Abstract
Mass–mobility measurements using a centrifugal particle mass analyzer (CPMA) and differential mobility spectrometer (DMS) are demonstrated. The CPMA, which classifies an aerosol by mass-to-charge ratio, is used upstream of a DMS, which measures the mobility size distribution of the mass-classified particles in real-time. This system allows for mass–mobility measurements to be made on transient sources at one particle mass or an entire effective density distribution for steady state sources in minutes. Since the CPMA classifies particles by mass-to-charge ratio and multiply charged particles are present, particles of several different masses will be measured by the DMS. Therefore, a correction scheme is required to make accurate measurements. To validate this measurement scheme, two different CPMA-DMS systems were used to measure the known density of di(2ethylhexyl) sebacate (DEHS). The first system consisted of a CPMA and standard DMS500 (Cambustion). This system measured an average effective density of 1027 kg/m3 or within 12.6% of the accepted value with an estimated uncertainty of 30.1% (with 95% confidence). The second system consisted of a CPMA and modified DMS. The modified DMS was a DMS500 with the corona charger disabled and sample and sheath flow rates lowered, decreasing the uncertainty in the mobility measurement. This system measured an average effective density of 964 kg/m3 or within 5.7% of the accepted value with an uncertainty of 9.5–10.4% depending on particle mobility size. Finally, it was determined that multiple-charge correction and size calibration were required, with each correction causing a maximum change in measured effective density greater than 10%.
Copyright 2013 American Association for Aerosol Research
1. INTRODUCTION
Particulate matter (PM) can have an adverse effect on the environment (Hinds Citation1999), human health (Pope Citation2000), and global climate (Forster et al. Citation2007). Therefore, particulate emissions are regulated and controlled in the interest of the public. While the measurement methods and legislation vary between source types (automotive [ISO Citation2002] versus aerospace [SAE Citation2010] for example), these regulations are usually number- and/or mass-based.
The relationship between particle mass (m) and mobility diameter (d m) is typically given through the mass–mobility exponent, d m defined as,
Traditionally, the effective density is measured using a differential mobility analyzer (DMA), a mass analyzer, and a condensation particle counter (CPC) in series, where the mass-analyzer could either be a centrifugal particle mass analyzer (CPMA; Olfert et al. Citation2006) or an aerosol particle mass analyzer (APM; Ehara et al. Citation1996). While both instruments classify particles by their mass-to-charge ratio by balancing opposing electrostatic and centrifugal forces, the CPMA’s inner cylinder is spun slightly faster than the outer cylinder to generate Couette flow. This subtle difference from the APM’s cylinders (which rotate at the same angular velocity) creates a stable system of forces on the particles, thereby improving the transmission efficiency of the CPMA over the APM (Olfert and Collings Citation2005). For the remainder of this article, the terms CPMA and APM are interchangeable as the proposed method was developed using a CPMA, but would also work with an APM.
These mass analyzers have been used to measure the effective density of diesel soot (Park et al. Citation2003a; Olfert et al. Citation2007; Barone et al. Citation2011), flame soot (Kim et al. Citation2009b; Xue et al. Citation2009; Cross et al. Citation2010; Ghazi et al. Citation2013), carbon nanofibres/nanotubes (Ku et al. Citation2006; Kim et al. Citation2009a), oils or secondary organic aerosols (SOA) (Malloy et al. Citation2009), metallic or silica agglomerates (Shin et al. Citation2009; Scheckman et al. Citation2009; Eggersdorfer et al. Citation2012), Aquadag and fullerine soot (Gysel et al. Citation2011), and nanoparticles produced by spray-drying (Lee et al. Citation2009, Citation2011). In this setup, a DMA, with a charge neutralizer, is used to electrically charge and select the aerosol based on electrical mobility. The particles exiting the DMA will have a narrow range of electrical mobilities. However, due to the possibility of multiple-charge states, the particles will likely have different mechanical mobilities. The CPMA further classifies the particles by mass-to-charge ratio. The concentration of classified particles is measured by a CPC. By stepping the voltage and/or rotational speed of the CPMA, the aerosol concentration is recorded as a function of the CPMA operating condition. If no multiply charged particles are present and the particles have a uniform effective density over the range of mobilities, then this function is approximately normally distributed and the maximum corresponds to the average mass of the particles exiting the CPMA. Alternatively, if the resolution of the DMA and CPMA is sufficiently high, or the fraction of multiply charged particles is sufficiently low, and the mass-to-charge ratios of the particles with equivalent electrical mobility are sufficiently different, then the multiply charged particles are seen as separate maxima in the number concentration function. These maxima can be fit with a least squares minimization using an asymmetric normal distribution or a lognormal distribution as done by Tajima et al. (Citation2011) or by a function constructed by the convolution of the DMA and CPMA transfer functions (Emery Citation2005; Barone et al. Citation2011). Otherwise, other correction schemes are required (Olfert et al. Citation2007; Shin et al. Citation2009).
Another method to determine the mass-mobility of aerosols uses a scanning mobility particle sizer (SMPS; Wang and Flagan Citation1990) and electrical low-pressure impactor (ELPI; Keskinen et al. Citation1992) in parallel. The mobility size distribution (measured by the SMPS) and aerodynamic diameter (measured by the ELPI) are related by the average effective density of the particles (Ahlvik et al. Citation1998). This method was used by Ristimaki et al. (Citation2002) to measure particles of DOS, Santovac vacuum oil, and Fomblin vacuum oil and by Virtanen et al. (Citation2004) to measure DOS and silver agglomerate particles. A variation of this method places a DMA in series with an ELPI. This experimental set-up was used by Maricq and Xu (Citation2004) to measure poly-α-olefin oil aerosol, diesel engine exhaust, and direct injection spark ignition engine exhaust. Fernandez de la Mora et al. (Citation2003) used a DMA and a focused impactor to measure the mobility and mass of silver particles with mobility diameters below 10 nm.
FIG. 1 Experimental set-up for neutralizer–CPMA–DMS system where:




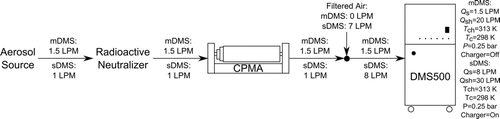
DeCarlo et al. (Citation2004) also showed that the relationship between mobility and vacuum aerodynamic diameter can be used to determine the effective density of aerosol particles. A DMA or SMPS is used to classify/measure the particles based on electrical mobility, while the vacuum aerodynamic diameter is measured using an aerosol mass spectrometer (AMS; Jayne et al., Citation2000). Malloy et al. (Citation2009) used the parallel combination of an SMPS and AMS to measure the effective density of SOA and this was compared against results obtained using a tandem APM-SMPS system, where the SMPS was scanned downstream of the APM operating at fixed conditions.
The mass–mobility relationship can also be determined for very small particles (<3 nm) using a tandem DMA-mass spectrometer system, where the particle mass is directly measured by the mass spectrometer, as demonstrated by Larriba et al. (Citation2011).
Although the DMA–CPMA–CPC system is relatively accurate (determined in the online supplemental information to be 9.4% in terms of effective density), the stepping aspect of this method limits its applications to steady state measurements. This article will explain how a CPMA and differential mobility spectrometer (DMS; Reavell et al., Citation2002; Biskos et al., Citation2005; Symonds et al., Citation2007) system, with a known or independently determined mobility size distribution, can measure the effective density of aerosol particles. In this system, the particles are neutralized by a radioactive source, classified by mass-to-charge ratio by the CPMA, and then the electrical mobilities of the mass-classified particles are measured in real-time with a DMS. Thus, the effective density of one particle size can be measured in real-time, which is necessary for transient sources, or the effective density of several particle sizes can be measured in a few minutes from steady state sources. However, these advantages come at the cost of uncertainty compared to the traditional DMA–CPMA–CPC system. This work will describe a correction scheme to account for the multiply charged particles classified by the CPMA. Two DMS500 (Cambustion, UK) systems were tested, a standard DMS500 and a modified DMS500. The modified DMS had its charger disabled and flow rates dropped to decrease the mobility measurement uncertainty. These two CPMA–DMS systems were validated by measuring the effective density of di(2ethylhexyl) sebacate (DEHS).
2. EXPERIMENTAL SET-UP
The CPMA–DMS system is shown in . For the specific example data shown herein, the aerosol was DEHS droplets suspended in nitrogen, generated by atomizing a solution of 2-propanol (HPLC grade) with 0.1% volume concentration DEHS using a BGI 3-Nozzle Collison Nebulizer. A radioactive neutralizer is used to charge the aerosol as a prerequisite for CPMA classification. The CPMA (Cambustion, UK) is set to a constant mass-to-charge setpoint (i.e., constant rotational speed and voltage) and the mobility size distribution of the classified aerosol is measured by a DMS. A CPMA classifies individual aerosol particles by their mass-to-charge ratio by balancing opposing electrostatic and centrifugal forces. The electrostatic force is generated by charging the particles upstream of the CPMA and placing a voltage potential across the CPMA’s inner and outer cylinders. The centrifugal force is generated by spinning the cylinders.
A DMS measures the real-time mobility size distribution of an aerosol by classifying particles using electrical mobility. A corona charger is used to charge the aerosol particles before entering the DMS classifier. The classifier consists of two concentric cylinders with a potential difference between them. Similar to a DMA, the motion of charged particles in an electric field is dependent on their electrical mobility, and hence their electrical mobility diameter. These mobility paths are measured by electrometer rings, placed on the inner face on the outside classifier cylinder. These rings count the number of charges that fall into each electrical mobility bin by measuring the current generated by charged particles grounding on the electrometer rings. An inversion algorithm then converts these ring currents into a electrical mobilty size spectral density plot.
2.1. Standard vs. Modified DMS
Two different DMS500 configurations were used in this study; a standard DMS (sDMS) and modified DMS (mDMS). The sDMS unipolar charging process has higher uncertainties at smaller particles sizes (0–100 nm) and is dependent on particle morphology (Symonds and Reavell, Citation2007; Symonds, Citation2010). Therefore, the mDMS had its charger disabled to improve its measurement uncertainty. Without the charger, the aerosol had lower electrical mobility and required a longer flight-time in the column to be classified. Therefore, the sample flow rate was dropped from 8 SLPM to 1.5 SLPM and the sheath flow rate was dropped from 30 SLPM to 20 SLPM, increasing the particle residence time. The lower sample flow rate also allowed direct sampling of the CPMA-classified aerosol without make-up air, as it is designed for 1.5 LPM, and lower flow rates are generally desired for higher resolution at lower rotational speeds.
However, these modifications limited the mDMS sizing range to 10–120 nm and reduced the current signal from the mDMS electrometer rings significantly. The inversion matrix used with the mDMS assumed all the particles were singly charged. This assumption amplified the error from existing multiply charged particles as a particle with n charges produced n times the current compared to a particle with 1 charge, making the mDMS measure n times the actual particle concentration. However, this amplication effect for the mDMS was accounted for within the correction method. This effect was accounted for in the sDMS by the inversion matrix considering the particle charge distribution leaving the sDMS corona charger. The inversion matrix used with the mDMS also produced 64 size-classes per decade, compared with the sDMS which had the standard 16 size-classes per decade.
3. THEORY
3.1. Effects of Uncharged Particles
In a CPMA, an uncharged particle experiences no electrostatic force and the centrifugal force is directly proportional to its mass. Therefore, if the particle has an extremely small mass, the centrifugal force is insufficient for classification to occur over its residence time in the CPMA. As a result, uncharged particles below a cutoff mass (which is dependent on the rotational speed of the cylinders) will pass through the CPMA. This cutoff mass was determined by calculating the CPMA transfer function in the absence of the electrostatic force (i.e., when the particles only experience diffusion and centrifugal forces). Further details regarding the CPMA cutoff mass and its calculation are outlined in Symonds et al. (Citation2013).
Uncharged particles are not measured by the modified DMS and therefore a correction for uncharged particles is not required. However, the corona charger in the standard DMS charges the uncharged particles affecting the mobility size distribution it measures. Standard DMS data can be corrected for uncharged particles in two ways:
I. | Manually setting the DMS ring currents generated by uncharged particles to a value of zero “by eye.” | ||||
II. | Calculating the theoretical DMS ring currents generated by the uncharged particles and removing these currents from the measured currents. |
The higher the electrical mobility of the particle, the lower the DMS ring number it lands on (i.e., ring number 1 measures the particle with the highest electrical mobility or smallest mobility-equivalent diameter). Therefore, the uncharged particles, which are always smaller than the classified particles, generate current on the lower DMS rings, which usually makes the DMS ring current distribution bimodal, as shown in the online supplemental information. Therefore, Method (I) works well when there is a clear definition between these modes or the DMS ring currents generated by the uncharged particles versus the classified particles. The effects of uncharged particles vary from being insignificant to generating a majority of the DMS ring currents, depending on the CPMA rotational speed, mobility size, and concentration of the aerosol being classified and the CPMA mass-to-charge setpoint.
While Method (I) is computationally faster, it does not work when the current generated by the uncharged particles and the classified particles are detected on common DMS rings as shown in the online supplemental information. Therefore, Method (II) must be applied to find and remove the current on each DMS ring generated from uncharged particles. The effect of uncharged particles in a standard DMS can be minimized by operating the CPMA at a higher resolution, which, in turn, classifies particles at a higher rotation speed, or setting the CPMA mass-to-charge setpoint to select from the leading edge (the smaller particle side) of the particle size distribution.
3.2. DMS Ring Current Correction Method
An iterative scheme was used to correct the effective density measurements for multiply charged particles for both the standard and modified DMS. The correction code was developed in Matlab R2012a and considers the effects of particle diffusion in both the CPMA and DMS. The code can be downloaded at www.cambustion.com/cpma. The Matlab CPMA diffusive transfer function was developed by Olfert and Collings (Citation2005) and the Matlab DMS diffusive transfer function was developed by Cambustion (Citation2011).
The following steps outline the method used for the multiple-charge correction as shown in . If necessary, the DMS ring currents can be corrected for noise before applying the multiple-charge correction. Noise removal and re-inversion are required at lower aerosol concentrations, especially for the modified DMS as the DMS corona charger is disabled resulting in lower currents. For example, the classified particles could generate current on DMS rings 5–10; however, ring 21 could also be detecting a small amount of current. This lone ring current is extremely unlikely to be generated physically as even a monodispersed particle distribution has a distribution of charges and starting points at the DMS classifier inlet. Therefore, DMS ring currents are usually generated on multiple consecutive rings and any lone, isolated ring current is likely to be instrument noise. In such a case, the current on ring 21 would be set to zero and the DMS measured mobility size distribution recalculated.
FIG. 2 Correction method to remove the effects of multiply charged particles and apply the size calibration to CPMA–DMS measurements. The subfigures illustrate the results for one data point and depict the: (a) unclassified aerosol mobility size distribution, (b) CPMA-classified mobility size distribution including uncharged particles, (c) CPMA-classified mobility size distribution, (d) unscaled DMS model ring currents, (e) scaled DMS model ring currents, (f) multiply charged corrected standard DMS ring currents, (g) multiply charged corrected modified DMS ring currents, and (h) multiply charged corrected size distribution fitted with a lognormal curve.
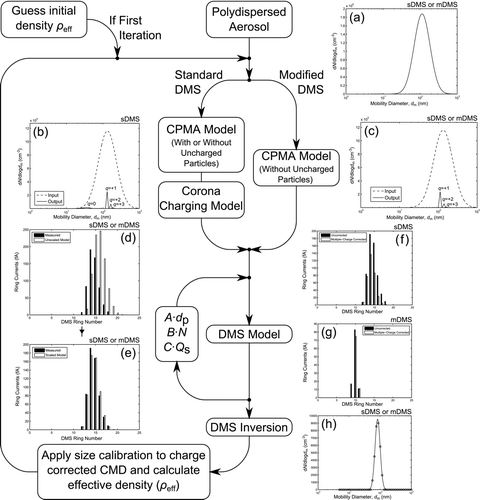
An initial guess for the effective density of the aerosol is made for the CPMA diffusive model. This guess is required as the width of the CPMA transfer function, also referred to as the CPMA resolution, is dependent on particle mobility and this is the reason that the correction process is iterative. The correction process allows a user-defined initial guess, using either a constant density or power law density distribution, or for the guess to be calculated for each CPMA mass-to-charge setpoint using the uncorrected (no multiple-charge correction or size calibration applied) measured DMS count median diameter (CMD). The effective densities are fitted with a density function with the functional form shown in Equation (Equation2). For each CPMA mass-to-charge setpoint, the diffusive CPMA transfer function is calculated using the density function. This transfer function is applied to the polydisperse aerosol, which has to be measured independently of the CPMA–DMS system (using, for example, a DMS or an SMPS), as shown in . These calculations determine the mobility size and number of particles that were classified by the CPMA for each particle charge state. shows the mobility size distribution of the aerosol exiting the CPMA for the standard DMS including uncharged particles, while shows the mobility size distribution of the aerosol exiting the CPMA for the modifed DMS or the standard DMS with no uncharged particles. If an APM was used instead of a CPMA, the only modification to the current correction scheme would be applying the APM transfer function rather than the CPMA transfer function.
The model assumes the polydispersed aerosol has an equilibrium bipolar charge distribution using either the method described by Wiedensohler (Citation1988) or Gopalakrishnan et al. (Citation2013). When the elemental charge state considered is greater than 2 or less than –2 (the limits of these models) the equilibrium charge fraction is determined using Gunn and Woessner (Citation1956). A brief description of these bipolar charging models is found in the online supplemental information. The model allows the user to specify the number of charge states that are classified by the CPMA. For the results shown, 1 to 6 elemental charges were considered by the CPMA transfer function. These limits on the number of elemental charge states were determined by running the model with increasing charge states and establishing when the results became independent of this parameter.
For the standard DMS, a corona or unipolar charging model is applied to determine the new charge states of the classified particles. This model was developed by Cambustion and allows the user to calculate the particle charging based on White (Citation1951) or Fuchs (Citation1963). A brief description of these unipolar charging models is shown in the online supplemental information. The corona charger generates a charge distribution for each particle mobility size. Based on Fuch’s charging model, a 100 nm particle has a 14.11% probability of having 3 elemental charges, 48.49% of having 4 charges, 31.61% of having 5 charges, 5.15% of having 6 charges and 0.64% of having other charge states. The correction code for the standard DMS has the option to use the entire charge distribution or the average charge state. The average charge state (DMS Model 2) is the mean number of elemental charges on a particle at one particular mobility size (for example, the 100 nm particle mentioned earlier has a mean charge state of 3.96 elementary charges). The entire charge distribution can be implemented in two ways. The first method (DMS Model 1) applies the charge probabilities to each CPMA-classified mobility size, determining the particle concentration for each particle mobility size and charge state. The second method (DMS Model 3) applies the charge distribution within the diffusive DMS model, and selects which bins to calculate based on the probability of each charge state. Therefore, if sufficient iterations are selected, DMS model 3 and DMS model 1 should agree. All of these DMS models apply the same DMS diffusive transfer function and any of the unipolar charger models implemented; the variation between them is the number of bins considered in the calculation and how these bins are generated in an effort to reduce computation requirements. Therefore, the diffusive DMS transfer function is applied based on the DMS model selected and determines the amount of theoretical current generated on each DMS ring. The theoretical ring currents are then compared against the measured ring currents as shown in .
The measured and theoretical currents do not always agree due to uncertainties in measured parameters or the theoretical models.Footnote 2 There are uncertainties in flows as the DMS does not measure the sample flow in real-time, but only when zeroing the instrument. Furthermore, the charging model does not account for the penetration efficiency of the corona charger used in the sDMS. Finally, both the CPMA and DMS models do not account for particles losses in the inlets and outlets of each instrument. Therefore, the disagreement between the measured and theoretical ring currents is minimized by using the Matlab function fmincon and:
• | Applying a factor A to the mobility size of the classified particles, which shifts the peak of the theoretical currents along the DMS rings. | ||||
• | Applying a factor B to the concentration of the classified particles, which scales the amplitude of the theoretical ring currents. | ||||
• | Applying a factor C to the sample flow rate of the DMS, but keeping the sum of the DMS sample flow rate and sheath flow rate a constant. This scales the width of the DMS transfer function or the width of rings that the particles land on, but keeps C independent of B. |
Constrained minimization is used to limit the scaling factors within a user-specified tolerance of the measured values and avoids the generation of unrealistic factors. This principle is also applied to every other fitting scheme in the code; for example, the mass–mobility exponent for the density function fit was limited between 1 and 3 for these results. After the scaling factors are determined through constrained minimization, as shown in , the current generated by multiply charged particles and uncharged particles (if considering that particular case for the standard DMS) is subtracted from the measured ring currents. shows the multiple-charge corrected ring currents for the standard DMS, while shows the multiple-charge-corrected ring currents for the modified DMS. As expected the current is removed from the larger ring numbers for the standard DMS, but is removed from the smaller ring numbers for the modified DMS. This difference is due to the corona charger in the standard DMS which changes the electrical mobility of the CPMA-classified particles.
Since the minimization applies linear scaling factors to a nonlinear system, not every data point agrees with the theoretical model. This disagreement is measured by the difference between the percent of current that should be removed from the DMS ring currents and the percent of current that is actually removed by the model. The percent of current that should be removed is calculated using the theoretical CPMA-classified mobility size distribution and is the sum of each particle concentration times its charge state divided by the concentration of singly charged particles. If the experimental data and theoretical model agree perfectly, this difference is zero.
Next, the corrected ring currents are re-inverted to a mobility size distribution using the DMS inversion matrix and fitted with a lognormal distribution as shown in . The CMD from the lognormal fit is corrected with one of the size calibration curves (shown in the online supplemental information). Using the multiple-charge and size-calibrated CMD and the CPMA mass-to-charge setpoint, the corrected effective density is calculated and used as the guess for the next iteration. The difference between the current and previous iteration effective density is determined for each data point and the mean of this array is used as a convergence tolerance. This correction process continues iterating until the convergence tolerance is below the user-specified limit.
4. RESULTS AND DISCUSSION
The experimental set-up and correction method was validated by measuring the effective density of DEHS with two neutralizer–CPMA–DMS systems. DEHS was chosen as its droplets have a spherical morphology and its density remains constant over a range of mobility sizes. The accepted effective density was determined from Haynes (Citation2013) to be 912 kg/m at the DMS classifier conditions (T = 298 K).
4.1. Size Calibration
Accurate particle sizing is extremely important for mass–mobility measurements as effective density is proportional to the particle mobility diameter cubed. A 5% measurement error in particle sizing results in a 15% error in measured effective density.Footnote 3 To limit the uncertainty in sizing both the standard and modified DMS were calibrated (shown in the online supplemental information).
4.2. CPMA–Modified DMS Results
The effective density of DEHS measured by the CPMA–mDMS system, at a CPMA resolution of 3 and using the Wiedensohler bipolar charging model within the correction process, is shown in . The average measured effective density was 964 kg/m or within 5.7% of the accepted value. The error bars represent the instrument bias uncertainty and is explained further in the online supplemental information. Applying a 95% confidence interval, the precision uncertainty of the CPMA–mDMS was 33 kg/m
and the total uncertainty was 89–115 kg/m
(10.3–10.9%) depending on the particle mobility size.
FIG. 3 (a) Measured effective density of DEHS using a CPMA with a resolution of 3 and modified DMS system, applying the Wiedensohler bipolar charging model within the correction process. (b) Comparison of DEHS effective density values calculated from the data described in (a) with: (i) no correction, (ii) size calibration only, (iii) multiple-charge correction only, and (iv) multiple-charge correction and size calibration.
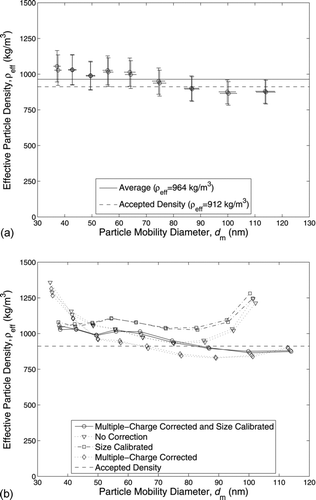
The multiple-charge correction and size calibration changed the effective density by a maximum of −29.2% compared to the uncorrected effective density (calculated from the uncorrected mDMS CMD). The magnitude of each correction is shown in . The size calibration (shown in the online supplemental information) showed the modified DMS oversized the particles from 50 to 100 nm and undersized the particles otherwise. Therefore, the size calibration either increased or decreased the measured effective density depending on the particle mobility size. However, the multiple-charge correction removed current from the lower rings (or smaller particle sizes), making the corrected CMD higher and the effective density lower over the entire mobility range. Compared to the uncorrected effective density, the size calibration caused a maximum change in effective density of –20.5%, while the multiple-charge correction caused a maximum change in effective density of –27.5%. Therefore, both the multiple-charge correction and size calibration are required to accurately operate a CPMA–mDMS system.
The Gopalakrishnan et al. bipolar charging model was also applied within the correction process, rather than Wiedensohler’s model, to estimate the effect of the bipolar charging model on the data inversion. This variation of the correction process, applied to the same data described earlier, calculated an average DEHS effective density of 997 kg/m or within 9.3% of the accepted value. This value is still within error of the accepted value and is within 3.4% of the average effective density generated using the Wiedensohler model. A plot comparing these results is shown in the online supplemental information.
DEHS results were also collected using a CPMA resolution of 5 to estimate the effect of different CPMA resolutions on the data inversion. This data measured an average DEHS effective density of 979 kg/m or within 7.3% of the accepted value. This value is also within error of the accepted value and is within 1.5% of the average effective density measured using a CPMA resolution of 3. A plot comparing these results is shown in the online supplemental information and demonstrates that a range of CPMA resolutions can be used within the CPMA–mDMS system.
4.3. CPMA–Standard DMS Results
The effective density measured by the CPMA–sDMS system is shown in Figure 4a. The CPMA resolution was 10. The full charging model was used (DMS model 1), which considers the entire change distribution for each particle size leaving the DMS corona charger. The Wiedensohler bipolar and Fuch's unipolar charging models were used and DMS ring currents generated by unchanged particles that pass through the CPMA were manually removed (Method 1). The averaged measured effective density was 1027 kg/m or within 12.6% of the accepted value. The error bars represent the instrument bias uncertainty and is explained further in the online supplemental information. Applying a 95% confidence interval, the precision uncertainty of the CPMA–sDMS was 41 kg/m
or 4.5% of the accepted value, which is insignificant compared to the bias uncertainty of 30.1%. Therefore, the total uncertainty in the measured effective density is approximately 30.1%. also shows the presence of a systematic error with a nearly constant offset from the accepted value.
FIG. 4 (a) Measured effective density of DEHS using CPMA with a resolution of 10 and standard DMS system, applying the Wiedensohler bipolar and Fuchs unipolar charging models within the correction process. (b) Comparison of DEHS effective density values calculated from the data described in (a) with: (i) no correction, (ii) size calibration only, (iii) multiple-charge correction only, and (iv) multiple-charge correction and size calibration.
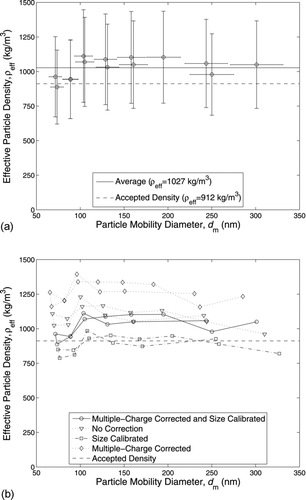
FIG. 5 DEHS-measured effective density using all three standard DMS models where the current generated by uncharged particles was manually removed for all cases, a CPMA resolution of 10 was used, and Wiedensohler bipolar and Fuchs unipolar charging models were applied within the correction process.
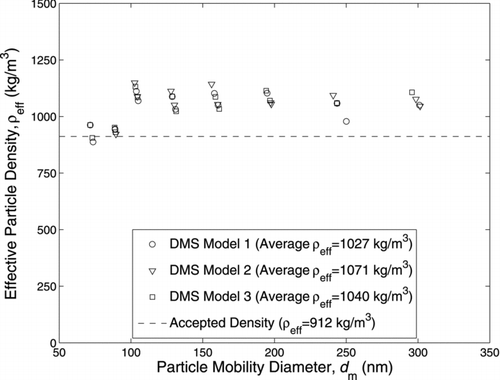
The multiple-charge correction and size calibration changed the effective density by a maximum of −13.3% compared to the uncorrected effective density (calculated from the uncorrected sDMS CMD). The magnitude of this correction is misleading, as the multiple-charge correction and size calibration were counteracting each other, as shown in . The standard DMS size calibration (shown in the online supplemental information) makes the corrected CMD higher (and the effective density lower), while the multiple-charge correction removed current from the upper rings (or larger particle sizes), making the corrected CMD lower (and the effective density higher). Compared to the uncorrected effective density, the size calibration caused a maximum change in effective density of −23.3%, while the multiple-charge correction caused a maximum change in effective density of +28.4%. Therefore, both the multiple-charge correction and size calibration are not small enough to neglect and are required to accurately operate a CPMA–sDMS system.
The White unipolar charging model was also applied within the correction process, rather than Fuchs’ model, to estimate the effect of the unipolar charging model on the data inversion. This variation of the correction process, applied to the same data described above, calculated an average DEHS effective density of 1033 kg/m or within 13.3% of the accepted value. This value is still within error of the accepted value and is within 0.6% of the average effective density generated using the Fuchs, model. A plot comparing these results is shown in the online supplemental information.
DEHS results were also collected using a CPMA resolution of 5 to estimate the effect of different CPMA resolutions on the data inversion. These data measured an average DEHS effective density of 1028 kg/m or within 12.7% of the accepted value. This value is also within error of the accepted value and is within 0.05% of the average effective density measured using a CPMA resolution of 10. A plot comparing these results is shown in the online supplemental information and demonstrates that a range of CPMA resolutions can be used within the CPMA-sDMS system.
4.3.1. Average vs. Full Charging Models
For the CPMA–sDMS system, the average charging model (DMS Model 2) only considers the mean charge for each particle mobility size leaving the DMS corona charger, rather than the entire charge distribution (DMS Model 1 or 3). DMS Model 1 applies the charge probabilities to each CPMA-classified mobility size concentration, while DMS Model 3 applies the diffusive DMS model, based on the charging probabilities. The effects of these assumptions are shown in where for all of the cases the current generated by uncharged particles was manually removed, a CPMA resolution of 10 was used, and Wiedensohler bipolar and Fuchs unipolar charging models were applied within the correction process. The effective densities found using the average charging model (DMS Model 2) disagreed on average by 4.3% with the values found using DMS Model 1. However, DMS Model 2 only generated 12 valid data points out of 16 inputs, where the theoretical and measured DMS ring currents agreed within a set tolerance of 5%, compared to 14 valid data points generated by DMS Model 1. The effective densities found using DMS Model 3 disagreed on average by 1.3% with the values found using DMS Model 1. DMS Model 3 generated 15 valid data points out of 16 inputs. On average, the time to apply the correction per point per iteration took 526 s for DMS Model 1, 323 s for DMS Model 2, and 548 s for DMS Model 3. Due to the nature of Fuchs’ unipolar model (solving differential equations), DMS model 3 takes longer to calculate than DMS model 1; however, if White’s unipolar model (which has an analytical solution) is applied instead, DMS model 3 becomes faster than DMS model 1.
4.3.2. Automatic vs. Manual Uncharged Particle Removal
For the standard DMS, the effects of calculating and removing the current generated by the uncharged particles using the CPMA diffusive transfer function versus manually removing it are shown in using DMS Model 1, a CPMA resolution of 10, and Wiedensohler bipolar and Fuchs unipolar charging models within the correction process for both cases. The effective densities found using the uncharged model disagreed on average by 1.6% with the values found by manually removing the current generated by uncharged particles. This disagreement is insignificant compared to the system uncertainty of 31%. The results that had the current generated by uncharged particles manually removed took on average 526 s per point per iteration to calculate, while removing the current generated by uncharged particles through the model took on average 1394 s per point per iteration. This large discrepancy is due to the number of points calculated in each CPMA model. The CPMA uncharged particle model calculates the transfer function at each particle mass in the mobility range (approximately 500 points); however, the CPMA charged particle model calculates the transfer function in steps that are centered about the CPMA mass-to-charge setpoint (approximately 200 points). Therefore, to decrease computation time, the current generated by uncharged particles can be manually removed. However, the uncharged particle model should still be used when the current generated by the uncharged particles versus the classified particles is indistinguishable (i.e., measured on common DMS rings).
5. CONCLUSIONS AND SUMMARY
Two different CPMA–DMS systems and the multiple-charge correction method were validated by measuring the effective density of DEHS. DEHS was used as it has a known and size-independent density of 912 kg/m. The CPMA–mDMS system measured an effective density of 964 kg/m
or within 5.7% of the accepted value, while the CPMA–sDMS system measured 1027 kg/m
or within 12.6% difference of the accepted value. It was found that both the multiple-charge correction and size calibration were significant, each causing a maximum change in effective density greater than 10%, for either system. Therefore, both the multiple-charge correction and size calibration must be applied to obtain accurate results from the CPMA–mDMS or CPMA–sDMS system. The uncertainty in effective density, assuming a 95% confidence interval, was 9.5–10.4% for the CPMA–mDMS system depending on particle mobility size and 30.1% for the CPMA–sDMS system. While these uncertainties are higher than previously described mass–mobility measurement techniques, a CPMA–DMS system can measure transient sources at one particle mass or determine a complete aerosol density distribution in minutes from steady state sources.
CPMA-DMS_Paper_SI.zip
Download Zip (136.9 KB)Acknowledgments
This research would not have been possible without the support from Cambustion, GARDN, MDS Aero Support Corporation, NSERC, and Alberta Innovates–Technology Futures.
[Supplementary materials are available for this article. Go to the publisher’s online edition of Aerosol Science and Technology to view the free supplementary files.]
Notes
This equation is purely empirical and does not apply to the mass-mobilities of all nonspherical particles, such as in the transition regime (slip regime effects) as outlined by Sorensen (Citation2011).
The commercial DMS500 is empirically calibrated with aerosols of a known size and concentration.
From Equation (Equation2), , where u is the uncertainty.
REFERENCES
- Ahlvik , P. , Ntziachristos , L. , Keskinen , J. and Virtanen , A. 1998 . Real Time Measurements of Diesel Particle Size Distribution with an Electrical Low Pressure Impactor . Technical Report SAE 980410
- Barone , T. L. , Lall , A. A. , Storey , J. M. E. , Mulholland , G. W. , Prikhodko , V. Y. Frankland , J. H. 2011 . Size-Resolved Density Measurements of Particle Emissions from an Advanced Combustion Diesel Engine: Effect of Aggregate Morphology . Energy & Fuels , 25 ( 5 ) : 1978 – 1988 .
- Biskos , G. , Reavell , K. and Collings , N. 2005 . Description and Theoretical Analysis of a Differential Mobility Spectrometer . Aerosol Sci. Technol. , 39 ( 6 ) : 527 – 541 .
- Cambustion . 2011 . DMS500 Fast Particulate Spectrometer with Heated Sample Line High Ratio Diluter User Manual 3.5 ed.
- Cross , E. S. , Onasch , T. B. , Ahern , A. , Wrobel , W. , Slowik , J. G. Olfert , J. 2010 . Soot Particle Studies Instrument Inter-Comparison Project Overview . Aerosol Sci. Technol. , 44 ( 8 ) : 592 – 611 .
- DeCarlo , P. , Slowik , J. , Worsnop , D. , Davidovits , P. and Jimenez , J. 2004 . Particle Morphology and Density Characterization by Combined Mobility and Aerodynamic Diameter Measurements. Part 1: Theory . Aerosol Sci. Technol. , 38 ( 12 ) : 1185 – 1205 .
- Eggersdorfer , M. , Grohn , A. , Sorensen , C. , McMurry , P. and Pratsinis , S. 2012 . Mass–Mobility Characterization of Flame-Made Zro2 Aerosols: Primary Particle Diameter and Extent of Aggregation . J. Colloid Interface Sci. , 387 ( 1 ) : 12 – 23 .
- Ehara , K. , Hagwood , C. and Coakley , K. 1996 . Novel Method to Classify Aerosol Particles According to Their Mass-to-Charge Ratio–Aerosol Particle Mass Analyser . J. Aerosol Sci. , 27 ( 2 ) : 217 – 234 .
- Emery , M. S. 2005 . “ Theoretical Analysis of Data from DMA–APM System ” . USA : Master's thesis, Particle Technology Laboratory., Department of Mechanical Engineering, University of Minnesota .
- Fernandez de la Mora , J. , De Juan , L. , Liedtke , K. and Schmidt-Ott , A. 2003 . Mass and Size Determination of Nanometer Particles by Means of Mobility Analysis and Focused Impaction . J. Aerosol Sci. , 34 ( 1 ) : 79 – 98 .
- Forster , P. , Ramaswamy , V. , Artaxo , P. , Berntsen , T. , Betts , R. Fahey , D. 2007 . Climate Change 2007: The Physical Science Basis , Cambridge , UK : Cambridge University Press . Chapter 2
- Fuchs , N. 1963 . On the Stationary Charge Distribution on Aerosol Particles in a Bipolar Ionic Atmosphere . Geofis. Pura Appl. , 56 ( 1 ) : 185 – 193 .
- Ghazi , R. , Tjong , H. , Soewono , A. , Rogak , S. N. and Olfert , J. S. 2013 . Mass, Mobility, Volatility, and Morphology of Soot Particles Generated by a Mckenna and Inverted Burner . Aerosol Sci. Technol. , 47 ( 4 ) : 395 – 405 .
- Gopalakrishnan , R. , Meredith , M. J. , Larriba-Andaluz , C. and Hogan , C. J. H. Jr. 2013 . Brownian Dynamics Determination of the Bipolar Steady State Charge Distribution on Spheres and Non-Spheres in the Transition Regime . J. Aerosol Sci., , 63 : 126 – 145 .
- Gunn , R. and Woessner , R. 1956 . Measurements of the Systematic Electrification of Aerosols . J. Colloid Sci. , 11 ( 3 ) : 254 – 259 .
- Gysel , M. , Laborde , M. , Olfert , J. S. , Subramanian , R. and Grohn , A. J. 2011 . Effective Density of Aquadag and Fullerene Soot Black Carbon Reference Materials Used for sp2 Calibration . Atmos. Meas. Tech. , 4 ( 12 ) : 2851 – 2858 .
- Haynes , W. M. 2012–2013 . CRC Handbook of Chemistry and Physics , Boca Raton , FL : CRC Press/Taylor and Francis . 93rd ed. Internet Vers. 2013 (Accessed May 29, 2013)
- Hinds , W. C. 1999 . Aerosol Technology- Properties, Behaviour and Measurement of Airborne Particles. , 2nd ed. , John Wiley and Sons .
- ISO . 2002 . Heavy-Duty Engines—Measurement of Gaseous Emissions from Raw Exhaust Gas and of Particulate Emissions Using Partial Flow Dilution Systems Under Transient Test Conditions . ISO16183
- Jacobson , M. 2001 . Strong Radiative Heating Due to the Mixing State of Black Carbon in Atmospheric Aerosols . Nature , 409 ( 6821 ) : 695 – 697 .
- Jayne , J. , Leard , D. , Zhang , X. , Davidovits , P. , Smith , K. Kolb , C. 2000 . Development of an Aerosol Mass Spectrometer for Size and Composition Analysis of Submicron Particles . Aerosol Sci. Technol. , 33 ( 1–2 ) : 49 – 70. .
- Keskinen , J. , Pietarinen , K. and Lehtimaki , M. 1992 . Electrical Low-Pressure Impactor . J. Aerosol Sci. , 23 ( 4 ) : 353 – 360 .
- Kim , S. , Mulholland , G. and Zachariah , M. 2009a . Density Measurement of Size Selected Multiwalled Carbon Nanotubes by Mobility–Mass Characterization . Carbon , 47 ( 5 ) : 1297 – 1302 .
- Kim , S. C. , Wang , J. , Shin , W. G. , Scheckman , J. H. and Pui , D. Y. H. 2009b . Structural Properties and Filter Loading Characteristics of Soot Agglomerates . Aerosol Sci. Technol. , 43 ( 10 ) : 1033 – 1041 .
- Ku , B. K. , Emery , M. S. , Maynard , A. D. , Stolzenburg , M. R. and McMurry , P. H. 2006 . In Situ Structure Characterization of Airborne Carbon Nanofibres by a Tandem Mobility–Mass Analysis . Nanotechnology , 17 ( 14 ) : 3613 – 3621 .
- Larriba , C. , Hogan , C. J. Jr. , Attoui , M. , Borrajo , R. , Garcia , J. F. and de la Mora , J. F. 2011 . The Mobility–Volume Relationship below 3.0 nm Examined by Tandem Mobility-Mass Measurement . Aerosol Sci. Technol. , 45 ( 4 ) : 453 – 467 .
- Lee , S. Y. , Chang , H. , Ogi , T. , Iskandar , F. and Okuyama , K. 2011 . Measuring the Effective Density, Porosity, and Refractive Index of Carbonaceous Particles by Tandem Aerosol Techniques . Carbon , 49 ( 7 ) : 2163 – 2172 .
- Lee , S. Y. , Widiyastuti , W. , Tajima , N. , Iskandar , F. and Okuyama , K. 2009 . Measurement of the Effective Density of Both Spherical Aggregated and Ordered Porous Aerosol Particles Using Mobility- and Mass-Analyzers . Aerosol Sci. Technol. , 43 ( 2 ) : 136 – 144 .
- Liu , Z. , Swanson , J. , Kittelson , D. B. and Pui , D. Y. H. 2012 . Comparison of Methods for Online Measurement of Diesel Particulate Matter . Environ. Sci. Technol. , 46 ( 11 ) : 6127 – 6133 .
- Malloy , Q. G. J. , Nakao , S. , Qi , L. , Austin , R. , Stothers , C. Hagino , H. 2009 . Real-Time Aerosol Density Determination Utilizing a Modified Scanning Mobility Particle Sizer-Aerosol Particle Mass Analyzer System . Aerosol Sci. Technol. , 43 ( 7 ) : 673 – 678 .
- Maricq , M. and Xu , N. 2004 . The Effective Density and Fractal Dimension of Soot Particles from Premixed Flames and Motor Vehicle Exhaust . J. Aerosol Sci. , 35 ( 10 ) : 1251 – 1274 .
- McMurry , P. , Wang , X. , Park , K. and Ehara , K. 2002 . The Relationship Between Mass and Mobility for Atmospheric Particles: A New Technique for Measuring Particle Density . Aerosol Sci. Technol. , 36 ( 2 ) : 227 – 238 .
- Olfert , J. and Collings , N. 2005 . New Method for Particle Mass Classification—The Couette Centrifugal Particle Mass Analyzer . J. Aerosol Sci. , 36 ( 11 ) : 1338 – 1352 .
- Olfert , J. S. , Reavell , K. S. , Rushton , M. G. and Collings , N. 2006 . The Experimental Transfer Function of the Couette Centrifugal Particle Mass Analyzer . J. Aerosol Sci. , 37 ( 12 ) : 1840 – 1852 .
- Olfert , J. S. , Symonds , J. P. R. and Collings , N. 2007 . The Effective Density and Fractal Dimension of Particles Emitted from a Light-Duty Diesel Vehicle with a Diesel Oxidation Catalyst . J. Aerosol Sci. , 38 ( 1 ) : 69 – 82 .
- Park , K. , Cao , F. , Kittelson , D. B. and McMurry , P. H. 2003a . Relationship Between Particle Mass and Mobility for Diesel Exhaust Particles . Environmental Science & Technology , 37 ( 3 ) : 577 – 583 .
- Park , K. , Kittelson , D. B. and McMurry , P. H. 2003b . A Closure Study of Aerosol Mass Concentration Measurements: Comparison of Values Obtained with Filters and by Direct Measurements of Mass Distributions . Atmos. Environ. , 37 : 1223 – 1230 .
- Pope , C. A. 2000 . Review: Epidemiological Basis for Particulate Air Pollution Health Standards . Aerosol Sci. Technol. , 32 ( 1 ) : 4 – 14 .
- Reavell , K. , Hands , T. and Collings , N. 2002 . A Fast Response Particulate Spectrometer for Combustion Aerosols . Technical Report SAE 2002-01-2714
- Rissler , J. , Swietlicki , E. , Bengtsson , A. , Boman , C. , Pagels , J. Sandstrom , T. 2012 . Experimental Determination of Deposition of Diesel Exhaust Particles in the Human Respiratory Tract . J. Aerosol Sci. , 48 : 18 – 33 .
- Ristimaki , J. , Virtanen , A. , Marjamaki , M. , Rostedt , A. and Keskinen , J. 2002 . On-Line Measurement of Size Distribution and Effective Density of Submicron Aerosol Particles . J. Aerosol Sci. , 33 ( 11 ) : 1541 – 1557 .
- SAE . 2010 . Aircraft Exhaust Nonvolatile Particle Matter Measurement Method Development . AIR6037
- Scheckman , J. H. and McMurry , P. H. 2011 . Deposition of Silica Agglomerates in a Cast of Human Lung Airways: Enhancement Relative to Spheres of Equal Mobility and Aerodynamic Diameter . J. Aerosol Sci. , 42 ( 8 ) : 508 – 516 .
- Scheckman , J. H. , McMurry , P. H. and Pratsinis , S. E. 2009 . Rapid Characterization of Agglomerate Aerosols by In Situ Mass–Mobility Measurements . Langmuir , 25 ( 14 ) : 8248 – 8254 .
- Shin , W. G. , Mulholland , G. W. , Kim , S. C. , Wang , J. , Emery , M. S. and Pui , D. Y. H. 2009 . Friction Coefficient and Mass of Silver Agglomerates in the Transition Regime . J. Aerosol Sci. , 40 ( 7 ) : 573 – 587 .
- Sorensen , C. 2011 . The Mobility of Fractal Aggregates: A Review . Aerosol Sci. Technol. , 45 ( 7 ) : 755 – 769 .
- Symonds , J. P. R. 2010 . Calibration of Fast Response Differential Mobility Spectrometers , London , , UK : Metrology of Airborne Nanoparticles, Standardisation and Applications (MANSA), National Physical Laboratory (NPL) . June 8–9, 2010
- Symonds , J. P. R. and Reavell , K. 2007 . Calibration of a Differential Mobility Spectrometer European Aerosol Conference, Salzburg, Austria, September 9–14, 2007
- Symonds , J. P. R. , Reavell , K. S. and Olfert , J. S. 2013 . The CPMA– Electrometer System a Suspended Particle Mass Concentration Standard . Aerosol Sci. Technol., , 47 ( 8 ) : i – iv .
- Symonds , J. P. R. , Reavell , K. S. J. , Olfert , J. S. , Campbell , B. W. and Swift , S. J. 2007 . Diesel Soot Mass Calculation in Real-Time with a Differential Mobility Spectrometer . J. Aerosol Sci. , 38 ( 1 ) : 52 – 68 .
- Tajima , N. , Fukushima , N. , Ehara , K. and Sakurai , H. 2011 . Mass Range and Optimized Operation of the Aerosol Particle Mass Analyzer . Aerosol Sci. Technol. , 45 ( 2 ) : 196 – 214 .
- Virtanen , A. , Ristimaki , J. and Keskinen , J. 2004 . Method for Measuring Effective Density and Fractal Dimension of Aerosol Agglomerates . Aerosol Sci. Technol. , 38 ( 5 ) : 437 – 446 .
- Wang , S. and Flagan , R. C. 1990 . Scanning Electrical Mobility Spectrometer . Aerosol Sci. Technol. , 13 ( 2 ) : 230 – 240 .
- White , H. J. 1951 . Particle Charging in Electrostatic Precipitation . Trans. Am. Inst. Electr. Eng. , 70 ( 2 ) : 1186 – 1191 .
- Wiedensohler , A. 1988 . An Approximation of the Bipolar Charge Distribution for Particles in the Submicron Size Range . J. Aerosol Sci. , 19 : 387 – 389 .
- Xue , H. , Khalizov , A. F. , Wang , L. , Zheng , J. and Zhang , R. 2009 . Effects of Coating of Dicarboxylic Acids on the Mass-Mobility Relationship of Soot Particles . Environ. Sci. Technol. , 43 ( 8 ) : 2787 – 2792 .