Abstract
Indoor ultrafine particles (UFP, <100 nm) undergo aerosol processes such as coagulation and deposition, which alter UFP size distribution and accordingly the level of exposure to UFP of different sizes. This study investigates the decay of indoor UFP originated from five different sources: a gas stove and an electric stove, a candle, a hair dryer, and power tools in a residential test building. An indoor aerosol model was developed to investigate differential effects of coagulation, deposition, and ventilation. The coagulation model includes Brownian, van der Waals, and viscosity forces, and also fractal geometry for particles of >24 nm. The model was parameterized using different values of the Hamaker constant for predicting the coagulation rate. Deposition was determined for two different conditions: central fan on versus central fan off. For the case of a central fan running, deposition rates were measured by using a nonlinear solution to the mass balance equation for the whole building. For the central fan off case, an empirical model was used to estimate deposition rates. Ventilation was measured continuously using an automated tracer gas injection and sampling system. The study results show that coagulation is a significant aerosol process for UFP dynamics and the primary cause for the shift of particle size distribution following an episodic high-concentration UFP release with no fans operating. However, with the central mechanical fan on, UFP deposition loss is substantial and comparable to the coagulation loss. These results suggest that coagulation should be considered during high concentration periods (>20,000 cm−3), while particle deposition should be treated as a major loss mechanism when air recirculates through ductwork or mechanical systems.
Copyright 2012 American Association for Aerosol Research
INTRODUCTION
Ultrafine particles (UFP) have been associated with adverse human health effects such as pulmonary and cardiovascular diseases (Bräuner et al. Citation2007; Stölzel et al. Citation2007). These have relatively large surface area, can carry toxic air pollutants, and have high alveolar deposition efficiency, presenting high potential to translocate to the circulation (Oberdörster et al. Citation2007). Given that people spend a majority of their time indoors (Klepeis et al. Citation2001), they are exposed to indoor UFP sources. These sources are mainly associated with activities of occupants such as combustion due to cooking and using consumer products that release UFP. The UFP emissions from these indoor sources are intermittent; however, the indoor UFP concentrations due to indoor sources are often much higher than those caused by outdoor UFP sources (Wallace and Howard-Reed Citation2002; Matson Citation2005).
Indoor UFP are subject to aerosol transport processes such as coagulation, deposition, and evaporation/condensation. In the indoor environment, ventilation is also a significant mechanism of loss of UFP. Several studies in the past decade have modeled indoor particle dynamics, including particle emission, deposition, and penetration (Lai and Nazaroff Citation2000; Long et al. Citation2001; Thatcher et al. Citation2002; He et al. Citation2004; Afshari et al. Citation2005; Wallace Citation2006; Hussein et al. Citation2009a, Citation2009b; Glytsos et al. Citation2010; Rim et al. Citation2010), even though only few studies have extensively monitored dynamics of size-resolved UFP in real buildings (Wallace Citation2006; Rim et al. Citation2010). Few researchers (Nazaroff and Cass 1989; Wallace et al. Citation2008; Glytsos et al. Citation2010) have investigated particle coagulation indoors, since it is quite challenging to simultaneously track the separate effects of coagulation, deposition, and ventilation. Even though considerable modeling efforts have been made previously to explore the aerosol coagulation theory and mathematical modeling (Whitby and McMurry Citation1997; Jacobson Citation2005; Seinfeld and Pandis Citation2006), experimental measurements to explore coagulation of indoor UFP are limited compared with research on outdoor UFP. Glytsos et al. (Citation2010) monitored UFP number concentrations due to several indoor sources and indicated that strong coagulation effects shift the particle size distribution to larger sizes during candle burning, smoking, onion frying, and hair dryer operation. Dennekamp et al. (Citation2001) reported that coagulation seems to be important for the evolution of UFP released from both gas and electric stoves.
However, a majority of earlier studies on indoor aerosol have not been able to track size-resolved coagulation of particles much smaller than 10 nm in diameter mainly due to instrument limitations; Wallace et al. (Citation2008) measured particles as small as 2.5 nm using the nano-differential mobility analysis techniques and discovered that some indoor sources, such as stovetop (but not oven) cooking with gas and electric stoves, emit >90% of the particles in the <10-nm range.
The effect of coagulation is more important for smaller particles (<10 nm) because of their high mobility. Besides particle size, particle coagulation is also a function of Brownian motion, van der Waals force, viscosity, and fractal aggregate dimension. Glytsos et al. (Citation2010) showed that the Brownian coagulation kernel estimated with the Fuchs correction can describe temporal change in number concentration with reasonable accuracy for particles larger than 10 nm. Brownian motion is random particle movement caused by collisions with surrounding gas molecules and is an important mechanism for particle coagulation (Nazaroff Citation2004). The Fuchs correction introduces the influence of particle size relative to particle mean free path and yields better predictions of coagulation kinetics for small particles in the transition and free molecular regimes (Seinfeld and Pandis Citation2006). Along with Brownian motion, the van der Waals force enhances the rate of coagulation due to the interaction of polarized dipoles and is particularly significant for dynamics of nano-sized particles (<50 nm). However, viscosity tends to reduce the likelihood of collision due to fluid resistance between approaching particles (Jacobson Citation2005). The nonspherical shapes of UFP, such as chain aggregates of a certain fractal dimension, are also important in estimating particle mobility and collision rate (Kostoglou and Konstandopoulos Citation2001). All these elements of a coagulation model were incorporated in our earlier study of gas and electric stoves and an electric toaster oven (Wallace et al., Citation2008).
However, Wallace et al. (Citation2008) made use of a theoretical deposition model for smooth surfaces based on the Lai and Nazaroff model (Citation2000). In the present study, we experimentally measured and developed a size-resolved UFP deposition curve for the case of central forced fan operating, based on more than 75 days of indoor and outdoor UFP measurements in a test house on the campus of the National Institute of Standards and Technology (NIST). The experimentally measured deposition rates are considerably higher than the Lai–Nazaroff model for smooth surfaces, and thus result in increased importance of deposition. Therefore, we have reinvestigated UFP dynamics due to the sources (gas and electric stove) described by Wallace et al. (Citation2008), and have added an experimental/modeling investigation of three consumer products (candle, hair dryer, and power tools). We have examined relative effects of coagulation and deposition on particle dynamics at various concentration levels. The sources considered here represent at least three different processes generating UFP: combustion, emissions from heating elements, and emissions from electric motors. Combustion of natural gas produces two types of particles: small spherical particles and larger aggregates of primary particles (Dobbins Citation2007). Heating elements are thought to produce UFP consisting of clusters of atoms leaving the surface (Schmidt-Ott Citation1988; Schripp et al. Citation2011). Electric motors produce copper particles from the action of graphite brushes on commutator (Szymczak et al. Citation2007).
For these varied sources of UFP, we have developed a particle dynamics model that predicts temporal changes in size distribution following an episodic release of high concentration UFP. Given that previous studies have explored the period of particle emission and reported source strengths as emission rates (Wallace et al. Citation2008; Buonanno et al. Citation2009; Glytsos et al. Citation2010), we focus on the period of decay to examine the differential effect of coagulation compared to deposition and ventilation. For this decay period, the model analyzes three indoor particle loss mechanisms: coagulation, deposition, and ventilation. The study also performs scaling analysis with physical parameters that influence coagulation and distribution of particle size.
METHODS
Dynamics of the indoor UFP size distributions were investigated using experimental measurements and analytical modeling. Experimental measurements were conducted in a full-scale single-storey test house, which consists of three bedrooms, two baths, a kitchen, and a dining room and a living area. The total floor area and volume of the house were 140 m2 and 340 m3, respectively. In the test house, particle monitoring tests were conducted from January 2007 to September 2010. The floor plan and details of the house layout are described in the paper published by Rim et al. (Citation2010). The house was furnished with limited tables, chairs, and measurement equipment, but was not fully furnished with bedroom and living room furniture. During the tests, one or two researchers occupied the house. A summary of the experimental measurements and test conditions is provided in . For each indoor particle source, three to five tests were conducted, with variations in the particle emission period and the central forced fan operating mode.
TABLE 1 Test conditions
In the tests with gas and electric stoves, particles were generated in the kitchen, the central forced fan was continuously running, and the Scanning Mobility Particle Sizer (SMPS) was in the master bedroom (MBR). All interior doors and registers were open. When the central mixing fan is running, particles deposit on the air filter and duct surfaces, as well as indoor surfaces. In this case, the measurement domain was the whole house. The mixing fan circulated complete air in the house through the ductwork every 10 min (six house volume of air per hour). We carefully examined the measurement data of tracer gas (SF6) at six different locations within the house. Using the multiple-position monitoring data, the relative standard deviation (RSD) of the SF6 concentrations was calculated for every 10-min interval to evaluate the uniformity of air mixing with the central mixing fan operating. For the particle measurement periods, the majority of the data had RSD < 10%, indicating that mixing was indeed rapid due to the operation of central fan during the experiments.
For the candle, hair dryer, and power tool (belt sander and power saws) tests, particles were generated in the MBR, the door was closed, the central fan was turned off, mixing fans ran in the MBR, and the SMPS monitored particles in the MBR. In these cases, the entire measurement area was a single room, with doors and registers sealed, so any delay due to achieving a well-mixed condition was negligible.
During the experiments, the indoor temperature ranged from 19 to 27°C and relative humidity (RH) ranged from 17 to 60%. The air change rate was measured using an automated monitoring system in the house. A tracer gas (SF6) was released into the living room at 4-h intervals and the decaying concentrations were monitored at 10-min intervals at six locations in the house (MBR, bedroom 2, living room, family room, dining room, and kitchen). The concentrations were measured using gas chromatography (GC) with electron capture detection (ECD), and the air change rate was estimated by regressing the natural logarithm of the tracer gas concentration versus time. The averaged air change rates observed for the particle emission and decay period in the test house ranged from 0.14–0.51 h−1. The uncertainty in the measured air change rate averaged 8% with a maximum of 15%, based on combining the two independent measurement uncertainties in quadrature: (1) the uncertainty due to incomplete air mixing plus precision (4.3 to 6.5%), and (2) the uncertainty due to calibration drift of the instrument (5%). The particle sources were turned on for 10–120 min, the subsequent particle decay was monitored for 60 min (twenty four 2.5-min scans), and the dynamic analytical model was applied to the decay period.
FIG. 1 Double logarithmic plot of particle size (Dp ) and deposition rates (k) for two different experimental conditions: (a) fan on, and (b) fan off. For the fan on condition (a), measured values (points) represent the deposition rates observed with the central fan operating. In this case, the measurement domain is the whole house and particles deposit on air filter, duct surfaces, and indoor surfaces. All error bars represent the standard error of the mean (SEM). A linear logarithmic equation is estimated for the measurements to evaluate the deposition rates for unmeasured particle sizes. For the fan off condition (b), modeled values were derived from the Lai–Nazaroff model. In this case, the entire domain is a single room and the deposition mainly occurred at the room surfaces.
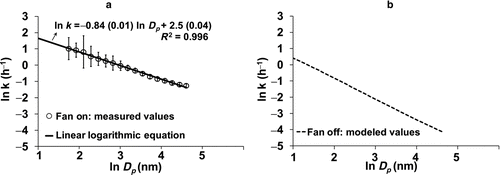
The particles from each source were monitored in the MBR using an SMPS (Model 3936, TSI, St. Paul, MN), which consists of an electrostatic classifier (Model 3080), a nano-DMA (Model 3085), and a water-based condensation particle counter (CPC; Model 3786). This system monitored particles ranging from 2–100 nm using two sheath flow rates of 15 L/s and 6 L/s with 10:1 sheath/aerosol flow ratio. It was extremely important to ensure that inlet sheath flow was laminar and accurately regulated, and precisely matched the exhaust flow. As small flow disturbances can result in decreased resolutions, aerosol flow rates were measured before and after each experiment using a bubble flow rate meter. At least three measurements were taken and all three were required to be within 3% of the desired flow rate. The sampling rate of UFP was set at 2.5 min: 2 min of measurement and 30 s for voltage to return to baseline. On the basis of the measurement efficiency curve for the water-based CPC, the minimum measurable particle size was 2 nm. In this study, a stronger radiation source (3080 N electrostatic classifier, TSI, Shoreview, MN) was used to improve the charging efficiency for the smallest particles. The uncertainty of measuring particle number concentration was estimated to be 12% based on combining the individual uncertainties of flow rate, particle charge distribution, voltage adjustment, and particle charge efficiency in quadrature (TSI Citation2007).
Two different sets of particle deposition rates were used depending on the fan-operating mode. For the tests that were performed with the central forced fan operating, the central fan circulated all the air in the house through the ductwork every 10 min and air mixing was rapid. Under these circumstances, the deposition rate was a composite of at least three processes: deposition to interior surfaces in the house; removal by the furnace filter (low efficiency mechanical filter); and deposition to ductwork. The integrated deposition loss was estimated using the mass balance equation. Measuring of detailed effects of the three individual deposition loss mechanisms is beyond the scope of our work and is described in Howard-Reed et al. (Citation2003). The present study used experimental measurements of particle deposition for 25 different data sets with the central mixing fan operating, each covering approximately three days (Rim et al. Citation2010). The points in are average deposition rates of 25 three-day periods for particles ranging from 5–100 nm; error bars are SDs. Using these average points, we regressed the data to estimate particle deposition rates for particle sizes from 2–5 nm. The linear logarithmic relationship yielded deposition rates for the whole house with the central mixing fan operating ranging from 0.28–6.8 h−1 for particle sizes of 2 to 100 nm ().
The other set of tests were run with the central fan off. For this case, the deposition rates were derived from the Lai–Nazaroff model (Citation2000), using a friction velocity of 3 cm/s. Typical friction velocity for indoor environment ranges from 0.3 to 3.0 cm/s, based on experimental and modeling studies on momentum boundary layer thickness and free stream bulk flows in rooms (Zhang et al. Citation1995; Lai and Nazaroff Citation2000). In our experiments with central fan off, we used mixing fans to achieve uniform air mixing in the room; therefore we used the higher value (3.0 cm/s) to model room deposition rates. We also used surface to volume ratio (1.9 m2/m3) and particles’ specific gravity of 1.0. In this case, the deposition rate (k) ranged from 0.016–2.0 h−1 for particle size between 2 nm and 100 nm ().
For each of the indoor sources, the source strength was calculated using particle losses due to deposition rate and air change rate, the peak total concentration, and the involved volume (Wallace et al. Citation2004) as shown in Equation (1)
Analytical Model
The measurements of particle dynamic behavior were analyzed with an indoor aerosol model that considered coagulation, deposition, and ventilation in a single and well-mixed air volume. Effects of condensation and evaporation were not considered, even though particle condensation might have increased particle mass in some tests. To address these effects, conservation of particle mass was checked and data affected by condensation and evaporation were excluded from the analysis. The governing equation for the analytical model is as follows:


The first two terms on the right-hand side in Equation (2) represent the particle gain and loss due to coagulation in which two particles collide and stick together. The third term represents the particle loss due to deposition and ventilation. The effects of outdoor particle penetration were not considered for the measurement period, since the peak UFP concentrations due to indoor sources are at least 30 times larger than that due to infiltrated outdoor particles.
In the present study, the concentration due to coagulation was solved by using the semi-implicit procedure in Jacobson (Citation2005) with a volume fraction factor (f) in order to preserve volume concentrations of the particles. A volume concentration (ρ q,t ) for size bin q can be written as
The particle size distribution at the start of the decay (peak total number concentration) was used as the initial concentration profile in the model. The coagulation kernel (β) can be affected by several factors: Brownian motion, van der Waals force, viscous force, fractal geometry, convective Brownian enhancement, gravitational sedimentation, and turbulent motions. The details for calculating the coagulation kernel are described in Jacobson (Citation2005). In the present study, we tested three coagulation models: (1) Brownian motion with the Fuchs correction [Brownian]; (2) Brownian motion with the Fuchs correction + van der Waals and viscous forces [Brownian +VDW]; and (3) Brownian motion with the Fuchs correction + van der Waals and viscous forces + fractal geometry [Brownian + VDW + Fractal 24]. With regard to fractal geometry, it was assumed that particles >24 nm could be particle aggregates that obey a power law: V ∼ Lx , where V is the particle volume, L is a characteristic length of the aggregate, and x is the fractal dimension. Treatment of aggregates as fractal particles increases the rate of coagulation. Fractal dimensions of soot aggregates tend to range between 1.5 and 3.0 (Xiong and Friedlander Citation2001), and for the present study a value of 1.7 was chosen for fractal dimension on the basis of the soot-aggregate study of atmospheric aerosol (Jacobson and Seinfeld Citation2004).
For each of the three models considered here, the study considered three values of the Hamaker constant: 20 kBT, 100 kBT, and 200 kBT (where kB is the Boltzmann constant and T is absolute temperature). The Hamaker constant is an empirical value that represents potential binding interaction between two colliding particles; in general it ranges from 6 × 10−20 J to 150 × 10−20 J and is greater for metal particles than organic particles (Hinds Citation1999). However, actual values are not known with precision.
illustrate the calculated coagulation kernel of any two particles between 2 nm and 100 nm. In the graph, the x- and y-axes represent the diameters of the particles. The contour values represent the collision rate between the two particle sizes. The figures indicate that the collision rate is the largest for a small particle colliding with a large particle. The collision rate between two small particles, particularly <50 nm, becomes larger when the effects of van der Waals and viscosity forces are considered (). The Hamaker constant also influences the coagulation kernel. Given that the Hamaker constant is a material-dependent factor that represents potential interaction between two particles, the collision rate increases as the Hamaker constant increases from 20 kBT to 200 kBT (). Fractal agglomerates lead to an increase in coagulation rate, especially for particles larger than 24 nm (). When a particle aggregate (>24 nm) collides with a small particle, the rate increases up to a factor of 7 compared with the case without the fractal effect.
FIG. 2 Coagulation kernel: (a) Brownian; (b) Brownian + VDW with the Hamaker constant of 20 kBT; (c) Brownian + VDW Hamaker constant of 200 kBT; (d) Brownian + van der Waals + Fractal 24 with the Hamaker constant of 200 kBT. Adding the fractal effect does not affect the collision rate within 24-nm limit. Note that the Hamaker constant affects the coagulation kernel only when van der Waals and viscosity forces (VDW) are considered.
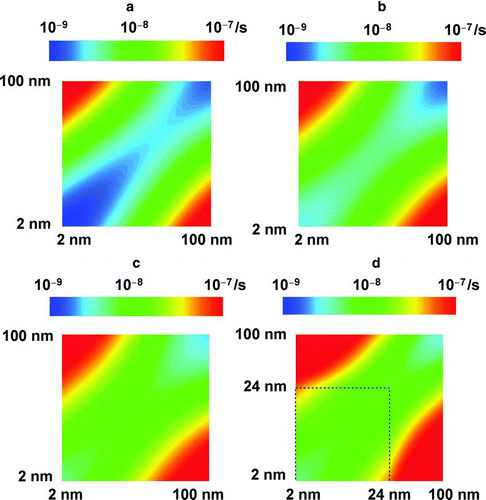
Using the calculated coagulation kernel and the initial particle size distribution observed during the decay period, the model predicted the evolution of the particle size distribution over the subsequent period. An analytical model fitting was performed against the experimental particle decay data. The fitting error was estimated as the root mean square (RMS) difference in particle number between the measurement and model prediction for eight consecutive scans (20 min) following the peak. Based on the fitting error, the present study found the best coagulation model and the optimal values of the Hamaker constant that most closely match the observed evolution of size distributions resulting from four indoor sources. The model results also provided differential effects of coagulation and deposition on ultrafine particle size dynamics under two different cases of air mixing.
RESULTS AND DISCUSSION
illustrate the model prediction and observations of evolution over time of the particle size distribution due to coagulation, deposition, and ventilation for the studied six indoor sources. The particle peak occurs between 4 nm and 10 nm for the gas stove, electric stove, candle, and belt sander, while the peak is larger than 10 nm for the circular saw and hair dryer. As seen in the figures, the particle size distribution shifts over time toward larger diameters. This shift mainly results from the coagulation effect: loss of small particles and gain of large particles due to particle collisions. The coagulation effect seems to be greater for small particles than for larger particles, given the faster decrease in number concentration of small particles. The fast disappearance of small particles occurs because of higher number concentrations and higher mobility of small particles than larger particles.
FIG. 3 Examples of analytical model fittings: (a) gas stove (GAS1), (b) electric stove (ELEC4), (c) candle burning (CAND1), (d) hair dryer (HAIR4), (e) belt sander (BELTS), (f) circular saw (CIRSAW). The numerals (N1, N3…) refer to the scans following the beginning of the decay period. The discrepancies between measurement and modeling are summarized as average root mean square errors in .
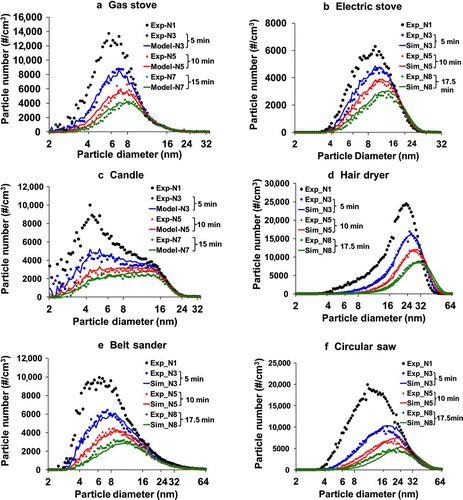
FIG. 4 Size-resolved change in number concentration between first and second scan due to coagulation, deposition, and ventilation: (a) gas stove (GAS1); and (b) hair Dryer (HAIR4). The central forced fan was operating in the GAS1 test, while it was off in the HAIR4 test. Note that the peak concentration for HAIR4 test was approximately twice that for the GAS1 test.
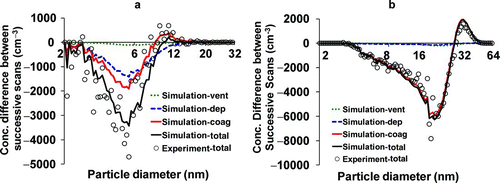
TABLE 2 Summary of the analytical model results
suggest that the temporal change in particle concentration is larger when the particle concentration is higher. This result supports the second-order dependence of coagulation rate on the particle number concentration as shown in Equation 2 (Nazaroff Citation2004). The particle number concentration becomes lower with time and the coagulation effect decreases. For instance, with the central fan operating in the house, when the UFP number concentration (2–100 nm) decreases down to 20,000 cm−3, the coagulation loss was approximately 20% of the deposition loss.
Due to the greater coagulation effect with higher number concentration, the model prediction of particle size distribution shift is better with higher particle concentrations and becomes less accurate as the number concentration reduces. This decrease in accuracy with lower number concentrations is likely due to the increased effect of deposition and ventilation, as well as the errors caused by local airflow effect. Also, the error accumulation with time marching in the model may also result in decrease in accuracy with lower concentrations. However, the figures suggest that the analytical model can predict the general pattern of change in the UFP size distribution, i.e., decreasing particle number and increasing geometric mean diameter with time.
illustrate differential effects of coagulation, deposition, and ventilation on the temporal change in particle number concentration between successive scans following the peak, with the simulation results from the analytical model shown by the lines. The figures also show the integrated change in total particle number observed in experimental measurements (dots). The measurement data of the change in total particle number are scattered around the analytical model results. illustrates a test with gas stove (GAS1) with the central forced fan operating. Because of the high deposition rate, the particle deposition loss is much higher than ventilation loss and nearly comparable to the coagulation loss. According to , the contributions of coagulation, deposition, and ventilation to the total particle number loss over the initial 2.5 min following the peak are 47%, 48%, and 5%, respectively. This result suggests that when a central fan mechanically recirculates air in a building, the influence of deposition loss on the change in particle size distribution can be substantial. However, as particle concentrations decrease with time, deposition tends to dominate over coagulation. shows results with the central fan turned off for one of the tests with the hair dryer (HAIR4). In this case, deposition loss only occurs on room surfaces; therefore the deposition loss is much smaller than the coagulation loss. In this case, the contribution of coagulation, deposition, and ventilation to the total particle loss over the initial 2.5 min are 93%, 4%, and 3%, respectively. This result demonstrates that when the central forced air fan is off, coagulation is dominant over deposition and ventilation.
summarizes the UFP size distribution and the best coagulation model/Hamaker constant for the four tested indoor sources. Based on the column showing best coagulation model, it appears that considering Brownian motion with the Fuchs correction, van der Waals and viscous forces are important in modeling indoor particle coagulation. The importance of Brownian forces and the Fuchs correction for modeling particle coagulation has been shown by previous studies (Nazaroff and Cass Citation1989; Seinfeld and Pandis Citation2006; Glytsos et al. Citation2010). The present study results suggest that van der Waals and viscous forces are also important for modeling indoor UFP coagulation. Van der Waals forces enhance particle coagulation due to random charge fluctuations and dipole–dipole attraction among uncharged particles. Previous studies have indicated that van der Waals forces significantly enhance the rate of coagulation of particles smaller than 50 nm, while viscosity forces reduce the van der Waals enhancement due to the fluid resistance between approaching particles (Jacobson Citation2005; Seinfeld and Pandis Citation2006). The enhancement due to van der Waals forces is a function of the Hamaker constant, which varies with the composition of particle.
The results in show the best fitting values of the Hamaker constant for the indoor sources, demonstrating that the optimal value of the Hamaker constant varies with indoor source and particle composition. In general, for the gas stove and candle, a value between 20 kBT and 100 kBT produces optimal fit to the experimental data, while a constant between 100 kBT and 200 kBT seems to be more appropriate for electric stove and hair dryer. This result suggests that particles from gas stove and candle likely consist of organic matter and those from electric stove and hair dryer are metal particles. Further studies of particle collection and imaging using Transmission Electron Microscopy (TEM) are planned to investigate more details of particle composition and binding interaction.
With regard to fractal effect, considering fractal geometry (24 nm) beside Brownian and van der Waals + viscosity forces does not substantially improve the model prediction for gas stove, electric stove, and candle. These results may be due to the fact that for these sources the geometric mean occurs at a particle size (<10 nm) much smaller than the fractal cutoff size (24 nm). On the other hand, the fractal effect seems to be notable for some of the hair dryer tests (HAIR1 and HAIR2) and power tool test (CIRSAW), in which the peak concentration occurs at particle sizes larger than 10 nm and 22 nm, respectively. It might be possible that fractal cutoff size close to the mode of the particle distribution may result in increased effect of fractal dimension on the analytical model fitting. Nonetheless, the fractal effect is unclear for the rest of the hair dryer/power tool tests, suggesting that Brownian and van der Waals + viscosity forces are more crucial factors to consider than fractal effects in modeling of the coagulation.
The best-fitting Hamaker constants found in this study agree with the findings of Wallace et al. (Citation2008). Although Wallace et al. (Citation2008) used only two alternate Hamaker constants of 20 kBT and 200 kBT, both studies found relatively lower Hamaker constants (20–100 kBT) for gas combustion sources and higher values (100–200 kBT) for heating element sources. However, in comparison to Wallace et al. (Citation2008), the present study suggests that particle deposition is a crucial process beside coagulation for indoor UFP dynamics, especially when a central air mixing system is operating. Also, this study indicates that the high UFP concentration occurs due to consumer products (candle, hair dryer, and power tools) and many of the released particles are smaller than 10 nm. However, the best-fitting coagulation model parameters vary with the UFP source, size distribution, and particle composition.
CONCLUSIONS
The present study investigated dynamic aerosol processes for evolution of the UFP size distribution following episodic releases of UPF in a residential building. Using experimental measurements of time-varying, size-resolved particle concentrations, the temporal change in the UFP size distribution was observed for five different indoor sources. Using the experimental data, the present study developed a particle dynamics model that analyzed the differential influence of coagulation, deposition, and ventilation on particle size dynamics. The study confirms previous findings that coagulation is an important aerosol process affecting UFP dynamics when particle concentrations are high due to indoor sources such as cooking (Wallace et al., Citation2008), and has extended these findings to several additional sources (candles, hair dryers, and power tools). The coagulation effect was higher with smaller UFP and higher number concentrations. The study results suggest that deposition should be considered depending on the operating mode of the central mechanical fan. When the central fan was turned on, particle deposition loss was substantial and comparable with the coagulation loss. When it was turned off, deposition loss was not significant and coagulation was the primary cause for the shift of UFP size distribution. Compared with coagulation and deposition, air change was a minor effect in these tests. Therefore, in terms of aerosol modeling for episodic indoor UFP releases, coagulation should be considered during high concentration periods (>20,000 cm−3), while deposition should be treated as a major loss mechanism when air recirculates through ductwork or mechanical systems.
The analytical model that considers Brownian, van der Waals/viscosity forces and fractal geometry along with deposition and ventilation can predict with reasonable accuracy the temporal particle size distribution due to indoor sources. The study results also suggest that using an appropriate Hamaker constant and deposition rate is essential for an accurate prediction of indoor UFP dynamics.
Disclaimer: The full description of the procedures used in this paper requires the identification of certain commercial products and their suppliers. The inclusion of such information should in no way be construed as indicating that such products or suppliers are endorsed by NIST or are recommended by NIST, or that they are necessarily the best materials, instruments, software, or suppliers for the purposes described.
Acknowledgments
Donghyun Rim's participation in this project was funded by the National Institute of Standards and Technology (NIST) through a US Intergovernmental Personal Act with the University of Texas at Austin. Michal Green participated in this study as a foreign guest researcher at the NIST while on sabbatical from the Israel Atomic Energy Commission; she contributed by collecting ultrafine particle data from consumer product tests. Jung-Il Choi is supported by Basic Science Research program (2011–0014558) and World Class University (WCU) program (R31–10049) through the National Research Foundation of Korea (NRF) funded by the Ministry of Education, Science and Technology. However, the manuscript does not necessarily reflect the views of these agencies and no official endorsement should be inferred.
REFERENCES
- Afshari , A. , Matson , U. and Ekberg , L. E. 2005 . Characterization of Indoor Sources of Fine and Ultrafine Particles: A Study Conducted in a Full-Scale Chamber . Indoor Air , 15 : 141 – 150 .
- Bräuner , E. V. , Forchhammer , L. , Møller , P. , Simonsen , J. , Glasius , M. Wåhlin , P. 2007 . Exposure to Ultrafine Particles from Ambient Air and Oxidative Stress-Induced DNA Damage . Environ. Health Perspect. , 115 ( 8 ) : 1177 – 1182 .
- Buonanno , G. , Morawska , L. and Stabile , L. 2009 . Particle Emission Factors During Cooking Activities . Atmos. Environ., , 43 : 3235 – 3242 .
- Dennekamp , M. , Howarth , S. , Dick , C. A. J. , Cherrie , J. W. , Donaldson , K. and Seaton , A. 2001 . Ultrafine Particles Andnitrogen Oxides Generated by Gas and Electric Cooking . Occup. Environ. Med. , 58 : 511 – 516 .
- Dobbins , R. A. 2007 . Hydrocarbon Nanoparticles Formed in Flames and Diesel Engines . Aerosol Sci. Technol. , 41 : 485 – 496 .
- Glytsos , T. , Ondracek , J. , Djumbova , L. , Kopanakis , I. and Lazaridis , M. 2010 . Characterization of Particulate Matter Concentrations during Controlled Indoor Activities . Atmos. Environ. , 44 : 1539 – 1549 .
- He , C. , Morawska , L. , Hitchins , J. and Gilbert , D. 2004 . Contribution From Indoor Sources to Particle Number and Mass Concentrations in Residential Houses . Atmos. Environ. , 38 : 3405 – 3415 .
- Howard-Reed , C. , Wallace , L. A. and Emmerich , S. J. 2003 . Effect of Ventilation Systems and Air Filters on Decay Rates of Particles Produced by Indoor Sources in an Occupied Townhouse . Atmos. Environ., , 37 ( 38 ) : 5295 – 5306 .
- Hinds , W. C. 1999 . Aerosol Technology: Properties, Behavior, and Measurement ofAirborne Particles , New York : Wiley .
- Hussein , T. , Hruska , A. and Dohanyosova , P. 2009a . Deposition Rates on Smooth Surfaces and Coagulation of Aerosol Particles Inside a Test Chamber . Atmos. Environ. , 43 : 905 – 914 .
- Hussein , T. , Kubincová , L. , Džumbová , L. , Hruška , A. , Dohánzosová , P. Hemerka , J. 2009b . Deposition of Aerosol Particles on Rough Surfaces Inside a Test Chamber . Building Environ. , 44 : 2056 – 2063 .
- Jacobson , M. Z. 2005 . Fundamentals of Atmospheric Modeling , Cambridge , UK : Cambridge University Press . 2nd ed.
- Jacobson , M. Z. and Seinfeld , J. H. 2004 . Evolution of Nanoparticle Size and Mixing State Near the Point of Emission . Atmos. Environ. , 38 : 1839 – 1850 .
- Klepeis , N. E. , Nelson , W. C. , Ott , W. R. , Robinson , J. P. , Tsang , A. M. Switzer , P. 2001 . The National Human Activity Pattern Survey (NHAPS): A resource for Assessing Exposure to Environmental Pollutants . J. Exposure Anal. Environ. Epidemiol. , 11 : 231 – 252 .
- Kostoglou , M. and Konstandopoulos , A. 2001 . Evolution of Aggregate Size and Fractal Dimension During Brownian Coagulation . J. Aerosol Sci. , 32 : 1399 – 1420 .
- Lai , A. C. K. and Nazaroff , W. W. 2000 . Modeling Indoor Particle Deposition from Turbulent Flow Onto Smooth Surfaces . J. Aerosol Sci. , 31 : 463 – 476 .
- Long , C. M. , Suh , H. H. , Catalano , P. J. and Koutrakis , P. 2001 . Using Time- and Size-Resolved Particulate Data to Quantify Indoor Penetration and Deposition Behavior . Environ. Sci. Technol. , 35 : 2089 – 2099 .
- Matson , U. 2005 . Indoor and Outdoor Concentrations of Ultrafine Particles in Some Scandinavian Rural and Urban Areas . Sci. Total Environ. , 343 : 169 – 176 .
- Nazaroff , W. W. 2004 . Indoor Particle Dynamics . Indoor Air , 14 : 175 – 183 .
- Nazaroff , W. W. and Cass , G. R. 1989 . Mathematical Modeling of Indoor Aerosol Dynamics . Environ. Sci. Technol. , 23 : 157 – 166 .
- Oberdörster , G. , Stone , V. and Donaldson , K. 2007 . Toxicology of Nanoparticles: A Historical Perspective . Nanotoxicology , 1 : 2 – 25 .
- Rim , D. , Wallace , L. and Persily , A. 2010 . Infiltration of Outdoor Ultrafine Particles into a Test House . Environ. Sci. Technol. , 44 : 5908 – 5913 .
- Schmidt-Ott , A. 1988 . New Approaches to in Situ Characterization of Ultrafine Agglomerates . J Aerosol Sci. , 19 ( 5 ) : 553 – 557 .
- Schripp , T. , Kirsch , I. and Salthammer , T. 2011 . Characterization of Particle Emission from Household Electrical Appliances . Sci. Total Environ. , 409 ( 13 ) : 2534 – 2540 .
- Seinfeld , J. H and Pandis , S. N. 2006 . Atmospheric Chemistry and Physics-2nd Edition , Hoboken , New Jersey : Wiley .
- Szymczak , W. , Menzel , N. and Keck , L. 2007 . Emission of Ultrafine Copper Particles by Universal Motors Controlled by Phase Angle . J Aerosol Sci. , 38 ( 5 ) : 520 – 531 .
- Stölzel , M. , Breitner , S. , Cyrys , J. , Pitz , M. , Wolke , G. Kreyling , W. 2007 . Daily Mortality and Particulate Matter in Different Size Classes in Erfurt, Germany . J. Expo. Sci. Environ.Epidemiol. , 17 ( 5 ) : 458 – 467 .
- Thatcher , T. L. , Lai , A. C. , Moreno-Jackson , R. , Sextro , R. G. and Nazaroff , W. W. 2002 . Effects of Room Furnishings and Air Speed on Particle Deposition Rates Indoors . Atmos. Environ., , 36 : 1811 – 1819 .
- TSI . 2007 . Measuring Nanoparticle Size Distributions in Real-Time: Key Factors for Accuracy: Application Note SMPS-003 , Shoreview, , MN : TSI Incorporated .
- Wallace , L. 2006 . Indoor Sources of Ultrafine and Accumulation Mode Particles: Size Distributions, Size-Resolved Concentrations, and Source Strengths . Aerosol Sci. Technol. , 40 : 348 – 360 .
- Wallace , L. A. , Emmerich , S. J. and Howard-Reed , C. 2004 . Source Strengths of Ultrafine and Fine Particles Due to Cooking with a Gas Stove . Environ. Sci. Tech. , 38 : 2304 – 2311 .
- Wallace , L. A. and Howard-Reed , C. H. 2002 . Continuous Monitoring of Ultrafine, Fine, and Coarse Particles in a Residence for 18 Months in 1999–2000 . J. Air Waste Manage. Assoc. , 52 ( 7 ) : 828 – 844 .
- Wallace , L. A. , Wang , F , Howard-Reed , C. and Persily , A. 2008 . Contribution of Gas and Electric Stoves to Residential Ultrafine Particle Concentrations Between 2 nm and 64 nm: Size Distributions and Emission and Coagulation Rates . Environ. Sci. Tech., , 42 : 8641 – 8647 .
- Whitby , E. R. and McMurry , P. H. 1997 . Modal Aerosol Dynamics Modeling . Aerosol Sci. Tech. , 27 : 673 – 688 .
- Xiong , C. and Friedlander , S. K. 2001 . Morphological Properties of Atmosphericaerosol Aggregates . Appl. Phys. Sci. , 98 : 11851 – 11856 .
- Zhang , J. S. , Shaw , C. Y. , Nguyen-Thi , L. C. , MacDonald , R. A. and Kerr , G. 1995 . Field Measurements of Boundary Layer Flows in Ventilated Rooms . ASHRAE Trans., , 101 ( Part 2 ) : 116 – 124 .