Abstract
An understanding of soot aggregate optical properties is needed for the interpretation of optical measurements of soot. A modified cluster–cluster aggregation (MCCA) was used to numerically generate fractal aggregates containing 15–600 primary particles of
dp = 30 nm diameter. For each size, the aggregates with fractal dimension Df = 1.78 and 2.1 (with kf = 2.24 and 2.26, respectively) were selected, and the scattering and absorption cross sections were calculated using the discrete dipole approximation (DDA). For uncoated aggregates, the optical properties of these numerically generated aggregates were compared with the Rayleigh–Debye–Gans (RDG) approximation. It was found that the differences in the optical cross sections obtained from the two methods were small. In addition, it was also found that the aggregates with similar fractal dimension and fractal prefactor exhibit small variations in the absorption and scattering cross sections. For aggregates with transparent coatings, DDA predicted larger absorption than the core-shell Mie model for volume-equivalent spherical particles. The coating-induced absorption enhancement increases with coating thickness from 10% for a 5-nm layer to about 50%–75% absorption enhancement for a coating thickness of 20 nm. The presence of the coating on the aggregates also results in an increase in the discrepancy between the scattering predicted by the DDSCAT and the equivalent core-shell Mie model with the scattering discrepancies become larger as the coating thickness increases (the scattering discrepancies increases from 65% to 75% and from 20% to 25% for aggregates with N = 200 and 15, respectively).
Copyright 2013 American Association for Aerosol Research
1 INTRODUCTION
Soot particles in the atmosphere have adverse impacts on global climate by absorbing solar radiation and accelerating global warming (Bond and Bergstrom Citation2006). The contribution of aerosols to climate forcing, however, is still not fully understood in part due to uncertainties in soot radiative properties. Furthermore, these properties are needed for quantitative measurement of soot using optical methods. The challenges arise due to the complex soot morphology, poorly characterized refractive index of soot, and the possibility of transparent coatings on the soot particles.
Large variations in soot refractive index contribute to difficulties in characterizing soot radiative properties (Bond and Bergstrom Citation2006). Published variations in soot refractive index may reflect its dependence on the optical wavelength and actual differences in soot nanostructure, but the discrepancies may
also result from errors in the geometric model of the soot aggregates that are used to interpret scattering and absorption
measurements.
Soot particles are usually aggregates of fine spheroidal “primary” particles. These clusters are often modeled as mass fractals. That is, the number of primary particles in the aggregate (N) and its radius of gyration (Rg ) are related as follows:
Real-world particles, such as those produced in engine combustion, may be covered by unburned or partially oxidized fuel and engine lubricants as well as other organic resins (Lammel and Novakov Citation1995). Medalia et al. (1983) and Stanmore et al. (2001) reported that soot emitted by diesel engine may be encapsulated with soluble organics with a mass fraction of up to 60%, depending on the engine-operating condition. The presence of the transparent coating complicates the calculation of the optical properties. It is believed that the coating affects the soot optical properties by (a) collapsing the aggregate and making it more compact, and (b) acting as a lens that focuses incoming light into the black carbon core of the particle. The two processes brought about by the coating process result in an increase in the light scattering by the particles. For light absorption processes, (a) and (b) have contradictory effects: the collapse of the aggregate results in a decrease in particle absorption, while the addition of the coating causes an increase in absorption (Gangl et al. Citation2008; Khalizov et al. Citation2008).
Soot aggregates covered with a thick coating may collapse to form compact structures. In this case, the optical properties can be accurately predicted using the concentric core-shell Mie model. The near-spherical geometry also makes traditional size measurements easier to interpret. As a consequence, the majority of experimental studies focused on compact soot (Schnaiter et al. Citation2004; Gangl et al. Citation2008; Bueno et al. Citation2011). Some aggregates such as those found in engine exhaust, however, may only be coated with a thin layer of organic (e.g., oil or fuel) and hence, they may still retain their fractal-like structure.
Sorensen (Citation2001) provided an extensive review on the optical properties of aggregates. The light scattering is highly affected by the presence of the intracluster interaction between the primary particles in the aggregate, which in turn is highly influenced by the aggregate geometry. This means that scattering and absorption of fractal soot aggregates must be calculated using special approximation methods or advanced numerical models. In previous studies, the optical properties of this type of aggregates were often calculated by simple core-shell Mie model based on the diameter obtained from the scanning mobility particle sizer (SMPS). It is likely that this assumption will produce significant error in the estimated scattering and absorption.
Approximation methods such as Rayleigh–Debye–Gans (RDG) theory have been used for predicting the aggregate optical properties (Jullien and Botet Citation1987; Dobbins and Megaridis Citation1991; Köylü and Faeth Citation1993) since it is computationally efficient and can provide a reasonable estimation of the aggregate scattering (Sorensen Citation2001). The applications of RDG approximation, however, are usually limited for particles with refractive index near unity and the particle size must be significantly smaller than the wavelength (Bohren and Huffman Citation1983; Farias et al. Citation1996; Sorensen Citation2001).
Numerical methods that have been used in the study of soot aggregate optics include the T-matrix method (Mishchenko et al. Citation1996), method of moments (MoM) (Harrington Citation1987), generalized multisphere Mie (GMM) solution (Xu Citation1995, Citation1997), coupled electric and magnetic dipole (CEMD) method
(Mulholland et al. Citation1994), and discrete dipole approximation (DDA) (Draine and Flatau Citation1994). These numerical methods can be categorized as surface based (such as T-matrix or GMM) and volume based (such as DDA). Surface-based methods are usually faster while providing accurate estimation of the radiative properties of complex-shaped particles. However, they are usually only applicable for homogeneous aggregates composed of nonoverlapping spherical primary particles. For inhomogeneous particles such as soot aggregates covered with coating, volume-based numerical methods must be employed. In this study, the volume-based discrete dipole approach (i.e., DDA) was employed to solve the scattering and absorption problem due to its capability of calculating optical properties of objects with complicated
geometry such as coated aggregates. The main drawback of using the DDA approach is that it requires significant computer resources. Hence, the majority of studies and climate models represented coated soot by either using a simple core-shell sphere model (Schnaiter et al. Citation2003; Gangl et al. Citation2008) or applying an “effective medium” theory (Fuller et al. Citation1999; Yin and Liu Citation2010). A recent simulation study by Liu et al. (Citation2012) utilizing the core-mantle generalized multiparticle Mie with primary particle point of contact in the coating layer, however, indicated that the latter simple approximation produces inaccurate results for coated aggregate due to the inaccurate model representation.
The main focus of this study is to investigate the optical properties of coated particles using numerically generated aggregates to model soot. The DDA is used to calculate the optical properties for a refractive index chosen to represent soot. The DDA is first used to estimate the optical properties of uncoated aggregate and the results are evaluated using the commonly used Rayleigh–Debye–Gans fractal aggregate (RDG-FA) model. Afterward, DDA is employed to estimate the optical properties of aggregates coated with nonabsorbing material with the purpose of evaluating the deviation between the scattering and the absorption cross sections of coated aggregate with respect to its volume-equivalent Mie core-shell sphere.
2 METHODOLOGY
2.1 Aggregate Simulation
The aggregates used in this study were generated by an algorithm similar to that used by Rogak and Flagan (1992). This “modified cluster–cluster aggregation” (MCCA) differs from the cluster–cluster aggregation (CCA) in that CCA simulates the aggregation of clusters with various (unknown) mass ratios (Kolb et al. Citation1983; Meakin Citation1983; Heinson et al. Citation2010) while MCCA specifies the mass ratio β of the colliding clusters. In MCCA, the growing cluster of Ni (initially Ni = 1; i.e., a cluster grows from a single primary particle) is located at the center of the control space. An added cluster (of size 1 or βNi , whichever is larger) is then randomly placed near the boundary of the control space and allowed to travel following Brownian motion until it either collides with the growing cluster (the two clusters are then joined at the nearest point of contact) or strays far enough from the growing cluster and the simulation is restarted. The simulation is run until the number of primaries within the aggregate reaches the user-specified N. Two slightly different versions of MCCA (henceforth labeled as “current version” and “Rogak and Flagan (1992)”) were run for this study; in the end, the aggregates generated were very similar. Details on the MCCA algorithm are given in the online supplemental information (Section S1).
Two sets of aggregates with Df = 1.78 and 2.1 were generated using both versions of MCCA with different values of the mass ratio β. These aggregates contain N = 15, 50, 100, 200, 400, and 600 primary particles. Ten aggregates were produced for each specific N and each MCCA version. A total of 120 aggregates were generated for each Df . The sample aggregates are shown in . A MATLAB program was then used to extract the aggregate geometrical properties such as the three-dimensional (3D) radius of gyration (Rg ), which can be determined from the locations of the primary particles (ri ) within the aggregate:
TABLE 1 MCCA aggregate aspect ratio and fractal prefactor
shows the 3D aspect ratio (based on the principal axis; Fry et al. Citation2004, see the online supplemental information, Section S2). also includes the fractal prefactors derived by using EquationEquation (1) in conjunction with Df derived from the PCM. The values of the kf obtained with the PCM represent the average from 120 aggregates for each Df .
We have also studied PC-MCCA aggregates produced in a prior study (Liu and Smallwood Citation2010). The PC-CCA method uses particle-cluster growth to generate a collection of small aggregates (i.e., N ≤ 30) that obey the statistical scaling law shown in EquationEquation (1) for a specified kf and Df . Randomly selected aggregates from this collection were then joined to form larger aggregates using tunable CCA. Fractal aggregates containing N = 50, 100, 200, 400, and 600 primary particles were generated using the PC-CCA constrained to have kf = 2.3, Df = 1.78 and 2.1, and ap = 15 nm, which are typical values for combustion-generated soot.
2.2 Discrete Dipole Approximation (DDA)
The DDA (or “coupled dipole”) uses the volume-integral equation method for discretization of Maxwell's equation and can provide a flexible approach for simulating the light scattering by arbitrarily shaped particles. DDSCAT requires a small interdipole separation (d) compared with (1) any structural lengths in the target and (2) the wavelength λ. For calculation of the total optical cross sections, the second criterion can be adequately satisfied if |m|kd < 1, where k = 2π/λ (Draine and Goodman 1993; Draine and Flatau Citation1994; Draine 2000). When the target is a strong absorber or a more accurate calculation of differential scattering cross section is needed, a more conservative criterion |m|kd < 0.5 should be applied (Draine 2000).
2.2.1 DDSCAT Features
In practice, the variations in DDSCAT implementations are attributed to different configurations of dipole geometry, different models used to relate the dipole polarizabilities to complex dielectric constant, and different iterative linear system solvers used. The main DDSCAT features chosen in this study are given in ; further details are given in the online supplemental information (Section S3).
TABLE 2 DDSCAT parameters
The input parameter of the target geometry was set to ensure that the dipole distance d adheres to the conservative criterion |m|kd < 0.5. The detail discussion on the dipole distance is available in the online supplemental information (Section S3.2). For the uncoated aggregate, a default target generation “N_SPHERE” was employed, where the x, y, and z coordinates of the center of the primary particles and their radius were used as DDSCAT input.
The DDSCAT computation will provide absorption and scattering efficiencies (i.e., Q ext, Q abs, Q sca). From these efficiencies, optical cross sections (i.e., C ext, C abs, C sca) can be calculated as follows:
2.2.2 DDSCAT Accuracy
The accuracy of DDSCAT was tested on the scattering and absorption cross sections of concentric coated spheres, for which exact solutions are known. Calculations were performed for light with 532-nm wavelength, corresponding to light from an Nd:YAG laser used in many optical instruments. The distance between dipoles in the DDSCAT was set to be 2.5 nm. The diameter of the core spherical particle is assumed to be 135 nm and exhibits soot-like optical properties with refractive index, m core = 1.6 + 0.6i. The nonabsorbing coating is assumed to have a refractive index m coat = 1.46 + 0i [a value that has been used in past studies of soot coated with α-pinene (Schnaiter et al. Citation2003) and oleic acid (Slowik et al. Citation2007)]. It was found that the scattering and absorption cross sections predicted
by the DDSCAT are within 1.8% and 2.5%, respectively, of the Mie predictions (see the online supplemental information; Section S3).
3 RESULTS AND DISCUSSION
3.1 Optical Properties of Uncoated Particles
DDSCAT was applied to aggregates with Df = 1.78 to Df = 2.1 (95% confidence interval of ±0.012 and 0.007, respectively) for various values of N. The wavelength, refractive index, and dipole distance were the same as in the validation computations. All calculations use dp = 30 nm, which corresponds to the primary particle size parameter xp = πdp/λ = 0.177. Orientation averaging was performed in DDSCAT by specifying the target orientations Φ = 0 to 180 (divided into 18 equal intervals),
Θ = 0 to 180 (divided into 9 equal intervals), and β = 0 to 90 (divided into 3 equal intervals) for a total of 486 orientations. The detailed explanation of the angles used in the orientation averaging is included in the online supplemental information (Figure S6). For each value of Df and N, 20 different MCCA aggregates were used.
FIG. 3 Normalized aggregate absorption cross sections (GMM and DDSCAT results are indistinguishable at N = 400 and N = 600).
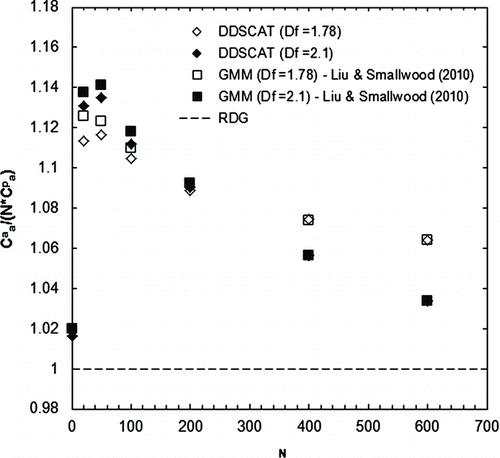
TABLE 3 The average and standard deviation of the optical cross section of the PC-CCA and MCCA as predicted by DDSCAT
summarizes the scattering cross section of the MCCA aggregates, as predicted by DDSCAT, along with predictions for other types of clusters and optical models. The aggregate scattering cross sections (Ca s ) were normalized with the cross section of primary particles (Cp s = 0.4463 nm2). The GMM points were previously published elsewhere (Liu and Smallwood Citation2010); these are based on the GMM model with aggregates generated by the PC-CCA algorithm. The widely used RDG-FA approximation (Dobbins and Megaridis Citation1991; Köylü and Faeth Citation1993) uses a simplified model of cluster geometry, considering only N and Df : it does not use the actual complex aggregate shape. In addition, an RDG simulation was performed for the numerically generated aggregates (i.e., same MCCA aggregates used in the DDSCAT computation) using T-matrix program without multiple scattering. All methods predicted that scattering increases with Df . Both DDSCAT and GMM predict higher scattering than the RDG calculations, particularly for small aggregates (i.e., N < 100). The discrepancy is smaller for large N, probably because the average density of a fractal aggregate decreases with N, making multiple scattering less important. Also, one expects an improvement in the accuracy of the RDG theory as the phase-shift parameter decreases with aggregate size (Sorensen Citation2001).
shows the normalized absorption cross sections for the same aggregates and models displayed in . The aggregate absorption cross sections (Ca s ) were normalized by the absorption cross section of primary particles
(Cp a = 135.29 nm2). Both DDSCAT and GMM include the interaction between primary particles in an aggregate while RDG does not, so RDG predicts unit normalized absorption. DDSCAT and GMM predicted that the aggregate absorption initially increases with size for small aggregate, which can be attributed to the absorption enhancement induced by the aggregation of the primary particles. For large aggregates (i.e., N > 100), the absorption decreases with N. This decrease in the absorption is likely caused by the increase in the particle shielding within the aggregate, which mitigates the aggregation-induced effect. In general, the absorption enhancement observed in this study is consistent with the absorption calculations by Mulholland et al. (Citation1994).
In order to evaluate the effect of cluster generation algorithm on optical properties, the same optical model (DDSCAT) was used for a set of MCCA and PC-CCA clusters (). The set of aggregates included five PC-CCA aggregates for each N and Df . Aggregates generated from different growth algorithms had nearly identical absorption and scattering cross sections. This is consistent with prior computational studies (Liu and Mishchenko Citation2007; Liu and Smallwood Citation2010), which found that the aggregate geometrical configuration has insignificant effect on the orientation-averaged optical cross sections of soot aggregates provided that Df and kf are similar. This means that it is reasonable to use a small number of aggregates in the numerical calculations of orientation-averaged total scattering and absorption cross sections of random-oriented ensemble of aggregates. Kolokolova et al. (Citation2006) observed that aggregates generated with either ballistic or diffusion-limited CCA may exhibit different scattering cross sections. This might be explained by differences in the fractal prefactors as described by
Heinson et al. (Citation2010). Therefore, care is needed in generalizing the results of the present work.
3.2 Optical Properties of Coated Aggregates
Having characterized the simulated aggregates and verified the DDSCAT calculations for uncoated aggregates, we now turn to the chief contribution of this study, i.e., the prediction of optical properties for coated aggregates. We consider the case of thin coatings that do not affect the solid aggregate morphology, as illustrated in . For clarity, the coating thickness δ is shown on an isolated primary particle.
In this study, the DDSCAT was applied to predict the scattering and absorption cross sections of coated aggregates and the results are compared with the coated sphere Mie model.
In the simulation, the nonabsorbing coating material was assumed to have m coat = 1.46 + 0i, with the purpose of mimicking the organic coating used in the past experimental studies.
The Mie predictions were based on the core sphere with volume
compares the absorption of coated aggregates with that of volume-equivalent spheres for δ = 5 nm. In DDSCAT, the dipoles for the coating form spherical shells that overlap (because the core primary particles are in contact); the extra dipoles in the overlap volumes were removed in order to provide a more accurate representation. Due to this overlap, the coating increases the particle volume by a factor of 1.8–1.9 relative to the uncoated core; neglecting the overlap would result in a volume of (40/30)3 = 2.37 times the core volume. The DDSCAT simulation was performed for 20 aggregates for each Df and N combination. The standard error in the absorption ratio was less than 1.2% for each point. As shown by the circle symbol in , the DDSCAT provided accuracy within 1% of the Mie theory for estimating the absorption for the concentric coated spherical particle with a core diameter of 30 nm and a coating thickness δ of 5 nm. For this case, the aggregate absorbs more light, especially for low Df . For Df = 1.78, the discrepancy between DDSCAT and the core-shell Mie model increases with size until N ∼ 100, and then decreases for N > 100. Bueno et al. (Citation2011) observed a better agreement between the experimental data and the Mie model was for larger aggregates, which is consistent with the trend discussed above.
FIG. 6 Ratio of DDSCAT/Mie for coated aggregate absorption for various coating thicknesses (aggregate N = 200 and Df = 1.78).
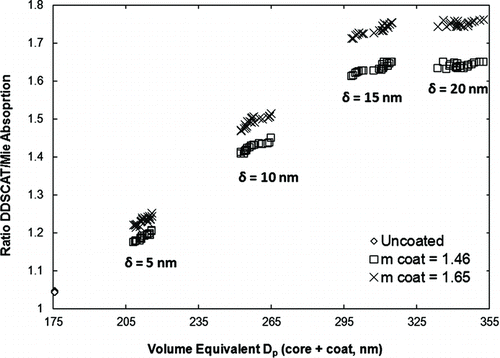
For a specified size and fractal dimension (N = 200, Df = 1.78), the effects of coating thickness and refractive index on aggregate absorption and scattering are investigated further. The core primary particle diameter is 30 nm as before and the core refractive index, m core = 1.6 + 0.6i. In addition to the baseline refractive index (m coat = 1.46 + 0i), calculations are performed for m coat = 1.65 + 0i (a plausible increase given the wide range of possible coating materials on aggregates). Thicker coatings, with a higher refractive index, increase the absorption of the aggregates relative to that of the volume-equivalent sphere as shown in . The 20 uncoated core aggregates are represented by the points at the left-hand axis (Dp ≈ 175 nm); this shows that the Mie model and DDSCAT differ by only 5%. Further information on the absorption cross sections as predicted by the Mie core-shell sphere model can be found in the online supplemental information (Section S4).
FIG. 7 Ratio of DDSCAT/Mie for coated aggregate scattering for various coating thicknesses (aggregate N = 200 and Df = 1.78).
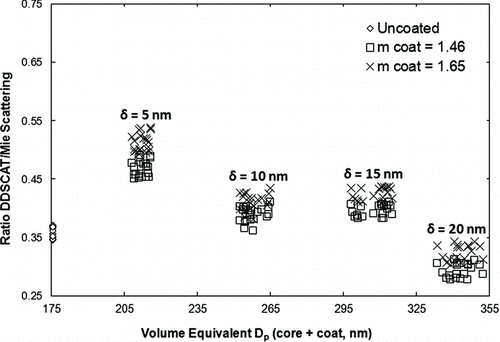
FIG. 8 Ratio of DDSCAT/Mie for coated aggregate absorption for various coating thicknesses (aggregate N = 15 and Df = 1.78).
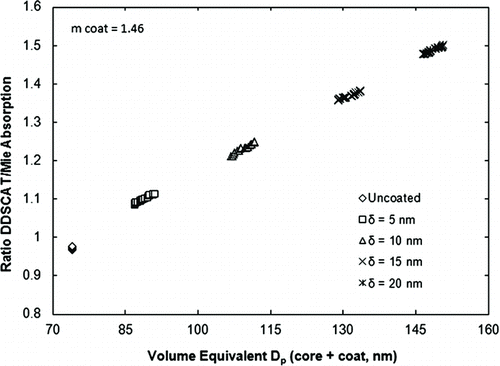
For the same aggregates and coatings of ,
displays the normalized scattering. Even for uncoated aggregates, scattering is much lower than that of the volume-equivalent sphere, implying the importance of analyzing soot as an aggregate instead of a spherical particle for scattering-based soot measurement techniques. The discrepancies between the aggregate scattering predicted by the DDSCAT and the core-shell Mie model decrease when the transparent coating was first introduced. It is suspected that this is caused by the enhancement effect on the aggregate scattering due to the coating that initially suppressed the aggregate multiple scattering effects.
The effect of coating on the absorption for small aggregates (with N < 30) was also investigated. This type of aggregate is commonly observed in the engine exhaust. In this case, the core particles are specified to be aggregates with N = 15, Df = 1.78, and m coat = 1.46. It can be observed in that similar as before, the coating enhances the absorption of aggregates compared with its volume-equivalent sphere counterpart. For small aggregates, it should be noted that absorptions for uncoated aggregates are slightly less compared with its volume-equivalent spherical particles. In addition, it can be observed that the discrepancy between the absorption predicted by the DDSCAT and the equivalent core-shell Mie model is still growing even for the largest value of delta (δ). It is likely that this increasing trend will eventually reach a plateau at a thicker coating condition, just like in the large aggregate case.
shows the scattering for the same set of aggregates investigated in . In general, there seems to be a decreasing trend in DDSCAT/Mie scattering ratio as the coating increases.
4 CONCLUSIONS
In this article, the optical properties of simulated coated and uncoated fractal-like aggregates have been investigated numerically. Essential groundwork includes careful characterization of the aggregate morphology and validation of the scattering/absorption calculations.
Fractal aggregates containing 15–600 primary particles of dp = 30 nm in diameter are numerically generated using an MCCA method. For each size, the aggregates with fractal dimension Df = 1.78 and 2.1 (with kf = 2.24 and 2.26, respectively) were selected. The DDA (software package: DDSCAT version 7.0) has been used to estimate the optical properties for fractal aggregates produced from MCCA, using a refractive index representative of soot. For uncoated aggregates, the scattering and absorption predictions from DDSCAT and the RDG approximation were close. It was observed that aggregates with similar fractal properties (Df and kf ) exhibited small variation in the orientation-averaged scattering and absorption cross sections, which are the properties of practical interest.
Optical cross sections for both small (N = 15) and large
(N = 200) aggregates coated with nonabsorbing material were investigated. The DDSCAT aggregate model generally predicted higher absorption than the volume-equivalent core-shell Mie sphere. The increase in the coated aggregate absorption is influenced by the amount of the coating and the coating refractive index. It is observed that the influence of the coating refractive index on the coated aggregate absorption was relatively insignificant when the coating is thin (about 10% absorption enhancement at coating thickness δ = 5 nm), but became more important as the coating thickness increased (about 50%–75% absorption enhancement at coating thickness δ = 20 nm). Similarly, the presence of the coating on aggregates also generally results in an increase in the discrepancy (about 10% increase from uncoated aggregate to coated aggregate with δ = 20 nm) between the scattering predicted by the DDSCAT and the equivalent core-shell Mie model. It should be noted, however, that the increase in scattering discrepancy becomes less important considering that the discrepancies between uncoated aggregate and its volume-equivalent Mie sphere are already at 20%–60%, depending on the aggregate size. These findings highlight the importance of using a true aggregate model in estimating the optical properties of coated soot particles that are not fully
collapsed.
uast_a_749972_sm9123.zip
Download Zip (589.8 KB)Acknowledgments
[Supplementary materials are available for this article. Go to the publisher's online edition of Aerosol Science and Technology to view the free supplementary files.]
REFERENCES
- Bohren , C. F. and Huffman , D. R . 1983 . Absorption and Scattering of Light by Small Particles , New York : Wiley .
- Bond , T. and Bergstrom , R. W. 2006 . Light Absorption by Carbonaceous Particles: An Investigative Review . Aerosol Sci. Technol. , 39 : 27 – 67 .
- Bueno , P. A. , Havey , D. K. , Mulholland , G. W. , Hodges , J. T. , Gillis , K. A. Dickerson , R. R. 2011 . Photoacoustic Measurements of Amplification of the Absorption Cross Section for Coated Soot Aerosols . Aerosol Sci. Technol. , 45 ( 10 ) : 1217 – 1230 .
- Dobbins , R. A. and Megaridis , C. M. 1991 . Absorption and Scattering of Light by Polydisperse Aggregates . Appl. Opt. , 30 : 4747 – 4754 .
- Draine , B. T. and Flatau , F. J. 1994 . Discrete-Dipole Approximation for Scattering Calculations . J. Opt. Soc. Am. A , 11 : 1491 – 1499 .
- Farias , T. L. , Köylü , U. O. and Carvalho , M. G. 1996 . Range of Validity of the Rayleigh–Debye–Gans Theory for Optics of Fractal Aggregates . Appl. Opt. , 35 : 6560 – 6567 .
- Fry , D. , Mohammad , A. , Chakrabarti , A. and Sorensen , C. M. 2004 . Cluster Shape Anisotropy in Irreversibly Aggregating Particulate Systems . Langmuir , 20 : 7871 – 7879 .
- Fuller , K. A. , Malm , W. C. and Kreidenweis , S. M. 1999 . Effects of Mixing on Extinction by Carbonaceous Particles . J. Geophys. Res. , 104 : 15941 – 15954 .
- Gangl , M. , Kocifaj , M. , Videen , G. and Horvath , H. 2008 . Light Absorption by Coated Nano-Sized Carbonaceous Particles . Atmos. Environ. , 42 : 2571 – 2581 .
- Harrington , R. 1987 . The Method of Moments in Electromagnetics . J. Electromagn. Waves Appl. , 1 : 181 – 200 .
- Heinson , W. R. , Sorensen , C. M. and Chakrabarti , A. 2010 . Does Shape Anisotropy Control the Fractal Dimension in Diffusion-Limited Cluster-Cluster Aggregation? . Aerosol Sci. Technol. , 44 : i – iv .
- Jullien , R. and Botet , R. 1987 . Aggregation and Fractal Aggregates , Singapore : World Scientific .
- Khalizov , A. , Xue , H. , Wang , L. , Zheng , J. and Zhang , R. 2008 . Enhanced Light Absorption and Scattering by Carbon Soot Aerosol Internally Mixed with Sulfuric Acid . J. Phys. Chem. A , 113 : 1066 – 1074 .
- Kolb , M. , Botet , R. and Jullien , R. 1983 . Scaling of Kinetically Growing Clusters . Phys. Rev. Lett. , 51 : 1123 – 1126 .
- Kolokolova , L. , Kimura , H. , Ziegler , K. and Mann , I. 2006 . Light-Scattering Properties of Random-Oriented Aggregates: Do They Represent the Properties of an Ensemble of Aggregates? . J. Quant. Spectrosc. Radiat. Transf. , 100 : 199 – 206 .
- Köylü , Ü. Ö. and Faeth , G. M. 1993 . Radiative Properties of Flame-Generated Soot . ASME J. Heat Transf. , 115 : 409 – 417 .
- Lammel , G. and Novakov , T. 1995 . Water Nucleation Properties of Carbon Black and Diesel Soot Particles . Atmos. Environ. , 29 : 813 – 823 .
- Liu , C. , Panetta , R. L. and Yang , P. 2012 . The Influence of Water Coating on the Optical Scattering Properties of Fractal Soot Aggregates . Aerosol Sci. Technol. , 46 : 31 – 43 .
- Liu , F. and Smallwood , G. 2010 . Radiative Properties of Numerically Generated Fractal Soot Aggregates: The Importance of Configuration Averaging . J. Heat Transf. , 132 : 023308
- Liu , L. and Mishchenko , M. I. 2007 . Scattering and Radiative Properties of Complex Soot and Soot-Containing Aggregate Particles . J. Quant. Spectrosc. Radiat. Transf. , 106 : 262 – 273 .
- Meakin , P. 1983 . Formation of Fractal Clusters and Networks by Irreversible Diffusion-Limited Aggregation . Phys. Rev. Lett. , 51 : 1119 – 1122 .
- Mishchenko , M. I. , Travis , L. D. and Mackowski , D. W. 1996 . T Matrix Computations of Light Scattering by Non-Spherical Particles: A Review . J. Quant. Spectrosc. Radiat. Transf. , 55 : 535 – 575 .
- Mulholland , G. W. , Bohren , C. F. and Fuller , K. A. 1994 . Light Scattering by Agglomerates: Coupled Electric and Magnetic Dipole Method . Langmuir , 10 : 2533 – 2546 .
- Schnaiter , M. , Horvath , H. , Mohler , O. , Naumann , K.-H. , Saathoff , H. and Schock , O. 2003 . UV-VIS-NIR Spectral Optical Properties of Soot and Soot-Containing Aerosols . J. Aerosol Sci. , 34 : 1421 – 1444 .
- Schnaiter , M. , Linke , C. , Mohler , O. , Naumann , K.-H. , Saathoff , H. Wagner , R. 2005 . Absorption Amplification of Black Carbon Internally Mixed with Secondary Organic Aerosol . J. Geophys. Res. , 110 : D19107
- Slowik , J. , Cross , E. S. , Han , J.-H. , Kolucki , J. , Davidovits , P. Williams , L. R. 2007 . Measurements of Morphology Changes of Fractal Soot Particles Using Coating and Denuding Experiments: Implications for Optical Absorption and Atmospheric Lifetime . Aerosol Sci. Technol. , 41 : 734 – 750 .
- Sorensen , C. M. 2001 . Light Scattering by Fractal Aggregates: A Review . Aerosol Sci. Technol. , 35 : 648 – 687 .
- Xu , Y.-L. 1995 . Electromagnetic Scattering by an Aggregate of Spheres . J. Appl. Opt. , 34 : 4573 – 4588 .
- Xu , Y.-L. 1997 . Electromagnetic Scattering by an Aggregate of Spheres: Far Field . J. Appl. Opt. , 36 : 9496 – 9508 .
- Yin , J. Y. and Liu , L. H. 2010 . Influence of Complex Component and Particle Polydispersity on Radiative Properties of Soot Aggregate in Atmosphere . J. Quant. Spectrosc. Radiat. Transf. , 111 : 2115 – 2126 .