Abstract
Solar ultraviolet (UV) radiation plays a significant role in climate, atmospheric chemical processes, and ecosystem balance. Aerosol optical depth (AOD) at UV wavelengths, the UV-AOD, is an important quantity for studying the extinction of UV radiation in the atmosphere. Ground-based UV-AOD observations, such as those from the AErosol RObotic NETwork (AERONET), are limited in spatial coverage. Current space derived UV-AOD from the Total Ozone Mapping Spectrometer (TOMS) or the Ozone Monitoring Instrument (OMI), on the other hand, are subject to large errors associated with low resolution, cloud contamination, assumed height of aerosol layer, and low sensitivity to aerosols in the lower troposphere. In this study, a new UV-AOD product is derived for the year 2009 by extrapolating the Moderate Resolution Imaging Spectra-radiometer (MODIS) visible bands AOD product (VIS-AOD) to 380 and 340 nm. Results are evaluated against UV-AOD measurements taken at AERONET sites. Over the oceans, four extrapolating methods are investigated by using two to five wavelengths. The best result, which has a correlation coefficient (R) of 0.90 at both wavelengths and root mean square errors (RMSE) of 0.062 and 0.068 at 380 and 340 nm, respectively, is achieved by combining a linear-extrapolation and a second-order polynomial fitting that takes into account the wavelength dependence of the Ångström exponent. Moreover, more than 80% of the data fall within the uncertainty range of ±0.05 ± 0.20τ. Over land, UV-AOD is extrapolated using the Ångström exponent derived from VIS-AODs at 470 and 660 nm, the only two wavelengths available from the MODIS AOD product. Compared with AERONET observations, the correlation coefficient is about 0.90 at both 380 and 340 nm, while the RMSE increases to 0.152 at 380 nm and 0.174 at 340 nm, due to the larger uncertainty of MODIS AOD over land. With the relatively low biases, this UV-AOD product will be valuable for climate and atmospheric chemistry research.
Copyright 2012 American Association for Aerosol Research
1. INTRODUCTION
Solar ultraviolet (UV) radiation has great effects on climate change, atmospheric chemical processes, and ecosystem balance (Caldwell et al. Citation2007; Hader et al. Citation2007; Zepp et al. Citation2007). Atmospheric aerosols, through scattering and absorption, have significant impacts on UV radiation. The increase of anthropogenic aerosol loading since the industrial revolution is thought to have decreased surface UV radiation (Liu et al. Citation1991; Stanhill and Ianetz Citation1997). The spatial and temporal impacts of aerosols on UV radiation significantly affect the atmospheric chemical processes in the troposphere, especially for the ozone photochemical reaction in the boundary layer due to the dominant role of ozone in absorbing UV-B radiation (Andreae and Crutzen Citation1997; Madronich et al. Citation1998; Pöschl Citation2005).
The atmospheric aerosol optical depth (AOD) at the ultraviolet band (UV-AOD) is an important parameter that describes the aerosol impact on UV radiation. Observations at near UV wavelengths are helpful for detecting absorbing aerosols. Firstly, near-UV measurement is highly sensitive to aerosol absorption, but insensitive to aerosol phase function effects as discussed by Torres et al. (Citation1998). The study showed that in the 320–400 nm range the use of spherical particle approximation to retrieve aerosol products produces smaller errors than in the visible bands. Secondly, the low near UV surface albedo reduces the sensitivity errors from assumed values of surface albedo when BRDF effects on space-borne measurements are neglected (Herman and Celarier Citation1997; Koelemeijer et al. Citation2003).
Measurements of UV-AOD with high accuracy are available from many ground based observation networks, such as the AErosol RObotic NETwork (AERONET). A comprehensive dataset of aerosol properties, including AOD, complex refractive index, Ångström exponent, aerosol distribution, spectral single scattering albedo (SSA), and asymmetry parameter, are retrieved from the sunphotometer measurements of AERONET (Holben et al. Citation1998; Holben et al. Citation2001). These data go through rigorous calibration and cloud screening processes. However, the spatial coverage of the surface networks is limited. Space-borne measurements, on the other hand, have the advantage of covering a large area at a relatively high temporal resolution (King et al. Citation1999).
Currently, the main satellite products of aerosol UV observations are provided by the Total Ozone Mapping Spectrometer (TOMS) and the Ozone Monitoring Instrument (OMI). Measurement in the UV range from space is a useful method for detecting absorbing aerosols (smoke and dust), which cannot be effectively discriminated in the visible range. Measurement in the UV range has another advantage of being able to detect aerosols above backgrounds, which are bright in the visible range, such as clouds and snow (Torres and Bhartia Citation1999; Torres et al. Citation2007; Torres et al. Citation2010). The main UV aerosol products retrieved from TOMS measurements are AODs at 340 and 380 nm and Aerosol Index (AI) dating back to 1976. The total column amount of aerosol in the atmosphere during daylight hours were retrieved from TOMS measurements, providing monthly averages of UV-AOD on a 1° × 1° resolution over land and ocean until 2000. The TOMS-derived optical depths of UV-absorbing aerosols are within 30% of AERONET measurements, and for the nonabsorbing optical depths, the agreement is about 20% (Torres et al. Citation2002). Launched in 2004, OMI has become the main provider of global UV-AOD. OMI UV aerosol products contain AOD, AAOD (Aerosol Absorption Optical Depth), SSA, and AI. The main problem of directly detecting AOD from UV band radiation from space is the strong molecular scattering in the boundary layer. TOMS or OMI UV aerosol products are sensitive to the absorption of dust or smoke in the free atmosphere rather than that in the boundary layer where most of the anthropogenic pollution exists. UV observations are also very sensitive to the height of aerosol layer, which is usually an unknown parameter for aerosol optical property retrieval. Therefore, AOD products from direct satellite monitoring at UV bands still need to be improved to satisfy the requirements of climate, ecological, and atmospheric chemistry studies. Some challenges include low spatial resolutions, cloud contamination, uncertainty of the assumed aerosol layer height, and insensitivity to the boundary layer aerosols (Torres et al. Citation1998; Torres et al. Citation2001). Comparing space-borne UV-AOD at 388 nm from OMI's OMAERUV product with AERONET measurements at 380 nm over four AERONET desert sites, the correlation coefficient is about 0.74, and the root mean square error (RMSE) is 0.26 (Torres et al. Citation2007). The AOD derived from OMI shows more sensitivity to cloud contamination than the AAOD, and the RMSE for daily cloud-free collocated AOD between OMI and MODIS (Moderate Resolution Imaging Spectra-radiometer) is 0.15 (Ahn et al. Citation2008). Hence, more studies need to be done to retrieve AOD products at UV bands from satellite measurements.
MODIS aboard both NASA's Terra (launched in 1999) and Aqua (launched in 2002) satellites provides measurements to derive spectral AOD at visible channels (VIS-AOD) with relatively high spatial resolution of about 10 km × 10 km (Kaufman et al. Citation1997a; Tanré et al. Citation1997). The MODIS aerosol retrieval algorithm is mainly applied over dark surfaces (Kaufman et al. Citation1997b; Remer et al. Citation2005), and the “Deep Blue” algorithm can be used over desert at short wavelengths, where surface reflectivity is low (Hsu et al. Citation2004). Compared with AERONET and some other individual measurements around the world, MODIS VIS-AOD falls within the predicted uncertainty of Δτ = ±0.03 ± 0.05τ over ocean and of Δτ = ±0.05 ± 0.15τ over land (Chu et al. Citation2002; Chu et al. Citation2003; Li et al. Citation2003; Li et al. Citation2005; Remer et al. Citation2005). The improved second-generation of VIS-AOD over land validated with AERONET shows a linear relation of y = 1.01x + 0.03, and a correlation coefficient of R = 0.9, with a better ability to retrieve fine mode aerosols (Levy et al. Citation2007). Since AOD and its spectral dependence can be expressed as a power law relationship (Angstrom Citation1929), and a second order fit for multiwavelength satellite observations can be used to obtain the spectral relationship of AOD versus wavelength (Eck et al. Citation1999), it is possible to derive UV-AOD from VIS-AOD as shown in many studies based on ground measurements (Eck et al. Citation1999; Hu et al. Citation2007; Satheesh et al. Citation2009).
In this study, four approaches are investigated to extrapolate UV-AOD from MODIS VIS-AOD products. Results are evaluated against the AERONET measurements. Data used in this study and the extrapolation methods are presented in Section 2. Validation of results is conducted in Section 3. Finally, summary and conclusions are given in Section 4.
2. DATA AND METHODS DESCRIPTION
2.1. Data
The MODIS level-2 aerosol VIS-AOD products (C005) (Levy et al. Citation2007) from both the Terra (MOD04) and Aqua (MYD04) satellites in 2009 (http://ladsweb.nascom.nasa.gov/) were used in this analysis. The simultaneously observed UV-AOD data at both 380 and 340 nm from the global AERONET stations (74 sites over land and 25 sites over ocean) were applied in comparison with satellite remote sensing data (http://aeronet.gsfc.nasa.gov). The version of AERONET data is level 2.0 which means calibration and cloud screening have been done for the data. shows the distribution of the AERONET stations used in the comparisons. The diamonds in the figure show the locations of the AERONET stations over land and the dots are those over ocean. The existing 2009 space-borne OMI near-UV aerosol algorithm (OMAERUV) AOD products (V003) at 354 and 388 nm, and multiwavelength algorithm (OMAERO) AOD products (V003) at 342 and 388 nm (http://disc.sci.gsfc.nasa.gov) were also incorporated in this study for comparison purposes.
2.2. Extrapolation Theory
AOD and its spectral dependence can be expressed approximately as a power law relationship (Angstrom Citation1929):
2.3. Extrapolation Methods for MODIS Aerosol Product
2.3.1. Extrapolation Over Ocean
The extrapolation methods using the theories discussed above have been tested by using AERONET VIS-AOD. The extrapolated UV-AOD agrees well with direct observations as shown in Supplemental Information 1. Therefore, the extrapolation methods can be applied to MODIS AOD data.
Based on the theory discussed in Section 2.2, the following four methods were applied to extrapolate UV-AOD at 340 and 380 nm from MODIS VIS-AOD over ocean:
a. | Method 1: Ignoring the curvature, the MODIS AOD at 470 nm was extrapolated to 340 and 380 nm, by using the Ångström exponent calculated from MODIS AOD at 470 and 660 nm through Equation (2) (Nakajima and Higurashi Citation1998). | ||||
b. | Method 2: For the first step, the coefficients in EquationEquation (3) were derived from MODIS AOD at 470, 550, 660, and 870 nm. Then for the second step, EquationEquation (3) was used again to obtain UV-AOD at 340 and 380 nm for the cases with negative curvatures (α 2 < 0). For the cases with positive curvatures (α 2 ≥ 0), a linear least square fitting (in a log-log scale, EquationEquation (4)) for MODIS AOD at 470, 550, 660, and 870 nm was used to extrapolate UV-AOD at 340 and 380 nm (Satheesh et al. Citation2009). The reasons for this method are described in Section 3.2. | ||||
c. | Method 3: EquationEquation (3) was applied for all observations, no matter whether the curvature was positive or negative. | ||||
d. | Method 4: The linear least square fitting (in a log-log scale) was applied for all data, ignoring the curvature (Satheesh et al. Citation2009), which can be expressed as EquationEquation (4). |
2.3.2. Extrapolation over land
Because MODIS AOD is retrieved only at two visible channels (470 and 660 nm) with interpolated product at 550 nm over land, the UV-AOD over land was only extrapolated by using Method 1, i.e., the Ångström exponent was calculated from MODIS AOD at 470 and 660 nm, and then the UV-AOD at 380, 340 nm was obtained from AOD at 470 nm by applying the Ångström exponent to EquationEquation (1).
3. RESULTS VALIDATION
3.1. Validation of MODIS AOD at Visible Channels (470, 550, 660, and 860 nm)
Because UV-AOD is extrapolated from VIS-AOD, its quality is very sensitive to the accuracy of MODIS products at visible bands. The MODIS aboard both NASA's Terra and Aqua satellites has a fine spatial resolution and a wide spectral range ensuring a reliable cloud screening, which makes the aerosol products significantly less affected by cloud contamination (Kaufman et al. Citation1997a; Tanré et al. Citation1997). Previous studies showed that the retrieved AOD is at a high quality, especially over ocean, falling within the predicted uncertainty of Δτ = ±0.03 ± 0.05τ over ocean and Δτ = ±0.05 ± 0.15τ over land (Chu et al. Citation2002; Remer et al. Citation2005) compared with AERONET data. The MODIS aerosol products in 2009 remain high quality as shown in Supplemental Information 2. The correlation coefficients between MODIS and AERONET data range from 0.86 to 0.90 over ocean, and between 0.84 and 0.86 over land. Therefore, the high quality of MODIS VIS-AOD products in 2009 enables the extrapolation of UV-AOD with considerable accuracy.
3.2. Validation of Extrapolated UV-AOD Over Ocean
Over ocean, UV-AOD was derived from both MOD04 and MYD04 products using the four methods described in Section 2.3.1. The comparisons between the derived UV-AOD and those observed by AERONET are shown in and b for wavelengths at 380 and 340 nm, respectively. We can see that the extrapolated UV-AOD from MOD04 and MYD04 products using the same extrapolation method are similar to each other. However the extrapolated UV-AOD from MOD04 products show slightly better correlations with AERONET observations than the ones extrapolated from MYD04 products, although the MODIS VIS-AOD from the two products are similar. For the UV-AOD extrapolated using the same method, the extrapolation at 380 nm is better than the one at 340 nm, because the shorter the wavelength, the longer the extrapolation distance, and the larger the error becomes.
FIG. 2 (a) Comparisons of the extrapolated UV-AOD at 380 nm over ocean with AERONET observations. The solid diagonal line in the middle represents the line y = x, and the two solid lines above and below the middle line are y = 0.05 + 1.2x and y = −0.05 + 0.8x, respectively, corresponding to the uncertainty estimation of Δτ = ±0.05 ± 0.20τ. The dashed line is for the linear fitted result. The sub-plots 1–4 stand for the results using Method 1–4, respectively. For each method, the above plot uses MOD04 AOD data from Terra to extrapolate to 380 nm, and the lower one uses MYD04 data from Aqua. Most of the four methods show a good correlation (Rs range from 0.88 to 0.91) with AERONET data. Method 2 shows the lowest RMSEs (RMSEs from 0.062 to 0.071). Method 3 shows a relatively low R at 380 nm (R = 0.88 for MOD04 AOD). (b) The same as , but for UV-AOD at 340 nm. Rs range from 0.87 to 0.91, RSMEs from 0.068 to 0.106. Method 3 shows a relatively low correlation (R = 0.87) with AERONET values. Method 2 still shows least RMSE among all the methods.
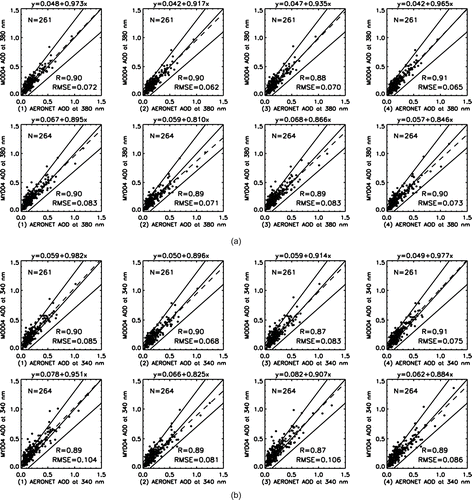
and b also show that Method 3 performs the worst in terms of both R and RMSE, especially at 340 nm. While results from both Methods 2 and 4 agree well with AERONET observations, Method 2 has the lowest RMSE because the wavelength dependence of the Ångström exponent was taken into consideration. The result of Method 2 shows 83.9% of the UV-AOD extrapolated for MOD04 falls into an uncertainty of Δτ = ±0.05 ± 0.20τ at 380 nm, and 81.6% at 340 nm. For MYD04, they are 77.7% and 75.4% for wavelengths at 380 and 340 nm, respectively (). The standard deviation (δ) of slope and intercept in the UV-AOD fitted relationship are shown in . The δs of intercept range from 0.006 to 0.008, and slope δs are from 0.024 to 0.032. The intercept δs are close to each other, but the slope δs of Method 2 show the least value, which means best-fitted relationship.
TABLE 1 Percentage of derived UV-AOD falling into uncertainty of (Δτ=±0.05±0.2τ)
TABLE 2 The fitted relationships of UV-AOD validation by AERONET and the corresphonding standard deviation (δ) for the slope and intercept
and b show examples of the extrapolation using Methods 3 and 4 for the types of aerosols with positive and negative curvatures, respectively. The extrapolated values using curvature fitting (Method 3) are closer to the AERONET values than the ones using linear least square fitting (Method 4) when the curvatures are negative for fine types of aerosols. The linear least square fitting results in higher values compared with the AERONET data when the curvature is negative (, curvatures are –1.45, –1.52, and –1.57). However, curvature fitting results in higher values than the linear least square fitting and agrees less with AERONET data when the curvature is positive (, curvatures are 1.13, 1.39, and 1.59). The reason is that positive curvature results from coarse aerosol particles whose Ångström exponents change very little and are close to zero. Therefore, the linear method, which has zero curvature, is better than the curvature fitting. Negative curvature implies fine particles whose Ångström exponents are larger and have significant dependence on wavelengths, which was found in previous studies (Eck et al. Citation1999; Reid et al. Citation1999). The second-order fit accounts for the curvature changes. Therefore, it performs better for fine particle retrievals where Angstrom exponents vary significantly. The poor correlation ( and b) of Method 3 results from MYD04 data are similar to the condition shown in . More UV-AOD values are higher than AERONET data. For MOD04 data, there are 164 of 261 data with positive curvature, and 202 out of 264 with positive curvature for MYD04 data, which means that many conditions dominated by coarse particles occur over the ocean, so that this Method has more overestimation than the others.
Method 3 takes the curvature change into consideration, resulting in a better agreement with AERONET observations than Method 4 for fine particles as demonstrated in Table S2 of Supplemental Information 4. Because most aerosols over ocean are coarse particles, such as sea salt, the advantage of taking the curvature into consideration is not so evident on a global scale for a single year. Method 2 is a combination of Methods 3 and 4. The curvature (representing particle size) is considered for both kinds of aerosols, Method 3 for fine particles and Method 4 for coarse particles. Method 2 shows the best result, with more data falling into the uncertainty range of Δτ = ±0.05 ± 0.20τ at both 380 and 340 nm, and smaller biases than Methods 3 and 4, though the improvement is not very evident, because there are not many fine particles over ocean. Method 1 just uses AOD at 470 and 660 nm, so it strongly depends on both bands. Method 4 uses AOD at 4 bands, which reduces the dependency on an individual band. Thus, Method 4 performs better than Method 1.
An uncertainty analysis for Method 2 was made by taking aerosol size into consideration. The aerosol data over ocean in 2009 was analyzed according to whether the dominant aerosols are coarse or fine particles based on examining the value of the AOD curvature. Then, the uncertainty of extrapolated UV-AOD was tested. MODIS VIS-AOD falls into an uncertainty of ±20%, which contains the errors caused by surface reflectance, cloud contamination, and aerosol model. The averages of the AOD at 470, 550, 660, and 870 nm were used to get UV-AOD at 380 and 340 nm. A ± 20% bias was given to MODIS AOD at randomly selected wavelengths, which results in an average of ±20% uncertainty in UV-AOD for fine particles when the curvature fitting method is applied. The analysis results are consistent with the validation results presented in and b. The highest bias (30%–40%) is caused by the errors of VIS-AOD at 550 and 660 nm, which have a large impact on the curvature calculated from EquationEquation (3). The linear least fitting method for coarse dominated aerosols shows a small uncertainty of ±5%, which means the linear function is less affected by MODIS VIS-AOD. Therefore, fine particle aerosol is more affected by the uncertainty of MODIS data. The total averaged uncertainty for Method 2 is about ±20%. The bias analysis is shown in Table S2. Bias between the extrapolated UV-AOD and the AERONET values is calculated at 380 and 340 nm for coarse and fine particles. The bias is small (<0.0836) when the atmospheric AOD over ocean is less than 0.5. However, the bias is usually higher than 0.1 when the AOD is large, especially for the interval of 1.0–1.5. Table S2 shows the bias of Method 3 for coarse particles is the largest, which means the curvature fitting method is not suitable for calculating coarse particle dominant aerosols. The fine particle bias of Method 2 is smaller than the one of Method 4. The results in Table S2 are also consistent with the results shown in .
FIG. 3 The relationship between AOD and wavelengths in a log-log scale for the positive curvature (a) and negative curvature (b). Triangles stand for MODIS VIS-AOD at 470, 550, 660, and 860 nm. Stars stand for AERONET UV-AOD at 340 and 380 nm; and diamonds for extrapolated MODIS UV-AOD using curvature fitting (dashed line); the solid line stands for linear least square fitting. The curvature method works better than linear least square fitting method for negative curvature condition (the fine particle aerosols), because of the spectral dependence of fine particles. And linear least square fitting method do a better job when aerosol is coarse particle dominated, because Angstrom Index of coarse particles is close to zero with little change.
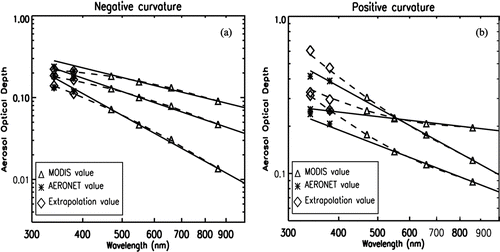
3.3. Validation of Extrapolated UV-AOD over land
As discussed in Section 2.3.2, UV-AOD over land was extrapolated by using only Method 1 due to the fact that MODIS VIS-AOD was only retrieved at two visible channels over land. Comparisons of the extrapolated UV-AOD from both MOD04 and MYD04 with AERONET values are shown in with R = 0.90 for the extrapolated UV-AOD at 380 nm and R = 0.89 at 340 nm. RMSEs are larger at 340 nm than at 380 nm. About 63% ∼ 70% of the data falls within the uncertainty range of ±0.05 ± 0.20τ (). MODIS AOD over land has higher errors than that over ocean because of the complexity of MODIS aerosol retrieval over land. However, the results still have reasonable accuracy for further application in climate, ecology, and atmospheric chemistry studies. The uncertainty of the land method is ±20%, mainly caused by the uncertainty of MODIS AOD over land. From the bias analysis in Table S2, we can see that the bias is low, ranging from –0.053 to 0.053 at two UV wavelengths. The bias is mainly positive when the UV-AOD is less than 0.3, and negative when the UV-AOD is in the range 0.3–1.5. This means that the UV-AOD extrapolated from MODIS products is underestimated, compared with AERONET measurements, in high aerosol loading cases, and overestimated for low aerosol loading cases. shows the standard deviation (δ) of slope and intercept in the fitted relationships of UV-AOD validation with AERONET. Both δs of slope and intercept are small, they are from 0.005 to 0.006 for intercept δ and 0.009 to 0.011 for slope δ.
FIG. 4 Comparisons of the extrapolated UV-AOD at 340 and 380 nm over land with AERONET observations. Only Method 1 is used over land because of limited bands of MODIS aerosol products. The extrapolated UV-AOD derived from MOD04 and MYD04 still shows a nice correlation with AERONET values as it does over ocean (Rs range from 0.89 to 0.90, RMSEs from 0.152 to 0.181). RMSEs are higher than the ocean extrapolated UV-AOD.
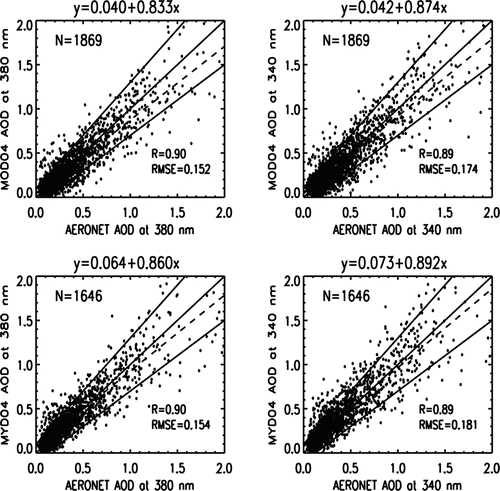
3.4. Validation of OMI OMAERUV and OMAERO Products
As a comparison, the AOD at UV bands retrieved from OMI was also compared with AERONET data over both ocean and land. OMI is aboard the Aura satellite which is a member of A-train (including Aqua-MODIS), measuring the upwelling radiance at the top of the atmosphere between 270 and 500 nm. It has a 2600 km wide swath and a spatial resolution varying from 13 km × 24 km at nadir to 28 km × 150 km at extremes (Levelt et al. Citation2006). The UV algorithm used by OMI has an outstanding ability of detecting absorbing aerosols like dust and smoke, even retrieving absorbing aerosols above clouds, ice, and snow (Torres et al. Citation1998; Torres et al. Citation2007; Torres et al. Citation2010). These are advantages over VIS detection. OMI products include AOD, AAOD, SSA, and AI at UV bands. AAOD is at a higher quality than AOD (Ahn et al. Citation2008). Near-UV aerosol algorithm (OMAERUV) AOD products at 354 and 388 nm and multiwavelength algorithm (OMAERO) AOD products at 342 and 388 nm were obtained from the NASA data server for the year 2009. They were compared with AERONET AOD at 340 and 380 nm. Each OMI data was made for the spatial mean of AOD from a 3 × 3 box centered on the AERONET station, and AERONET AOD was temporally averaged over ±30 min of OMI overpass. OMAERUV values with the highest quality retrievals (“AlgorithmFlags” = 0) were used. For OMAERO data, the retrievals whose root mean square of residual reflectance fell below 0.003 were chosen (Livingston et al. Citation2009).
The comparisons of OMAERUV and OMAERO UV-AOD products with AERONET values are shown in Figures S2a and b in Supplemental Information 3. Both OMAERUV and OMAERO overestimate UV-AOD over both ocean and land, with low correlation coefficients of R = 0.42–0.60. The UV-AOD over land is better than that over ocean, because land is more favorable for retrieval at UV wavelength than ocean. UV algorithm is insensitive to surface reflectance, which can reduce the errors caused by the estimation of surface reflectance. There are also fewer clouds over land than over ocean, which means less probability of cloud contamination. Cloud contamination caused by the low resolution of the OMI instrument is the main reason for overestimation. Previous evaluations of OMI aerosol products, compared with MODIS AOD and AERONET data, were all under cloud-free conditions for individual sites and for a short period of time (Ahn et al. Citation2008; Curier et al. Citation2008; Torres et al. Citation2007). Comparing space-borne UV-AOD at 388 nm from OMI's OMAERUV product with AERONET measurements at 380 nm over four AERONET desert sites, the correlation coefficient is about 0.74 (Torres et al. Citation2007). The OMAERO AOD for Europe and adjacent oceans yields correlation coefficients between 0.76 and 0.81 for ocean and between 0.59 and 0.70 for land, when compared with MODIS AOD (Curier et al. Citation2008). However, the evaluation of OMI aerosol products at a global scale for as a long period as a year will be unavoidably impacted by cloud contamination. Furthermore, various surface types over the globe, such as remote areas with clean boundary layers, urban polluted areas, oceans, and coastal areas, will complicate the product retrieval. The correlation between OMI and AERONET is therefore much lower here. Although the UV observations have advantages on detecting dust or biomass burning aerosols in the free atmosphere, especially for those over bright surfaces on visible bands, the UV measurements are not sensitive to the boundary layer aerosols and depend on assumed heights of aerosol layers due to strong molecular scattering. In addition, the OMI UV-AOD products are easily affected by sub-pixel cloud contamination due to low spatial resolution. Therefore, AOD products retrieved from direct satellite monitoring at UV bands still need to be improved. Compared with both the validation in this study and previous research, we can see the extrapolated MODIS UV-AOD is better than the OMI UV-AOD evaluated by AERONET data.
4. SUMMARY AND CONCLUSIONS
UV-AOD is an important quantity for atmospheric research, especially related to aerosol radiative forcing and atmospheric chemical reactions. Compared with the limited number of ground-based observations, satellite products have the advantage of deriving UV-AOD at a global scale. The existing OMI instrument has a good ability of detecting absorbing aerosols, even above bright backgrounds, which are difficult to be retrieved by VIS algorithm. AAOD, SSA, and AI are important products for researching the absorbing ability of aerosols, which greatly affect radiation transfer and aerosol chemistry. However, AOD retrievals from direct UV wavelength measurements have their shortcomings, including coarse spatial resolutions, assumed aerosol layer height, cloud contamination and insensitivity to boundary aerosols. MODIS performs well in detecting aerosols at visible wavelengths with a 10 km × 10 km resolution over land covered by dense dark vegetation and most oceans. Extrapolating MODIS AOD from visible channels to UV wavelength provides a good opportunity to obtain UV-AOD at a global scale with high spatial and temporal resolutions.
In this study, four methods over ocean and one method over land were investigated to extrapolate UV-AOD at 340 and 380 nm from MODIS level-2 VIS-AOD products (C005). Results show that the extrapolated UV-AOD correlated well with AERONET observations over oceans, especially when the curvature fitting is used for negative curvature and linear least square fitting for positive curvature conditions. The correlation coefficient can be as high as 0.90 (0.90) and RMSE can be as low as 0.062 (0.068) at 380 nm (340 nm). 83.9% of the UV-AOD extrapolated for MOD04 products falls into an uncertainty range of Δτ = ±0.05 ± 0.20τ at 380 nm, and 81.6% at 340 nm. The extrapolated UV-AOD over land also has a high correlation coefficient with AERONET observations and the RMSEs are 0.152 and 0.174 at 380 and 340 nm, respectively. Although the RMSEs over land are slightly larger than the ones over ocean due to the relatively large uncertainty of the MODIS VIS-AOD land products, they are still smaller than OMI UV-AOD products.
In summary, this study demonstrates that it is possible to derive high quality UV-AOD from MODIS VIS-AOD products at a global scale with high spatial and temporal resolutions. It should be noted that the quality of the derived UV-AOD depends highly on the VIS-AOD products. For the case of MODIS products, VIS-AOD over land is not as good as over ocean due to the complex land surface which results in less accuracy in extrapolated UV-AOD. Therefore, it is important to ensure that the AOD at VIS bands is highly accurate for high quality UV-AOD extrapolation. The extrapolated UV-AOD can also help to improve direct retrieval from UV bands, such as OMI UV-AOD. As both MODIS (aboard Aqua) and OMI (aboard Aura) are members of the A-Train satellites, there is a good opportunity in future studies to combine their advantages in AOD retrieval.
uast_a_687475_sup_25767426.zip
Download Zip (4.4 MB)Acknowledgments
The study is partially supported by the research grants from the National Natural Science Foundation of China (NSFC, Grant No.: 40775002 and 41175020), the National High Technology Research and Development Program (863 Major Project, Grant No.: SQ2010AA1221583001) of China. The authors thank the principal investigators and their staffs for establishing and maintaining the AERONET sites used in this investigation. The authors gratefully acknowledge the MODIS Science Data Support Team and the Earth Observing System Data Gateway for processing and distributing MODIS data used in this article, respectively. The authors also gratefully acknowledge the OMI team and the NASA Goddard Earth Sciences Data and Information Services Center for providing the OMI aerosol products used in this article.
[Supplementary materials are available for this article. Go to the publisher's online edition of Aerosol Science and Technology to view the free supplementary files.]
REFERENCES
- Ahn , C. , Torres , O. and Bhartia , P. K. 2008 . Comparison of Ozone Monitoring Instrument UV Aerosol Products with Aqua/Moderate Resolution Imaging Spectroradiometer and Multiangle Imaging Spectroradiometer Observations in 2006 . J. Geophys. Res.-Atmos. , 113 : 13
- Andreae , M. O. and Crutzen , P. J. 1997 . Atmospheric Aerosols: Biogeochemical Sources and Role in Atmospheric Chemistry . Science , 276 : 1052 – 1058 .
- Angstrom , A. 1929 . On the Atmospheric Transmission of Sun Radiation and on Dust in the Air . Geografiska Annaler , 11 : 156 – 166 .
- Caldwell , M. M. , Bornman , J. F. , Ballare , C. L. , Flint , S. D. and Kulandaivelu , G. 2007 . Terrestrial Ecosystems, Increased Solar Ultraviolet Radiation, and Interactions with Other Climate Change Factors . Photochem. Photobiol. Sci. , 6 : 252 – 266 .
- Chu , D. A. , Kaufman , Y. J. , Ichoku , C. , Remer , L. A. , Tanré , D. and Holben , B. N. 2002 . Validation of MODIS Aerosol Optical Depth Retrieval Over Land . Geophy. Res. Lett. , 29 8007, DOI: 10.1029/2001GL013205
- Chu , D. A. , Kaufman , Y. J. , Zibordi , G. , Chern , J. D. , Mao , J. Li , C. C. 2003 . Global Monitoring of Air Pollution Over Land from the Earth Observing System-Terra Moderate Resolution Imaging Spectroradiometer (MODIS) . J. Geophys. Res. -Atmos. , : 108 4661, DOI: 10.1029/2002JD003179
- Curier , R. L. , Veefkind , J. P. , Braak , R. , Veihelmann , B. , Torres , O. and de Leeuw , G. 2008 . Retrieval of Aerosol Optical Properties from OMI Radiances Using a Multiwavelength Algorithm: Application to Western Europe . J. Geophys. Res. , 113 : D17S90
- Eck , T. F. , Holben , B. N. , Reid , J. S. , Dubovik , O. , Smirnov , A. O’Neill , N. T. 1999 . Wavelength Dependence of the Optical Depth of Biomass Burning, Urban, and Desert Dust Aerosols . J. Geophys. Res.-Atmos. , 104 : 31,333 – 31,349 .
- Hader , D. P. , Kumar , H. D. , Smith , R. C. and Worrest , R. C. 2007 . Effects of Solar UV Radiation on Aquatic Ecosystems and Interactions with Climate Change . Photochem. Photobiol. Sci. , 6 : 267 – 285 .
- Herman , J. R. and Celarier , E. A. 1997 . Earth Surface Reflectivity Climatology at 340 nm;380 nm from TOMS Data . J. Geophys. Res. , 102 : 28,003 – 28,011 .
- Holben , B. N. , Eck , T. F. and Fraser , R. S. 1991 . Temporal and Spatial Variability of Aerosol Optical Depth in the Sahel Region in Relation to Vegetation Remote Sensing . Int. J. Remote. Sens. , 12 : 1147 – 1163 .
- Holben , B. N. , Eck , T. F. , Slutsker , I. , Tanré , D. , Buis , J. P. Setzer , A. 1998 . AERONET–A Federated Instrument Network and Data Archive for Aerosol Characterization . Remote. Sens. Environ. , 66 : 1 – 16 .
- Holben , B. N. , Tanré , D. , Smirnov , A. , Eck , T. F. , Slutsker , I. Abuhassan , N. 2001 . An Emerging Ground-based Aerosol Climatology: Aerosol Optical Depth from AERONET . J. Geophys. Res. , 106 : 12067 – 12097 .
- Hsu , N. C. , Si-Chee , T. , King , M. D. and Herman , J. R. 2004 . Aerosol Properties Over Bright-reflecting Source Regions . Geosci. Remote. Sens., IEEE Trans. , 42 : 557 – 569 .
- Hu , R. M. , Martin , R. V. and Fairlie , T. D. 2007 . Global Retrieval of Columnar Aerosol Single Scattering Albedo from Space-based Observations . J. Geophys. Res. , 112 : D02204
- Kaufman , Y. J. 1993 . Aerosol Optical Thickness and Atmospheric Path Radiance . J. Geophys. Res. , 98 : 2677 – 2692 .
- Kaufman , Y. J. , Setzer , A. , Ward , D. , Tanré , D. , Holben , B. N. Menzel , P. 1992 . Biomass Burning Airborne and Spaceborne Experiment in the Amazonas (BASE-A) . J. Geophys. Res. , 97 : 14,581 – 14,599 .
- Kaufman , Y. J. , Tanré , D. , Remer , L. A. , Vermote , E. F. , Chu , A. and Holben , B. N. 1997a . Operational Remote Sensing of Tropospheric Aerosol Over Land from EOS Moderate Resolution Imaging Spectroradiometer . J. Geophys. Res.-Atmos. , 102 : 17,051 – 17,067 .
- Kaufman , Y. J. , Wald , A. E. , Remer , L. A. , Bo-Cai , G. , Rong-Rong , L. and Flynn , L. 1997b . The MODIS 2.1-μm Channel-correlation with Visible Reflectance for Use in Remote Sensing of Aerosol . Geosci. Remote. Sens., IEEE Trans. , 35 : 1286 – 1298 .
- King , M. D. , Kaufman , Y. J. , Tanré , D. and Nakajima , T. 1999 . Remote Sensing of Tropospheric Aerosols from Space : Past, Present, and Future . Am. Meteorol. Soc. , 80 : 2229 – 2259 .
- Koelemeijer , R. B. A. , de Haan , J. F. and Stammes , P. 2003 . A Database of Spectral Surface Reflectivity in the Range 335 nm, 772 nm Derived from 5.5 Years of GOME Observations . J. Geophys. Res. , 108 : 4070
- Levelt , P. F. , van den Oord , G. H. J. , Dobber , M. R. , Malkki , A. , Huib , V. Johan de , V. 2006 . The Ozone Monitoring Instrument . Geosci. Remote. Sens., IEEE Trans. , 44 : 1093 – 1101 .
- Levy , R. C. , Remer , L. A. , Mattoo , S. , Vermote , E. F. and Kaufman , Y. J. 2007 . Second-generation Operational Algorithm: Retrieval of Aerosol Properties Over Land from Inversion of Moderate Resolution Imaging Spectroradiometer Spectral Reflectance . J. Geophys. Res. , 112 : D13211
- Li , C. C. , Lau , A. K. H. , Mao , J. T. and Chu , D. A. 2005 . Retrieval, Validation, and Application of the 1-km Aerosol Optical Depth from MODIS Measurements Over Hong Kong . IEEE Trans. Geosci. Remote. Sens. , 43 : 2650 – 2658 .
- Li , C. C. , Mao , J. T. , Lau , K. H. A. , Chen , J. C. , Yuan , Z. B. Liu , X. Y. 2003 . Characteristics of Distribution and Seasonal Variation of Aerosol Optical Depth in Eastern China with MODIS Products . Chinese Sci. Bull. , 48 : 2488 – 2495 .
- Liu , S. C. , McKeen , S. A. and Madronich , S. 1991 . Effect of Anthropogenic Aerosols on Biologically Active Ultraviolet Radiation . Geophys. Res. Lett. , 18 : 2265 – 2268 .
- Livingston , J. M. , Redemann , J. , Russell , P. B. , Torres , O. , Veihelmann , B. Veefkind , P. 2009 . Comparison of Aerosol Optical Depths from the Ozone Monitoring Instrument (OMI) on Aura with Results from Airborne Sunphotometry, Other Space and Ground Measurements During MILAGRO/INTEX-B . Atmos. Chem. Phys. , 9 : 6743 – 6765 .
- Madronich , S. , McKenzie , R. L. , Bjorn , L. O. and Caldwell , M. M. 1998 . Changes in Biologically Active Ultraviolet Radiation Reaching the Earth's Surface . J. Photoch. Photobio. B: Biol. , 46 : 5 – 19 .
- Nakajima , T. and Higurashi , A. 1998 . A Use of Two Channel Radiances for an Aerosol Characterization from Space . Geophys. Res. Lett. , 25 : 3815 – 3818 .
- Pöschl , U. 2005 . Atmospheric Aerosols: Composition, Transformation, Climate and Health Effects . Angew. Chem. Int. Edit. , 44 : 7520 – 7540 .
- Reid , J. S. , Eck , T. F. , Christopher , S. A. , Hobbs , P. V. and Holben , B. 1999 . Use of the Angstrom Exponent to Estimate the Variability of Optical and Physical Properties of Aging Smoke Particles in Brazil . J. Geophys. Res. , 104 : 27,473 – 27,489 .
- Remer , L. A. , Kaufman , Y. J. , Tanré , D. , Mattoo , S. , Chu , D. A. Martins , J. V. 2005 . The MODIS Aerosol Algorithm, Products, and Validation . J. Atmos. Sci. , 62 : 947 – 973 .
- Satheesh , S. K. , Torres , O. , Remer , L. A. , Babu , S. S. , Vinoj , V. Eck , T. F. 2009 . Improved Assessment of Aerosol Absorption Using OMI-MODIS Joint Retrieval . J. Geophys. Res. , 114 : D05209
- Schuster , G. L. , Dubovik , O. and Holben , B. N. 2006 . Angstrom Exponent and Bimodal Aerosol Size Distributions . J. Geophys. Res. , 111 : D07207
- Stanhill , G. and Ianetz , A. 1997 . Long-term Trends in, and the Spatial Variation of, Global Irradiance in Israel . Tellus B , 49 : 112 – 122 .
- Tanré , D. , Kaufman , Y. J. , Herman , M. and Mattoo , S. 1997 . Remote Sensing of Aerosol Properties Over Oceans Using the MODIS/EOS Spectral Radiances . J. Geophys. Res. , 102 : 16,971 – 16,988 .
- Torres , O. and Bhartia , P. K. 1999 . Impact of Tropospheric Aerosol Absorption on Ozone Retrieval from Backscattered Ultraviolet Measurements . J. Geophys. Res. , 104 : 21,569 – 21,577 .
- Torres , O. , Bhartia , P. K. , Herman , J. R. , Ahmad , Z. and Gleason , J. 1998 . Derivation of Aerosol Properties from Satellite Measurements of Backscattered Ultraviolet Radiation: Theoretical Basis . J. Geophys. Res. , 103 : 17,099 – 17,110 .
- Torres , O. , Bhartia , P. K. , Herman , J. R. , Sinyuk , A. , Ginoux , P. and Holben , B. 2002 . A Long-Term Record of Aerosol Optical Depth from TOMS Observations and Comparison to AERONET Measurements . J. Atmos. Sci. , 59 : 398 – 413 .
- Torres , O. , Chen , Z. , Jethva , H. , Ahn , C. , Freitas , S. R. and Bhartia , P. K. 2010 . OMI and MODIS Observations of the Anomalous 2008–2009 Southern Hemisphere Biomass Burning Seasons . Atmos. Chem. Phys. , 10 : 3505 – 3513 .
- Torres , O. , Decae , R. , Veefkind , P. and de Leeuw , G. 2001 . Omi Aerosol Retrieval Algorithm, in OMI Algorithms Theoretical Basis Document, Vol. III, Clouds, Aerosols and Surface UV Irradiance, P. Stammes, Ed., 2002 .
- Torres , O. , Tanskanen , A. , Veihelmann , B. , Ahn , C. , Braak , R. Bhartia , P. K. 2007 . Aerosols and Surface UV Products from Ozone Monitoring Instrument Observations: An Overview . J. Geophys. Res. , 112 : D24S47
- Zepp , R. G. , Erickson Iii , D. J. , Paul , N. D. and Sulzberger , B. 2007 . Interactive Effects of Solar UV Radiation and Climate Change on Biogeochemical Cycling . Photochem. Photobiol. Sci. , 6 : 286 – 300 .