Abstract
Airborne particulate matter samples were collected in an urban and a rural–suburban monitoring stations of the city of Rome, Italy, and the particles were analyzed through the Raman microspectroscopy. A careful examination of the spectral bands, performed with a five-(Voigt) curve deconvolution model previously described by the literature and here adapted to the purpose, lead to the characterization of the graphitic and carbonaceous material plus the identification of the mineral particles associated with it. Statistical analysis of the full-width at half-maximum (FWHM) values of the bands, as well as of their intensity ratio, revealed the presence of two classes of soot particles that can be ascribed to a different degree of crystallinity. The population of soot collected at the urban site, where the vehicular emission component prevails, exhibits mostly crystalline characteristics (with a D1 FWHM of 150–155 cm−1), whereas the population collected at the rural–suburban site, particularly the coarse fraction, shows a prevailing amorphous nature (with a D1 FWHM of ∼175 cm−1). A similar aspect emerges for the pure black carbon particles, mainly crystalline, and the black carbon particles associated with minerals, generally disordered. These results add useful information and characterization of the soot, a relevant component of the ambient air, and its different features with respect to the urban or rural–suburban areas.
Copyright 2014 American Association for Aerosol Research
1. INTRODUCTION
Soot is widely regarded as one of the most important air pollutants because of its occurrence in all urban areas and its impact on the environment, climate, and human health. Several authors have pointed out that the inhalation of particulate matter (PM) can cause acute and chronic toxicological effects, such as respiratory inflammations or lung disease on exposed people (Brunekreef and Forseberg Citation2005; Davidson et al. Citation2005; Englert Citation2005), and similar results are also shown by toxicological studies (Gualtieri et al. Citation2009, Citation2010). Therefore, due to its high content in the fine fraction and the ability to penetrate deeper in the lungs, soot particles are likely one of the most toxic components of ambient aerosol (Zerbi et al. Citation2008). Besides, the observed effects on the health are also correlated to the soot properties and composition, in particular to the presence of trace metals (Lee et al. Citation2006; Grobéty et al. Citation2010), minerals (Gieré et al. Citation2006), and organic compounds (Sakurai et al. Citation2003), that are responsible of oxidative stress on biological systems (Sørensen et al. Citation2003; Risom et al. Citation2005; Gualtieri et al. Citation2009). Soot—sometimes referred to as “black carbon” (BC) because of its optical properties (Mertes et al. Citation2004)—is usually defined as the product of incomplete combustion of organic matter (e.g., vehicular traffic, heating systems, industries, fossil-fueled power plants, incinerators), although it can also be generated by combustion of biomasses or woods and, in domestic ambients, by cooking. Owing to the variability of these processes, soot composition is given by a mix of graphitic carbon (or elemental carbon—EC), amorphous carbon, graphenes, and other organic compounds, such as hydrocarbons, aromatics, alkanes, fullerenes, and polyenes (Sadezky et al. Citation2005).
A different and important field of research concerns the role of soot in the regulation of climate and environmental processes (Andreae and Gelencsér 2006). Therefore, the understanding of the properties of air-dispersed soot in the atmosphere is important for recognizing the sources and evaluating the different impact related to its properties. For this aim, the classical approach to the airborne PM analysis (gas chromatography-mass spectrometry for organics and scanning electron microscopy and energy dispersive spectrometry [SEM-EDS] for minerals and trace metals) is not sufficient to obtain detailed information about soot properties and consequently the genetic processes. Differently, transmission electron microscopy (TEM) analyses are particularly suitable to characterize the properties of soot (Buseck et al. Citation1987; Posfái et al. Citation1999; Mathis et al. Citation2004; Chen et al. Citation2005). Many authors reported how the use of high-resolution transmission electron microscopy (HRTEM) images can highlight the presence of graphitic or amorphous components (Adachi and Buseck Citation2008), and the different structural frame of graphitic layers in the BC, related to different combustion processes such as pyrolysis of coal or combustion of liquid fuels (Su et al. Citation2004; Chen et al. Citation2005). This approach is very efficient, but it has the disadvantage of being hardly suitable for systematic and quantitative studies of particles because of the difficulty of analyzing large numbers of particles and quantifying the graphitic and amorphous components in soot aggregates. Moreover, TEM is particularly useful for analyzing small and thin particles that constitute soot aggregates but may be ineffective when trying to have information about the coarse soot aggregates.
A complementary technique useful for this field of study is the Raman microspectroscopy, employed for the characterization of graphitic materials (Sadezky et al. Citation2005) and soot particles (Blaha et al. Citation1978; Sze et al. Citation2001; Ivleva et al. Citation2007a), for the reason that the honeycomb carbon structure produces a typical vibrational spectrum that allows to recognizing crystalline graphite, ordered, disordered and amorphous carbon, as well as organic matter. Using Raman micro-spectroscopy it is possible to analyze single particles aggregates (Batonneau et al. Citation2006; Ivleva et al. Citation2007b; Stefaniak et al. Citation2009), collecting a spectrum from each one, and then performing a statistical analysis. This approach has been applied to a case study of the city of Rome, where samples of PM2.5 and PM10 were collected in an urban and a suburban–rural site, originally with the aim of assessing the crustal (mineral) component during some episodes of Saharan dust outbreaks over Italy (Matassoni et al. Citation2011). Those studies employed SEM-EDS and particle-induced X-ray emission (PIXE) techniques and here Raman microspectroscopy has been used to show the differences of soot's features at different sites, with the aim of identifying different soot components and possibly their sources.
2. EXPERIMENTAL SECTION
2.1. Particulate Sampling
The sampling program was performed with the collaboration of ISPRA (Istituto Superiore per la Protezione e la Ricerca Ambientale) and ARPA (Agenzia Regionale per la Protezione Ambientale) Lazio. Two sites were chosen for PM collection, both in the municipality of Rome: the urban sampling site at Corso Francia (CF), in the north outskirt, and the suburban–rural site at Castel Romano (CR), in the south-west countryside. The two sites are distant from each other about 27 km. In these sampling sites, both PM10 and PM2.5 were simultaneously collected, using a sampler Tecora Charlie at CR, and a Tecora Skypost PM at CF. The period of sampling we chose for this study lasted 10 days, from 26 February to 7 March 2009 and includes an episode of Saharan dust outbreaks in Italy. The sample collection was originally performed, over a wider length of time, with the early aim of accomplishing a SEM-EDS characterization of single particles, and PIXE studies, for assessing the Saharan dust contribution to PM10, PM2.5, and PM1 in urban and suburban areas of Rome (Matassoni et al. Citation2011, Citation2012), thus optimizing the parameters of the collection to this purpose.
Airborne PM was collected on Millipore polycarbonate filters with diameter of 47 mm and pore size of 0.8 μm. The sampling conditions were adjusted for collecting the optimal amount of PM on the filter (about 30–50 μg/cm2) for SEM analyses as described by the literature (Mamane et al. Citation2001; Casuccio et al. Citation2002). The air flux rate and sampling time, for both PM10 and PM2.5, were calculated using the mean value of particulate concentration at the two sites: 1 m3/h (0.28 l/s) for a total time of 192 min per day at CF, and 2.3 m3/h (0.64 l/s) for a total time of 240 min per day at CR. To get a good representativeness of the daily composition, the sampling was performed for 8 and 10 min each hour, respectively.
2.2. Micro-Raman Analyses
Raman analyses were carried out using a confocal Raman microprobe (Horiba Jobin-Yvon LabRam-IR) coupled with an optical microscope. The Raman device was equipped with a HeNe laser source (λ0 = 632.8 nm), a monochromator with holographic notch filter, a spectrometer with diffraction grating of 1800 g/mm, and a Peltier cooled CCD detector (1024 × 256 pixels). The laser beam power at the source was 20 mW and filters, with an attenuation factor from 1 to 10−4 of the source power, were used. A confocal hole and a slit were employed to regulate the radiation flux in the objective and to the spectrometer, respectively. The coupled optical microscope was equipped with three objectives with 10× (NA 0.25), 50× (NA 0.7), and 100× (NA 0.9) magnification and an eyepiece of 10× magnification. The diameter of the laser spot on the sample surface was 1 μm for the fully focused laser beam at 100× objective magnification. The spectral resolution was 1 cm−1 and the instrument was calibrated against the Stokes Raman signal of pure Si at 520 cm−1 using a silicon wafer. Instrument control and data acquisition, as well as the processing and analysis of Raman spectra, were performed with the HORIBA Scientific's LabSpec 5 software (http://www.horiba.com/scientific).
The aerosol samples were collected on polycarbonate filters, but it was not possible to obtain any useful Raman spectra from the particles because of the high interference of the polycarbonate material; therefore, it was necessary to remove all the particles from the filter. The operation has been done by carefully washing a portion of the filter (5 × 5 mm) in a hollow cylinder applied on a steel support and filled with 2 ml of isopropanol, letting the PM sediment at the bottom and, once the liquid was fully evaporated (at the temperature of 20°C), removing the cylinder. A steel support has been preferred, because it does not produce any Raman signal and well reduces sample heating (Sadezky et al. Citation2005; Ivleva et al. Citation2007a). For each sample, the Raman spectra of 40 particles have been collected: these particles have been chosen starting from a point in the support and selecting the first 10 particles in the four directions. Among the PM10 samples, only those particles with a diameter included in the range of 2.5–10 μm were selected, to assess a fraction complementary to the PM2.5 fraction, whereas for the latter only those particles with a diameter higher or equal to 1 μm were selected. The Raman spectra, in the region of 800–1800 cm−1, have been acquired from soot particles (whole soot or soot associated with other phases), in addition to several spectra of mineral particles. The optimal signal-to-noise ratio spectra were collected with the objective of 100× magnification, slit and hole aperture of 200 μm, grating of 1800 g/mm, integration time of 10 seconds with 4 accumulated spectra, and the use of filters for reducing the laser beam intensity. The filters analyzed were two (PM10 and PM2.5) for each of the 10 days of sampling, for two sampling sites (CF and CR): that is 40 samples for a total of 1527 particles successfully analyzed. Analyses have been carried out also on particles selected from the standard NIST SRM 1650b diesel exhaust PM as to allow a direct comparison with the literature data. Spectra that showed changes during the measurements were excluded, and second-order bands were not analyzed in this work.
3. THEORETICAL BASES
3.1. Deconvolution Model
Models commonly used in spectral analysis of graphitic and carbonaceous materials exploit integrated areas and peak heights of spectra (Tommasini et al. Citation2011), or use a deconvolution process with different combinations of curves (Sze et al. Citation2001). In this work, we employed the five-curve deconvolution model, proposed by Sadezky et al. (Citation2005), that has been proved to be suitable for analysis of Raman spectra of the soot particles as it yields a reproducible characterization (Ivleva et al. Citation2007a). Soot spectra generally display two wide and overlapping peaks with Raman shift values of about 1350 and 1600 cm−1, called D and G band, respectively. However, according to the model of Sadezky et al. (Citation2005) the peaks are actually the convolution of five bands named G, D1, D2, D3, and D4, as it is shown in for a case of our study.
FIG. 1 Example of band deconvolution, with linear background removal, of the Raman spectrum (λ0 = 632.8 nm) of a particle of soot, as we applied for a case of analysis in this work (PM2.5 from CF). In the bottom, the residuals’ curve.
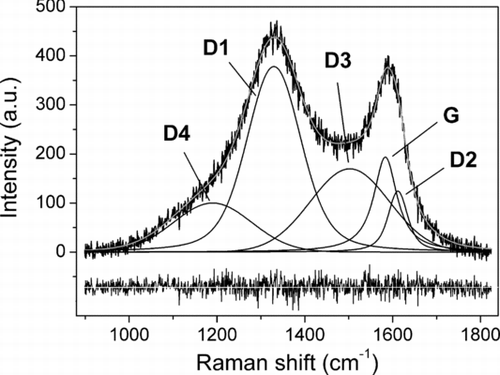
G band stands for “graphitic band,”, being typical of ordered graphitic materials: it is generated by the combination of bending and stretching vibrational modes of carbon atoms in the honeycomb structure (hexagonal frame of sp2 C atoms) with E2g symmetry. Graphite crystals exhibit only this band whose Raman shift value is ∼1580 cm−1. D1 band, observed at ∼1320 cm−1, stands for “disorder band” and is due to the breathing mode of hexagonal carbon atoms honeycomb framework. This vibrational mode is normally forbidden by Raman selection rules in ordered graphite and the rising of this band is strongly related to the presence of defective structures that yields a change in the atomic symmetry from E2g to A1g (Wang et al. Citation1990; Castiglioni and Tommasini Citation2007). Soot particles are characterized by such a defective structure because their nucleation and growth takes place in disequilibrium conditions (Sadezky et al. Citation2005). The band called D2 (formerly D’), partially overlapped with G band, is placed at ∼1620 cm−1. This band arises from the same vibrational modes of G band, but it is influenced by the presence of incomplete graphitic structure, at the borders of crystallites, that stretches C–C bonds producing a locally distorted symmetry E’2g, and its intensity is related to the size of crystallites of soot particles (Sadezky et al. Citation2005; Tommasini et al. Citation2011). The D3 band (also known as A band) occurs at ∼1500 cm−1 and is related to the presence of amorphous carbon and organic molecules, whereas the D4 band (also called I band), located at ∼1200 cm−1, is linked to the presence of impurities in the BC such as ions, metals, and polyenes (organic molecules with C=C bonds) (Sadezky et al. Citation2005).
3.2. Data Processing
The deconvolution of the collected Raman spectra were performed using Fityk, a software for nonlinear curve fitting and data analysis (Wojdyr Citation2010) applying the least-squares-based iterative algorithm Levenberg–Marquardt to optimize the parameters of the functions used in the modeling of the peaks. The fitting model of Sadezky et al. (Citation2005) makes use of four Lorentzian curves (for G, D1, D2, and D4 bands) and a Gaussian (for D3 band) since their combination produces the better fit of carbonaceous materials and soot spectra. In this work, five Voigt functions have been used for the fitting procedure of the experimental Raman spectra; in fact, according to the studies of Zanatta and Ferri (Citation2007), the Voigt function corresponds to the convolution of the phonon mode (Lorentzian profile) with the resolution of the optical setup (Gaussian profile). Moreover, as reported by Gołabczak and Konstantynowicz (Citation2009), Raman peaks of crystalline carbonaceous materials exhibit a Lorentzian profile while structural disorder produces a peak broadening with Gaussian profile. The choice of the band deconvolution procedure employing only Voigt functions was made on the basis of sound foundation, higher accuracy, and reproducibility of the fit, verified by a set of trials on soot and standard material spectra as explained below.
In our analysis, we excluded those spectra with intensity-of-the-peak/background ratio not sufficient for modeling and those that showed the presence of iron oxides. Such mineral phases generate a broadband in the range of D1 band, and the fitting procedure of these spectra fails due to the presence of too many parameters to optimize. The Raman spectra were analyzed by first removing the background contribution using a linear function, then employing a common starting file with five Voigt peaks having parameters as those reported by Sadezky et al. (Citation2005). The following peak parameters were optimized: height, center, Gaussian FWHM, and shape (i.e., ratio of Lorentzian and Gaussian widths). The first stage of the modeling let the peaks vary all the parameters, except for the position that was optimized afterward. This procedure allowed us to get a more reproducible process of peak modeling that always reached convergence and yielded, in all cases, an R-squared higher than 95%. To assessing the reliability of the scheme tests on a collected Raman spectrum were performed applying both the five-Voigt curve model and the one reported by Sadezky et al. (Citation2005). Average values of the center and FWHM of the peaks, as well as the D/G ratio, with their standard deviations, were calculated for a selected spectrum (Table S1). Ten iterative process of deconvolution has been performed by starting from the same peaks position and varying the following parameters: height, FWHM, and shape. The modeling of the pattern employing five Voigt curves was applied also on the spectra obtained from the particles of the standard NIST SRM 1650b diesel exhaust PM and the results are shown Table S2.
The main advantage of using five Voigt curves with respect to the method proposed by Sadezky et al. (Citation2005) is that the band deconvolution process needs less constraints to reach a good curve fitting. In fact, using a starting model for the fitting procedure with five Voigt curves, only the initial positions and “shape” parameters (i.e., the Lorentzian and Gaussian contributions) are necessary. Adopting the Sadezky's method it is necessary constraining the peaks positions in the first stages of the fitting. In particular, the Gaussian D3 band frequently shifts to a much higher or lower wavenumber values to better approximating the spectrum profile, thus introducing biased results. Using five Voigt curves faster and more stable profile fittings are obtained, as well as a better reproducibility with lower standard deviations of the values of the center and FWHM of the peaks.
4. RESULTS
4.1. Soot Characterization
The spectral analysis of overall data yielded peak parameters, summarized in the online supplemental information (Table S3), that show values of FWHM and center of the peaks in agreement with those reported by the literature about soot particles (Sadezky et al. Citation2005). Higher and lower values of the center of the peaks are reported by the authors only for the G and D1 bands, respectively. To understand if some differences may occur in the typology of soot in the samples, all the collected spectra were grouped in some classes according to the PM fraction (PM10 vs. PM2.5), the sampling site (CR vs. CF), and the particles composition, that is particles composed of only BC or BC associated with mineral phases (BC vs. BC+min). Each couple represents two complementary classes. For every class the mean peak parameters, as well as the average values for all the data, have been calculated and are reported in Table S4. If the peak parameters of each class are compared with those obtained from all the data, no significant difference is found for the center of the band, whereas a slight difference is observed for the FWHM values. However, all the data exhibit a high standard deviation, thus it is difficult to correlate this shifts to possible differences.
FIG. 2 Frequency histogram plots of the FWHM of D1 band (a), and G band (b) of all the peaks of the collected spectra.
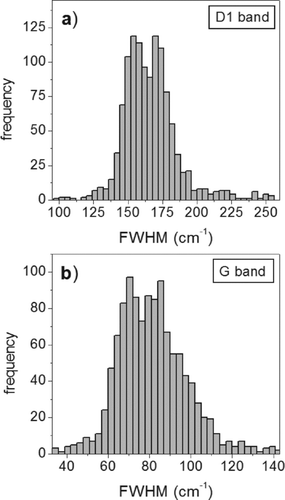
FIG. 3 Frequency histograms of the FWHM of D1 bands for each class of particles: (a) PM2.5, (b) PM10, (c) BC, (d) BC+min, (e) CF, and (f) CR.
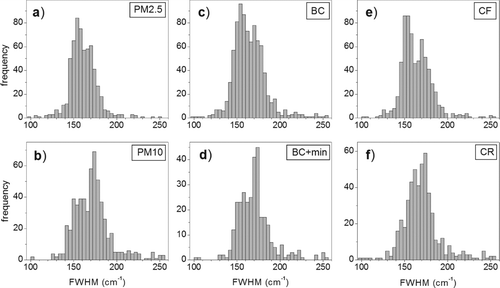
The analysis of the frequency histogram plots of each single parameter yielded more interesting results. Despite the peak height and area values show no significant distribution pattern, the FWHM values of D1 and G peaks show a distinct bimodal distribution pattern for all the analyzed data. As shown in , the main FWHM modes are about 155 and 170 cm−1 for the D1 band, and 70 and 85 cm−1 for the G band.
It has to be noticed that the uncertainties of the center or of the FWHM of the peaks, when calculated on a single measurement (Table S1), are lesser than the distance of the two mentioned modes. Frequency histograms were also examined for each of the six classes of PM ().
Regarding the FWHM of the D1 band, it appears that none of each class of particles shows the same distribution pattern as that of the “all data,” actually every class exhibits one of the two modes larger than the other. Furthermore, one may notice how, within each couple of classes, one exhibits a complementary pattern with respect to the other: the prevailing mode in the PM2.5 class is the one at 155 cm−1, whereas in the PM10 class it is the one at 175 cm−1. Regarding the classes of the sampling sites, the particles collected at CF exhibit a clear bimodal distribution pattern where the prevailing mode is the one located around 150 cm−1, whereas the soot particles collected at CR show the highest frequency of values around 175 cm−1. The same observations can be made about the classes BC and BC+min, respectively.
Concerning the areas of the five Raman bands the ratios of each D band with respect to the G band have been calculated, and the resulting average values are reported in Table S5. Because of the slight differences between the values and the high standard deviations, it is not possible to recognize major trends. In addition, the frequency histograms and box plots were considered. Statistical analysis shows that the D/G values exhibit a lognormal distribution pattern thus the average values and the standard deviations are calculated accordingly. Here, the distribution pattern of D4/G, reported (as logarithm) in , allows to distinguishing the different population of soot.
FIG. 4 Frequency distribution pattern of the ratio ID4/IG (as logarithm) of the FWHM for the PM2.5 and PM10.
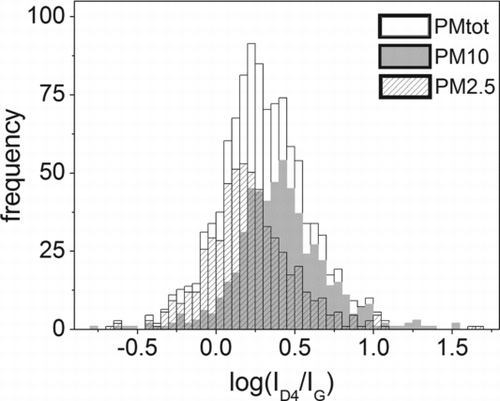
For the classes of PM2.5 and PM10, it is evident the presence of two populations of soot. Also interesting to note the box plot of the D1/G area ratio versus the D1 FWHM () where it can be seen that the averages and the dispersion of the D1/G area ratio increases according to the increase of the D1 FWHM.
4.2. Mineral Phases Characterization
Raman analysis of the particles of PM has been also used for the identification of a certain number of mineral phases associated with soot. For this purpose, the Raman microspectroscopy is a powerful technique, useful for identifying exactly the species present in the aggregates, with the only limitation that, in some cases, the Raman peaks produced by the soot are much more intense than those of the mineral phases. This may not allow a proper identification of the mineral species, but the location of their main peak is often sufficient to distinguish the mineral class (e.g., sulfates, carbonates, phosphates, nitrates). This is not a problem when oxides and silicates are present because they produce, at lower wavenumbers, strong peaks that allow a correct identification. The most widespread phases present in the samples analyzed are sulfates—easily recognizable for the main peak at ∼1000 cm−1 (SO4 symmetric stretching) and mainly associated with soot—commonly gypsum and anhydrite, whereas ammonium sulfate and jarosite are less frequent. Another important class of minerals, although less frequently associated with soot, is carbonates, identified for the strong peak at 1085 cm−1 (CO3 symmetric stretching): mainly calcite but also monohydrocalcite are present. Among silicates we mostly detected quartz, feldspars (plagioclase and orthoclase), pyroxenes, and rarely garnets. Ubiquitous in all the samples are iron oxides, specifically hematite, magnetite, and Fe-oxyhydroxides.
Other phases detected in the samples are phosphates (mainly apatite), nitrates (ammonium nitrate), oxalates (whewellite and weddellite), and oxides (rutile). The relative amount of particles, as percentage by number, differentiated by mineral classes for “all data” and the categories BC and BC+min are shown in .
FIG. 6 The relative amount of particles, as percentage by number, differentiated by mineral classes for “all data,” and the categories BC+min and min. Quartz particles, even belonging to silicates, have been counted separately for a more detailed information.
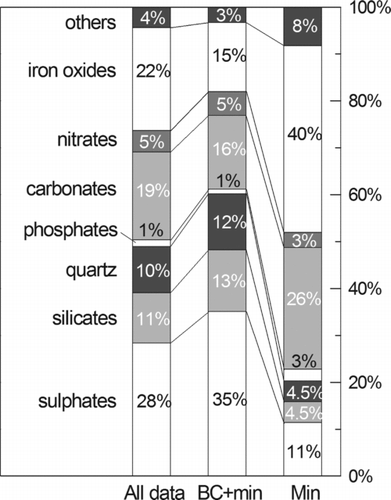
It is to notice that sulfates, as well as silicates and quartz, are mainly associated with soot, whereas carbonates and iron oxides are mainly found as isolated phases. Raman analyses on the particles at the two sampling sites show about the same mineralogical composition, with the exception of a higher amount of iron oxides at the urban sampling site CF, but the number of mineral species detected did not allow obtaining sufficient statistical data for further reflections.
5. DISCUSSION
As concerns the analytical methods, it is worth mentioning that the reason why the PM has been removed from the filters is the necessary consequence of the original goals and strategy adopted for the samples collection, as explained in Matassoni et al. (Citation2011). The PM10 and PM2.5 were collected on polycarbonate filters with devices equipped with the conventional sampling heads employed in Italy by the ARPAs to allow a comparison between the outcome of such studies and the PM values measured at the locations used for PM monitoring. As it is reported in Matassoni et al. (Citation2011), and shown in the online supplemental information (Figure S11), “The average particulate fraction determined by PIXE analysis was 18% for PM10 and 11% for PM2.5, with higher percentages during Saharan episodes (in-dust days) due to the higher contribution of the soil component and lower percentages during the most polluted days when carbon compounds are predominant. The PM mass fraction, which is not explained by PIXE, is mainly composed of H, C, N, and O, major aerosol elements that cannot be detected by this technique.” This implies that the carbonaceous mass is relevant and higher in the PM2.5 than in the PM10–2.5. Only consequently to that study, a part of each filter was employed for Raman analyses, and the only possible way to perform the measurements was that of removing the particles from the filters through carefully washing them, as it is carried out routinely in the TEM analysis procedure. Besides, the efficacy of particle removing was high, and observing carefully the washed filters with an optical microscope at a magnification of 1000×, no particles were spotted on the polycarbonate surface. Any aggregation of the particles removed was observed since their dimensions were included within the ranges of the cutoff values of the samplers. This, and the lack of grouping of particles of unusual shape on the steel support, let us suppose that the formation of particle aggregates is negligible. On the other hand, the fragmentation of particle aggregates was also considered unlikely since the ultrasonic removal of the material from the filters was not necessary and the smooth surface of polycarbonate does not hold the particles.
As regards the experimental results, the Raman spectra have shown that soot particles sampled in two sites of the city of Rome, within 10 days of sampling, have different characteristics that are supported by the analysis of the values of the FWHM of the D1 and G bands. Studies carried out by several authors (Sze et al. Citation2001; Sadezky et al. Citation2005; Ivleva et al. Citation2007a) report that the FWHM of the Raman bands typical of carbonaceous material, such as D1 and G, is a parameter strictly correlated with the degree of crystallinity of the material, and it is most sensitive to its modifications. In particular, the FWHM of the peak is higher in materials with low degree of crystallinity and increases in amorphous and microcrystalline carbon, due to the more disordered structure. Frequency histogram plots reported in clearly suggest that at least two main different typologies of soot are present in the aerosol of Rome: one with higher crystalline degree and another one with a less ordered graphitic structure. As shown by the frequency histogram plots of , the prevailing mode corresponding to more crystalline carbonaceous particles, located around 155 cm−1, is typical of the fine fraction (PM2.5) and the whole BC fraction. Differently, soot particles with greater size (PM10) and those associated to mineral phases (BC+min) show a prevailing mode corresponding to the amorphous/microcrystalline carbon, with a D1 FWHM of about 175 cm−1. Despite it is less evident, D1/G is another parameter sensitive to soot properties and is consistent with D1 FWHM trend, increasing as D1 FWHM increases ().
The attempt of identifying possible BC sources from our data, as in the cases reported by Ivleva et al. (Citation2007a) and Knauer et al. (Citation2009), suffers of a lack of direct comparison of the intensities due to the use of a different laser source. Sadezky et al. (Citation2005) reported that D1 FWHMs show no evidence of light-source dependence (Table S6), but we obtained substantially different values, both with Voigt and Lorentzian–Gaussian fit, for the same standard material NIST SRM 1650b (Table S2). Because of this, it is not possible to make a direct comparison on the absolute FWHM values, but only on the relative ones. Ivleva et al. (Citation2007a), analyzing both standard soot materials and aerosol containing black carbon, showed that D1 FWHM is directly related to the Humic-like substances (HULIS) content in different typologies of soot reported as the apparent elemental carbon to total carbon ratio (ECa/TC). Particularly the carbonaceous component exhibits a mean D1 FWHM of about 175 cm−1 in the finer fraction, that correspond to the D1 FWHM of diesel NIST SRM 1650, and larger values for the coarser fraction, up to a value similar to spark discharge soot (>200 cm−1). This increase of D1 FWHM is linked to the decrease of ECa content. From our data, it is evident that the main classes of the BC particles analyzed have properties similar to those of soot materials, that is consistent with the location of sampling sites, with a minor contribution of spark discharge soot-like materials. In particular, we found that the mode of the more crystalline particles exhibits D1 FWHM values close to the diesel NIST SRM 1650b, according to Ivleva et al. (Citation2007a). Similarly to the outcomes of their research, the particles showing lower D1 FWHM values are prevailing in the finer fraction (1–2.5 μm), whereas those with higher D1 FWHM values are prevailing in the coarser fraction (2.5–10 μm). The authors state that the intensity ratio of the D3 to the G band (ID3/IG) is another parameter highly sensitive to BC properties, but our results show no such evidence. This could be due to the experimental procedure adopted by the authors, such as the different laser source and fitting strategy, as well as to the samples properties due to the bulk analyses and the analyzed fractions. In fact, the authors found the most evident differences of ID3/IG for the PM fraction below 1 μm and the coarser fraction over the 2.5 μm, in the summer samples, whereas, differently, our data relate to the fraction of PM greater than 1 μm sampled at the winter's end. Moreover, we noticed that the ID4/IG parameter is rather useful to detect the differences among D1 FWHMs, and ultimately related to different populations of soot, as for PM2.5 and PM10 (). Although the ID3/IG parameter is considered suitable for revealing the differences on the population of soot, nothing with a similar meaning is reported by the literature about the ID4/IG parameter. Since the D4 band is linked to the amount of impurities, such as ions, metals, and polyenes in the BC (Sadezky et al. Citation2005), the parameter ID4/IG might be reasonably assumed as a distinctive marker.
Such analytical approach lead to the identification of different typologies of BC particles in atmospheric soot, with a clear differentiation depending on their size (PM2.5 vs. PM10), sampling site (CR vs. CF), and phase association (BC vs. BC+min), as shown in . These differences may be due to separate sources emitting soot particles with different size and graphitic component such as vehicular emissions from the nearby extended city and wood or biomass burning. The latter or its by-products released by the burning process for domestic heating might account for a part of the soot present in the ambient air of rural areas, although there is no evidence of such and activity at the CR sampling site. Apart from the source contributions, that may be both present in the rural–suburban area examined, it has been clearly observed by HRTEM investigations on these samples—prepared with the Jaffé Wick Method—the occurrence of amorphous aggregates associated to the crystalline carbonaceous particles of the PM10 fraction (Catelani Citation2010). It has been reported that secondary organic aerosol (SOA) particles, in an amorphous solid state, are formed in the atmosphere from condensable oxidation products of anthropogenic and biogenic volatile organic compounds (Virtanen et al. Citation2010 and references therein), and the phenomenon of the aging of the organic condensate of the soot particles might not be ruled out. Also, Adachi and Buseck (Citation2008) described BC particles growth as the sum of two distinct processes: aggregation of nanoparticles of soot, of less than 100 nm, and organic matter condensation on the aggregates. Nanoparticles emitted directly from the source at high temperature are more crystalline compared with the condensation material that has a higher content of organics, clearly exhibiting less ordered structures. Such particles are commonly characterized by a local crystal order—clearly evident in TEM high-resolution images—with concentric, onion like, arrangement of stacked graphitic layers (Buseck et al. Citation1987; Chen et al. Citation2005). Subsequent growth of these particles is due to aggregation and condensation phenomena, at lower temperature, of organic vapor phases produced during combustion processes, and other impurities. The larger the particles grow, due to the residing time in the atmosphere, the more they increase the coating from the condensation fraction (SOA). This mechanism generates soot particles with higher amount of amorphous carbon and impurities whose presence is detectable through the investigation of the D1 FWHM and ID4/IG values, respectively (Adachi and Buseck Citation2008). Such an evolution process might explain the differences between coarse and fine particles, relating them to a common source, as well as the differences between soot particles of the classes CR and CF. At CF, the main source is the exhaust of automotive engines of a high-traffic urban area, and the main process of soot particles growth is nanoparticles aggregation, with lower condensation of organic matter. On the other hand, the particles sampled at CR exhibit generally a lower crystallinity degree than those of CF because of the higher distance of sampling from the main source, thus a likely higher role of the condensation phenomenon of organic matter in the particles growth. It has to be said that the area around the sampling site of CR, being at the proximity of the city of Rome, is not much affected by rural activities, and methane is widely used as the source for domestic heating.
The hypothesis of the evolution process might explain the fact that those particles made up by both mineral phases and BC show a higher amorphous content. This is probably related to the fact that solid particles may favor the carbon condensation in the atmosphere, forming an internally mixed mineral-soot particles. This is particularly true for sulfates and nitrates (), that are typical condensation phases. In fact, some authors (Posfái et al. Citation1999; Su et al. Citation2004; Coz and Leck Citation2011) observed that sulfates and soot form internally mixed aggregates from submicrometric particles, where the soot largely affects the sulfate condensation. For silicates and the other crustal phases, indeed, it is more likely a mechanism of scavenging soot particles and organic compounds in the atmosphere, working as aggregation nuclei. On the other hand, our data let us suggest that silicates are more efficient scavengers than carbonates and other mineral phases.
Peak analysis of micro-Raman spectra with band deconvolution procedure is useful tool for evaluating the nature of the carbonaceous PM and yields information about the evolution processes. Such characteristics have been found to be much more appreciable by evaluating the peak features relating to different classes of particles and are in agreement with what is reported by the literature on this subject. The present collection and elaboration of data is suitable for giving a further point of view to the airborne particulate analysis, despite it requires a large amount of manual work, and a further effort may be done to improve and mechanize the experimental and analytical process.
Supplemental_Files_for_Publication.zip
Download Zip (15.6 KB)Acknowledgments
The authors would like to thank Dr. Damiano Centioli of the Institute for Environmental Protection and Research (ISPRA, former APAT) for his support to the research work and Dr. Andrea Lucotti of Dipartimento di Chimica, Materiali ed Ingegneria Chimica “G. Natta,” Politecnico di Milano for supplying the standard material.
[Supplementary materials are available for this article. Go to the publisher's online edition of Aerosol Science and Technology to view the free supplementary files.]
REFERENCES
- Adachi , K. and Buseck , P. R. 2008 . Internally Mixed Soot, Sulfates, and Organic Matter in Aerosol Particles from Mexico City . Atmos. Chem. Phys. , 8 : 6469 – 6481 .
- Andreae , M. O. and Gelencsér , A. 2006 . Black Carbon or Brown Carbon? The Nature of Light-Absorbing Carbonaceous Aerosol . Atmos. Chem. Phys. , 6 : 3133 – 3148 .
- Batonneau , Y. , Sobanska , S. , Laureyns , J. and Bremard , C. 2006 . Confocal Microprobe Raman Imaging of Urban Tropospheric Aerosol Particles . Environ. Sci. Technol. , 40 : 1300 – 1306 .
- Blaha , J. J. , Rosasco , G. J. and Etz , E. S. 1978 . Raman Microprobe Characterization of Residual Carbonaceous Material Associated with Urban Airborne Particulates . Appl. Spectrosc. , 32 ( 3 ) : 292 – 297 .
- Brunekreef , B. and Forseberg , B. 2005 . Epidemiological Evidence of Effects of Coarse Airborne Particles on Health . Eur. Respir. J. , 26 : 309 – 318 .
- Buseck , P. R. , Huang , B. and Keller , B. P. 1987 . Electron Microscope Investigation of the Structures of Annealed Carbons . Energ. Fuels , 1 : 105 – 110 .
- Castiglioni , C. and Tommasini , M. 2007 . Raman Spectroscopy of Disordered and Nano-Structured Carbon Materials: The Molecular Approach . Opt. Pura Apl. , 40 ( 2 ) : 169 – 174 .
- Casuccio , G. S. , Lersch , T. L. , Schlaegle , S. F. and Martello , D. 2002 . Characterization of Ambient Carbonaceous Particles using Electron Microscopy Techniques, vol. 47 , 624 – 626 . Boston, MA : ACS Fuel Chemistry Division Preprints . presented at the American Chemical Society 224th National Meeting, Symposium on Monitoring and Control of Air Toxics and Fine Particulate Matter (PM2.5), 18–22 August 2002. Paper
- Catelani , T. 2010 . “ Micro-Raman, FESEM and TEM analysis of airborne particulate soot. M.Sc. thesis, Università degli Studi di Firenze ” .
- Chen , Y. , Shah , N. , Braun , A. , Huggins , F. E. and Huffman , G. P. 2005 . Electron Microscopy Investigation of Carbonaceous Particulate Matter Generated by Combustion of Fossil Fuels . Energ. Fuels , 19 : 1644 – 1651 .
- Coz , E. and Leck , C. 2011 . Morphology and State of Mixture of Atmospheric Soot Aggregates During the Winter Season Over Southern Asia–A Quantitative Approach . Tellus, Ser.: Chem. Phys. Meteorol. , 63B1 : 107 – 116 .
- Davidson , C. I. , Phalen , R. F. and Solomon , P. A. 2005 . Airborne Particulate Matter and Human Health: A Review . Aerosol Sci. Technol. , 39 : 737 – 749 .
- Englert , N. 2005 . Fine Particles and Human Health—A Review of Epidemiological Studies . Toxicol. Lett. , 149 : 235 – 242 .
- Gieré , R. , Blackford , M. and Smith , K. 2006 . TEM Study of PM2.5 Emitted from Coal and Tire Combustion in a Thermal Power Station . Environ. Sci. Technol. , 40 : 6235 – 6240 .
- Gołabczak , M. and Konstantynowicz , A. 2009 . Raman Spectra Evaluation of the Carbon Layers with Voigt Profile . J. Achiev. Mat. Manuf. Eng. , 1 : 270 – 276 .
- Grobéty , B. , Gieré , R. , Dietze , V. and Stille , P. 2010 . Airborne Particles in the Urban Environment . Elements , 6 ( 4 ) : 229 – 234 .
- Gualtieri , M. , Mantecca , P. , Corvaja , V. , Longhin , E. , Perrone , M. G. Bolzacchini , E. 2009 . Winter Fine Particulate Matter from Milan Induces Morphological and Functional Alterations in Human Pulmonary Epithelial Cells (A549) . Toxicol. Lett. , 188 ( 1 ) : 52 – 62 .
- Gualtieri , M. , Øvrevik , J. , Holme , J. A. , Perrone , M. G. , Bolzacchini , E. Schwarze , P. E. 2010 . Differences in Cytotoxicity Versus Pro-Inflammatory Potency of Different PM Fractions in Human Epithelial Lung Cells . Toxicol. In Vitro , 24 : 29 – 39 .
- Ivleva , N. P. , McKeon , U. , Niessner , R. and Pöschl , U. 2007a . Raman Microspectroscopic Analysis of Size-Resolved Atmospheric Aerosol Particle Samples Collected with an ELPI: Soot, Humic-Like Substances, and Inorganic Compounds . Aerosol Sci. Tech. , 41 : 655 – 671 .
- Ivleva , N. P. , Messerer , A. , Yang , X. , Niessner , R. and Pöschl , U. 2007b . Raman Microspectroscopic Analysis of Changes in the Chemical Structure and Reactivity of Soot in a Diesel Exhaust After Treatment Model System . Environ. Sci. Technol. , 41 : 3702 – 3707 .
- Knauer , M. , Schuster , M. E. , Su , D. , Schogl , R. , Niessner , R. and Ivleva , N. P. 2009 . Soot Structure and Reactivity Analysis by Raman Microspectroscopy, Temperature-Programmed Oxidation, and High-Resolution Trasmission Electron Microscopy . J. Phys. Chem. A , 113 : 13871 – 13880 .
- Lee , D. , Miller , A. , Kittelson , D. and Zachariah , M. R. 2006 . Characterization of Metal-Bearing Diesel Nanoparticles Using Single-Particle Mass Spectrometry . Aerosol Sci. , 37 : 88 – 110 .
- Mamane , Y. , Willis , R. and Conner , T. 2001 . Evaluation of Computer-Controlled Scanning Electron Microscopy Applied to an Ambient Urban Aerosol Sample . Aerosol Sci. Technol. , 34 : 97 – 107 .
- Matassoni , L. , Pratesi , G. and Centioli , D. 2012 . Comments on “Quantification of Saharan Dust Contribution to PM10 Concentrations over Italy During 2003–2005. . Atmosph. Environ. , 46 : 680 – 681 .
- Matassoni , L. , Pratesi , G. , Centioli , D. , Cadoni , F. , Lucarelli , F. Nava , S. 2011 . Saharan Dust Contribution to PM10, PM2.5 and PM1 in Urban and Suburban Areas of Rome: A Comparison Between Single-Particle SEM-EDS Analysis and Whole-Sample PIXE Analysis . J. Environ. Monit. , 13 : 732 – 742 .
- Mathis , U. , Kaegi , R. , Mohr , M. and Zenobi , R. 2004 . TEM Analysis of Volatile Nanoparticles from Particle Trap Equipped Diesel and Direct-Injection Spark-Ignition Vehicles . Atmosph. Environ. , 38 : 4347 – 4355 .
- Mertes , S. , Dippel , B. and Schwarzenböck , A. 2004 . Quantification of Graphitic Carbon in the Atmospheric Aerosol Particles by Raman Spectroscopy and the Determination of Mass Absorption Efficiencies . Aerosol Sci. , 35 : 347 – 361 .
- Posfái , M. , Anderson , J. R. and Buseck , P. R. 1999 . Soot and Sulphate Aerosol Particles in the Remote Marine Troposphere . J. Geophys. Res. , 104 ( 21 ) : 685 – 693 .
- Risom , L. , Møller , P. and Loft , S. 2005 . Oxidative Stress-Induced DNA Damage by Particulate Air Pollution . Mutat. Res. , 592 : 119 – 137 .
- Sadezky , A. , Muckenhuber , H. , Grothe , H. , Niesser , R. and Pöschl , U. 2005 . Raman Micro-Spectroscopy of Soot and Related Carbonaceous Materials: Spectral Analysis and Structural Information . Carbon , 43 : 1731 – 1742 .
- Sakurai , H. , Tobias , H. J. , Park , K. , Zarling , D. , Docherty , S. Kittelson , D. B. 2003 . On-Line Measurements of Diesel Nanoparticle Composition and Volatility . Atmos. Environ. , 37 : 1199 – 1210 .
- Sørensen , M. , Autrup , H. , Møller , P. , Hertel , O. , Jensen , S. S. Vinzents , P. 2003 . Linking Exposure to Environmental Pollutants with Biological Effects . Mutat. Res. , 544 : 255 – 271 .
- Stefaniak , E. A. , Buczynska , A. , Novakovic , A. , Kuduk , R. and Van Grieken , R. 2009 . Determination of Chemical Composition of Individual Airborne Particles by SEM/EDX and Micro-Raman Spectrometry: A Review . J. Phys. , 162 : 1 – 16 .
- Su , D. S. , Jentoft , R. E. , Müller , J.-O. , Rothe , D. , Jacob , E. Simpson , C. D. 2004 . Microstructure and Oxidation Behaviour of Euro IV Diesel Engine Soot: A Comparative Study with Synthetic Model Soot Substances . Catal. Today , 90 : 127 – 132 .
- Sze , S.-K. , Siddique , N. , Sloan , J. J. and Escribano , R. 2001 . Raman Spectroscopic Characterization of Carbonaceous Aerosols . Atmos. Environ. , 35 : 561 – 568 .
- Tommasini , M. , Castiglioni , C. , Zerbi , G. , Barbon , A. and Brustolon , M. 2011 . A Joint Raman and EPR Spectroscopic Study on Ball-Milled Nanographites . Chem. Phys. Lett. , 516 : 220 – 224 .
- Virtanen , A. , Joutsensaari , J. , Koop , T. , Kannosto , J. , Yli-Pirilä , P. Leskinen , J. 2010 . An Amorphous Solid State of Biogenic Secondary Organic Aerosol Particles . Nature , 467 : 824 – 827 .
- Wang , Y. , Alsmeyer , D. C. and McCreery , R. L. 1990 . Raman Spectroscopy of Carbon Materials: Structural Basis of Observed Spectra . Chem. Mater. , 2 : 557 – 563 .
- Wojdyr , M . 2010 . Fityk: A General-Purpose Peak Fitting Program . J. Appl. Cryst. , 43 : 1126 – 1128 .
- Zanatta , A. R. and Ferri , F. A. 2007 . Crystallization, Stress, and Stress-Relieve Due to Nickel in Amorphous Silicon Thin Films . J. Appl. Phys. , 102 : 043509
- Zerbi , G. , Ferruggiari , A. , Fustella , G. , Tommasini , M. , Mantecca , P. Gualtieri , M. 2008 . Preliminary Observation on the Interactions Between Fine Atmospheric Particulate Matter (PM2.5) and Human Alveolar Epithelial Cells (A549) . Chem. Eng. Trans. , 16 : 387 – 394 .