Abstract
Predicting indoor exposures to ambient organic aerosol (OA) is complicated by shifts in the gas-particle partitioning of ambient organics with outdoor-to-indoor transport. This analysis aims to quantify the effect of changes in temperature and OA loading on the gas-particle partitioning of ambient organics transported indoors and explores whether accounting for shifts in partitioning closes the gap between measured indoor ambient OA concentrations and indoor concentrations calculated in a previous analysis using a model that accounts for only physical losses. Changes in the gas-particle partitioning of ambient organics with outdoor-to-indoor transport were calculated for 167 homes using measured temperatures and OA concentrations and published OA volatility distributions. Initially, it was assumed that ambient OA could be represented with a single volatility distribution. We then repeated the analysis treating ambient OA as the sum of distinct components, each with a distinct volatility distribution, derived from factor analysis of aerosol mass spectra (e.g., hydrocarbon-like OA [HOA], oxygenated OA [OOA]). We also evaluated the sensitivity of our calculations to uncertainty in the thermodynamic properties of ambient OA by varying the enthalpy of vaporization. Partitioning shifts were sensitive to enthalpy-of-vaporization assumptions and resulted in changes in indoor ambient OA concentrations of 13–27%. Our calculations indicate that phase changes are important determinants of residential exposure to ambient OA and are of sufficient magnitude to close the gap between measured and modeled indoor concentrations of ambient OA.
Copyright 2014 American Association for Aerosol Research
INTRODUCTION
Ambient PM2.5 exposure mitigation strategies, risk assessment, and health studies all benefit from accurate exposure prediction. Because people spend about 70% of time in their homes (Klepeis et al. Citation2001), the residence is an important setting for exposure to PM2.5 of ambient (outdoor) origin. The efficiency with which ambient PM2.5 penetrates into and persists in indoor air, and thus the fraction of ambient PM2.5 that people are exposed to in their homes, varies across PM2.5 species due to differences in particle size distributions and thermodynamic properties (Lunden et al. Citation2003a; Sarnat et al. Citation2006; Meng et al. Citation2007; Hodas et al. Citation2012). For chemically nonreactive species (e.g., sulfate, elemental carbon), outdoor-to-indoor transport is governed by the physical losses associated with penetration across the building envelope and deposition indoors (Riley et al. Citation2002; Nazaroff Citation2004; Sarnat et al. Citation2006; Hering et al. Citation2007; Hodas et al. Citation2013). It has been demonstrated for nitrate and polycyclic aromatic hydrocarbons (PAHs), however, that semivolatile species can also undergo phase changes with outdoor-to-indoor transport due to changes in temperature, surface area, and the availability of particulate matter for sorption (Lunden et al. Citation2003b; Naumova et al. Citation2003). Organics are a major component of outdoor and indoor-generated PM2.5 (Polidori et al. Citation2006). While previous work provides evidence that organics also undergo phase changes with outdoor-to-indoor transport (Lunden et al. Citation2008; Hodas et al. Citation2013), to our knowledge this process has not been incorporated into models used to predict ambient PM2.5 exposure.
The thermodynamic principles governing gas-particle partitioning of ambient organics are well established (Pankow Citation1994). Increasing temperature increases organic vapor pressures, shifting organic mass from the particle phase to the gas phase. On the other hand, organic aerosol (OA) provides a medium for sorption of semivolatile organics. As a result, increasing concentrations of OA (e.g., with the introduction of indoor sources) shift organic mass from the gas to the particle phase. While the thermodynamics are understood, modeling these processes for atmospheric organics is hindered by their complexity. Atmospheric organic matter is comprised of thousands of compounds with largely unknown identities and a broad range of thermodynamic properties (Goldstein and Galbally Citation2007; Hallquist et al. Citation2009). Thus, explicitly modeling the gas-particle partitioning of all individual organic compounds comprising ambient OA is not practical. The partitioning behavior of atmospheric organics is often parameterized with a volatility basis set (VBS; Donahue et al. Citation2006), which treats organics as a distribution of compounds binned by their volatilities. More specifically, the VBS is a distribution of saturation vapor pressures expressed in concentration units (C*) with log10 spacing that span the range of atmospherically-relevant organic saturation concentrations (Donahue et al. Citation2006). A volatility distribution with C* on the x-axis and total (gas + particle) organic mass (OM) on the y-axis can be used to describe the gas-particle partitioning of ambient organics as a function of temperature and OA loading (Donahue et al. Citation2006).
In this article, we present the first study to utilize this volatility distribution to model shifts in the gas-particle partitioning of ambient organics with transport into the indoor environment. We consider changes in temperature, and that indoor sources add considerable OA for sorptive partitioning. Recently, volatility distributions were generated for ambient OA and OA components measured in regions dominated by anthropogenic PM2.5 sources (Cappa and Jimenez Citation2010). We utilized these volatility distributions to explore the thermodynamic behavior of ambient OA with outdoor-to-indoor transport for multiple homes located in three urban regions of the United States: Elizabeth, NJ; Los Angeles County, CA; and Houston, TX.
Notably, a previous study demonstrated that shifts in gas-particle partitioning were significant contributors to variability in measured indoor OA for these homes and that not accounting for these shifts significantly contributed to error in predicted indoor concentrations of ambient OA (Hodas et al. Citation2013). In that study, indoor concentrations of ambient OA were calculated with a single compartment mass balance model that accounted for the physical processes that govern outdoor-to-indoor transport (i.e., the efficiency with which particles penetrate across the building envelope, depositional losses indoors, and losses in the filters of HVAC systems), but not shifts in gas-particle partitioning. A comparison between these modeled indoor concentrations of ambient OA and a statistical estimate of the measured indoor OA that could be attributed to outdoor sources demonstrated a lack of closure. An estimate of the measured ambient OA indoors was needed because the indoor concentrations measured in these occupied homes include OA of both outdoor and indoor origin (Hodas et al. Citation2013). The current article uses volatility distributions to illustrate and quantify the effect of changes in temperature and OA loading on the gas-particle partitioning of ambient organics found indoors and explores whether these changes in OA concentrations are of adequate magnitude to achieve model-measurement closure.
METHODS
Overview
As is described in detail below, the change in the gas-particle partitioning of ambient organics with outdoor-to-indoor transport was calculated for homes sampled during the Relationships of Indoor, Outdoor, and Personal Air (RIOPA) Study. Changes in partitioning due to indoor–outdoor temperature differences were considered, as was the fact that indoor sources emit OA to which ambient organics can sorb. We examined the extent to which positive (net sorption) and negative (net volatilization) shifts were driven by changes in OA loading and changes in temperature. Finally, we compared the magnitude of shifts in partitioning with the size of the measurement-model gap discussed above. This was accomplished using measured temperatures and OA concentrations from RIOPA (Weisel et al. Citation2005; Turpin et al. Citation2007) and published volatility distributions for ambient OA (Cappa and Jimenez Citation2010). It was assumed that volatility distributions generated for ambient OA measured during the MILAGRO campaign in Mexico City are representative of the volatility distributions of the ambient OA at each of the three RIOPA sites. This assumption is supported by the fact that mass thermograms (measurements of the fraction of mass remaining in the particle phase as a function of temperature) for the ambient OA in Riverside, CA are similar to those for Mexico City, suggesting similarities in the thermodynamic properties of ambient OA for regions dominated by anthropogenic OA sources (Huffman et al. Citation2009; Cappa and Jimenez Citation2010). Initially, the volatility of ambient OA was represented with a single volatility distribution using an enthalpy of vaporization (ΔH vap) of 100 kJ/mol, a value considered reasonable when ambient OA is treated as a mixture of compounds with a range of volatilities, as is the case for the VBS (Donahue et al. Citation2006; Cappa and Jimenez Citation2010). However, a preferred approach would be one in which differences in the sources and formation mechanisms (and thus differences in thermodynamic properties) of ambient OA with season and geographic region are accounted for. Thus, we repeated this analysis treating ambient OA as the sum of several distinct components, each with its own distinct volatility distribution, derived from factor analysis of aerosol mass spectra (assuming an ΔH vap of 100 kJ/mol for all components). In this alternative analysis, volatility distributions in different cities and seasons were represented by an average of the of the OA component volatility distributions weighted by the mass fractions of the OA components in each of those seasons and locations. We also evaluated the sensitivity of our calculations to uncertainty in the thermodynamic properties of ambient OA by repeating these analyses assuming a ΔH vap of 50 kJ/mol.
RIOPA Study Measurements
RIOPA study measurements are described in detail by Weisel et al. (Citation2005) and Turpin et al. (Citation2007). Measurements included indoor and outdoor particulate organic carbon (OC) samples collected for 173 homes. Briefly, OC concentrations were measured by thermal-optical transmittance with a Sunset Carbon Analyzer using the NIOSH temperature protocol and were corrected for the adsorption of gas-phase semivolatile organic compounds on the quartz fiber filters used for collection. OC concentrations (μgC/m3) were converted to OA concentrations (μg/m3) assuming an OA:OC ratio of 1.4. A total of 167 homes had all data required for the calculations conducted in this article (indoor and outdoor temperature and OC concentrations). Summary statistics for the RIOPA data used in our calculations are shown in .
TABLE 1 Summary statistics of measured indoor and outdoor temperatures and organic aerosol (OA) concentrations for the RIOPA study homes included in this article. Only homes for which all measurements shown above were available were included in this analysis (n = 167)
Volatility Distributions
The calculation of the volatility distributions for ambient OA is described in detail elsewhere (Cappa and Jimenez Citation2010). Briefly, during the MILAGRO campaign in Mexico City, aerosol was pulled through a thermodenuder that heated the aerosol stepwise between ambient temperature and 230°C. Organic mass fragments remaining in the particle phase at each temperature were measured in an aerosol mass spectrometer (TD-AMS; Huffman et al. Citation2009). These measurements and a detailed model of aerosol evaporation in the TD-AMS system were used to generate volatility distributions for ambient OA at 25°C and the campaign-average OA loading (17 μg/m3; Cappa and Jimenez Citation2010). The fitting approach involved fixing two sets of free parameters: (1) the range of C* bins that described the volatility distributions of the measured aerosol and (2) the total OM (gas-phase + particle-phase) in each of those C* bins (Ci ,tot). The authors used an iterative approach to determine the range of C* bins that maximized agreement between modeled aerosol evaporation and the TD-AMS measurements. Ci ,tot in each of the bins was calculated assuming an exponential relationship between C* and Ci ,tot and, again adjusting this relationship iteratively until model-measurement agreement was maximized. Volatility distributions were calculated for a range of ΔH vap assumptions, as well as for OA components derived from factor analysis of aerosol mass spectra: hydrocarbon-like OA (HOA), biomass burning OA (BBOA), oxygenated OA (OOA), semivolatile oxygenated OA (SV-OOA), and low-volatility oxygenated OA (LV-OOA; 2010; Cappa and Jimenez Citation2010). Factor analysis of AMS spectra categorizes OA based on the temporal variability of measured compound mass fragments. As a result, any given OA component is comprised of compounds with similar sources, formation mechanisms, and physiochemical properties (Zhang et al. Citation2005a,Citationb, Citation2007, Citation2011; Lanz et al. Citation2007; Ulbrich et al. Citation2009). Thus, while the contribution of any given OA component will vary temporally and spatially (Zhang et al. Citation2007), the physiochemical properties of this OA component are likely to be similar across seasons and geographic regions.
Shifts in Gas-Particle Partitioning with Outdoor-to-Indoor Transport
In the initial analysis in which ambient OA volatility was represented with a single distribution, volatility distributions were first calculated for the ambient conditions (i.e., temperature and OA loading) measured outside of each RIOPA home using the parameters presented in Cappa and Jimenez (Citation2010; Table S1 in the online supplementary information). Volatility distributions for the average outdoor conditions for the homes considered here (18.2°C, 4.87 μg/m3) are shown in . The full bar for each saturation vapor pressure bin (C*i ) indicates the total OM (gas + particle phase) in that volatility (saturation vapor pressure) bin. The shaded region indicates the fraction of that OM that is in the particle phase (ξi ) assuming absorptive partitioning into a single, well-mixed condensed phase:
FIG. 1 Volatility distributions for the average conditions measured outside of RIOPA homes (temperature = 18.2°C and OA loading = 4.87 μg/m3). (a) total OA assuming an enthalpy of vaporization of 100 kJ/mol; (b) total OA assuming an enthalpy of vaporization of 50 kJ/mol; (c)–(f) OA components derived from factor analysis assuming an enthalpy of vaporization of 100 kJ/mol for all components: hydrocarbon-like OA (HOA), oxygenated OA (OOA), semivolatile oxygenated OA (SV-OOA), and low-volatility oxygenated OA (LV-OOA). The distribution for “other” OA (Table S2) is assumed to be the same as that for total OA (ΔH vap = 100 kJ/mol; panel a). The full bar for each saturation vapor pressure bin (C*) indicates the total OM (gas + particle phase) in that volatility (saturation vapor pressure) bin. The shaded region indicates the fraction of that OM that is in the particle phase assuming absorptive partitioning into a single, well-mixed condensed phase. Adapted with permission from Cappa and Jimenez (Citation2010).
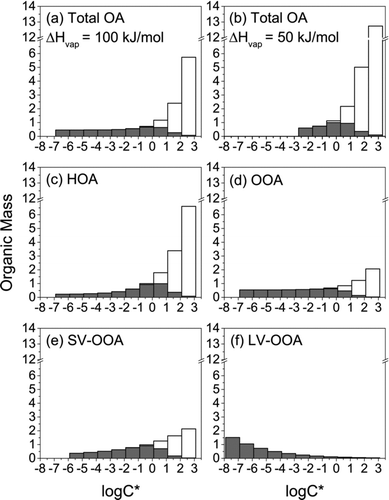
As noted above, the published volatility-distribution parameters (Cappa and Jimenez Citation2010; Table S1) are for an ambient temperature of 25°C. The distribution of C* bins for the temperature measured outside of each home was calculated assuming that the temperature-dependence of C* can be described by the Clausius-Clapeyron equation:
It should be noted that these volatility distributions were used only to simulate the change in partitioning behavior of the ambient organics. In other words, we used the volatility distributions to calculate the fraction of total ambient OM (gas and particle phase) that was in the condensed phase (ξtot) outside of each RIOPA home:
We then used this calculated fraction of OM in the particle phase outside of each RIOPA home (ξtot,out) and the measured outdoor OA concentration to determine the total OM in the gas plus particle phase outside of each home:
Using the same methods, we then calculated the fraction of that ambient C tot that would be found in the particle phase for the temperature and OA loading measured inside each RIOPA home (ξtot,in). In other words, we simulated the new equilibrium partitioning of the ambient OM after it was transported into the indoor environment. Note that measured indoor OA concentrations, which were used to estimate the total OA loading indoors (i.e., C OA in EquationEquation (1)), include emissions from indoor sources into which ambient organics can partition, as well as the fraction of ambient OA that has penetrated into and persisted in indoor air. We assumed that there was no change in the thermodynamic properties of the ambient organics with outdoor-to-indoor transport (i.e., the same volatility distribution could be used to represent ambient OA before and after transport indoors). Possible limitations of this assumption are discussed in the Results and Discussion section. Indoor concentrations of ambient OA (C OA,in,amb) were calculated by multiplying ξtot,in by C tot. Changes in OA mass due to re-partitioning with outdoor-to-indoor transport were calculated by subtracting measured outdoor OA concentrations from those calculated after repartitioning to indoor conditions (i.e., temperature and OA loading):
In a more sophisticated analysis, we treated ambient OA as a mixture of components derived from factor-analysis of aerosol mass spectra. In these chemically-resolved calculations, ΔH vap is assumed to be uniform across the OA components (100 kJ/mol); however, the volatility distribution for total OA varies across locations and seasons because the mass fractions of the OA components differ and each component has a unique volatility distribution (CitationFigures 1c–Citationf). While we had measurements of OA outside of each home, we did not have measurements of AMS OA components. Thus, measured OA component mass fractions for Riverside, New York City, and Houston (Drewnick et al. Citation2004a,Citationb; DeCarlo et al. Citation2006; Weimer et al. Citation2006; Cubison et al. Citation2008; Docherty et al. Citation2008; Jimenez et al. Citation2009) were used to apportion measured OA between the components for LA County, Elizabeth, and Houston RIOPA homes, respectively (Table S2). The measured mass fractions are season-specific and, thus, only homes that were sampled during the seasons for which OA component mass fractions were available were included in the calculations that utilized the volatility distributions for OA components. For Houston and Riverside, only warm season (May–October) mass fractions were available, while for New York, component mass fraction measurements were available for both the warm and cool (November–April) seasons (Table S2). Aggregate volatility distributions for each RIOPA region (i.e., those calculated as an average of these component-specific distributions weighted by the mass fraction of each component) are provided in Figure S1. Volatility distributions were calculated for the ambient conditions (temperature and OA loading) measured outside each RIOPA home for each of the OA components using the OA-component-specific parameters presented in Cappa and Jimenez (Citation2010; Table S1). Note that for these calculations, outdoor concentrations of each OA component (rather than the total outdoor OA concentrations) are used as inputs for C OA in EquationEquation (1). These were calculated by multiplying the component mass fractions in Table S2 by the OA measured outside of each RIOPA home. Shifts in the gas-particle partitioning of each of these OA components with outdoor-to-indoor transport were then calculated using the same methods as described above. The total ΔC OA due to shifts in partitioning for each RIOPA home was calculated by summing ΔC OA across all of the OA components.
In order to determine the data most important for predicting shifts in gas-particle partitioning with outdoor-to-indoor transport, we then explored whether changes in temperature or changes in OA loading were the dominant drivers of variability in calculated gas-particle partitioning shifts. This will clarify what measurements and data are most needed in order to incorporate this process into predictive ambient PM exposure models. For example, can shifts in partitioning largely be predicted knowing only the indoor temperature or is it necessary to also characterize indoor OA emission rates? Using multiple linear regression (MLR) with stepwise selection (α = 0.15 for variable entrance and removal threshold; SAS version 9.3, SAS Inc., Cary, NC, USA), we regressed ΔC OA on the indoor–outdoor temperature difference and the indoor–outdoor difference in OA mass loading, which was calculated by subtracting measured outdoor OA mass concentrations from measured indoor OA mass concentrations. This analysis systematically evaluates the predictive capability afforded by including either one or both variables. It should again be kept in mind that the total OA loading measured inside each home includes OA emitted or formed inside the home and is a different value than the OA of ambient origin calculated above. Variance inflation factors indicated that there was no correlation between indoor–outdoor differences in temperature and OA loading. For unoccupied homes, we would expect these two quantities to be correlated because temperature influences the fraction of OM in the particle phase (Pankow Citation1994); however, for these occupied homes, indoor sources of organics are the driving force for variability in measured indoor OA mass concentrations (Polidori et al. Citation2006). Outliers were identified with a student's t-test and were removed if t > 2. The MLR analysis was conducted for each ΔH vap assumption, as well as for each urban region in order to explore variability in partitioning shifts across climatic regions.
Model-Measurement Closure
In order to explore whether changes in OA concentrations due to shifts in partitioning with outdoor-to-indoor transport can explain, at least in part, the gap between measured and modeled indoor ambient OA concentrations observed in the previous study discussed above, we compared the previously calculated distribution of model error with the distributions of ΔC OA calculated here. In the previous analysis (Hodas et al. Citation2013), we compared (1) indoor concentrations of ambient OA calculated for RIOPA homes using an outdoor-to-indoor transport model that accounted only for home ventilation and physical loss processes (i.e., air exchange rates, particle penetration efficiencies, and depositional losses) and (2) a statistical estimate of the OA measured indoors that could be attributed to outdoor sources. As noted above, an estimate of the measured indoor OA of ambient origin was required because indoor OA measurements included ambient OA that had penetrated and persisted indoors, as well as OA emitted by indoor sources. We concluded that the remaining differences between modeled values and the statistical estimate of measured indoor OA of ambient origin could likely be attributed to phase changes with outdoor-to-indoor transport. If shifts in partitioning explain this closure gap, we would expect the magnitude of ΔC OA to be equal in magnitude, but opposite in sign of the model-measurement disagreement (i.e. ΔC OA would offset model error). In order to compare these quantities directly, we multiplied values of model error (modeled-measured) by −1. Thus, if the distributions of “model error” and ΔC OA are similar, shifts in partitioning with outdoor-to-indoor transport could plausibly close this model-measurement disagreement. We compared the distribution of model error to all calculations of ΔC OA (i.e., those assuming ΔH vap = 100 kJ/mol, those assuming ΔH vap = 50 kJ/mol, and those treating ambient OA as a mixture of factor-analysis components).
RESULTS AND DISCUSSION
In the main analysis (i.e., ΔH vap = 100 kJ/mol), partitioning shifts resulted in a loss of OA mass for 56% of homes (i.e., net volatilization; shifts from the particle phase toward the gas phase), and changes in ambient OA concentrations due to these shifts in partitioning (ΔC OA) ranged from −4.6 to 2.4 μg/m3 for individual homes (; Table S3). Negative values indicate net volatilization and positive indicate net absorption with outdoor-to-indoor transport. Calculated shifts in partitioning with outdoor-to-indoor transport were highly sensitive to ΔH vap assumption (). Both the magnitudes and the direction of partitioning shifts varied across these analyses. While the main analysis resulted in a loss of OA mass for 56% of homes, partitioning shifts resulted in an increase in OA mass for 61% of homes in the sensitivity analysis (ΔH vap = 50 kJ/mol). Values of ΔC OA for individual homes spanned a wider range in the sensitivity analysis (–10.5 to 5.7 μg/m3) compared to the main analysis. Outdoor-to-indoor transport resulted in an absolute change in C OA (i.e., net absorption or volatilization) of only 13%, on average, in the main analysis, while a 27% change, on average, was observed in the sensitivity analysis.
FIG. 2 Frequency distributions of the change in organic aerosol mass concentrations due to changes in gas-particle partitioning with outdoor-to-indoor transport (ΔC OA) assuming an enthalpy of vaporization (ΔH vap) of (a) 100 kJ/mol and (b) 50 kJ/mol. Negative values indicate net volatilization, whereas positive values indicate net absorption.
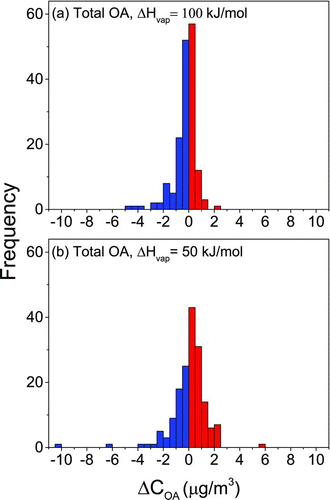
Differences with ΔH vap assumptions can partially be attributed to the fact that shifts in C* with temperature are dependent on ΔH vap (EquationEquation (2)). However, this effect is relatively small (Cappa and Jimenez Citation2010). The differences in gas-particle partitioning shifts are mostly the result of differences in the volatility distributions for these two ΔH vap assumptions. The volatility distribution generated for ΔH vap = 100 kJ/mol has more mass in lower C* bins (). In other words, when an ΔH vap of 100 kJ/mol is assumed, lower volatility material comprises a larger fraction of the OA and, thus, this OA is less sensitive to indoor–outdoor differences in OA loading than that with an ΔH vap of 50 kJ/mol. As is evident from , which shows shifts in gas-particle partitioning that would result if only indoor–outdoor temperature differences or only indoor–outdoor differences in OA loading were considered, the change in OA associated with temperature is relatively similar across ΔH vap values, but the OA with an ΔH vap of 50 kJ/mol shows a much greater sensitivity to changes in OA loading with outdoor-to-indoor transport.
FIG. 3 Frequency distributions of the change in organic aerosol mass concentrations due to changes in gas-particle partitioning with outdoor-to-indoor transport (ΔC OA) accounting only for (a)–(b) indoor–outdoor temperature differences and (c)–(d) indoor–outdoor differences in OA loading.
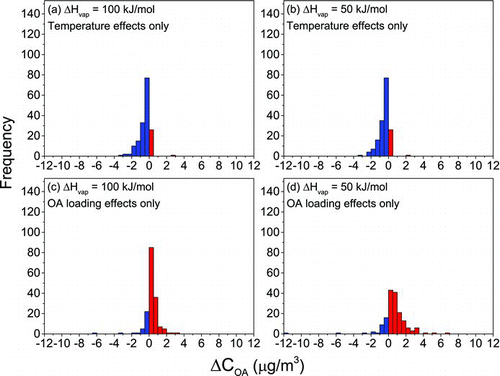
TABLE 2 Multiple linear regression (MLR) analyses investigating the contribution of indoor–outdoor differences in temperature and organic aerosol (OA) loading to changes in ambient OA concentrations (ΔC OA) due to shifts in gas-particle partitioning with outdoor-to-indoor transport. Partial R 2 describes the variance in ΔC OA explained by each variable individually. Model R 2 describes the total variance in ΔC OA described by the full MLR model (i.e., the cumulative R 2). Coefficient estimates describe the change in partitioning (i.e., ΔC OA; μg/m3) per unit difference between indoor and outdoor temperature (°C) and in OA loading (μg/m3)
This effect is also evident in our MLR results (). In the main analysis (ΔH vap = 100 kJ/mol), the change in temperature with outdoor-to-indoor transport was the dominant predictor of variability in ΔC OA, explaining 45% of this variability (R 2 = 0.45). The indoor–outdoor difference in OA loading explained 24% (R 2 = 0.24) of the variability in ΔC OA. When an ΔH vap of 50 kJ/mol was assumed, however, the indoor–outdoor difference in OA loading was the dominant driver of variability in shifts in partitioning, explaining 51% of the variability in ΔC OA (R 2 = 0.51). The indoor–outdoor temperature difference explained 23% of this variability (R 2 = 0.23). The MLR results indicate that both temperature and OA emissions inside homes are important for predicting shifts in partitioning of ambient organics with outdoor-to-indoor transport. Note that indoor–outdoor temperature differences also affect ambient exposure prediction by affecting residential air exchange rates.
Geographic differences in the results (, ) demonstrate that heterogeneity across climatic regions in indoor–outdoor temperature differences and in the human activities that influence these differences (e.g., air conditioning [AC] and heating use) impact gas-particle partitioning shifts with outdoor-to-indoor transport. In the relatively moderate climate of Los Angeles County, where both heating and AC use were low (Weisel et al. Citation2005; Meng et al. Citation2009), indoor temperatures were greater than outdoor temperatures for 98% of homes and the average indoor–outdoor temperature difference was 4.8°C. In Elizabeth, where some of the homes sampled in the winter had heating in use (Weisel et al. Citation2005; Meng et al. Citation2009), indoor temperatures were again greater than outdoor temperatures for the vast majority of homes (96%), but the average indoor–outdoor temperature difference was about twice that observed in Los Angeles County (9.9°C). Finally, in Houston, where central AC use was greater than for the other two urban regions (Weisel et al. Citation2005; Meng et al. Citation2009), there was a decrease in temperature with indoor transport for 30% of homes and indoor–outdoor temperature differences ranged from −7.2°C to 12.8°C. Interestingly, Houston is the only region for which there was a net increase in OA mass (shifts from the gas phase to the particle phase) for the majority of homes (64% compared to 31 and 30% for Los Angeles County and Elizabeth; ), indicating that human activities in addition to those associated with indoor emissions of OA (i.e., heating and AC use) can influence gas-particle partitioning. The greater range of indoor–outdoor temperature differences in Elizabeth and Houston likely explains why partitioning shifts were dominantly driven by changes in temperature for those two regions, while the change in OA loading was the dominant predictor of shifts in partitioning for Los Angeles County homes (). While not explored here directly because of sample-size restrictions, these results also indicate that there are likely seasonal differences in shifts in gas-particle partitioning (and OA exposure) in regions for which there is seasonal heterogeneity in indoor-outdoor temperature differences and in heating and AC use.
FIG. 4 Frequency distributions of the change in organic aerosol mass concentrations due to changes in gas-particle partitioning with outdoor-to-indoor transport (ΔC OA) for the three geographically and climatically diverse urban regions: (a) Los Angeles County, CA; (b) Elizabeth, NJ; (c) Houston, TX.
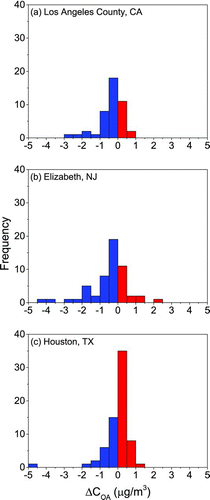
Shifts in gas-particle partitioning calculated for RIOPA homes using the volatility distribution generated for OA components were qualitatively similar to those calculated in the main analysis (ΔH vap = 100 kJ/mol); however, this aerosol demonstrated slightly lower sensitivity to temperature and OA-loading changes with outdoor-to-indoor transport (). These shifts resulted in an absolute change in C OA (i.e., net absorption or volatilization) of 11%, on average, compared to 13% for the main analysis. HOA was most sensitive to changes in temperature and OA loading with outdoor-to-indoor transport (20% change in OA concentrations, on average), followed by “other” OA (13% change on average), and OOA (12%, on average; ). Indoor transport resulted in a 7% change in C OA, on average, for SV-OOA, whereas outdoor-to-indoor transport did not induce shifts in partitioning for LV-OOA ().
FIG. 5 Frequency distributions of the change in organic aerosol mass concentrations due to changes in gas-particle partitioning with outdoor-to-indoor transport (ΔC OA) for (a) total OA assuming that OA can be represented as a mixture of factor-analysis components with mass fractions given in Table S2 and (b)–(f) for each OA factor analysis component: (b) HOA, (c) SV-OOA, (d) LV-OOA, (e) OOA, and (f) other OA. The bin widths for each distribution are set to the standard deviations of ΔC OA, illustrating differences across OA factor-analysis components. An enthalpy of vaporization of 100 kJ/mol was assumed for all components.
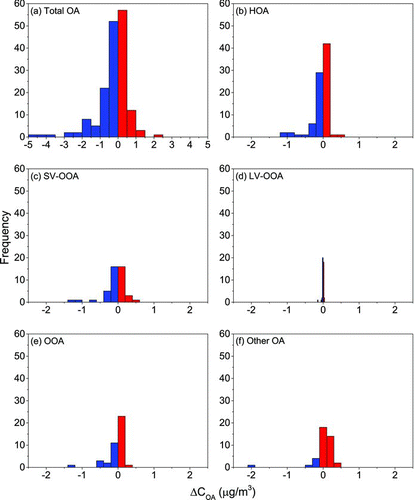
While there is uncertainty in the thermodynamic properties of ambient OA, we can speculate about which calculations of ΔC OA are most realistic. Partitioning models in which total ambient OA is represented by one or two compounds require ΔH vap values ≤ 50 kJ/mol to reproduce partitioning behavior observed in chamber studies and in the field (Donahue et al. Citation2006; Offenberg et al. Citation2006; Stanier et al. Citation2007; Cappa and Jimenez Citation2010). However, when a wider range of thermodynamic properties is considered in the representation of ambient OA (e.g., use of a volatility basis set as is the case here versus use of a two-product model) a more realistic ΔH vap of 100 kJ/mol reproduces observed partitioning behavior (Donahue et al. Citation2006; Cappa and Jimenez Citation2010). As a result, the calculations for which ΔH vap was assumed to be 100 kJ/mol are likely to be more representative of the partitioning behavior of ambient OA than those calculated assuming an ΔH vap of 50 kJ/mol. Treating ambient OA as a mixture of components derived from factor analysis of aerosol mass spectra likely provides the most realistic and robust estimate of gas-particle partitioning shifts with indoor transport. As noted above, the physiochemical properties of any particular component (e.g., HOA) are likely similar across seasons and geographic regions because each component is comprised of species that are covariant due to having common sources or formation mechanisms (Zhang et al. Citation2005a,Citationb, Citation2007, Citation2011; Lanz et al. Citation2007; Ulbrich et al. Citation2009). Notably, mass thermograms for OA components generated from TD-AMS measurements in Riverside, CA were similar to those for Mexico City, suggesting similarities in the thermodynamic properties of these OA components for regions dominated by anthropogenic OA sources (Huffman et al. Citation2009; Cappa and Jimenez Citation2010). This supports the use of these volatility distributions for a wide range of locations. However, AMS data describing the mass fractions of each OA component are also required before these volatility distributions can be applied to predict indoor concentrations of ambient OA. Greater use of the AMS to measure outdoor aerosol in exposure studies will facilitate such exposure modeling efforts.
Distributions of measurement-model disagreement and calculated values of ΔC OA are compared in . Median values of residual model error and of ΔC OA differ (note that median values of ΔC OA are near zero); however, the distributions of model error and ΔC OA are not significantly different. Thus, shifts in gas-particle partitioning provide a plausible explanation for the previously observed lack of closure. As expected based on the discussion above regarding the most realistic and robust volatility-distribution assumptions, distributions of model-measurement disagreement and ΔC OA are closest in magnitude when an ΔH vap of 100 kJ/mol is assumed () and when ambient OA is treated as a mixture of AMS factors (e.g., HOA, OOA; ).
FIG. 6 Distributions of (A) the difference between a statistical estimate of measured indoor OA of outdoor origin and indoor concentrations of ambient OA modeled accounting for only home ventilation and physical losses, (B) ΔC OA calculated assuming an ΔH vap of 100 kJ/mol, (C) ΔC OA calculated assuming an ΔH vap of 50 kJ/mol, and (D) ΔC OA calculated assuming that OA can be represented as a mixture of factor-analysis components with mass fractions given in Table S2.
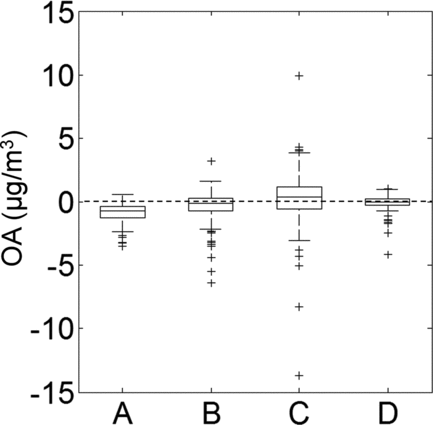
One limitation of this work is that the increased surface area in the indoor environment, compared to the outdoor environment, is not accounted for. Sorption to indoor surfaces such as carpets, wallboard, furniture, HVAC surfaces and even home occupants can be an important sink for gaseous SVOCs (Weschler and Nazaroff Citation2008) and could result in a shift of OM of outdoor origin from the particle phase toward the gas phase in order to reach a new equilibrium (Lunden et al. Citation2008; Weschler and Nazaroff Citation2008). Thus, we can conclude that interactions with indoor surfaces would result in greater evaporative losses of OA than were calculated for the RIOPA homes. Further, the importance of liquid water as a partitioning and reaction medium has been demonstrated for atmospheric aerosols (Ervens et al. Citation2011), but its role in the indoor environment has not been explored.
As noted in the Methods section, we assumed that there was no change in the thermodynamic properties of the ambient organics with outdoor-to-indoor transport (i.e., the same volatility distribution could be used to represent ambient organics before and after transport indoors). Many factors such as particle size and composition, characteristics of cracks in the building shell, human activities (e.g., opening/closing windows), and compound volatility and reactivity contribute to variability in the efficiency with which pollutants penetrate and persist indoors (Liu and Nazaroff Citation2001; Hodas et al. Citation2013). Reactive gases are expected to encounter larger losses and nonreactive gases smaller losses than particulate organics. Such differential losses may alter the volatility distribution of ambient organics. For example, depletion of the particle phase and not the gas phase would result in smaller evaporative losses or larger increases in ambient OA due sorption to indoor-generated OA than the values calculated here. It should also be noted that the timescale required for organic compounds to reach equilibrium partitioning between the gas and particle phases can vary over several orders of magnitude depending on vapor pressure (Liu et al. Citation2013). Nevertheless, in the case of PAHs, Naumova et al. (Citation2003) found that the majority of variability (84.5%) in time-averaged gas-particle partitioning indoors could be explained by PAH vapor pressure, indoor temperature, and the characteristics of the PM2.5 measured indoors (mass fractions of elemental and OC).
This article also does not consider interactions of ambient OA with gas-phase organics of indoor origin (e.g., terpenoids from cleaning products, PAHs from combustion sources; Weschler and Nazaroff Citation2004; Waring et al. 2011; Weschler 2011). Weschler and Nazaroff (Citation2008) note that the partitioning of gas-phase organics emitted or formed indoors to OA of outdoor origin could both increase the mass concentrations of the particles transported indoors and alter their chemical composition. These interactions illustrate the difficulties that could arise in separating the ambient and non-ambient contributions to OA exposure.
CONCLUSIONS
Accounting for shifts in gas-particle partitioning of ambient organics with transport into the indoor environment improved model-measurement closure. Calculated shifts resulted in changes in OA mass of between 11% and 27%, on average, depending on the assumed ΔH vap and whether OA was represented with a single volatility distribution or with a distribution generated assuming a mixture of AMS factor-analysis components. While uncertainties in the thermodynamic properties of ambient OA contribute to uncertainty in the magnitude and direction of partitioning shifts with outdoor-to-indoor transport, all calculations indicate that phase changes are important determinants of residential OA exposure. Of the calculations presented here, treatment of ambient OA as a mixture of components with distinct sources, formation mechanisms, and physiochemical properties (AMS factors, e.g., HOA, OOA) is likely to offer the most robust estimates of shifts in partitioning with outdoor-to-indoor transport across seasons and geographic regions. Expanding the spatial and temporal coverage of TD-AMS measurements, volatility distribution calculations like those presented in Cappa and Jimenez (Citation2010), and OA component mass fraction measurements will help to identify seasonal and geographic variations in OA volatility distributions and will help facilitate estimates of shifts in partitioning with outdoor-to-indoor transport.
FUNDING
This research was funded, in part, by the U.S. Environmental Protection Agency (Cooperative Agreement CR-83407201-0), NIEHS-sponsored UMDNJ Center for Environmental Exposures and Disease (NIEHS P30ES005022), and the New Jersey Agricultural Experiment Station. Natasha Hodas was supported by EPA STAR Fellowship Assistance Agreement no. FP-917336 and the Mid-Atlantic States Section of the Air and Waste Management Association (MASS-A&WMA) Air Pollution Education and Research Grant (APERG) Program. Barbara Turpin was supported in part by USDA-NIFA. The RIOPA study was supported by the Health Effects Institute (#98-23-2) and the Mickey Leland National Urban Air Toxics Center.
SUPPLEMENTAL MATERIAL
Supplemental data for this article can be accessed on.
Supplemental_Files_for_Publication.zip
Download Zip (69.9 KB)ACKNOWLEDGMENTS
We gratefully acknowledge the RIOPA study investigators, field teams, and study participants.
REFERENCES
- Cappa , C. D. and Jimenez , J. 2010 . Quantitative Estimates of the Volatility of Ambient Organic Aerosol . Atmos. Chem. Phys. , 10 : 5409 – 5424 .
- Cubison , M. J. , Alfarra , M. R. , Allan , J. , Bower , K. N. , Coe , H. McFiggans , G. B. 2008 . The Characterisation of Pollution Aerosol in a Changing Photochemical Environment . Atmos. Chem. Phys. , 6 : 5573 – 5588 .
- DeCarlo , P. F. , Kimmel , J. R. , Trimborn , A. , Northway , M. J. , Jayne , J. T. Aiken , A. C. 2006 . Field-Deploybale, High-Resolution, Time-of-Flight Aerosol Mass Spectrometer . Anal. Chem. , 78 : 8281 – 8289 .
- Docherty , K. S. , Stone , E. A. , Ulbrich , I. M. , DeCarlo , P. F. , Snyder , D. C. Schauer , J. J. 2008 . Apportionment of Primary and Secondary Aerosols in Southern California During the 2005 Study of Organic Aerosols in Riverside (SOAR-1) . Environ. Sci. Technol. , 42 : 7655 – 7662 .
- Donahue , N. M. , Robinson , A. L. , Stanier , C. O. and Pandis , S. N. 2006 . Coupled Partitioning, Dilution, and Chemical Aging of Semivolatile Organics . Environ. Sci. Technol. , 40 : 2635 – 2643 .
- Drewnick , F. , Jayne , J. T. , Canagaratna , M. , Worsnop , D. R. and Demerjian , K. L. 2004b . Measurement of Ambient Aerosol Composition During the PMTACS-NY using an Aerosol Mass Spectrometer. Part II: Chemically Speciated Mass Distributions . Aerosol Sci. Technol. , 38 : 104 – 117 .
- Drewnick , F. , Schwab , J. J. , Jayne , J. T. , Canagaratna , M. , Worsnop , D. R. and Demerjian , K. L. 2004a . Measurement of Ambient Aerosol Composition During the PMTACS-NY using an Aerosol Mass Spectrometer. Part I: Mass Concentrations . Aerosol Sci. Technol. , 38 : 92 – 103 .
- Ervens , B. , Turpin , B. J. and Weber , R. J. 2011 . Secondary Organic Aerosol Formation in Cloud Droplets and Aqueous Particles (aqSOA): A Review of Laboratory, Field and Model Studies . Atmos. Chem. Phys. , 11 : 11069 – 11102 .
- Goldstein , A. H. and Galbally , I. E. 2007 . Known and Unexplored Organic Constituents in the Earth's Atmosphere . Environ. Sci. Technol. , 41 : 1514 – 1521 .
- Hallquist , M. , Wenger , J. C. , Baltensperger , U. , Rudich , Y. , Simpson , D. Claeys , M. 2009 . The Formation, Properties and Impact of Secondary Organic Aerosol: Current and Emerging Issues . Atmos. Chem. Phys. , 9 : 5155 – 5236 .
- Hering , S. V. , Lunden , M. M. , Thatcher , T. L. , Kirchstetter , T. W. and Brown , N. J. 2007 . Using Regional Data and Building Leakage to Assess Indoor Concentrations of Particles of Outdoor Origin . Aerosol. Sci. Technol. , 41 : 639 – 654 .
- Hodas , N. , Lunden , M. , Meng , Q. Y. , Baxter , L. , Özkaynak , H. Baxter , L. K. 2012 . Variability in the Fraction of Ambient Fine Particulate Matter Found Indoors and Heterogeneity in Health Effect Estimates . J. Expo. Sci. Environ. Epidemiol. , 22 : 448 – 454 .
- Hodas , N. , Meng , Q. Y. , Lunden , M. M. and Turpin , B. J. 2014 . Toward Refined Estimates of Ambient PM2.5 Exposure: Evaluation of a Mechanistic Outdoor-to-Indoor Transport Model . Atmos. Environ. , : 229 – 236 . 83
- Huffman , J. A. , Docherty , K. S. , Aiken , A. C. , Cubison , M. J. , Ulbrich , I. M. DeCarlo , P. F. 2009 . Chemically-Resolved Volatility Measurements from Two Megacity Field Studies . Atmos. Chem. Phys. , 9 : 7161 – 7182 .
- Jimenez , J. L. , Canagaratna , M. R. , Donahue , N. M. , Prevot , A. S. H. , Zhang , Q. Kroll , J. H. 2009 . Evolution of Organic Aerosol in the Atmosphere . Science , 326 : 1525 – 1529 .
- Klepeis , N. E. , Nelson , W. C. , Ott , W. R. , Robinson , J. P. , Tsang , A. M. Switzer , P. 2001 . The National Human Activity Pattern Survey (NHAPS): A Resource for Assessing Exposure to Environmental Pollutants . J. Expo. Sci. Environ. Epidemiol. , 11 : 231 – 252 .
- Lanz , V. A. , Alfarra , M. R. , Baltensperger , U. , Buchmann , B. , Hueglin , C. and Prevot , A. S. H. 2007 . Source Apportionment of Submicron Organic Aerosols at an Urban Site by Factor Analytical Modeling of Aerosol Mass Spectra . Atmos. Chem. Phys. , 7 : 1503 – 1522 .
- Liu , C. , Shiu , S. , Weschler , C. , Zhao , B. and Zhang , Y. 2013 . Analysis of the Dynamic Interaction Between SVOCs and Airborne Particles . Aerosol Sci. Technol. , 47 : 125 – 136 .
- Liu , D. L., and Nazaroff , W. W. 2001 . Modeling Particle Penetration Across Building Envelopes . Atmos. Environ. , 35 : 4451 – 4462 .
- Lunden , M. M. , Kirchstetter , T. W. , Thatcher , T. L. , Hering , S. V. and Brown , N. J. 2008 . Factors Affecting the Indoor Concentration of Carbonaceous Aerosols of Outdoor Origin . Atmos. Environ. , 42 : 5660 – 5671 .
- Lunden , M. M. , Revzan , K. L. , Fischer , M. L. , Thatcher , T. L. , Littlejohn , D. Hering , S. C. 2003b . The Transformation of Outdoor Ammonium Nitrate Aerosols in the Indoor Environment . Atmos. Environ. , 37 : 5633 – 5644 .
- Lunden , M. M. , Thatcher , T. L. , Hering , S. V. and Brown , N. J. 2003a . The Use of Time- and Chemically-Resolved Particulate Data to Characterize the Infiltration of Outdoor PM2.5 into a Residence in the San Joaquin Valley . Environ. Sci. Technol , 37 : 4724 – 4732 .
- Meng , Q. Y. , Spector , D. , Colome , S. and Turpin , B. J. 2009 . Determinants of Indoor Exposure to PM2.5 of Indoor and Outdoor Origin During the RIOPA Study . Atmos. Environ. , 43 : 5750 – 5758.27 .
- Meng , Q. Y. , Turpin , B. J. , Lee , J. H. , Polidori , A. , Weisel , C. P. and Morandi , M. 2007 . How Does Infiltration Behavior Modify the Composition of Ambient PM2.5 in Indoor Spaces? An Analysis of RIOPA Data . Environ. Sci. Technol. , 41 : 7315 – 7321 .
- Naumova , Y. Y. , Offenberg , J. H. , Eisenreich , S. J. , Meng , Q. Y. , Polidori , A. Turpin , B. J. 2003 . Gas/Particle Distribution of Polycyclic Aromatic Hydrocarbons in Coupled Indoor/Outdoor Atmospheres . Atmos. Environ. , 37 : 703 – 719 .
- Nazaroff , W. W. 2004 . Indoor Particle Dynamics . Indoor Air , 14 : 175 – 183 .
- Offenberg , J. H. , Kleindienst , T. E. , Jaoui , M. , Lewandowski , M. and Edney , E. O. 2006 . Thermal Properties of Secondary Organic Aerosols . Geophys. Res. Lett. , 33(3) : L03816 doi: 10.1029/2005GL024623
- Pankow , J. F. 1994 . An Absorption Model of Gas/Particle Partitioning of Organic Compounds in the Atmosphere . Atmos. Environ. , 28 : 185 – 188 .
- Polidori , A. , Turpin , B. , Meng , Q. Y. , Lee , J. H. , Weisel , C. Morandi , M. 2006 . Fine Organic Particulate Matter Dominates Indoor-Generated PM2.5 in RIOPA Homes . J. Expo. Sci. Environ. Epidemiol. , 16 : 321 – 331 .
- Riley , W. J. , McKone , T. E. , Lai , A. C. K. and Nazaroff , W. W. 2002 . Indoor Particulate Matter of Outdoor Origin: Importance of Size-Dependent Removal Mechanisms . Environ. Sci. Technol. , 36 : 200 – 207 .
- Sarnat , S. E. , Coull , B. A. , Ruiz , P. A. , Koutrakis , P. and Suh , H. H. 2006 . The Influences of Ambient Particle Composition and Size on Particle Infiltration in Los Angeles, CA, Residences . J. Air Waste Manag. Assoc. , 56 : 186 – 196 .
- Stanier , C. O. , Pathak , R. K. and Pandis , S. N. 2007 . Measurements of the Volatility of Aerosols from α-Pinene Ozonolysis . Environ. Sci. Technol. , 41 : 2756 – 2763 .
- Turpin , B. J. , Weisel , C. P. , Morandi , M. , Colome , S. , Eisenreich , S. and Buckley , B. 2007 . Relationships of Outdoor Indoor and Personal Air (RIOPA): Part II. Analysis of Concentrations of Particulate Matter Species . Res. Report (Health Effects Instit.) , 130 : 79 – 92 .
- Ulbrich , I. M. , Canagaratna , M. R. , Zhang , Q. , Worsnop , D. R. and Jimenez , J. L. 2009 . Integration of Organic Components from Positive Matrix Factorization of Aerosol Mass Spectrometric Data . Atmos. Chem. Phys. , 9 : 2891 – 2918 .
- Waring , M. S. , Wells , J. R. and Siegel , J. A. 2011 . Secondary Organic Aerosol Formation from Ozone Reactions with Single Terpenoids and Terpenoid Mixtures . Atmos. Environ , 45 : 4235 – 4242 .
- Weimer , S. , Drewnick , F. , Hogrefe , O. , Schwab , J. J. , Rhoads , K. Orsini , D. 2006 . Size-Selective Nonrefractory Ambient Aerosol Measurements During the Particulate Matter Technology Assessment and Characterization Study–New York 2004 Winter Intensive in New York City . J. Geophys. Res. , 111 : D18305 doi: 10.1029/2006JD007215
- Weisel , C. P. , Zhang , J. , Turpin , B. J. , Morandi , M. T. , Colome , S. Stock , T. H. 2005 . Relationship of Indoor, Outdoor and Personal Air (RIOPA) Study: Study Design, Methods and Quality Assurance/Control Results . J. Expo. Sci. Environ. Epidemiol , 15 : 123 – 137 .
- Weschler , C. J. 2011 . Chemistry in Indoor Environments: 20 Years of Research . Indoor Air , 21 : 205 – 218 .
- Weschler , C. J and Nazaroff , W. W. 2004 . Cleaning Products and Air Fresheners: Exposure to Primary and Secondary Air Pollutants . Atmos. Environ. , 38 : 2841 – 2865 .
- Weschler , C. J and Nazaroff , W. W. 2008 . Semivolatile Organic Compounds in Indoor Environments . Atmos. Environ. , 42 : 9018 – 9040 .
- Zhang , Q. , Alfarra , M. R. , Worsnop , D. R. , Allan , J. D. , Coe , H. Canagaratna , M. R. 2005a . Deconvolution and Quantification of Hydrocarbon-Like and Oxygenated Organic Aerosols Based on Aerosol Mass Spectrometry . Environ. Sci. Technol. , 39 : 4938 – 4952 .
- Zhang , Q. , Jimenez , J. L. , Canagaratna , M. R. , Allan , J. D. , Coe , H. Ulbrich , I. 2007 . Ubiquity and Dominance of Oxygenated Species in Organic Aerosol in Anthropogenically-Influenced Northern Hemisphere Midlatitudes . Geophys. Res. Lett. , 34 ( 13 ) doi: 10.1029/2007GL029979
- Zhang , Q. , Jimenez , J. L. , Canagaratna , M. R. , Ulbrich , I. M. , Ng , N. L. Worsnop , D. R. 2011 . Understanding Atmospheric Organic Aerosols via Factor Analysis of Aerosol Mass Spectrometry: A Review . Anal. Bioanal. Chem. , 401 : 3045 – 3067 .
- Zhang , Q. , Worsnop , D. R. , Canagaratna , M. R. and Jimenez , J. L. 2005b . Hydrocarbon-Like and Oxygenated Organic Aerosols in Pittsburgh: Insights into Sources and Processes of Organic Aerosols . Atmos. Chem. Phys. , 5 : 3289 – 3311 .