Abstract
A new technique for measuring the primary organic aerosol (POA) emissions from internal combustion engines is presented. The method combines thermal-optical OC/EC analysis and thermal desorption gas chromatography mass spectrometry (TD-GC-MS) of quartz filter samples collected using a dilution sampler to quantify the total emissions of low-volatility organics and to distribute them across the volatility basis set. These data can be used in conjunction with partitioning theory to predict the gas-particle partitioning and thus the total amount of POA over the entire range of atmospheric conditions. The approach is evaluated using POA emissions data from two gas-turbine engines and one diesel generator. To evaluate the new method, we directly measured the effects of temperature and concentration on gas-particle partitioning of the emissions from each. Predictions based on the volatility distributions derived from the filter analyses are consistent with the direct partitioning measurements. The new approach represents a major improvement over the traditional assumption of nonvolatile POA emissions, which over predicts actual POA emissions from these sources by a factor of 2–4 at typical ambient concentration and temperature. By using quartz filter samples, this new technique is designed to be applied to routine source test data. Volatility distributions derived using this new approach can also be applied directly to the large catalog of quartz filter data used by existing emission inventories and models. The emissions data derived from this approach are designed for use in the next generation of chemical transport models and emissions inventories that employ the volatility basis set approach to explicitly track the gas-particle partitioning of POA emissions.
Copyright 2012 American Association for Aerosol Research
1. INTRODUCTION
Internal combustion engines emit a complex mixture of organic compounds that span a wide range of volatility (Hildemann et al. Citation1991; Schauer et al. Citation1999; Presto et al. Citation2011). The portion of these emissions that partitions into the condensed phase is known as primary organic aerosol (POA). A significant fraction of the POA emissions from many sources is semivolatile at atmospheric conditions (Robinson et al. Citation2010). Therefore, the gas-particle partitioning of POA evolves as emissions move away from the hot, concentrated conditions at the engine exit to cooler, more dilute downwind conditions (Robinson et al. Citation2007, Citation2010). Gas-particle partitioning of semivolatile organics, and thus the amount of POA, also varies with changing atmospheric temperature and particle concentrations. Dynamic gas-particle partitioning complicates interpretation of emissions data measured using dilution samplers, which are generally not operated at atmospheric conditions (Robinson et al. Citation2010). Therefore, accurate characterization of POA emissions requires measurements that characterize its semivolatile nature and that can be used to predict the changes in gas-particle partitioning over the entire range of atmospherically relevant conditions.
The gas-particle partitioning of POA in the atmosphere is controlled by absorptive partitioning theory (Pankow Citation1994; Donahue et al. Citation2006). The POA emission factor (EFPOA) can be written as
Partitioning theory indicates that to predict the contribution of emissions to atmospheric OA one needs to know both EFTOT and the set of fi . The range of C* bins varies from study to study, though in many cases C* = 10−2 μg m−3 is the lowest volatility bin (Donahue et al. Citation2006; Grieshop et al. Citation2009; Riipinen et al. Citation2010; Ranjan et al. Citation2012). While some of the emissions have even lower volatility (C* < 10−2 μg m−3), this material is condensed at essentially all atmospheric conditions and therefore can be lumped into the C* = 10−2 μg m−3 bin. The upper limit of C* depends on the application. An upper limit of C* = 102 μg m−3 should be adequate to describe POA partitioning in the atmosphere (but not necessarily in dilution samplers or in high concentration plumes). This manuscript focuses on characterizing that part of the distribution. Quantifying the emissions in bins up to C* = 106 μg m−3 is required to describe the production of secondary organic aerosol from vapors co-emitted with POA (Donahue et al. Citation2006) or gas-particle partitioning at very high concentrations (hundreds of μg m−3).
The standard approach for determining the volatility distribution of POA is to directly measure changes in its gas-particle partitioning by systematically varying either the temperature or concentration of the exhaust. This has been done with dilution samplers (Lipsky and Robinson Citation2006; Grieshop et al. Citation2009), thermodenuders (Huffman et al. Citation2008, 2009; Grieshop et al. Citation2009), and smog chambers (Grieshop et al. Citation2007; Ranjan et al. Citation2012). The data are then fit using absorptive partitioning theory to determine the volatility distribution (fi ) and total emissions (EFTOT). However, there are several drawbacks with this approach. It requires a large number of gas-particle partitioning measurements made over a wide range of conditions (temperature and COA), which is time consuming and therefore difficult to employ for routine source testing. Uncertain evaporation kinetics and sampling artifacts can complicate interpretation of dilution measurements, especially at atmospherically relevant COA (Lipsky and Robinson Citation2006; Grieshop et al. Citation2009). Wall losses can limit the range of COA available in smog chamber dilution experiments (Grieshop et al. Citation2007, Citation2009). Interpretation of thermodenuder data is complicated by uncertainty in the enthalpy of vaporization (ΔHV ) and uncertain evaporation kinetics of the aerosol (An et al. Citation2007; Stanier et al. Citation2007; Cappa and Jimenez Citation2010; Saleh et al. Citation2011). Finally, fits of the gas-particle partitioning data are not unique (Cappa and Jimenez Citation2010); a large number of combinations of fi , EFTOT, and ΔHV can satisfy the same data.
A need exists for a method to determine volatility distributions from combustion sources that is both fast and offers unique volatility distributions. Of particular interest are techniques that can be applied to filter samples that are routinely collected during source characterization experiments. Gas chromatography is widely applied to analyze filter samples to characterize organic aerosol chemical composition. Gas chromatography can also be used to determine vapor pressures of individual nonpolar and semipolar compounds (Jensen and Schall Citation1966; Hinckley et al. Citation1990). Recent articles have used gas chromatography to classify organic aerosol volatility (Isaacman et al. Citation2011) and to investigate gas-particle partitioning of individual semivolatile species (Williams et al. Citation2010). However, we are not aware of any research that has investigated if gas chromatography data can be used to quantitatively predict the gas-particle partitioning of the entire POA.
In this article, we demonstrate that thermal desorption gas chromatography mass spectrometry (TD-GC-MS) analysis of quartz filter samples can be used to quantitatively predict gas-particle partitioning of POA emissions from internal combustion engines. First, we present direct measurements of gas-particle partitioning of POA emissions for three combustion sources: a diesel engine and two gas-turbine engines. Second, we derive volatility distributions (fi ) from TD-GC-MS analysis and EFTOT from thermal-optical OC/EC analyses of quartz filter samples collected using a dilution sampler. Finally, we compare predictions based on these data and partitioning theory (EquationEquation (1)) to the direct measurements of gas-particle partitioning.
2. MATERIALS AND METHODS
Experiments were performed to characterize the gas-particle partitioning of POA emissions from a CFM56–2B1 gas turbine engine mounted on a KC-135 Stratotanker airframe (Presto et al. Citation2011), a T63 gas-turbine engine mounted in a test cell (Drozd et al. Citation2012), and a small diesel generator (Yanmar L-A series air-cooled 1-cylinder, 4-cycle 6.6 hp) (Lipsky et al. 2006; Grieshop et al. Citation2009; Ranjan et al. Citation2012). Source specific details, such as engine load and fuel composition, are described in the online supplemental material.
The gas-particle partitioning of the POA emissions from each source was investigated at different levels of dilution under isothermal conditions inside a smog chamber using the approach of Ranjan et al. (Citation2012). A thermodenuder was used to quantify the effects of temperature on gas-particle partitioning. The partitioning data are compared to predictions based on volatility distributions derived from gas chromatography of quartz filter samples.
The basic experimental set up has been described previously (Presto et al. Citation2011; Ranjan et al. Citation2012). Briefly, high-temperature, undiluted exhaust from a source was sampled into a 12.7 mm (0.5 inch) O.D. heated transfer line constructed of stainless steel (). The length of the transfer line varied from 2- (diesel engine) to 25-m (CFM56) depending on the source. The transfer line was held at 150°C to reduce thermophoretic and condensational losses. At the end of the transfer line, the exhaust flow (∼20 slpm) was split into two. One portion (∼8–10 slpm) was rapidly mixed with dried, HEPA- and activated-carbon-filtered air using a dilution tunnel operating at a dilution ratio of approximately 10:1 (Lipsky and Robinson Citation2006), which essentially reduced the emissions to ambient temperature. Samples of diluted exhaust were collected from the dilution sampler using two sample trains: (1) a quartz filter (47 mm, Tissuquartz 2500 QAOUP) followed by a Tenax TA sorbent tube; and (2) a Teflon filter followed by a quartz filter to estimate sampling artifacts. A CO2 monitor (Model LI-820, Li-Cor Biosciences) was used to measure the CO2 concentration in the dilution sampler.
FIG. 1 Schematic of experimental setup. Hot, undiluted emissions are sampled into a heated transfer line (150°C) and split into two flows. One portion of the flow is sent to a dilution tunnel for collecting filter and sorbent samples. The other portion of the exhaust is sent through an ejector dilutor and into a smog chamber to measure equilibrium POA partitioning.
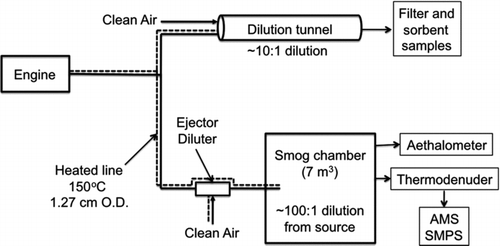
The remainder of the exhaust in the transfer line (10–12 slpm) was diluted using two ejector diluters (Dekati, Model DI-1000) operating on conditioned air heated to 150°C and then transferred into a 7-m3 Teflon smog chamber that was initially filled with dried, HEPA and activated carbon filtered air. The exhaust was added either as a series of small aliquots or as a constant stream at low flow (∼5–10 slpm) (Ranjan et al. Citation2012). When it entered the chamber, the exhaust rapidly mixed with the air inside the chamber, which cooled the exhaust to ambient temperature. Each aliquot of exhaust increased the aerosol concentrations inside the chamber, shifting gas-particle partitioning of semivolatile organics to the particle phase (Ranjan et al. Citation2012).
A suite of instrumentation was used to characterize the gas- and particulate-phase pollutants inside the smog chamber. Data from a scanning mobility particle sizer (SMPS, TSI, Inc., St. Paul, MN, USA) and an Aerodyne quadrupole aerosol mass spectrometer (AMS) were used to measure particle concentrations. A seven-channel aethalometer (Model AE-31, Magee Scientific, Berkeley, CA, USA) was used to measure black carbon (BC). Aerosol volatility was measured using a custom-built thermodenuder system based on the design of An et al. (Citation2007) (Grieshop et al. Citation2009). The thermodenuder consisted of a stainless steel heating section (2.7 cm ID × 65 cm L) followed by an activated carbon filled cooling/stripping section (An et al. Citation2007). The centerline residence time of the heated section of the thermodenuder was 18.6 s at ambient temperature. The aerosol from the chamber was alternately sampled through the thermodenuder or an ambient temperature bypass line every 15 min using the AMS and SMPS.
2.1. Data Analysis Methods
2.1.1. Filters and Sorbents
The quartz filters were analyzed using a Sunset Laboratories OC/EC analyzer using the IMPROVE-A protocol (Chow et al. Citation2007) and with a Thermal Desorption System (TDS, Gerstel Inc., Baltimore, MD, USA) and Cooled Injection System (CIS) coupled to a gas chromatograph equipped with a mass-selective detector (Agilent 6890 GC and 5975 MSD). Before TD-GC-MS analysis, each filter sample was spiked with a deuterated internal standard to track analyte recovery. The internal standard contained five-deuterated n-alkanes ranging from n-hexadecane-d34 (C16D34) to n-hexatriacontane-d74 (C36D74).
The GC was equipped with an Agilent HP-5ms capillary column, which primarily separates nonpolar analytes by boiling point. Therefore, elution time provides a measure of the volatility (Jensen and Schall Citation1966; Hinckley et al. Citation1990). The TD-GC-MS system was operated to quantify compounds spanning eight orders of magnitude in volatility (C* from 106–10−2 μg m−3). Details on the TD-GC-MS analysis procedure are in the online supplemental material.
2.1.2. Calculation of Emission Factors
The particle data measured in both the dilution sampler and smog chamber are reported as fuel-based emission factors (particle mass per unit mass of fuel burnt) calculated using a fuel carbon mass balance
2.1.3. Smog Chamber Wall Losses
To calculate emission factors using measured smog chamber concentrations, the data must be corrected for losses to the chamber walls. We use the approach described in Ranjan et al. (Citation2012) (Equation (3)).
The other case (ω = 1 in Equation (3c)) assumes that wall-bound particles remain in phase equilibrium with the suspended particles and organic vapors. Thus, all particles, whether suspended or wall-bound, have the same composition (e.g., all particles have the same OA:BC ratio). Because the exhaust is either added continuously or as a series of successive aliquots and the suspended organic aerosol concentrations are typically small (<20 μg m−3), the suspended mass is generally larger than the mass on the walls, which minimizes the difference between these two wall loss assumptions. We present the second case (ω = 1) here, which is currently the most common method for wall loss corrections used in smog chamber tests of secondary organic aerosol formation.
Neither of the two wall loss corrections described above accounts for the direct loss of semivolatile vapors to the chamber walls (Loza et al. Citation2010; Matsunaga and Ziemann Citation2010). Matsunaga and Ziemann (Citation2010) reported that semivolatile organic compounds partition to the chamber walls reversibly according to Henry's Law, and that the loss of organic vapors to the chamber walls occurred even in the absence of suspended or wall-bound organic particles. The loss of semivolatile vapors to the chamber walls would alter the gas-particle partitioning of the suspended organic aerosol, leading to lower observed organic aerosol concentrations than under “wall free” conditions. However, the direct loss of vapors to the chamber walls appears to be composition dependent (Loza et al. Citation2010; Matsunaga and Ziemann Citation2010), with lower losses for reduced compounds. For example, Matsunaga and Ziemann (Citation2010) reported that approximately 20% of the mass of C16 n-alkane vapors was lost to the wall versus approximately 60% mass loss for a C13 alcohol (the greatest reported loss). The semivolatile emissions from internal combustion engines are dominated by reduced compounds (Schauer et al. Citation1999); therefore, n-alkanes are presumably a reasonable approximation for the composition of the emissions. Using the method outlined by Matsunaga and Ziemann (Citation2010), their values for n-alkanes, and concentrations typical of the experiments presented here, we calculate that 20–30% of the particle mass in the C* = 100 μg m−3 bin will be lost to the wall after 100 min. Less than 10% of the particle mass will be lost for lower C* bins. The total mass loss (gas + vapor) to the walls reaches 20–40% for 10−1 < C* < 100 μg m−3, though much of this mass is lost from the vapor phase and not the particle phase. Therefore, direct losses of vapors are not expected to significantly influence POA concentrations in these experiments.
3. RESULTS AND DISCUSSION
3.1 Direct Measurements of Gas-Particle Partitioning of POA Emissions
shows in-chamber equilibrium partitioning data measured by adding small aliquots of diluted exhaust from the diesel generator to the smog chamber. After each injection, the wall-loss corrected organic aerosol concentration (C OA) initially rose and then fell to a steady state value due to mixing inside the chamber. The plateaus in C OA following each aliquot of exhaust indicate the equilibrium partitioning of the organic aerosol.
FIG. 2 In-chamber equilibrium partitioning data from a diesel generator experiment. Panel (a) shows the time series of measured CO2 concentration, suspended organic aerosol concentration, and wall-loss corrected COA. Panel (b) is a partitioning plot of the data from panel (a). It shows POA emission factor (EFPOA) as a function of COA. The thick black line shows the predicted EFPOA from the OC/EC and TD-GC-MS analysis of a quartz filter samples (Table S3). The dashed black line shows the predicted EFPOA when IVOC emissions captured by a Tenax sorbent bed placed downstream of the quartz filter are included in the partitioning calculation.
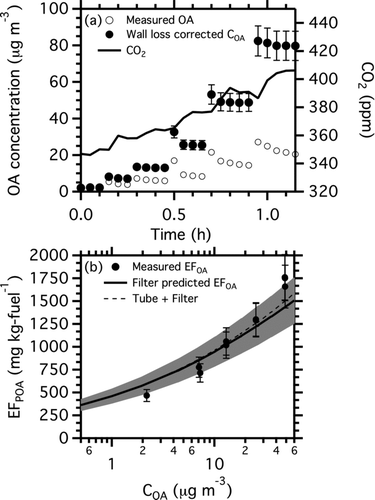
presents the data from using a partitioning plot, which is a scatter plot of POA emission factor versus C OA. As expected from partitioning theory (EquationEquation (1)), EFPOA monotonically increases with increasing C OA. In addition, the magnitude of the increase is large (a factor of 4 with a factor 30 increase in exhaust concentration), clearly demonstrating that a large fraction of the POA emissions from this diesel generator are semivolatile, consistent with earlier work (Lipsky and Robinson Citation2006; Shrivastava et al. Citation2006; Grieshop et al. Citation2009; Ranjan et al. Citation2012). The BC emission factor (not shown), which is nonvolatile and thus not influenced by partitioning, is constant as a function of C OA (Ranjan et al. Citation2012). For this test, C OA was determined from both AMS and SMPS data. The SMPS estimates use the density of the lubricating oil used in the diesel generator (0.8 g ml−1) and assume spherical particles. The two instruments yielded C OA values within the measurement uncertainties; the SMPS data are shown as points in .
presents partitioning plots of smog chamber data for the T63 and CFM56 engines. The C OA and POA emission factor for in-chamber partitioning measurements were estimated from SMPS data assuming unit density and correcting for the contributions from BC (Presto et al. Citation2011). The BC correction works well when the OA:BC ratio is greater than approximately 10:1. This is the case for the diesel generator or the T63 operating on FT fuel at idle load (dilution tunnel OA:BC = 30.5). However, for lower OA:BC ratios, such as the T63 engine using JP8 fuel (, dilution tunnel OA:BC = 5.1), the BC correction is less accurate. The AMS could not be used to measure the in-chamber C OA in the gas-turbine experiments because a large fraction of the emissions were smaller than 60 nm and therefore fall outside of the AMS transmission window (Jayne et al. Citation2000).
FIG. 3 Partitioning plots for (a) T63 engine, Fischer Tropsch (FT) fuel at idle load; (b) T63 engine, blended fuel at idle load; (c) T63 engine, JP8 fuel, Idle load; and (d) CFM56 engine, JP8 fuel, 4% load. The large point in the upper right of each panel is the artifact-corrected (quartz minus quartz behind Teflon filter) POA emission factor measured with filter samples from a dilution tunnel. The horizontal black dashed line shows the nonvolatile POA assumption. The small points indicate in-chamber partitioning data. Filled points in all panels are from SMPS data corrected for interference from BC. Crosses in panel (c) are AMS data. The multiple shapes (diamonds and triangles) in panel (d) indicate separate experiments. The thick black line is the predicted EFPOA from TD-GC-MS analysis of quartz filter samples. The shaded region shows the range of EFPOA predictions for the mean volatility distributions and the range of EFTOT in Table S3. The thin black lines show the predicted EFPOA when the 20% undesorbed mass is placed into a nonvolatile bin, as described in the text. The OA:BC ratios measured in the dilution tunnel for each of the panels were (a) 30.5, (b) 7.8, (c) 5.1, and (d) 8.2. OA:BC ratios were about a factor of two lower in the chamber than in the dilution tunnel because of differences in OA partitioning.
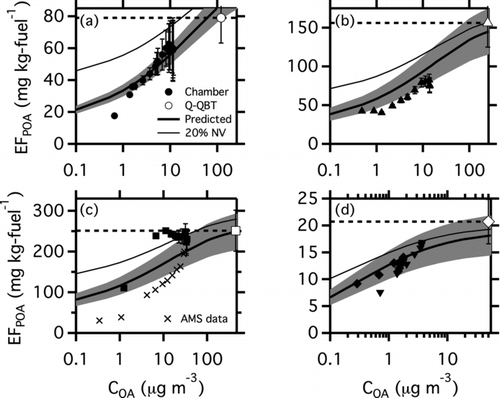
The POA emission factor for both gas-turbine engines monotonically increases with increasing C OA. Therefore, similar to the diesel engine, a large fraction of the POA emissions from the two gas-turbine engines is also semivolatile. We believe that uncertainty with the BC correction to the SMPS data contributes to the POA emission factor measured during the JP8 idle test in having a flatter shape as a function of COA, as opposed to the monotonically increasing EFPOA shown in the other panels of . This may also be the result of adsorptive, rather than absorptive, partitioning dominating at lower OA:BC ratios (Goss and Schwarzenbach Citation1998; Dachs and Eisenreich Citation2000; Lohmann and Lammel Citation2004; Roth et al. Citation2005). For that test, the AMS measurements are also included as crosses to serve as a lower bound for the in-chamber measurements because a large fraction of the POA mass was in particles smaller than 60 nm and therefore are not detected by the AMS.
To illustrate the dramatic effects of changes in partitioning, also plots a traditional POA emission factor based on quartz filter data collected from the dilution sampler. The filter data have been corrected for sampling artifacts (bare quartz filter minus a quartz behind Teflon filter [Subramanian et al. Citation2004]). The horizontal black dashed line indicates the expected behavior if the artifact corrected POA collected on the filter from the dilution sampler was nonvolatile, which is the standard assumption of most models and emissions inventories. The nonvolatile assumption clearly does not match the measured changes in partitioning.
For the gas-turbine engines, the POA emissions measured in-chamber are significantly smaller (by up to a factor of 4) than the artifact corrected POA emission factors measured in the dilution tunnel (). This difference is due to partitioning—the C OA levels in the chamber span the atmospherically relevant range (roughly 1–20 μg m−3) versus the much higher concentrations in inside the dilution sampler (C OA = 50–400 μg m−3). Therefore, the data indicate that up to 80% of the artifact corrected POA emissions measured using a quartz filter sampler collected from a dilution sampler exist as vapor in the atmosphere. The fraction is even higher if one considers the bare (nonartifact corrected) quartz filter.
shows the effect of temperature on gas-particle partitioning for the gas-turbine experiments presented in . The data are plotted as thermograms—POA mass fraction remaining (MFR) as a function of temperature in the thermodenuder. In every experiment, 60% or more of the POA evaporates upon mild heating. This further demonstrates the semivolatile nature of the POA emissions from these sources.
FIG. 4 Thermograms, plots of POA mass fraction remaining (MFR) as a function of thermodenuder temperature, for the gas-turbine experiments shown in . The symbols are measured MFR from the smog chamber. Black lines are predicted MFR using the volatility distributions listed in Table S3. The grey lines in panels (a–c) show the modeled MFR for particles composed entirely of the lubricating oil used in the T63 engine. The dashed black line in panel (c) shows the effect of assuming that the undesorbed material is nonvolatile, as discussed in the text.
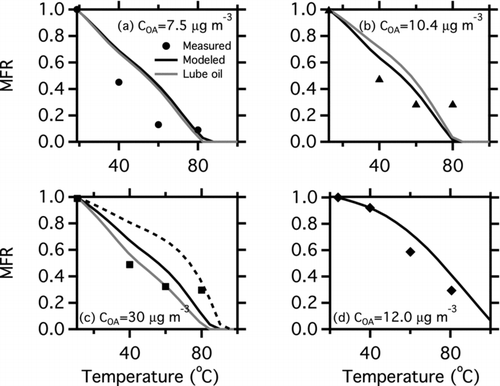
The full set of emissions measurements (tunnel + chamber + thermodenuder) show that EFPOA increases with C OA or decreases with temperature. This is indicative of absorptive organic partitioning of the emissions, and further evidence that defining a single, nonvolatile OA emission factor from dilution tunnel data can overestimate the POA emissions observed at ambient C OA (Robinson et al. Citation2007, 2010).
3.2 Determination of Volatility Distributions from TD-GC-MS Analysis of Quartz Filter Samples
Gas chromatography has been used to determine vapor pressures of individual nonpolar and semipolar compounds (Jensen and Schall Citation1966; Hinckley et al. Citation1990). In this section, we apply it to classify the volatility of the organic emissions collected on a quartz filter.
shows a selected ion mode TD-GC-MS chromatograph for m/z 57 from a quartz filter sample from the T63 engine operating at idle load on JP8 fuel. M/z 57 (C4H9 +) is a fragment produced from electron impact ionization of reduced hydrocarbons; it is the most abundant signal in the mass spectrum from the quartz filter samples. The chromatograms of other mass fragments associated with hydrocarbons (m/z 43, 55, 83, etc.) are essentially the same as m/z 57.
FIG. 5 (a) C* as a function of GC residence time for a number normal, branched, and cyclic alkanes, naphthalane and substituted naphthalenes, hopanes, oxygenated aromatics, and acids. Vertical dashed lines denote the boundaries of C* bins. Numbers at the top of the bins indicate log(C*) for each bin. More volatile components (higher C*) have shorter retention times. (b) M/z 57 chromatograph of a filter sample collected from the T63 engine operating on JP8 fuel at idle load. (c) Volatility distribution, presented as fi (left axis) and emission factor (right axis) derived from data shown in panel (b). EFTOT, the total emission rate of low-volatility organic material, is the sum of the bars. Error bars show the standard deviation obtained from the analysis of multiple samples with the same engine and load conditions.
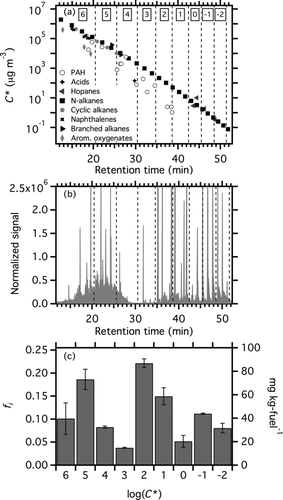
Approximately 90% of the signal in , and therefore, a similar portion of the organic mass collected on the quartz filter, elutes as a pair of broad humps often referred to as the unresolved complex mixture (UCM). The first hump corresponds to approximately C12 to C20 hydrocarbons and is presumably mostly adsorbed vapors (artifact). The second hump corresponds to approximately C23 to C35 hydrocarbons. UCM is thought to be composed of a mixture of branched and cyclic hydrocarbons that cannot be separated by traditional one-dimensional gas chromatography (Hildemann et al. Citation1991; Schauer et al. Citation1999).
To interpret the m/z 57 chromatograms, we developed a calibration for C* as a function of GC elution time using a suite of standard compounds, including normal, branched, and cyclic alkanes, naphthalene and substituted naphthalanes, 3- and 4-ring polycyclic aromatic hydrocarbons, aromatic oxygenates, hopanes, and acids. These data are shown in .
A single C* calibration curve can be used to bin the GC-MS data for aliphatic compounds (normal, branched, and cyclic alkanes and hopanes) and naphthalanes into the basis set. PAHs, aromatic oxygenates, and acids do not fall along the same C* calibration curve as the aliphatic compounds and naphthalanes. This may be driven by differences in polarity (Isaacman et al. Citation2011) that shift elution times for the PAHs and aromatic oxygenates. However, these compounds make up a small fraction of the speciated organic emissions (<5%) (Schauer et al. Citation1999; Citation2002). Therefore, we assume that the UCM is mainly comprised of hydrocarbons and not more polar species, such as PAHs.
To derive the volatility distribution for the emissions collected on a quartz filter, the m/z 57 chromatograph is binned based on elution time using the C* calibration shown in . Essentially, we are assuming that the C* versus elution time relationship for the UCM is the same as our calibration based on the analysis of individual aliphatic compounds. The boundaries of the bins are shown as vertical grey bars in . The mass fraction of the organics in each bin (fi ) is defined based on the relative fraction of m/z 57 signal in each bin, after correction for recovery of the deuterated standard. Following the approach of Shrivastava et al. (Citation2006), the total emission rate (EFTOT) is estimated as 1.2 times the OC determined from OC/EC analysis of the quartz filter. The set of fi and EFTOT values can then be used in conjunction with EquationEquation (1) to predict EFPOA over a wide range of atmospheric conditions.
Before comparing predictions inferred from the quartz filter data, we discuss a number of uncertainties that underlie the approach. It assumes that the m/z 57 chromatograph is representative of the volatility distribution of the entire POA. This is a critical assumption. We evaluate it by comparing the predictions based on the volatility distributions derived from TD-GC-MS analysis to direct measurements of gas-particle partitioning. If the emissions contained a significant fraction of highly polar species and/or species that do not generate m/z 57 upon ionization in the mass spectrometer, the volatility distributions determined from m/z 57 alone may not accurately reproduce the actual gas-particle partitioning, and other ions would need to be included in the analysis. If, for example, the emissions contained a substantial fraction of PAH or organic acids, a separate C* versus retention time calibration curve would be required for that portion of the emissions (). This may be the case for wood smoke or ambient samples. For example, Hays et al. (Citation2004) used m/z 57, 91, 134, 142, 184, 200, and other ions to apportion UCM for residential wood burning samples, and observed significant differences in the chromatographs for hydrocarbon (m/z 57) versus PAH (e.g., m/z 200) portions of the UCM. However, for the internal combustion engines tested here, m/z 57 is the most abundant ion in the mass spectrum, suggesting that the emissions are dominated by hydrocarbons. Furthermore, other fragments associated with the UCM signal and m/z indicative of other moieties such as m/z 55 for cyclic hydrocarbons elute at the same time as m/z 57, and therefore, do not add additional information beyond what is provided by the m/z 57 chromatograph. In addition, the total integrated m/z 57 signal is linearly correlated (R2 = 0.74) with OC mass concentration as determined by OC/EC analysis (Figure S2), suggesting that the m/z 57 signal is a reasonable proxy for the total organic mass on the filter for internal combustion engine exhaust samples.
One uncertainty is the efficiency of the thermal desorption of organic material from the filter. Incomplete desorption may occur because the maximum temperature in TD-GC-MS analysis (320°C) may be too low to extract all of the organics from the filter. Pyrolysis could also affect thermal desorption, but it is less of a concern for largely nonpolar emissions samples with limited inorganic constituents (Yu et al. Citation2002). The thermal desorption efficiency was investigated by reanalyzing the filter punches in the OC/EC analyzer after analysis by TD-GC-MS. This provided a quantitative measure of any residual carbon left on the filter. After correcting for EC, only 10–20% of the OC was not desorbed from the quartz filters in the TD-GC-MS analysis. The potential effects of this undesorbed material on the inferred volatility distribution are discussed below.
Another uncertainty is that a fraction of the organics collected a quartz filter may not elute from the GC column. This is a major problem for samples that contain large amounts of oxygenated compounds, such as in wood smoke (Schauer et al. Citation2001) or ambient aerosols (Rogge et al. Citation1993). Fortunately, POA emissions from petroleum-fuel fired engines are mainly comprised of reduced and semipolar compounds. Hildemann et al. (Citation1993) compared results from GC-FID analysis of derivatized and underivitized extracts to assess the fraction of highly polar and/or unelutable material. For fossil fuel fired sources (diesel engines, gasoline engines, fuel-oil boiler), they report that 80% or more of the mass eluted in the underivitized sample, indicating excellent recovery.
Based on the C* calibration curve, the first UCM hump in is composed of intermediate volatility organic compounds (IVOCs, 106 < C* < 103 μg m−3). Given its high volatility it should exist as vapors in both the ambient atmosphere and in the dilution tunnel. We believe that these vapors have adsorbed to the quartz filter (sampling artifact). This material contributes 36% the m/z 57 signal in , which is comparable to the sampling artifact estimated using a quartz behind Teflon filter (28% of bare quartz filter mass for this experiment).
The C* calibration curve indicates that the second UCM hump (56% of m/z 57 signal) in is comprised mainly of SVOCs (semivolatile organic compounds, 103 < C* < 10−1 μg m−3). These species actively partition between the vapor and condensed phases both in the atmosphere and the dilution tunnel. The changes in gas-particle partitioning of these SVOCs with dilution drives the changes in POA emission factors shown in and . The abundance of SVOCs in the chromatograph of the filter samples is further evidence of the semivolatile nature of the POA emissions. Finally, a small fraction (8% of the m/z 57 signal) of the emissions have C* < 10−2 μg m−3, and exist as POA in both the ambient atmosphere and the dilution tunnel.
presents the volatility distribution derived for the chromatograph shown in . As expected, a large fraction of the emissions collected on a quartz filter are classified as SVOCs. Figure S1 shows volatility distributions for the T63 engine operating at cruise load on JP8 fuel and for the lubricating oil used in the T63. The volatility distribution of the SVOC emissions at idle load are similar to the lubricating oil used in the engine (Figure S1c), suggesting that lubricating oil constitutes a substantial fraction of the POA emissions at idle. Previous measurements indicate that lubricating oil is a major component of gas-turbine engine POA (Yu et al. Citation2010; Presto et al. Citation2011).
3.3 Predicted Versus Measured Partitioning
In this section, we compare direct measurements of POA partitioning (data in –) to predictions of POA partitioning based on the volatility distributions derived from the TD-GC-MS analysis of quartz filter samples. The thick black lines in and 3 show predicted EFPOA based on EFTOT determined from OC/EC analysis and fi determined from integration of the TD-GC-MS m/z 57 chromatograph. Tables of the volatility distributions and EFTOT used for the calculations are contained in the supplemental materials. The shaded areas in and show the range in predicted POA emission factors for a constant volatility distribution (e.g., the mean distributions in Table S3) and a range of EFTOT, determined from samples collected from multiple engine runs at the same fuel and load conditions.
For the diesel generator data shown in , there is reasonable agreement between the predicted and observed EFPOA for C OA ≤ ∼25 μg m−3. Therefore, the approach works well over essentially the entire atmospherically relevant range of C OA. However, the predicted EFPOA is lower than the observations at higher C OA.
We believe that part of the discrepancy between the observed and predicted EFPOA for C OA > 45 μg m−3 may be the result of IVOC species partitioning to the aerosol phase at high C OA, specifically emissions in the C* = 103 μg m−3 bin. Data from the backup Tenax tubes indicates that quartz filters only capture a portion of this material (Figure S3), which primarily exists as vapor in the sampling system. The dashed black line in shows the impact of including IVOC emissions from the Tenax sorbent sample on the predicted EFPOA. Adding the contribution of IVOCs from the sorbent sample accounts for 20% of the difference between the measured EFPOA and the prediction from the filter sample alone at C OA = 45 μg m−3. The under prediction for C OA > 45 μg m−3 may also be due in part to increased uncertainty in the wall loss correction.
compares predicted and measured partitioning for POA emissions from the T63 and CFM56 engines. There is good agreement between the predicted and observed EFPOA for both the T63 engine operating on FT fuel () and the CFM56 engine (). The artifact corrected filter data are also predicted within 10–15%, which is well within the uncertainty of both the prediction and the artifact correction.
For the T63 operating on blended fuel (), the predicted EFPOA is about 20% higher than the in-chamber data, but within 10% for the dilution tunnel data. The slope of the predicted POA emissions and the in-chamber data are similar, suggesting that the volatility distribution may be correct but that EFTOT may be overestimated.
The worst agreement occurs for the T63 operating on JP8 fuel (). As discussed above, the in-chamber C OA for this case is more uncertain because of higher BC concentrations. However, the predicted EFPOA is much closer to the chamber data (AMS and SMPS) than the traditional, nonvolatile POA emission factor estimated from filter data. The predicted EFPOA also lies between the SMPS and AMS estimates. The SMPS estimate for EFPOA is relatively flat. This may be the result of the difficulties with the BC correction discussed above, or may be the result of adsorption and/or slow evaporation of organic material from the BC surface (Goss and Schwarzenbach Citation1998; Dachs and Eisenreich Citation2000; Lohmann and Lammel Citation2004; Roth et al. Citation2005). The AMS data do not exhibit a flat profile of EFPOA because the addition of exhaust to the chamber causes the particles to grow via condensation. This increases the size of the particles, thereby increasing the fraction of the particles larger than 60 nm (and therefore detected by the AMS), and gives the AMS data the appearance that EFPOA monotonically increases. The predicted EFPOA agrees with the dilution tunnel measurements.
The lines in are predicted changes in partitioning with temperature using the thermodenuder model of Riipinen et al. (Citation2010). The inputs to the model were the volatility distributions determined from the TD-GC-MS analysis (Table S3), and the C OA and particle median diameter from the chamber measurements. The enthalpy of vaporization was varied with C* according to ΔHv (C*) = −11 log(C*298 K) + 85 kJ mol−1 (Ranjan et al. Citation2012). The black lines show the model predictions for the volatility distributions determined from the emissions. Grey lines in show the model results for the volatility distribution of the lubricating oil used in the T63 engine. The similarity between the model predictions for the measured emissions and the lubricating oil are further indication that the POA from the T63 contains a large oil component.
The CFM56 data () have the best agreement between modeled and measured MFR. The model accurately predicts MFR at 40°C, but over predicts MFR at 60°C and 80°C by 20–30%. This is consistent with the agreement observed in the in-chamber partitioning data (). Similar agreement was observed for the diesel volatility distribution derived by this method and previously published thermodenuder data for the same engine (Figure S4) (Grieshop et al. Citation2009; Ranjan et al. Citation2012).
The poorest agreement is observed for the T63 FT Idle experiment (). The model over predicts the MFR at 40°C by a factor of 1.5 and at 60°C by a factor of 3. This is puzzling because of the strong agreement between the predicted and measured EFPOA for this case (). While the model over predicts the MFR at 40 and 60°C, it accurately captures the near-total evaporation at 80°C.
The T63 blend Idle and T63 JP8 Idle cases () show similar behavior. The model over predicts MFR at 40 and 60°C by up to 20%. For each case, the measured MFR reaches 0.4 at 60°C, but does not increase at 80°C. This may be the result of adsorption becoming more important as organic material evaporates from the particles. At high OA:BC ratios, the organic partitioning is dominated by absorptive partitioning, and the enthalpy of vaporization (ΔHv ) is the primary barrier to evaporation (Pankow Citation1994; Donahue et al. Citation2006). However, as OA:BC falls, adsorption of organics to the BC core becomes more important. Adsorption carries an additional enthalpy (Pankow Citation1994). Therefore, an aerosol subject to both absorption and adsorption will exhibit higher MFR in the thermodenuder. The model does not account for adsorption. In the atmosphere, POA partitioning is thought to be largely an absorptive process.
Overall the agreement between measured and predicted POA partitioning in is good, but not perfect. However, in all cases, it is much better than the current assumption of nonvolatile POA used in most models and emission inventories. The nonvolatile POA assumption applied to artifact corrected filter measurements systematically over predicts the observed EFPOA. Most inventories do not account for organic artifacts, and instead use EFPOA as the emissions collected on a bare quartz filter. In such cases, the over prediction will be even larger than that shown in and . Note the difference in scale—in this work, we define EFTOT from a bare quartz filter, whereas the inventories define the same quantity as EFPOA. The data presented here indicate that 60–80% of traditionally defined POA exist as vapors in atmosphere (Robinson et al. Citation2007; Robinson et al. Citation2010).
As noted above, 10–20% of the organic mass does not desorb from the filter during the TD step of the TD-GC-MS analysis. The thick black lines in and are based on the measured distribution, and therefore, assume that any undetected mass (due to either incomplete thermal desorption or poor elution) has the same distribution as the measured material. The thin black lines in show predictions assuming that the 20% of the emissions that do not desorb are nonvolatile. With the possible exception of the T63 JP8 idle case (), treating the residual mass as nonvolatile leads to substantial over prediction (by as much as a factor of 2.5) of the in-chamber partitioning data (). Assuming that the undesorbed mass is nonvolatile also does not systematically improve the prediction of the dilution tunnel filter data. shows the impact of this assumption on the TD model predictions as a dashed black line. Assuming that the undesorbed mass is nonvolatile leads to an approximately 50% over prediction of the MFR at 40 and 60°C. The effect is similar for the other cases in . Therefore, it appears that for the sources tested here, the m/z 57 signal of the quartz filter sample provides a reasonable estimate of the POA volatility distribution.
4. CONCLUSIONS
This article demonstrates a new technique to characterize the POA emissions from internal combustion engines. The method combines OC/EC and TD-GC-MS analyses of quartz filter samples to determine both the total emissions of low volatility organics (EFTOT) and their volatility distribution (fi ). These data can be used in conjunction with partitioning theory (EquationEquation (1)) to predict the changes in POA emissions over the entire range of atmospheric conditions. – demonstrate that this approach can reproduce the measured changes in gas-particle partitioning of POA emissions from three sources over a wide range of atmospherically relevant conditions.
The new technique represents a major advancement over the traditional approach used to characterize POA emissions, which is based on OC/EC analysis of quartz filter samples collected using a dilution sampler. Those emissions are commonly treated as nonvolatile in models and inventories—essentially assuming that the gas-particle partitioning of the POA inside the dilution sampler is representative of the full range of atmospheric conditions. The data in this and other articles (Lipsky and Robinson Citation2006; Robinson et al. Citation2007, Citation2010; Grieshop et al. Citation2009) demonstrate that for many sources this is a poor assumption. For example, for the three sources tested here, assuming nonvolatile POA overestimates EFPOA by a factor of 2–4. In addition, the traditional approach does not account for the variability in gas-particle partitioning of POA with changing atmospheric conditions.
The new technique also represents an important step forward from previous research-grade approaches used to characterize the volatility distribution of POA emissions from internal combustion sources (Shrivastava et al. Citation2006; Grieshop et al. Citation2009; Cappa and Jimenez Citation2010; Ranjan et al. Citation2012), which require making many gas-particle partitioning measurements over a wide range of conditions. This is extremely resource intensive, and therefore, not suitable for routine source testing. The new method requires an additional analytical step of standard quartz filter samples. We expect this method to work well for other fossil-fuel sources, such as gasoline and diesel powered vehicles (CitationMay et al. in prep). More evaluation, and perhaps the addition of other ions besides or in addition to m/z 57 (Hays et al. Citation2004), is needed for emissions with more polar species, such as biomass burning emissions or for analyzing ambient samples.
There are many advantages to basing the new method on the analysis of quartz filter samples. It can be easily applied to existing source test data, which routinely collect quartz filters, and to historical filter samples. Furthermore, the volatility distributions derived from this technique can be directly applied to the large number of quartz filter POA emission factors already used to by models and emission inventories. A shortcoming with the quartz filter based approach is that it does not quantitatively collect all of the low volatility organics. This would require combining the filter samples with sorbent measurements, an approach that will be addressed in a future manuscript. The data in this article indicate that this does not appear to be problem from the perspective of predicting gas-particle partitioning of POA at atmospheric conditions, but a quartz filter based approach will not capture a large portion of the low-volatility organic vapors that are important for secondary organic aerosol formation (Donahue et al. Citation2006; Robinson et al. Citation2007). Therefore, comprehensive source characterization will require sorbent samples.
uast_a_700430_sup_26724053.zip
Download Zip (239 KB)Acknowledgments
Funding was provided by the U.S. Department of Defense Strategic Environmental Research and Development Program (SERDP) under project WP-1626 and by the US Environmental Protection Agency National Center for Environmental Research (NCER) through the STAR program (R833748). The views, opinions, and/or findings contained in this article are those of the authors and should not be construed as an official position of the funding agencies.
[Supplementary materials are available for this article. Go to the publisher's online edition of Aerosol Science and Technology to view the free supplementary files.]
REFERENCES
- An , W. J. , Pathak , R. K. , Lee , B.-H. and Pandis , S. N. 2007 . Aerosol Volatility Measurement Using an Improved Thermodenuder: Application to Secondary Organic Aerosol . J. Aerosol Sci. , 38 : 305 – 314 .
- Cappa , C. D. and Jimenez , J. L. 2010 . Quantitative Estimates of the Volatility of Ambient Organic Aerosol . Atmos. Chem. Phys. , 10 : 5409 – 5424 .
- Chow , J. C. , Watson , J. G. , Chen , L. -W. A. , Chang , M. C. O. , Robinson , N. F. Trimble , D. 2007 . The IMPROVE_A Temperature Protocol for Thermal/Optical Carbon Analysis: Maintaining Consistency with a Long-Term Database . J. Air Waste Manage. Assoc. , 57 : 1014 – 1023 .
- Dachs , J. and Eisenreich , S. J. 2000 . Adsorption Onto Aerosol Soot Carbon Dominates Gas-Particle Partitioning of Polycyclic Aromatic Hydrocarbons . Environ. Sci. Technol. , 34 : 3690 – 3697 .
- Donahue , N. M. , Robinson , A. L. , Stanier , C. O. and Pandis , S. N. 2006 . Coupled Partitioning, Dilution, and Chemical Aging of Semivolatile Organics . Environ. Sci. Technol. , 40 : 2635 – 2643 .
- Drozd , G. T. , Miracolo , M. A. , Presto , A. A. , Corporan , E. , Lipsky , E. M. and Robinson , A. L. 2012 . Particulate and Organic Vapor Emissions From an In-Use Helicopter Engine . Energy Fuels , Submitted
- Goss , K. U. and Schwarzenbach , R. P. 1998 . Gas/Solid and Gas/Liquid Partitioning of Organic Compounds: Critical Evaluation of the Intepretation of Equilibrium Constants . Environ. Sci. Technol. , 32 : 2025 – 2032 .
- Grieshop , A. P. , Donahue , N. M. and Robinson , A. L. 2007 . Is the Gas-Particle Partitioning in Alpha-Pinene Secondary Organic Aerosol Reversible? . Geophys. Res. Lett. , 34 : L14810 doi: 10.1029/2007GL029987
- Grieshop , A. P. , Miracolo , M. A. , Donahue , N. M. and Robinson , A. L. 2009 . Using Thermal Denuder and Dilution Data to Characterize Gas-Particle Partitioning of Combustion Aerosols . Environ. Sci. Technol. , 43 : 4750 – 4756 .
- Hays , M. D. , Smith , N. D. and Dong , Y. 2004 . Nature of Unresolved Complex Mixture in Size-Distributed Emissions from Residential Wood Combustion as Measured by Thermal Desorption-Gas Chromatography-Mass Spectrometry . J. Geophy. Res. , 109 : D16S04 doi: 10.1029/2003JD004051
- Hildebrandt , L. , Donahue , N. M. and Panids , S. N. 2009 . High Formation of Secondary Organic Aerosol from the Photo-Oxidation of Toluene . Atmos. Chem. Phys. , 9 : 2973 – 2986 .
- Hildemann , L. M. , Cass , G. R. and Mazurek , M. A. 1993 . Mathematical Modeling of Urban Organic Aerosol: Properties Measured by High-Resolution Gas Chromatography . Environ. Sci. Technol. , 27 : 2045 – 2055 .
- Hildemann , L. M. , Mazurek , M. A. , Cass , G. R. and Simoneit , B. R. T. 1991 . Quantitative Characterization of Urban Sources of Organic Aerosol by High-Resolution Gas Chromatography . Environ. Sci. Technol. , 25 : 1311 – 1325 .
- Hinckley , D. A. , Bidleman , T. F. , Foreman , W. T. and Tuschall , J. R. 1990 . Determination of Vapor Pressures for Nonpolar and Semipolar Organic Compounds from Gas Chromatograhic Retention Data . J. Chem. Eng. Data , 35 : 232 – 237 .
- Huffman , J. A. , Docherty , K. S. , Aiken , A. C. , Cubison , M. J. , Ulbrich , I. M. DeCarlo , P. F. 2009 . Chemically-Resolved Aerosol Volatility Measurements from two Megacity Field Studies . Atmos. Chem. Phys. , 9 : 7161 – 7182 .
- Huffman , J. A. , Ziemann , P. J. , Jayne , J. T. , Worsnop , D. R. and Jimenez , J. L. 2008 . Development and Characterization of a Fast-Stepping/Scanning Thermodenuder for Chemically-Resolved Aerosol Volatility Measurements . Aerosol Sci. Technol. , 42 : 395 – 407 .
- Isaacman , G. , Worton , D. R. , Kreisberg , N. M. , Hennigan , C. J. , Teng , A. P. Hering , S. V. 2011 . Understanding Evolution of Product Composition and Volatility Distribution Through In-Situ Gcxgc Analysis: A Case Study of Longifolene Ozonolysis . Atmos. Chem. Phys. , 11 : 5335 – 5346 .
- Jayne , J. T. , Leard , D. C. , Zhang , X. F. , Davidovits , P. , Smith , K. A. Kolb , C. E. 2000 . Development of an Aerosol Mass Spectrometer for Size and Composition Analysis of Submicron Particles . Aerosol Sci. Technol. , 33 : 49 – 70 .
- Jensen , D. J. and Schall , E. D. 1966 . Determination of Vapor Pressures of Some Phenoxyacetic Herbicides by Gas-Liquid Chromatography . J. Agric. Food Chem. , 14 : 123 – 126 .
- Lipsky , E. M. and Robinson , A. L. 2006 . Effects of Dilution on Fine Particle Mass and Partitioning of Semivolatile Organics in Diesel Exhaust and Wood Smoke . Environ. Sci. Technol. , 40 : 155 – 162 .
- Lohmann , R. and Lammel , G. 2004 . Adsorptive And Absorptive Contributions to the Gas-Particle Partitioning of Polycyclic Aromatic Hydrocarbons: State of Knowledge and Recommended Parameterization for Modeling . Environ. Sci. Technol. , 38 : 3793 – 3803 .
- Loza , C. L. , Chan , A. W. H. , Galloway , M. M. , Keutsch , F. N. , Flagan , R. C. and Seinfeld , J. H. 2010 . Characterization of Vapor Wall Loss in Laboratory Chambers . Environ. Sci. Technol. , 44 : 5074 – 5078 .
- Matsunaga , A. and Ziemann , P. J. 2010 . Gas-Wall Partitioning of Organic Compounds in a Teflon Film Chamber and Potential Effects on Reaction Product and Aerosol Yield Measurements . Aerosol Sci. Technol. , 44 : 881 – 892 .
- May , A. A. , Presto , A. A. , Nguyen , N. T. , Hennigan , C. J. , Gordon , T. D. and Robinson , A. L. in prep . Investigating Gas-Particle Partitioning of Motor Vehicle Emissions: 1. Light Duty Gasoline Vehicles .
- Pankow , J. F. 1994 . An Absorbtion-Model Gas-Particle Partitioning of Organic Compounds in the Atmosphere . Atmos. Environ. , 28 : 185 – 188 .
- Presto , A. A. , Nguyen , N. T. , Ranjan , M. , Reeder , A. J. , Lipsky , E. M. Hennigan , C. J. 2011 . Fine Particle and Organic Vapor Emissions From Staged Tests of an In-Use Aircraft Engine . Atmos. Environ. , 45 : 3603 – 3612 .
- Ranjan , M. , Presto , A. A. , May , A. A. and Robinson , A. L. 2012 . Temperature Dependence of Gas–Particle Partitioning of Primary Organic Aerosol Emissions from a Small Diesel Engine . Aerosol Sci. Technol. , 46 : 13 – 21 .
- Riipinen , I. , Pierce , J. R. , Donahue , N. M. and Pandis , S. N. 2010 . Equilibration Times of Organic Aerosol Inside Thermodenuders: Evaporation Kinetics Versus Thermodynamics . Atmos. Environ. , 44 : 597 – 607 .
- Robinson , A. L. , Donahue , N. M. , Shrivastava , M. K. , Weitkamp , E. A. , Sage , A. M. Grieshop , A. P. 2007 . Rethinking Organic Aerosols: Semivolatile Emissions and Photochemical Aging . Science , 315 : 1259 – 1262 .
- Robinson , A. L. , Grieshop , A. P. , Donahue , N. M. and Hunt , S. W. 2010 . Updating the Conceptual Model for Fine Particle Mass Emissions from Combustion Systems . J. Air Waste Manage. Assoc. , 60 : 1204 – 1222 .
- Rogge , W. F. , Mazurek , M. A. , Hildemann , L. M. , Cass , G. R. and Simoneit , B. R. T. 1993 . Quantification of Urban Organic Aerosols at a Molecular Level – Identification, Abundance, and Seasonal Variation . Atmos. Environ. Part A - General Topics , 27 : 1309 – 1330 .
- Roth , C. M. , Goss , K. U. and Schwarzenbach , R. P. 2005 . Sorption of a Diverse Set of Organic Vapors to Diesel Soot and Road Tunnel Aerosols . Environ. Sci. Technol. , 39 : 6632 – 6637 .
- Saleh , R. , Shihadeh , A. and Khylstov , A. Y. 2011 . On Transport Phenomena and Equilibration Time Scales in Thermodenuders . Atmos. Meas. Tech. , 4 : 571 – 581 .
- Schauer , J. J. , Kleeman , M. J. , Cass , G. R. and Simoneit , B. R. T. 1999 . Measurement of Emissions from Air Pollution Sources. 2. C1 Through c30 Organic Compounds from Medium Duty Diesel Trucks . Environ. Sci. Technol. , 33 : 1578 – 1587 .
- Schauer , J. J. , Kleeman , M. J. , Cass , G. R. and Simoneit , B. R. T. 2001 . Measurement of Emissions from Air Pollution Sources. 3. C1-C29 Organic Compounds from Fireplace Combustion of Wood . Environ. Sci. Technol. , 35 : 1716 – 1728 .
- Schauer , J. J. , Kleeman , M. J. , Cass , G. R. and Simoneit , B. R. T. 2002 . Measurement of Emissions from Air Pollution Sources. 4. C1–C32 Organic Compounds from Gasoline-Powered Motor Vehicles . Environ. Sci. Technol. , 36 : 567 – 575 .
- Shrivastava , M. K. , Lipsky , E. M. , Stanier , C. O. and Robinson , A. L. 2006 . Modeling Semivolatile Organic Aerosol Mass Emissions from Combustion Systems . Environ. Sci. Technol. , 40 : 2671 – 2677 .
- Stanier , C. O. , Pathak , R. K. and Pandis , S. N. 2007 . Measurement of the Volatility of Aerosol from Alpha-Pinene Ozonolysis . Environ. Sci. Technol. , 41 : 2756 – 2763 .
- Subramanian , R. , Khylstov , A. Y. , Cabada , J. C. and Robinson , A. L. 2004 . Positive and Negative Artifacts in Particulate Organic Carbon Measurements with Denuded and Undenuded Sampler Configurations . Aerosol Sci. Technol. , 38 : 27 – 48 .
- Weitkamp , E. A. , Sage , A. M. , Donahue , N. M. and Robinson , A. L. 2007 . Organic Aerosol Formation From Photochemical Oxidation of Diesel Exhaust in a Smog Chamber . Environ. Sci. Technol. , 41 : 6969 – 6975 .
- Williams , B. J. , Goldstein , A. H. , Kreisberg , N. M. and Hering , S. V. 2010 . In Situ Measurments of Gas/Particle-Phase Transitions for Atmospheric Semivolatile Organic Compounds . Proc. Nat. Acad. Sci. , 107 : 6676 – 6681 .
- Yu , Z. , Liscinsky , D. S. , Winstead , E. L. , True , B. S. , Timko , M. T. Bhargava , A. 2010 . Characterization of Lubrication Oil Emissions from Aircraft Engines . Environ. Sci. Technol. , 44 : 9530 – 9534 .
- Yu , J. Z. , Xu , J. and Yang , H. 2002 . Charring Characteristics of Atmospheric Organic Particulate Matter in Thermal Analysis . Environ. Sci. Technol. , 36 : 754 – 761 .