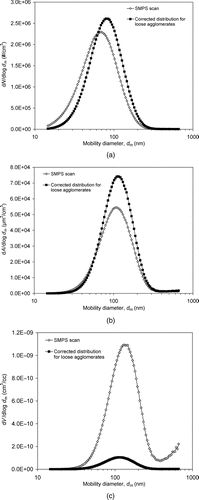
FIG. 9 Comparison between silver agglomerate mass concentrations obtained by different methods. Filter sampling: 2.77 ± 0.07 ng/cm3; UNPA 1: 2.96 ± 0.02 ng/cm3 (d p = 11.8 nm by TEM); UNPA 2: 2.50 ± 0.02 ng/cm3 (d p = 8.4 nm by sensitivity data fitting); Effective density: 1: 3.56 ± 0.03 ng/cm3 (correlation 1), 2: 4.24 ± 0.03 ng/cm3 (correlation 2); SMPS (based on sphere assumption): 25.74 ± 0.35 ng/cm3.
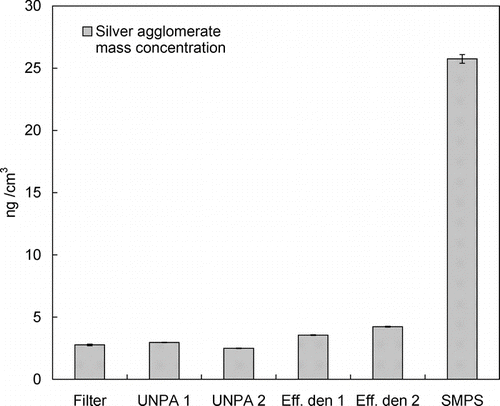
As an example, we compare in the size distributions thus corrected to the standard SMPS scan for spark-generated silver agglomerates at RT. The primary particle size d p of 11.8 nm was used in the calculations. It is seen that the discrepancy in the number size distributions shown in is relatively small. This discrepancy is solely due to the different singly charged fractions of spheres and loose agglomerates. In this case, the graph implies that η sph < η agg for d m < ∼60 nm and the opposite for d m > ∼60 nm. In contrast, the differences in the surface area and volume distributions shown in and mainly result from the different ways of calculating surface area and volume for a sphere and a loose agglomerate. For spheres, instead of EquationEquations (8) and Equation(9), surface area and volume distributions are calculated by A sph=n sph(πd 2 m) and V sph=n sph(πd 3 m/6), respectively. Note that the discrepancy in the surface area distributions is not as large as one would expect intuitively. This is because the comparison is based on mobility diameter, so the larger specific surface area of an agglomerate is somehow cancelled by its smaller mass than that of a sphere with the same mobility diameter. A larger difference in surface area distribution than that in would result from a comparison based on volume-equivalent diameter. Finally, the biggest difference is noticed in between the two volume distributions. The peak differential volume concentration in the corrected distribution is estimated to be 11 times lower than that in the raw scan, assuming particles as spherical.
Real-time measurement of agglomerate mass concentration is of great interest in the present study and in practice. With the knowledge of particle volume distribution, the total mass concentration of loose agglomerates can be obtained by multiplying the integral of the volume distribution with material density (density of silver = 10.5 g/cm3). Besides the SMPS and the UNPA methods, the mass concentration of spark-generated silver agglomerates at RT was evaluated using the effective density, which is defined as:
To provide direct verification of the foregoing online mass measurements, filter collection was performed for silver agglomerates at RT. The aerosol mass concentration measured by three 1-h samples, each sampling under a flow rate of 1.5 lpm, is 2.77 ± 0.07 ng/cm3. The mass concentrations measured by all the preceding methods are compared in . In the figure, the labels “UNPA 1” and “UNPA2” denote that the correction is based on primary particle sizes by TEM analysis and sensitivity data fitting, respectively (); “Eff. den 1” and “Eff. den 2” suggest the use of the correlations 1 and 2 of the effective density mentioned above; and “SMPS” denotes result based on the assumption of spherical particles. Firstly, good agreement is found between the UNPA results and the filter collection result. It is also found that the d p used in UNPA 2 being 29% smaller than the value used in UNPA 1 causes a decrease in the calculated mass concentration by 15%. The smaller decrease in calculated mass concentration is partly due to an increase in N according to EquationEquation (1) and a shift in the number distribution (η agg varies with d p) as a result of reduced d p. Secondly, the two effective density results are slightly larger than yet still in reasonable agreement with the filter collection result. Improved agreement might be achieved if effective density data were available for the silver agglomerates in the current study. Finally, the calculation of mass concentration based on sphere assumption is 9.3 times higher than the gravimetric measurement, which corroborates the result in and the findings by Lall et al. (Citation2006) and Wang et al. (Citation2010). To conclude, a complementary comparison is made in for the mass distributions of loose silver agglomerates obtained by UNPA and the effective density. Despite the difference in magnitude, it can be seen that the peak sizes in these mass distributions are in good agreement.
CONCLUSIONS
The UNPA was used to measure metal nanoparticle agglomerates generated by spark discharge. Gold, silver, and nickel agglomerates consisting of relatively uniform primary particles were generated by this system. A deeper look into the sintering of spark-generated metallic agglomerates was taken with the aid of UNPA. The agglomerate structural change during sintering proves to be well characterized by the sensitivity measurement, along with TEM and mobility analyses. Besides, the primary particle sizes calculated by fitting the sensitivity data of agglomerates at RT are in reasonable agreement with the TEM sizing results. The seemingly systematic error between the two approaches requires further investigation but does not affect the agglomerate mass concentration calculated by UNPA as much. Finally, the mass concentration of loose silver agglomerates calculated by UNPA shows good agreement with the filter sampling result, followed by the prediction from the effective density. It suggests that UNPA can be a good alternative to the gravimetric method for measuring the mass concentration of loose agglomerates. Our results also indicate that the misinterpretation of the SMPS data for agglomerate mass can be avoided through a careful analysis of the data using the Lall and Friedlander model or the effective density, if the latter is available. In summary, it is known from this study that UNPA can give relatively good estimation of the primary particle size and mass concentration for loose agglomerates satisfying the assumptions in Lall–Friedlander theory (Citation2006). However, to what extent the technique can be applied to non-ideal agglomerates or aggregates remains an open question right now.
Acknowledgments
This work was supported by the NSF (National Science Foundation) grant (award ID: 1056479) on “Real Time Measurement of Agglomerated or Aggregated Airborne Nanoparticles Released From a Manufacturing Process and Their Transport Characteristics.” The authors thank Professor Günter Oberdörster at University of Rochester, New York, NY, for lending the spark generator.
REFERENCES
- Brown , R. C. and Hemingway , M. A. 1995 . Electric Charge Distribution and Capacitance of Agglomerates of Spherical Particles: Theory and Experimental Simulation . J. Aerosol Sci. , 26 ( 8 ) : 1197 – 1206 .
- Chan , P. and Dahneke , B. 1981 . Free-Molecule Drag on Straight Chains of Uniform Spheres . J. Appl. Phys. , 52 ( 5 ) : 3106 – 3110 .
- Chang , J. S. 1981 . Theory of Diffusion Charging of Arbitrarily Shaped Conductive Aerosol Particles by Unipolar Ions . J. Aerosol Sci. , 12 : 19 – 26 .
- Chen , Y. , Shah , N. , Braun , A. , Huggins , F. E. and Huffman , G. P. 2005 . Electron Microscopy Investigation of Carbonaceous Particulate Matter Generated by Combustion of Fossil Fuels . Energy Fuels , 19 : 1644 – 1651 .
- Crump , J. G. , Flagan , R. C. and Seinfeld , J. H. 1983 . Particle Wall Loss Rates in Vessels . Aerosol Sci. Technol. , 2 : 303 – 309 .
- Crump , J. G. and Seinfeld , J. H. 1981 . Turbulent Deposition and Gravitational Sedimentation of an Aerosol in a Vessel of Arbitrary Shape . J. Aerosol Sci. , 12 : 405 – 415 .
- Fissan , H. , Neumann , S. , Trampe , A. , Pui , D. Y. H. and Shin , W. G. 2007 . Rationale and Principle of an Instrument Measuring Lung Deposited Nanoparticle Surface Area . J. Nanoparticle Res. , 9 : 53 – 59 .
- Forrest , S. R. and Witten , T. A. 1979 . Long-Range Correlations in Smoke-Particle Aggregates . J. Phys. A: Math. Gen. , 12 ( 5 ) : L109 – L117 .
- Fuchs , N. A. 1963 . On the Stationary Charge Distribution on Aerosol Particles in a Bipolar Ionic Atmosphere . Geofis. Pura Appl. , 56 : 185 – 193 .
- Gormley , P. G. and Kennedy , M. 1949 . Diffusion from a Stream Flowing through a Cylindrical Tube . Proc. Roy. Irish Acad. , 52 : 163 – 169 .
- Gwaze , P. , Schmid , O. , Annegarn , H. J. , Andreae , M. O. , Huth , J. and Helas , G . 2006 . Comparison of Three Methods of Fractal Analysis Applied to Soot Aggregates from Wood Combustion . J. Aerosol Sci. , 37 : 820 – 838 .
- Helsper , C. , Molter , W. , Loffler , F. , Wadenpohl , C. , Kaufmann , S. and Wenninger , G. 1993 . Investigations of a New Aerosol Generator for the Production of Carbon Aggregate Particles . Atmos. Environ. , 27A ( 8 ) : 1271 – 1275 .
- Jenkins , N. T. , Pierce , W. M. G. and Eagar , T. W. 2005 . Particle Size Distribution of Gas Metal and Flux Cored Arc Welding Fumes . Welding J. , 84 : 156S – 163S .
- Kelly , W. P. and McMurry , P. H. 1992 . Measurement of Particle Density by Inertial Classification of Differential Mobility Analyzer-Generated Monodisperse Aerosols . Aerosol Sci. Technol. , 17 : 199 – 212 .
- Kim , S. C. , Wang , J. , Emery , M. S. , Shin , W. G. , Mulholland , G. W. and Pui , D. Y. H. 2009 . Structural Property Effect of Nanoparticle Agglomerates on Particle Penetration through Fibrous Filter . Aerosol Sci. Technol. , 43 : 344 – 355 .
- Koylu , U. O. , Faeth , G. M. , Farias , T. L. and Carvalho , M. G. 1995 . Fractal and Projected Structure Properties of Soot Aggregates . Combust. Flame , 100 : 621 – 633 .
- Lall , A. A. and Friedlander , S. K. 2006 . On-Line Measurement of Ultrafine Aggregate Surface Area and Volume Distributions by Electrical Mobility Analysis: I. Theoretical Analysis . J. Aerosol Sci. , 37 : 260 – 271 .
- Lall , A. A. , Seipenbusch , M. , Rong , W. and Friedlander , S. K. 2006 . On-Line Measurement of Ultrafine Aggregate Surface Area and Volume Distributions by Electrical Mobility Analysis: II. Comparison of Measurements and Theory . J. Aerosol Sci. , 37 : 272 – 282 .
- Mandelbrot , B. B. 1982 . The Fractal Geometry of Nature , San Francisco : Freemann .
- Maricq , M. M. and Xu , N. 2004 . The Effective Density and Fractal Dimension of Soot Particles from Premixed Flames and Motor Vehicle Exhaust . J. Aerosol Sci. , 35 : 1251 – 1274 .
- Medalia , A. I. and Heckman , F. A. 1969 . Morphology of Aggregates: II. Size and Shape Factors of Carbon Black Aggregates from Electron Microscopy . Carbon , 7 : 567 – 582 .
- Medved , A. , Dorman , F. , Kaufman , S. L. and Poecher , A. 2000 . A New Corona-Based Charger for Aerosol Particles . J. Aerosol Sci. , 31 : S616 – S617 .
- Oh , H. , Park , H. and Kim , S. 2004 . Effects of Particle Shape on the Unipolar Diffusion Charging of Nonspherical Particles . Aerosol Sci. Technol. , 38 : 1045 – 1053 .
- Oh , C. and Sorensen , C. M. 1997 . Light Scattering Study of Fractal Cluster Aggregation Near the Free Molecular Regime . J. Aerosol Sci. , 28 : 937 – 957 .
- Park , K. , Cao , F. , Kittelson , D. B. and McMurry , P. H. 2003 . Relationship between Particle Mass and Mobility for Diesel Exhaust Particles . Environ. Sci. Technol. , 37 : 577 – 583 .
- Park , K. , Kittelson , D. B. and McMurry , P. H. 2004 . Structural Properties of Diesel Exhaust Particles Measured by Transmission Electron Microscopy (TEM): Relationships to Particle Mass and Mobility . Aerosol Sci. Technol. , 38 : 881 – 889 .
- Pratsinis , S. E. 1998 . Flame Aerosol Synthesis of Ceramic Powders . Prog. Energy Combust. Sci. , 24 : 197 – 219 .
- Puri , R. , Richardson , T. F. , Santoro , R. J. and Dobbins , R. A. 1993 . Aerosol Dynamic Processes of Soot Aggregates in a Laminar Ethene Diffusion Flame . Combust. Flame , 92 : 320 – 333 .
- Roth , C. , Ferron , G. A. , Karg , E. , Lentner , B. , Schumann , G. , Takenaka , S. and Heyder , J. 2004 . Generation of Ultrafine Particles by Spark Discharging . Aerosol Sci. Technol. , 38 : 228 – 235 .
- Samson , R. J. , Mulholland , G. W. and Gentry , J. W. 1987 . Structural Analysis of Soot Agglomerates . Langmuir , 3 : 272 – 281 .
- Schmidt-Ott , A. 1988 . New Approaches to In Situ Characterization of Ultrafine Agglomerates . J. Aerosol Sci. , 19 ( 5 ) : 553 – 563 .
- Schwyn , S. , Garwin , E. and Schmidt-Ott , A. 1988 . Aerosol Generation by Spark Discharge . J. Aerosol Sci. , 19 ( 5 ) : 639 – 642 .
- Shin , W. G. , Mulholland , G. W. and Pui , D. Y. H. 2010 . Determination of Volume, Scaling Exponents, and Particle Alignment of Nanoparticle Agglomerates using Tandem Differential Mobility Analyzers . J. Aerosol Sci. , 41 : 665 – 681 .
- Shin , W. G. , Qi , C. , Wang , J. , Fissan , H. and Pui , D. Y. H. 2009 . The Effect of Dielectric Constant of Materials on Unipolar Diffusion Charging of Nanoparticles . J. Aerosol Sci. , 40 : 463 – 468 .
- Shin , W. G. , Wang , J. , Mertler , M. , Sachweh , B. , Fissan , H. and Pui , D. Y. H. 2010 . The Effect of Particle Morphology on Unipolar Diffusion Charging of Nanoparticle Agglomerates in the Transition Regime . J. Aerosol Sci. , 41 : 975 – 986 .
- Stark , W. J. and Pratsinis , S. E. 2002 . Aerosol Flame Reactors for Manufacture of Nanoparticles . Powder Technol. , 126 : 103 – 108 .
- Wang , J. , Shin , W. G. , Mertler , M. , Sachweh , B. , Fissan , H. and Pui , D. Y. H. 2010 . Measurement of nanoparticle agglomerates by combined measurement of electrical mobility and unipolar charging properties . Aerosol Sci. Technol. , 44 : 97 – 108 .
- Watters , R. L. Jr. , DeVoe , J. R. , Shen , F. H. , Small , J. A. and Marinenko , R. B. 1989 . Characteristics of Aerosols Produced by the Spark Discharge . Anal. Chem. , 61 : 1826 – 1833 .
- Weber , A. P. , Baltensperger , U. , Gaggeler , H. W. and Schmidt-Ott , A. 1996 . In Situ Characterization and Structure Modification of Agglomerated Aerosol Particles . J. Aerosol Sci. , 27 : 915 – 929 .
- Weber , A. P. and Friedlander , S. K. 1997 . In Situ Determination of the Activation Energy for Restructuring of Nanometer Aerosol Agglomerates . J. Aerosol Sci. , 28 ( 2 ) : 179 – 192 .
- Wen , H. Y. , Reischl , G. P. and Kasper , G. 1984 . Bipolar Diffusion Charging of Fibrous Aerosol Particles: I. Charging Theory . J. Aerosol Sci. , 15 ( 2 ) : 89 – 101 .
- Wiedensohler , A . 1988 . An Approximation of the Bipolar Charge Distribution for Particles in the Submicron Size Range . J. Aerosol Sci. , 19 : 387 – 389 .