Abstract
Agglomeration is encountered in many natural or industrial processes, like growth of aerosol particles in the atmosphere and during material synthesis or even flocculation of suspensions, granulation, crystallization, and with colloidal particle processing. These particles collide by different mechanisms and stick together forming irregular or fractal-like agglomerates. Typically, the structure of these agglomerates is characterized with the fractal dimension, Df , and pre-exponential factor, kn , of simulated agglomerates of monodisperse primary particles (PP) for ballistic or diffusion-limited particle–cluster and cluster–cluster collision mechanisms. Here, the effect of PP polydispersity on Df and kn is investigated with agglomerates consisting of 16-1024 PP with closely controlled size distribution (geometric standard deviation, σg = 1–3). These simulations are in excellent agreement with the classic structure (Df and kn ) of agglomerates consisting of monodisperse PPs made by four different collision mechanisms as well as with agglomerates of bi-, tridisperse, and normally distributed PPs. Broadening the PP size distribution of agglomerates decreases monotonically their Df , and for sufficiently broad PP distributions (σg > 2.5) the Df reaches about 1.5 and kn about 1 regardless of the collision mechanism. Furthermore, with increasing PP polydispersity, the corresponding projected area exponent, Dα , and pre-exponential factor, ka , decrease monotonically from their standard values for agglomerates with monodisperse PPs. So Df as well as Dα and ka can be an indication for PP polydispersity in mass mobility and light scattering measurements if the dominant agglomeration mechanism is known, like diffusion-limited and/or ballistic cluster–cluster coagulation in aerosols.
Copyright 2012 American Association for Aerosol Research
INTRODUCTION
Significant advances have been made in agglomerate characterization by employing the so-called fractal theory and relating agglomerate structure to its generation pattern through the fractal dimension, Df . For example, agglomerates made by diffusion-limited cluster–cluster agglomeration (DLCA) have Df = 1.78 (Jullien et al. Citation1984), or by diffusion-limited particle–cluster agglomeration (DLA) have Df = 2.5 (Tolman and Meakin Citation1989), ballistic cluster–cluster agglomeration (BCCA) have Df = 1.90 (Meakin and Jullien Citation1988), and ballistic particle–cluster agglomeration (BPCA) have Df = 3.0 (Ball and Witten Citation1984) as were summarized for aerosols by Schaefer and Hurd (Citation1990). These Df values have become the standard in agglomerate (physically bound particles) and even aggregate (chemically- or sinter-bound particles) characterization despite the fact that such particles may not fully obey fractal theory but are sufficiently close to it to be called fractal-like.
This concept has served well the process design of a wide spectrum of filamentary particles (carbon black, nickel, and fumed silica), made up mostly by aerosol coagulation. In fact, a number of characterization techniques and process design concepts have been developed capitalizing on these Df values to extract other particle properties (e.g., collision diameter, primary particle size) and design reactors for manufacturing such particles. They are characterized by a power law relating the number of primary particles in an agglomerate to primary particle radius and radius of gyration or collision radius. For example, in aerosol reactor design, the coagulation rate of spherical particles is corrected to that of fractal-like ones in the free molecular (Matsoukas and Friedlander Citation1991) and continuum (Oh and Sorensen Citation1997) regimes by introducing the radius of gyration and Df in their collision area term (Mountain et al. Citation1986).
What might have been overlooked in characterization and simulations of fractal-like particles is that the above Df values have been developed for agglomerates of monodisperse primary particles. Notable exceptions come from Tence et al. (Citation1986) and Bushell and Amal (Citation1998) who had examined the effect of primary particle polydispersity on agglomerate structure and scattering behavior. They numerically generated BCCA and DLCA agglomerates having Gaussian-distributed (Tence et al. Citation1986) and mono-, bi-, and tridisperse (Bushell and Amal Citation1998) primary particles and found no effect on Df for their employed polydispersities.
For coagulating aerosols, however, this needs to be carefully examined, as Brownian coagulation-driven particle formation leads to polydisperse particles. Their distribution, even at a single streamline is, at best, as narrow as that given by the self-preserving theory, e.g., having geometric standard deviation, σg , of about 1.45 (Landgrebe and Pratsinis Citation1989). When agglomerates are made in aerosol reactors at different (e.g., radial) residence time distributions and collected, typically, by filtration, much broader size distributions can be obtained. Agglomerates with quite polydisperse primary particle size distribution are obtained during gas-to-particle conversion or when primary particles with different residence time histories are mixed. So, the common extraction of Df by microscopic counting of such agglomerates and primary particles may not necessarily lead to an accurate assessment of their formation pathway as has been proposed by Schaefer and Hurd (Citation1990). Similarly, the use of Df in designing aerosol synthesis of such particles needs to account for agglomerates of polydisperse primary particles.
Here, fractal-like agglomerates consisting of 16, 64, 256, 512, and 1024 primary particles having closely controlled size distributions are generated by DLA, DLCA, BCCA, and BPCA. So, the employed primary particle radii are lognormally distributed with geometric standard deviation σg ranging from 1 (monodisperse) to 3 and the structure characteristics (Df and pre-exponential factor kn ) of such agglomerates are calculated by fractal theory for these collision mechanisms and compared to the literature at limiting cases. Furthermore, the projected area exponent and pre-exponential factor that are used in aerosol characterization by mass-mobility measurements are obtained as a function of primary particle polydispersity.
Theory
Agglomerates grown by particle collisions exhibit a power law scaling between number of primary particles, np , and radius of gyration, rg (Mandelbrot Citation1982):
This corresponds to the average primary particle radius determined experimentally by nitrogen adsorption, r BET, and small-angle X-ray scattering (SAXS), rVS (Hyeon-Lee et al. Citation1998). The rva is equal to rp for agglomerates of monodisperse spherical primary particles.
The projected agglomerate area aa onto a plane is also related to np by a power law (Medalia Citation1967):
This holds well even in the transition regime up to Knudsen number Kn = 0.28 (Rogak et al. Citation1993). Equations (Equation5) and (Equation6) are used to characterize agglomerates by mass-mobility measurements (Park et al. Citation2003).
FIG. 1 Agglomerates consisting of 1024 monodisperse primary particles made by (a) diffusion-limited (DLCA) and (b) ballistic cluster–cluster (BCCA) agglomeration as well as by (c) diffusion-limited (DLA) and (d) ballistic particle–cluster (BPCA) agglomeration. These agglomerates have Df = 1.79, 1.89, 2.25, and 2.81 identical to those calculated by Botet et al. (1982), Tence et al. (Citation1986), Witten and Sander (Citation1981), and Sutherland (Citation1966), respectively.
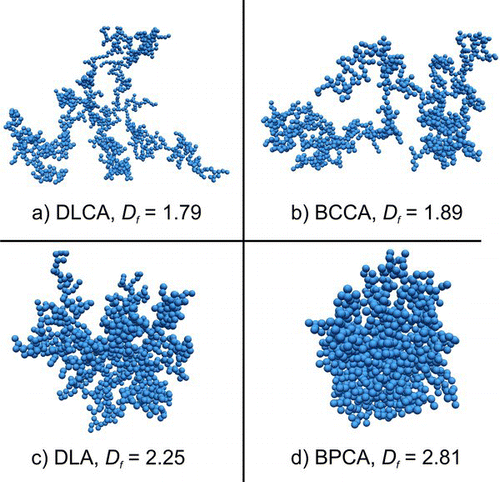
The aa is calculated by Monte Carlo integration (Meakin Citation1988) and averaged over 50 homogeneously distributed random angles for each agglomerate. The Df and kn as well as Dα and ka are obtained by ensemble averaging over agglomerates of different np (Eggersdorfer et al. Citation2010) and similarly to the evaluation of experiments (Sorensen et al. Citation1992). The Df or Dα is determined from the slope of rg or aa versus the np or nva in a double logarithmic plot, while the intersection with the y-axis is the logarithm of the prefactor kn or ka , respectively (Medalia Citation1967; Forrest and Witten Citation1979).
RESULTS AND DISCUSSION
Validation
One hundred agglomerates of each number (np = 16-1024) and polydispersity (σg = 1–3) of primary particles (PP) are generated by DLA (Witten and Sander Citation1981), DLCA (Botet et al. Citation1984), BCCA (Tence et al. Citation1986), and BPCA (Sutherland Citation1966) to determine Df and kn by Equation (Equation1) and Dα and ka by Equation (Equation5). shows typical images of agglomerates made with monodisperse primary particles (σg = 1) for DLA, DLCA, BCCA, and BPCA similar to Schaefer and Hurd (Citation1990). These agglomerates have Df = 1.79 ± 0.03 (DLCA), 1.89 ± 0.03 (BCCA), 2.25 ± 0.02 (DLA), and 2.81 ± 0.03 (BPCA). The DLCA and BCCA values are in excellent agreement with Jullien et al. (Citation1984) and Tence et al. (Citation1986). The Df of DLA and BPCA are slightly smaller than their asymptotic limits of 2.5 and 3 by Tolman and Meakin (Citation1989) and Ball and Witten (Citation1984), respectively. This is a finite size effect, as the Df of small agglomerates is always lower than their asymptotic Df (Meakin Citation1999). kn = 1.40 ± 0.12 for monodisperse DLCA agglomerates () is consistent with Sorensen and Roberts (Citation1997). The BCCA, DLA, and BPCA agglomerates of monodisperse primary particles have kn = 1.36 ± 0.1, 0.86 ± 0.04, and 0.46 ± 0.03 (, actual np and rp ).
TABLE 1 The prefactor kn and fractal dimension Df of agglomerates made by DLCA, BCCA, DLA, and BPCA as obtained using the actual PP number np and radius rp are compared to kn and Df obtained assuming monodisperse PPs of equivalent average number nva and radius rva (Equations (3) and (4))
Agglomerates with bi- and tridisperse primary particles were made also by DLCA to compare to Bushell and Amal (Citation1998). These agglomerates consist of primary particles of two and three distinct diameters, respectively. Their bidisperse agglomerates with a PP radius ratio of 3:1, and about 4% of the large particles were calculated here to have Df = 1.79 ± 0.03. Similarly their tridisperse PP radius ratio of 5:3:1 with distributions of 1:18:81% and PP radius ratio of 5:2:1 with distributions of 10:60:30% were calculated here to have Df = 1.77 ± 0.03 and 1.79 ± 0.03, respectively. All were in excellent agreement with Bushell and Amal (Citation1998) who had reported Df = 1.78 ± 0.03. Tence et al. (Citation1986) obtained by BCCA agglomerates of primary particles having a Gaussian-like distribution (n = 2 and β = 5) that corresponds to a σ g = 1.35. The present simulations with primary particles having this σ g resulted in agglomerates that had a Df = 1.91 ± 0.05, which is quite close to that of 1.89 ± 0.03 (Tence et al. Citation1986). These results validate the employed algorithms to address the structure of agglomerates having arbitrarily broad distributions of primary particles.
Effect of Primary Particle Polydispersity on Fractal Dimension and Prefactor
The primary particles are lognormally distributed with a geometric standard deviation σg = 1–3. shows a TEM-like image of 100 DLCA agglomerates consisting of 512 PPs with σg = 2.
shows close-up snapshots of randomly chosen agglomerates consisting of 1024 PPs of σg = 2 and 3 made by DLCA, BCCA, DLA, and BPCA along with the corresponding Df . The broader the PP size distribution and thus the larger the difference between PPs, the stringier and more open are the agglomerates having a lower Df than the benchmark agglomerates of monodisperse PPs (). At σg = 3, all agglomerates look similar somehow, regardless of the collision mechanism.
quantifies these pictures by showing the Df of agglomerates as a function of their constituent PP size distribution from σg = 1 (monodisperse) to 3 for DLCA (circles), BCCA (squares), DLA (triangles), and BPCA (diamonds). Df decreases with increasing σg and for σg = 3 the different collision mechanisms converge to nearly the same Df ≈ 1.5. Clearly, the presence of various primary particle sizes results in more open agglomerate structures. The more polydisperse are the PPs, the more open space they leave between them within an agglomerate.
FIG. 3 Agglomerates of polydisperse primary particles having geometric standard deviation σg = 2.0 (left column) and 3.0 (right column) made by diffusion-limited (DLCA) and ballistic cluster–cluster agglomeration (BCCA) as well as by diffusion-limited (DLA) and ballistic particle–cluster agglomeration (BPCA). The structure of agglomerates consisting of very polydisperse (σg = 3) particles is quite similar (Df = 1.48 – 1.57) indicating that their polydispersity essentially “washes out” differences in agglomeration mechanisms.
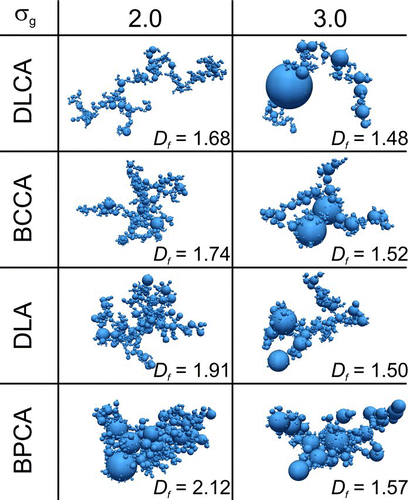
Polydispersity adds another element of disorder when uniform primary particles are replaced with nonuniform ones. As the motion of particles or clusters is independent from each other, their arrival or sticking point upon collision is fixed. Take, for example, particle–cluster agglomeration. The trajectory of the particle till its collision with a cluster is determined by its mobility diameter. As individual polydisperse PPs arrive at the cluster, more irregular and open structures are developed than with uniform PPs. As a result, the compact agglomerate structures made by DLA and BPCA of monodisperse PPs with Df of 2.25 and 2.81, respectively, are affected most dramatically by the PP polydispersity (). Similar concepts apply to cluster–cluster agglomeration. The larger particles contribute much more to rg than the smaller ones since rg is calculated with the mass-weighted mean square displacement (Equation (2)). So Df is very sensitive to PP polydispersity. The differences between particle masses increase for increasing σg so that at high polydispersities fewer particles effectively contribute to Df . This results in Df = 1 in the limit of two effective particles as rg represents the high-order moments of the distribution (square root of the ratio between 2nd and 0th moment of mass-based distribution).
FIG. 4 The asymptotic (a) fractal dimension Df and (b) prefactor kn of 500 agglomerates as a function of the geometric standard deviation, σg , of their primary particles (PP) for different collision or agglomeration mechanisms. At σg = 1 (monodisperse PP), the classic Df of the corresponding structures is obtained. With increasing σg , the Df decreases gradually reaching asymptotically ≈ 1.5 (a) and kn ≈ 1 (b) at σg = 3, nearly independent of the agglomeration mechanism.
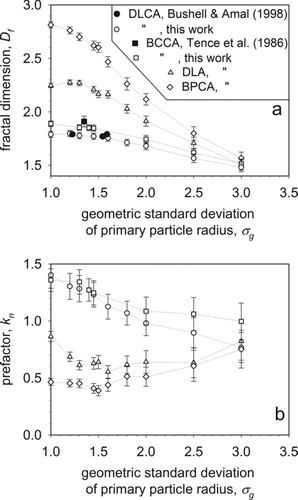
shows also the DLCA simulations (filled circles) of bi- and tridisperse PPs by Bushell and Amal (Citation1998) as well as the BCCA ones (filled square) of Tence et al. (Citation1986). Clearly, both studies had been carried out with relatively narrow PP-size distributions to observe any reduction of Df by the polydispersity of primary particles seen here. This monotonic reduction of Df with increasing σg can be used to determine the effect of PP polydispersity on the structure of agglomerates by all four collision mechanisms.
shows the prefactor kn for the agglomerates made here as a function of PP σg also. While Df decreases monotonically for increasing σg , regardless of collision mechanism, not all kn exhibit such a monotonic behavior. Nevertheless, all reach a kn ≈ 1 for very broad PP polydispersities (σg > 2.5). For DLCA and BCCA particles, kn decreases continuously with increasing PP polydispersity, while for both DLA and BPCA kn reaches a minimum here at σg = 1.6 and 1.5, respectively.
FIG. 5 The projected area exponent Dα (a) and prefactor ka (b) of DLCA (circles), BCCA (squares), DLA (triangles), and BPCA (diamonds) agglomerates as a function of the geometric standard deviation σg of their constituent primary particles. Dα and ka decrease monotonically for increasing σg regardless of the agglomerate generation mechanism.
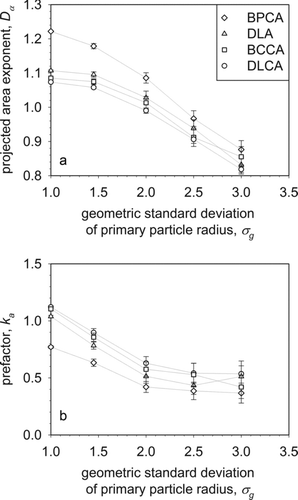
For σg = 1.45, the standard deviation of the self-preserving size distribution (SPSD) obtained by Brownian coagulation that corresponds best to DLCA, both Df and kn are within the error bars of agglomerates with monodisperse PPs and thus indistinguishable from each other. This indicates that PP polydispersity may not affect the structure of DLCA agglomerates made by aerosol coagulation on a single process streamline or residence time history. When, however, such particles are collected from a filter where particles of various residence time (and even temperature) histories are arriving, there would be a significant effect depending on their actual polydispersity. In practical systems, the primary particle size distribution is often unknown and for simplicity assumed to be monodisperse.
compares the Df and kn determined with the actual rp and np to that determined by the assumption of an average PP size of polydisperse (σg = 1–3) PPs using rva and nva as might be determined by microscopic counting, nitrogen adsorption, or SAXS. For all agglomeration mechanisms, the Df is not affected by more than 10%. In contrast, the kn is overestimated significantly when assuming all primaries to have the same size for all collision mechanisms (, last column).
FIG. 6 The fractal dimension Df versus projected area exponent 2Dα for DLCA (circles), BCCA (squares), DLA (triangles), and BPCA (diamonds) agglomerates and σg = 1–3. The broken line corresponds to Df = 2Dα . For cluster–cluster agglomerates, 2Dα is always larger than Df , while particle–cluster agglomerates have a transition at Df ≈ 2.1 between σg = 1.5 and 2.
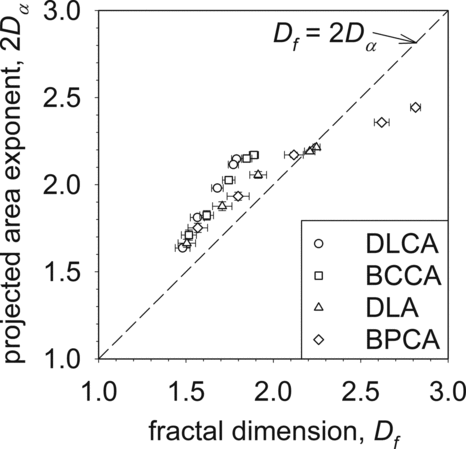
Effect of Primary Particle Polydispersity on Projected Surface Area and Mass-Mobility Characterization
The projected surface area aa of agglomerates controls the mass and heat transfer, e.g., the collision frequency with particles much smaller than the gas mean free path and thus the mobility of agglomerates in the free molecular and transition regime (Meakin Citation1988; Rogak et al. Citation1993). For DLCA agglomerates of monodisperse PPs, Dα = 1.08 ± 0.002 in Equation (Equation5), which is consistent with Pierce et al. (Citation2006). Similar to Df , the Dα decreases with increasing σg (). The aa depends on the square of PP radius. So, the largest PPs dominate while the smallest PPs hardly contribute to aa . Agglomerates of the same mean PP radius but broader σg also have a larger aa and, in turn, a reduced Dα regardless of the collision mechanism.
shows the prefactor ka of Equation (Equation5) as a function of σg . The ka decreases until σg = 2.0 and levels off for higher polydispersities. Though even for σg = 3.0, where Df and kn are nearly independent of the collision mechanism indicating a similar mass distribution of the agglomerates, Dα and ka differ for the four collision types. The DLCA particles have always the largest ka and smallest Dα , since their most open structure results in primary particles that are less shielded by neighbors compared to BCCA, DLA, and BPCA agglomerates ( and ). For all collision mechanisms, the monotonic dependence of Dα and ka on σg can be used to extract the effect of PP polydispersity of agglomerates by mass-mobility measurements as with Df ().
As two measures of fractal dimension are available for these agglomerates of various PP polydispersities and collision mechanisms, it is worth comparing them. So, shows the Df
versus 2Dα
by combining and . For both diffusion-limited and ballistic cluster–cluster agglomeration, the rg
-based Df
is smaller than the rm
-based 2Dα
regardless of PP polydispersity, with rm
= dm
/2. This is not surprising as DLCA and BPCA agglomerates have a Df
< 2 and very open chain-like structures even for a monodisperse PP-size distribution, so always rm
< rg
(Sorensen Citation2011) and Df
< 2Da
. Particle–cluster agglomerates (triangles & diamonds), however, are rather compact at σg
< 2 with Df
> 2 (), so Df
> 2Dα
. Both BPCA and DLA agglomerates of monodisperse PPs have rm
> rg
similar to that of a sphere with (Hiemenz Citation1986). With increasing PP polydispersity, however, the particle–cluster agglomerates become more open so
and exhibit a transition at Df
≈ 2.1 from Df
> 2Dα
to Df
< 2Dα
(between σg
= 1.5 and 2 in and ) as their rm
< rg
. For Df
> 2, the projected areas are compact objects with screening of individual PP while for Df
< 2 almost all PPs are accessible (Mulholland et al. Citation1988). So the polydispersity of PPs affects most dramatically the structure of particle–cluster agglomeration made by both diffusion-limited and ballistic collisions.
CONCLUSIONS
Agglomerates consisting of polydisperse primary particles were generated by DLCA, BCCA, DLA, and BPCA. The effect of primary particle size distribution on resulting agglomerate fractal dimension Df and prefactor kn , projected area exponent Dα , and prefactor ka was investigated. Asymptotic Df and kn values were obtained for agglomerates of primary particles having geometric standard deviation σg ranging from 1.0 to 3.0. These simulations were in agreement with the literature for fractal agglomerates of monodisperse primary particles and even with limited studies of fractal-like particles containing Gaussian-like, bi- and tridisperse primary particles.
It was discovered that polydisperse primary particles result in more open agglomerate structures with lower Df , Dα, and ka than the classic with monodisperse PPs. This is most notable for particle–cluster (up to 50% reduction) and to a lesser extent for cluster–cluster (up to 20% reduction) ballistic and diffusion-limited collision-generated agglomerates. Furthermore, it was shown that the assumption of an average primary particle size, which is commonly applied in particle characterization, hardly affects Df but significantly overestimates kn for polydisperse PPs for all collision mechanisms. Most remarkably, for processes generating agglomerates by a known collision mechanism, a Df , Dα , or ka smaller than the benchmark values of agglomerates consisting of monodisperse PPs might be an indication for agglomerates of polydisperse primary particles.
Acknowledgments
This research was funded by ETH Research Grant (ETHIIRA) ETH-11 09-1 and the European Research Council.
REFERENCES
- Ball , R. C. and Witten , T. A. 1984 . Causality Bound on the Density of Aggregates . Phys. Rev. A , 29 : 2966 – 2967 .
- Botet , R. , Jullien , R. and Kolb , M. 1984 . Hierarchical Model for Irreversible Kinetic Cluster Formation . J. Phys. A: Math. Gen. , 17 : L75 – L79 .
- Bushell , G. and Amal , R. 1998 . Fractal Aggregates of Polydisperse Particles . J. Colloid Interface Sci. , 205 : 459 – 469 .
- Eggersdorfer , M. L. , Kadau , D. , Herrmann , H. J. and Pratsinis , S. E. 2010 . Fragmentation and Restructuring of Soft-Agglomerates under Shear . J. Colloid Interface Sci. , 342 : 261 – 268 .
- Forrest , S. R., and Witten , T. A. 1979 . Long-Range Correlations in Smoke–Particle Aggregates . J. Phys. A: Math. Gen. , 12 : L109 – L117 .
- Hiemenz , P. C. 1986 . Principles of Colloid and Surface Chemistry , New York : Marcel Dekker. Inc .
- Hyeon-Lee , J. , Beaucage , G. , Pratsinis , S. E. and Vemury , S. 1998 . Fractal Analysis of Flame-Synthesized Nanostructured Silica and Titania Powders Using Small-Angle X-Ray Scattering . Langmuir , 14 : 5751 – 5756 .
- Jullien , R. , Kolb , M. and Botet , R. 1984 . Aggregation by Kinetic Clustering of Clusters in Dimensions d Greater than 2 . J. Phys. Lett. , 45 : L211 – L216 .
- Landgrebe , J. D. and Pratsinis , S. E. 1989 . Gas-Phase Manufacture of Particulates—Interplay of Chemical Reaction and Aerosol Coagulation in the Free Molecular Regime . Ind. Eng. Chem. Res. , 28 : 1474 – 1481 .
- Mandelbrot , B. B. 1982 . The Fractal Geometry of Nature , New York : W.H. Freeman .
- Matsoukas , T. and Friedlander , S. K. 1991 . Dynamics of Aerosol Agglomerate Formation . J. Colloid Interface Sci. , 146 : 495 – 506 .
- Meakin , P. 1988 . Fractal Aggregates . Adv. Colloid Interface Sci. , 28 : 249 – 331 .
- Meakin , P. 1999 . A Historical Introduction to Computer Models for Fractal Aggregates . J. Sol-Gel Sci. Technol. , 15 : 97 – 117 .
- Meakin , P. and Jullien , R. 1988 . The Effects of Restructuring on the Geometry of Clusters Formed by Diffusion-Limited, Ballistic, and Reaction-Limited Cluster Cluster Aggregation . J. Chem. Phys. , 89 : 246 – 250 .
- Medalia , A. I. 1967 . Morphology of Aggregates .1. Calculation of Shape and Bulkiness Factors—Application to Computer-Simulated Random Flocs . J. Colloid Interface Sci. , 24 : 393 – 404 .
- Mountain , R. D. , Mulholland , G. W. and Baum , H. 1986 . Simulation of Aerosol Agglomeration in the Free Molecular and Continuum Flow Regimes . J. Colloid Interface Sci. , 114 : 67 – 81 .
- Mulholland , G. W. , Samson , R. J. , Mountain , R. D. and Ernst , M. H. 1988 . Cluster Size Distribution for Free Molecular Agglomeration . Energy Fuels , 2 : 481 – 486 .
- Oh , C. and Sorensen , C. M. 1997 . Light Scattering Study of Fractal Cluster Aggregation Near the Free Molecular Regime . J. Aerosol Sci. , 28 : 937 – 957 .
- Park , K. , Cao , F. , Kittelson , D. B. and McMurry , P. H. 2003 . Relationship between Particle Mass and Mobility for Diesel Exhaust Particles . Environ. Sci. Technol. , 37 : 577 – 583 .
- Pierce , F. , Sorensen , C. M. and Chakrabarti , A. 2006 . Computer Simulation of Diffusion-Limited Cluster–Cluster Aggregation with an Epstein Drag Force . Phys. Rev. E , 74
- Rogak , S. N. , Flagan , R. C. and Nguyen , H. V. 1993 . The Mobility and Structure of Aerosol Agglomerates . Aerosol Sci. Technol. , 18 : 25 – 47 .
- Schaefer , D. W. and Hurd , A. J. 1990 . Growth and Structure of Combustion Aerosols—Fumed Silica . Aerosol Sci. Technol. , 12 : 876 – 890 .
- Sorensen , C. M. 2011 . The Mobility of Fractal Aggregates: A Review . Aerosol Sci. Techol. , 45 : 765 – 779 .
- Sorensen , C. M. , Cai , J. and Lu , N. 1992 . Light-Scattering Measurements of Monomer Size, Monomers per Aggregate, and Fractal Dimension for Soot Aggregates in Flames . Appl. Opt. , 31 : 6547 – 6557 .
- Sorensen , C. M. and Roberts , G. C. 1997 . The Prefactor of Fractal Aggregates . J. Colloid Interface Sci. , 186 : 447 – 452 .
- Sutherland , D. N. 1966 . Comments on Vold's Simulation of Floc Formation . J. Colloid Interface Sci. , 22 : 300 – 302 .
- Tence , M. , Chevalier , J. P. and Jullien , R. 1986 . On the Measurement of the Fractal Dimension of Aggregated Particles by Electron-Microscopy—Experimental-Method, Corrections, and Comparison with Numerical-Models . J. Phys. , 47 : 1989 – 1998 .
- Tolman , S. and Meakin , P. 1989 . Off-Lattice and Hypercubic-Lattice Models for Diffusion-Limited Aggregation in Dimensionalities 2–8 . Phys. Rev. A , 40 : 428 – 437 .
- Witten , T. A. and Sander , L. M. 1981 . Diffusion-Limited Aggregation: A Kinetic Critical Phenomenon . Phys. Rev. Lett. , 47 : 1400 – 1403 .