Abstract
Measurements of submicron particulate matter (PM) were performed at an urban background station, in Helsinki, Finland during wintertime to investigate the chemical characteristics and sources of PM1. The PM1 was dominated by sulfate and organics. The source apportionment indicated that organic aerosol (OA) was a mixture from local sources (biomass burning (BBOA), traffic, coffee roaster (CROA)), secondary compounds formed in local wintertime conditions (nitrogen containing OA (NOA), semivolatile oxygenated OA (SV-OOA), and regional and long-range transported compounds (low volatile oxygenated OA, LV-OOA). BBOA was dominated by the fragments C2H4O2 + and C3H4O2 + (m/z 60.021 and 73.029) from levoglucosan, or other similar sugar components, comprising on average 32% of the BBOA mass concentration. The ratio between fragments C2H4O2 +/C3H4O2 + was significantly lower for CROA (=1.1) when compared to BBOA (=2.1), indicating that they consisted of different sugar compounds. In addition, a component containing substantial amount of nitrogen compounds (NOA) was observed in a sub-arctic region for the first time. The NOA contribution to OA ranged from 1% to 29% and elevated concentrations were observed when ambient relative humidity was high and the visibility low. Low solar radiation and temperature in wintertime were observed to influence the oxidation of compounds. A change in aerosol composition, with an increase of LV-OOA and decrease in BBOA, SV-OOA and NOA was noticed during the transition from wintertime to springtime. Size distribution measurements with high-time resolution enabled chemical characterization of externally mixed aerosol from different sources. Aged regional long-range transported aerosols were dominant at around 0.5 μm (vacuum aerodynamic diameter), whereas traffic and CROA emissions dominated at around 120 nm.
Copyright 2014 American Association for Aerosol Research
INTRODUCTION
Fine particle aerosols have been intensively investigated during the last decades due to their impacts on climate and human health (Nel Citation2005; IPCC Citation2007). Changes in the Arctic are accelerating the pace of global warming (IPCC Citation2007) and, therefore, the impact of close-to-arctic urban areas to changes in the albedo, carbon and nitrogen biogeochemical cycles may be relevant. In this context, information regarding the chemical composition of fine particles is important in those areas permanently or seasonally covered by snow. For example, Sand et al. (Citation2013) found that black carbon (BC) emitted within the Arctic has an Arctic surface temperature response (per unit of emitted mass) almost five times higher than in mid-latitudes. The metropolitan area of Helsinki, with 1.3 million inhabitants, is located in the sub-arctic region (60°12′N, 24°58′E) at the shore of the Baltic Sea and south of the Boreal forest region. The climate of Helsinki has typical sub-arctic characteristics during the wintertime with average air temperature from January to March of −4.2°C, global solar radiation (sum of direct and diffuse radiation) of 118 MJ m−2 and only 81 h of sunshine (Pirinen et al. Citation2012). This leads to very limited photochemical activity. Moreover, poor air mixing, often atmospheric stable conditions, high vehicle emissions, e.g., due to cold engine starts, and residential heating (from wood, and to smaller extent oil) are factors that affect the particulate matter (PM) concentrations and processes in atmospheric aerosol chemistry.
To evaluate PM impacts on climate change it is important to collect information about the PM composition, size distribution (SD), and concentration. In traditional filter collections the poor time resolution, typically 24–72 h (Pakkanen et al. Citation2001; Sillanpää et al. Citation2005), has limited the information that can be extracted. Also, due to poor time resolution in filter collections source analysis is hard to accomplish and only few studies have provided detailed information about the PM sources in sub-arctic areas. Especially, organic and organic-nitrogen related sources in sub-arctic urban areas are poorly known.
Typical annual average of PM1 levels in Helsinki is 7.2 ± 3.2 μg m−3 and that is dominated by organic carbon (OC) and sulfate from local and long-range transported (LRT) sources (Saarikoski et al. Citation2008). An Aitken mode has been previously related to local origin; however, a detailed investigation of the chemical composition and the sources of this mode was not available (Sillanpää et al. Citation2005).
In this study, high-time resolution measurements were carried out using the Aerodyne High-Resolution Time-of-Flight Aerosol Mass Spectrometer (HR-ToF-AMS) to characterize submicron aerosol during the wintertime 2009 in Helsinki, Finland. The aim of this study was to elucidate sources, SD, and transformation processes of fine PM in sub-arctic conditions. Real-time measurements of aerosol chemical composition enabled source analysis and the comparison of the trends in aerosol chemistry with those of gas phase compounds. Additionally, the size-dependent chemistry measurements were used to obtain information about the sources of chemical species in submicron aerosol.
EXPERIMENTAL
Site Description and Ancillary Data
The instrumentation operated from January 9th to March 13th 2009 at an urban background station (Station for Measuring Ecosystem-Atmosphere Relations, SMEAR III, 26 m above mean sea level; Järvi et al. (Citation2009)) in Helsinki, Finland. The site is located 5 km northeast from the center of Helsinki and 200 m east from a major road (42,000 vehicles/day). At SMEAR III trace gases such as ozone (O3), nitrogen oxides (NO, NO2), carbon monoxide (CO), and sulfur dioxide (SO2) are measured continuously. In addition, meteorological parameters (wind direction and speed, visibility, air temperature, and relative humidity (RH)) are measured in the same location by the Finnish Meteorological Institute.
The period of this study represented most of the time mid-winter conditions with permanent snow cover. The average temperature over the period was −2.8°C ± 3.7 with minimum and maximum values of −16.5 and 5.3°C, respectively. Most of the time RH was higher than 80% and the dominant wind direction was northeast. The meteorological data are summarized in Figure S1.
High-Resolution Time-of-Flight Aerosol Mass Spectrometer
The Aerodyne High-Resolution Time-of-Flight Aerosol Mass Spectrometer (Aerodyne Research Inc., Billerica, MA, USA; DeCarlo et al. (Citation2006), hereinafter referred to as “AMS”) has been widely used in laboratory and field experiments to study sub-micron PM. The AMS is able to determine major nonrefractory fine PM species, such as nitrate, sulfate, ammonium, chloride, and organics, with high-time resolution. In addition, the high-time resolution of the instrument enables measurements of size-resolved chemical composition of submicron non-refractory aerosol (Jayne et al. Citation2000). In the AMS, aerosol particles are sampled through aerodynamic lens, forming a narrow particle beam which is transmitted into the detection chamber where nonrefractory species are flash vaporized upon impact on a hot surface (∼600°C) under high vacuum and are chemically analyzed after electron impact ionization (70 eV) in the high-resolution time-of-flight mass spectrometer. In this study, a measurement interval of 5 min was adopted, alternating between mass spectrum (V and W modes with 2 min each) and SD mode (PToF, 1 min). The latter was normalized by the mass spectrum mode. The V mode is more sensitive to lower concentrations, and the W mode has higher resolution to separate different ions. All the data presented in this paper were collected in V-mode. Concerning the sampling line a cyclone was used to cut-off particles larger than 1 μm. The sampling was made using a separate pump and one l min−1 flow rate to ensure short transport time to the AMS sample intake.
AMS Data Analysis
In this study, data from the AMS were analyzed with the software packages Squirrel v. 1.52C and Pika v. 1.11C (Sueper, Citation2008) within Igor Pro 6 (Wavemetrics, Lake Oswego, OR, USA). The organic aerosol (OA) fraction was further investigated using the Positive Matrix Factorization (PMF2; Paatero and Tapper Citation1994) version 4.2 in the robust mode and the custom software tool PMF evaluation panel version 2.6 developed and described by Ulbrich et al. (Citation2009), which enabled evaluation of the PMF results together with several statistical analyses.
Collection Efficiency
The collection efficiency (CE) in the AMS is defined as the ratio of the mass of particles detected by the instrument to the mass of particles introduced to the AMS inlet. Several studies have shown that CE depends on the lens transmission, particle shape and particle phase (Alfarra et al. Citation2004; Huffman et al. Citation2005; Matthew et al. Citation2008). These studies and the study of beam width probe by Salcedo et al. (Citation2007) indicate that the particle phase (solid vs. liquid) is the dominant term in determining particle bounce at the vaporizer and CE. Middlebrook et al. (Citation2012) reported that RH in sampling line, acidity/neutralization of sulfate, ammonium nitrate content, and organic liquid content are the main factors influencing particle phase, and proposed a parameterization to estimate CE for ambient AMS data.
In this study, CE was calculated with the parameterization of Middlebrook et al. (Citation2012), which takes into account the chemical composition of particles. The RH in the sampling line was not measured and therefore the current values of CE do not contain this correction. However, the RH in the sampling line can be estimated using the ratio between water vapor pressure (p) and the saturated water vapor pressure (p s) at ambient temperature and temperature inside the container. The value of p was calculated based on the highest outside water vapor pressure (T = 3.7°C and RH = 97%) and the p s was calculated assuming constant air temperature inside the container at 18°C. The ratio serves as an upper estimated level for RH in the sampling line, which in this case is 37%. At this RH liquid water on the particles does not affect CE (Middlebrook et al. Citation2012). The calculated CE varied between 0.50 and 0.91 and is presented in Figure S1. All the AMS mass concentrations shown in this paper are calculated using these CE values.
Other Measurements
Measurement of the total mass concentration of submicron aerosol particles was performed with the tapered element oscillating microbalance (TEOM, model 1400ab; Green et al. Citation2008) and gravimetric analysis with filter samples. OC and elemental carbon (EC; hereafter called BC as it was measured optically) were measured using the semi-continuous carbon analyzer (Sunset Laboratory Inc., Portland, OR) and inorganic ions with the Particle-into-Liquid-Sampler (PILS; Metrohm Peak Inc; Orsini et al. Citation2003) coupled with two ion chromatographs (Saarikoski et al. Citation2008). The SD of aerosol particles was measured using a differential mobility particles sizer (DMPS) (Järvi et al. Citation2009).
AMS data were compared with the results of PILS, Sunset OC/EC analyzer, TEOM, manual gravimetry, and DMPS. In general, the comparisons presented good agreement (R >0.8) for most of the instruments (Table S1). Additional information about the instrumental comparison is shown in the supplemental information (SI).
RESULTS AND DISCUSSION
Overview of Aerosol Chemical Composition
During the measurement period from January to March, 2009 the PM1 concentration ranged between 1 and 28 μg m−3. PM1 was composed equally of OA (33%) and sulfate (33%), followed by ammonium (12%), nitrate (11%), and BC (11%). The average total PM1 (nonrefractory + BC mass) over the period (6.9 μg m−3) was similar to what was reported in the study of Saarikoski et al. (Citation2008). The acidity of the particles was investigated through the ratio of stoichiometric neutralization (Zhang et al. Citation2007). The NH4 + meas/NH4 + neu ratio was <0.75 67% of the studied period suggesting that particles presented acidic character most of the time. Ion measurements (Figure S3) indicated that most of the sulfate in the particles was in the form of ammonium bisulfate (NH4HSO4) (Figure S4). During the measurement period a few episodes were observed when sulfate was in the form of sulfuric acid (H2SO4). During the episodes, CE was close to one. For example, during the episode observed on January 21st the total PM1 measured by the AMS with a default CE of 0.5 would have been overestimated by a factor of 2 (32.4 μg m−3, instead of 15.7 μg m−3).
Characterization of OA Components
PMF was used to identify different components of OA. Six factors were needed to chemically characterize different OA components, low-volatility oxygenated OA (LV-OOA), semi-volatile oxygenated OA (SV-OOA), nitrogen-containing OA (NOA), coffee roastery OA (CROA), biomass burning OA (BBOA), and hydrocarbon-like OA (HOA) (Figure S2).
FIG. 1 Average diurnal profiles of the organics, sulfate, nitrate, ammonium, and BC from the AMS (a), trace gases SO2, NO, NO2, CO, and O3 (b), and OA components (c) over the campaign period.
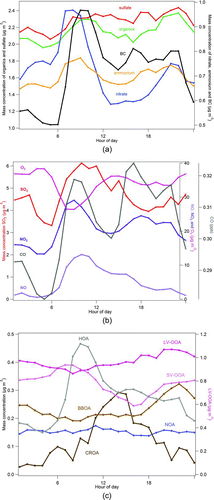
LV-OOA comprised 48% of OA on average being the most dominant component. LV-OOA is typically related to aged and oxidized particles (Jimenez et al. Citation2009). In this study, LV-OOA resulted from two factors dominated by the oxygenated fragment CO2 +, however, with different fractions, 13% and 24% each, and also different fractions of less oxidized fragments like CHO+ and C2H3O+. The presence of similar type of OA particles with different amounts of oxygenated fragments in the same air masses could indicate that LV-OOA is from different origins, for instance, one more local and another LRT, of which the first represents 55% and the second 45% of the total LV-OOA mass. The time series of both LV-OOAs were very similar with a correlation of 0.91 (r, Pearson correlation) and therefore the two LV-OOA factors were recombined.
LV-OOA had a good agreement with sulfate, which is most likely aged regional or LRT from eastern European countries, because of rather efficient SO2 removal from the local sources (coal combustion). Moreover, the origin of the air masses during the periods when LV-OOA and sulfate were elevated (February 19th–25th, March 5th–9th) was confirmed by HYSPLIT (Draxler and Hess Citation1998) backward air trajectories (Figure S7) and the simulations with the chemical transport model System for Integrated modeling of atmospheric composition (Sofiev et al. Citation2006, not shown here). The diurnal profile of LV-OOA is rather stable and the lack of a clear diurnal variation supports the assumption of the regional or long-rang transport origin of this component (). The diurnal behavior of sulfate was similar to that of LV-OOA. LV-OOA was the most oxidized factor with O:C and OM:OC ratios of 0.73 and 2.09, respectively (Table1).
SV-OOA represented 16% of OA, on average. This component is similar to SV-OOA described by Timonen et al. (Citation2013) and correlates well (r = 0.91) with the average SV-OOA presented by Ng et al. (2010; differences inside the standard deviation). In springtime SV-OOA was speculated to be related to biogenic emission from the boreal forest (Timonen et al. Citation2013); however, this is unlikely in the current study due to the sub-arctic winter conditions (Hakola et al. Citation2012). Therefore SOA formation from anthropogenic emissions such as traffic or heating emissions may be responsible for observed SV-OOA. SV-OOA was much less oxidized than LV-OOA.
The third identified component representing 14% of OA is HOA. HOA is often associated with traffic emissions based on similarities between the mass spectra, both dominated by hydrocarbon fragments and elevated concentrations during the rush hours (Canagaratna et al. Citation2004). Also in this study, the elevated concentrations from 7:00–11:00 h in the morning were observed for HOA, BC, and CO, all products of incomplete fuel combustion. The HOA morning peak was observed at the diurnal profile of organics (). The broadening of organics morning peak was a consequence of the combination of morning rush hour and stable meteorological conditions, low air temperatures, and wind speeds, which hamper the dispersion of pollutants. HOA had the lowest O:C and highest H:C ratio of all OA components ().
Biomass burning is used for domestic heating and saunas in Finland in wintertime (Saarnio et al. Citation2012). During this winter campaign, the contribution of BBOA ranged between 1% and 39% of OA, being 12% on average. The diurnal profile presented a clear increase in the evening hours from 20:00 to 22:00 h (), which was likely a consequence of wood burning in domestic heating appliances and sauna stoves (Saarnio et al. Citation2012). During the same hours, CO and BC, which have also been related to incomplete combustion of wood, had elevated concentrations. Moreover, when the mass concentration of BBOA was averaged according to the filter sampling times (PM1) a very good correlation with the sum of the biomass burning tracer anhydrosugars (MAs: levoglucosan, mannosan, and galactosan) was achieved (R = 0.92, ). Of the two BBOA factors reported by Timonen et al. (Citation2013), the mass spectrum obtained for BBOA in this study is more similar to local BBOA. However, in this study BBOA was less oxidized than local BBOA in Timonen et al. (Citation2013) suggesting the proximity to the source, which supports the assumption of its local character.
TABLE 1 Elemental analysis ratios for OA components
FIG. 2 Mass concentration of BBOA time series averaged into MAs filter sampling time (MAs presented previously in Saarnio et al. [Citation2012]).
![FIG. 2 Mass concentration of BBOA time series averaged into MAs filter sampling time (MAs presented previously in Saarnio et al. [Citation2012]).](/cms/asset/b507f65b-7b7b-429e-bafb-d37668e3e0d8/uast_a_875115_o_f0002g.jpg)
In this study the MAs, which are dominated by levoglucosan (86% on average), comprised 32% of the BBOA mass concentration (). This contribution was lower than that obtained in the Po Valley (61%, Saarikoski et al. Citation2012); however, it was significantly higher than in the Mexico City (6%, Aiken et al. Citation2009). In the Po Valley, the authors explained that the reason for such an elevated levoglucosan contribution was the separation of the oxidized fraction of BBOA into a different component (OOA-c). In Mexico City, the meteorological situation (elevated air temperatures and solar radiation) provided better conditions for the levoglucosan decomposition that may explain the lower contribution to BBOA.
The OM:OC (1.39) and O:C (0.21) ratios for BBOA are lower in this study () than in Po Valley, Mexico City and Helsinki in springtime. That is likely due to the winter conditions which hamper the oxidation of biomass burning particles as well as the proximity of biomass burning sources (mostly local biomass burning).
OA component containing substantial amount of nitrogen fragments (NOA) was observed in a sub-arctic region for the first time. The concentration of NOA ranged from 0.1 (1%) to 1.5 (29%) μg m−3 representing on average 7.5% of OA. The NOA mass spectrum contains fragments, such as CHN+, CH4N+, CH5N+, C2H4N+, and C3H8N+, which are responsible for 5% of the NOA total signal and N:C ratio of 0.03 (). In addition, the fragments CHO+, C2H3O+,
FIG. 3 Increase of the NOA component mass concentration as a function of the RH, colored by the visibility.
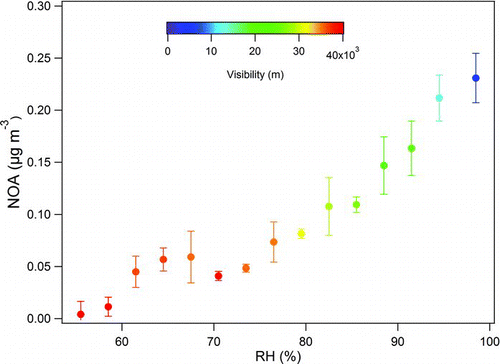
OA component containing nitrogen fragments in the Mexico City has been reported to be related to industrial sources, however, with the lower ratios of OM:OC and O:C (1.40 and 0.13; Aiken et al. (Citation2009)). In New York, Sun et al. (Citation2011) reported a NOA component with OM:OC, O:C and N:C ratios of 1.69, 0.37 and 0.05, respectively. In the same study the authors observed a clear diurnal profile for NOA with higher concentrations in the middle of the day and they related this component to marine origin. Although the atomic ratios obtained in this study (OM:OC 1.67, O:C 0.35 and N:C 0.03) are similar to New York, no diurnal profile was observed. Moreover, there is no significant algae activity from the Baltic Sea during wintertime conditions (Leck et al. Citation1990). In the Po Valley, Italy, Saarikoski et al. (Citation2012) described a NOA component with similar OM:OC and O:C ratios (1.5 and 0.28) but a much higher N:C ratio (0.08). Interestingly, the authors observed that the nocturnal surface layer break-up caused large changes in NOA concentrations, usually in the late morning hours, resulting in a clear diurnal profile of NOA. Mass spectrum shows that these NOA components found in different locations have different fragments and likely different chemical composition.
The last component identified by the PMF analysis was the local coffee roastery OA (CROA). This component has been identified and described previously by Timonen et al. (Citation2013) in Helsinki in springtime. Both mass spectra are very similar with the typical caffeine molecular fragments at C2H4N+, C4H7 +, C3H3N2 +, C6H9 +, C6H10 +, and C7H9O+, in agreement with the NIST Chemistry WebBook (http://webbook.nist.gov/chemistry). The typical biomass burning fragments, C2H4O2 + and C3H4O2 +, were also identified in this mass spectrum, however with a smaller fraction than in BBOA. Moreover, the ratio between C2H4O2 + and C3H4O2 + is different for BBOA and CROA, BBOA presents a ratio of 2.1 for C2H4O2 + to C3H4O2 +that is typical for biomass burning sources (Cubison et al. Citation2011) whereas CROA presents a ratio of 1.1. Different ratios suggested that other sugars may produce the same fragments as cellulose. In fact, the coffee beans are expected to contain also polysaccharides such as arabinolactosan, which may produce different fragmentation pattern. Also different temperatures involved in the burning of biomass and the process of roasting (typically 180–300°C) may have an effect on fragmentation pattern.
Timonen et al. (Citation2013) observed CROA mass concentration up to 3 μg m−3 when a coffee roastery, located 2 km southwest of the station, was directly upwind of the site (∼210°). Similar results were obtained in this study (Figure S5); however, another coffee roastery, located 10 km east of the station (∼90°), contributed to the CROA mass concentration up to 1 μg m−3 this time. Because the second coffee roastery was further from the measurement station there was more time for particles to be oxidized and, therefore, to have an increased O:C ratio. Further information related to the size of those particles is discussed below.
OA and the Number of Hours of Sunshine
During the measurement period the average OM:OC ratio was 1.56 ± 0.12, which is 11% lower than obtained in springtime in the same year (1.74 ± 0.12; Timonen et al. Citation2013). That is most likely due to the different meteorological conditions of each season. For instance, in May in Helsinki, there are on average seven times more sunshine hours than in January (Pirinen et al. Citation2012). During the wintertime campaign, no significant changes in the meteorological parameters, such as in air temperature, were observed (Figure S1); however, the number of hours of sunshine in March was three times higher than in January. The number of sunshine hours was probably the reason why the composition of OA changed in the course of the campaign. For instance, when comparing the first and last 15 days of the campaign an increase of LV-OOA from 40% to 58% was observed. Moreover, a decrease in BBOA from 15% to 8%, SV-OOA from 20% to 16% and NOA from 7% to 3% were also observed. These results indicated the importance of the number of hours of sunshine in the oxidation process of the OA. The presence of sunshine and therefore solar radiation is responsible for production of strong atmospheric oxidants, such as ozone and OH radical, which are related to OA oxidation state increase.
Another evidence of the reduced hours of sunshine was the clear anti-correlation between the trace gases NO2 and O3 (). The anti-correlation suggested that besides direct emission from traffic, NO2 was also a product from the chemical reaction between the trace gases NO and O3 (Seinfeld and Pandis Citation1998), of which O3 was likely transported from the upper troposphere. This chemical reaction is dominant throughout the day in places where the amount of light is limited and photochemistry is weak (Anttila et al. Citation2012).
TABLE 2 Main parameters used in each mode of the three episodes
Size Distributions
AMS enables the real time determination of size-resolved chemical composition of different particle modes and their origin. To investigate particle SDs for different PM sources, three episodes were selected. Episode 1 (from 6 February 20:00 h to 7 February 06:00 h) represented typical aerosol SD for this location (Timonen et al. Citation2008). Episode 2 (12 February 6:30 h to 13:30 h) represented traffic emissions and episode 3 (3 March 14:20 h to -15:40 h) coffee roastery emissions. Because no SD of BC was available, the fragment of m/z 57 (mainly saturated hydrocarbon C4H9 +) from the AMS was used as a surrogate of BC (Figure S6).
Episode 1
The first episode represented the average winter conditions; air temperature was around 0°C, RH above was 90%, dominant wind direction was southeast, and the backward air mass trajectories showed that the air masses arrived in Helsinki from Eastern Europe, suggesting a high contribution of LRT pollutants.
When a log-normal distribution (using the algorithm by Winklmayr et al. Citation1990) was fitted to the SD of episode 1, an accumulation mode centered at 510 nm (D va , geometric standard deviation σ = 1.70, ) was found for all the constituents (), suggesting that aerosol particles were internally mixed. DMPS SD had an accumulation mode centered at 589 nm (σ = 1.71). The difference in the mode center between the two instruments was small; however, the difference in the observed PM mass was substantial. During this episode the AMS+BC mass corresponded to only 60% of the calculated DMPS mass (). Several reasons could contribute to such discrepancy and are discussed in the SI.
Episode 2
The second episode represented traffic emissions. The meteorological conditions were similar to episode 1, except for the wind direction, which was from northeast (). The SD () had a clear lower mode centered at 122 nm (σ = 1.42), and the accumulation mode centered at 440 nm (σ = 1.60). The lower mode was dominated by nitrate (36%) followed by organics (20%). The presence of ammonium (17%) and very minor amount of sulfate indicated that the lower mode was likely a result from the reaction between nitric acid (HNO3) and ammonia (NH3), which corresponded to the early morning rush hour ammonium nitrate peak (7:00–9:00 h, ). Ammonium nitrate formation depends on the availability of NH3 and sulfate.
The accumulation mode was dominated by sulfate and it contained only a small amount of nitrate (6%) suggesting different PM source than in the lower mode. During the episode 2 also, the SD of the DMPS data showed two separate modes (130 and 470 nm). The high nitrate fraction was likely responsible for the elevated density of the lower mode (1.61 g cm−3). Despite the elevated amount of sulfate in the accumulation mode, the average density was 1.57 g cm−3. This value was slightly lower than in the lower mode probably due to the elevated amount of organics (29%), which based on the O:C and H:C ratios have an average density of 1.12 g cm−3. The low organic density for this period was likely a consequence of the strong traffic emissions during the morning rush hours. Similar to the episode 1, the mass in the accumulation mode for the DMPS was about 30% larger than that of AMS. Besides the reasons discussed in the SI, it is possible that the high nitrate fraction in the lower mode was forcing particles toward liquid phase. Once in the liquid phase, the particles would stick on the heater vaporizer and increase the CE. This fact suggested that externally mixed aerosol particles may need different CE for different particle sizes (Middlebrook et al. Citation2012).
Episode 3
The third episode represented emissions from the local coffee roastery. Although, in terms of SD, this episode looked similar to episode 2 with two modes; one centered at 124 nm (σ = 1.39) and another at 477 nm (σ = 1.64); however, the chemical composition of those modes differs (). The lower mode was dominated by organics (59%) and BC (26%). The average mass spectrum of organics in this mode presented, besides the typical hydrocarbons found in traffic emission (HOA), typical m/z's from CROA (m/z 67, 82, 109, and 194; Figure S8). In addition, during this episode the ratio between the m/z 194 (caffeine molecule) and OA (f194) increased abruptly and coincided with the change in wind direction (). The fraction f194 was used as CROA tracer ().
The SD of CROA (SDCROA) can be obtained using the three-dimensional factorization model proposed by Ulbrich et al. (Citation2012). However, because the main fragments of this source (e.g., m/z 67, 82, 109, and 194) represented a significant fraction of this component (15% for the first three), a simpler approach is proposed here based on the CROA mass spectrum and SD data of the main fragments. In order to obtain the average SD of each fragment (SDxx) the aerosol background concentration, which is present mainly in the accumulation mode, was removed using the average SD of each fragment for the periods straight before (A xx(t−1)), during (A xx(t)) and straight after (A xx(t+1)) the episode. In addition, the respective average OA concentrations were used as shown in EquationEquation (1).
Where A xx(t), A xx(t−1), and A xx(t+1) represent the average SD of m/z xx scaled according to the CROA mass spectrum obtained by the PMF solution. OA(t−1) and OA(t+1) represent the average mass concentration of OA before and after the episode. Each period comprehended 1:30 h. Hence, the CROA average SD was calculated as shown in EquationEquation (2).
FIG. 5 Mass size distributions before, during, and after episode 3 for the main fragments of CROA and the average size distribution of CROA.
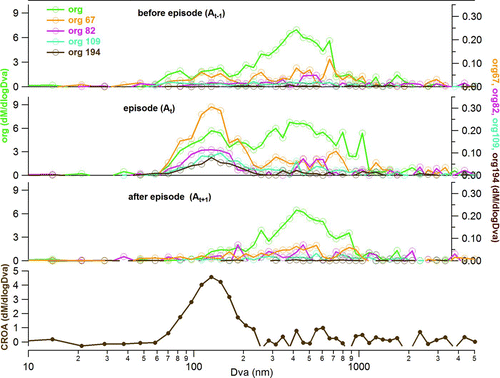
The presence of stable concentration of BC during the whole episode suggested that this compound might have a different source. Those emissions were probably from the traffic in Helsinki city center throughout the day.
The same two modes were observed by the DMPS, one centered at 522 nm and with about 30% more mass than on the basis of AMS measurement, and a second mode at 156 nm. Similar to the lower mode found in episode 2, also here, the mass obtained by the AMS was much higher. However, in this episode the fractions of nitrate and sulfate, ions typically responsible for particle acidity, were small. Therefore, we speculate that the high amount of CHN+ and CHNO+ fragments, present in the caffeine molecule, may cause phase transform to liquid particles and therefore an increase in the CE. This assumption implies in an overestimation in the mass of the lower mode of the AMS when the bulk CE is estimated according to Middlebrook et al. (Citation2012) without taking into account its size dependence.
CONCLUSIONS
Submicron aerosol particles were dominated by OA and sulfate in Helsinki in winter and the data showed that most of the sulfate and part of OA were aged regional or long-ranged transported. This result was supported by the highly oxidized LV-OOA component, which dominated OA and correlated with sulfate concentrations. Besides the OA components typically identified in fine PM (LV-OOA, SV-OOA, BBOA, and HOA) two factors containing nitrogen fragments (NOA and CROA) were found. NOA formation seemed to occur during foggy conditions, when RH was high and visibility low. The presence of fog and low air temperature may have facilitated low-molecular weight and highly water-soluble nitrogen-containing molecules, such as alkyl amines, to remain in the particulate phase. In addition, the emissions from two nearby coffee roasteries were observed. CROA had clearly different C2H4O2 + and C3H4O2 + - ratio (1.1) when compared to BBOA (2.1), suggesting that also other sugars (e.g., polysaccharides) may produce the same fragments as MAs.
Highly time-resolved data of SD measurements enabled the characterization of chemical composition of externally mixed particles where different size modes originated from different sources. SDs of three different episodes were investigated for the chemical composition and oxidation state in order to obtain information on different sources. SDs had typically accumulation mode representing aged long-range transported PM and in two cases also second mode in smaller size consisting of local emissions (traffic and coffee roastery). During the periods when traffic influences the PM, e.g., the early morning rush hour, externally mixed aerosol with an accumulation mode and a lower mode around 120 nm with a very distinct chemical composition were observed. The comparison between the AMS and DMPS confirmed that both instruments are able to detect at least two separate modes. The SD data showed that the difference is not only due to the lens transmission in the AMS, but it was present all over the size bins measured by both the instruments. In order to understand better the aerosol processes and sources in detail, the use of chemically resolved SD information is important and should be explored more in future studies.
High-resolution AMS measurements provided important information about sources and transformation of PM in sub-arctic locations. Wintertime conditions with low solar radiation and temperature were observed to influence the oxidation of compounds. A change in aerosol composition, with an increase of LV-OOA and decrease in BBOA, SV-OOA and NOA was observed during the transition from wintertime to springtime. The results exemplified the importance in correcting the AMS data with the CE due to the acidity, especially regarding sulfate, which is elevated most of the time during winter in Helsinki. In addition, different CE may be needed for the different aerosol modes.
FUNDING
Financial support from the Helsinki Energy, Ministry of Traffic and Communications, TEKES via the CLEEN MMEA-programme, Graduate School in Physics, Chemistry, Biology, and Meteorology of Atmospheric Composition and Climate Change (University of Helsinki), and Cryosphere-Atmosphere Interactions in a Changing Arctic Climate (CRAICC) is gratefully acknowledged. Ingrid M. Ulbrich and Jose L. Jimenez were supported by DOE (BER/ASR) DE-SC0006035.
SUPPLEMENTAL MATERIAL
Supplemental data for this article can be accessed on.
Suppl_WinterChem_3.zip
Download Zip (2.4 MB)REFERENCES
- Aiken , A. C. , Salcedo , D. , Cubison , M. J. , Huffman , J. A. , DeCarlo , P. F. Ulbrich , I. M. 2009 . Mexico City Aerosol Analysis During MILAGRO using High Resolution Aerosol Mass Spectrometry at the Urban Supersite (T0) - Part 1: Fine Particle Composition and Organic Source Apportionment . Atmos. Chem. Phys. , 9 : 6633 – 6653 .
- Alfarra , M. R. , Coe , H. , Allan , J. D. , Bower , K. N. , Boudries , H. Canagaratna , M. R. 2004 . Characterization of Urban and Rural Organic Articulate in the Lower Fraser Valley using Two Aerodyne Aerosol Mass Spectrometers . Atmos. Environ. , 38 : 5745 – 5758 .
- Anttila , P. , Rantamäki , M. , Orava , H. and Niemi , J. V. 2012 . Characteristics of Wintertime Pollution in Various Northern European Urban Environments , 206 Hertfordshire , , UK : University of Hertfordshire .
- Canagaratna , M. R. , Jayne , J. T. , Ghertner , D. A. , Herndon , S. , Shi , Q. Jimenez , J. L. 2004 . Chase Studies of Particulate Emissions from in use New York City Vehicles . Aerosol. Sci. Technol. , 38 : 555 – 573 .
- Cubison , M. J. , Ortega , A. M. , Hayes , P. L. , Farmer , D. K. , Day , D. Lechner , M. J. 2011 . Effects of Aging on Organic Aerosol from Open Biomass Burning Smoke in Aircraft and Laboratory Studies . Atmos. Chem. Phys. , 11 : 12049 – 12064 .
- DeCarlo , P. F. , Kimmel , J. R. , Trimborn , A. , Northway , M. , Jayne , J. T. Aiken , A. C. 2006 . Field-Deployable, High-Resolution, Time-of-Flight Aerosol Mass Spectrometer . Anal. Chem. , 78 : 8281 – 8289 .
- Draxler , R. R. and Hess , G. D. 1998 . “ An Overview of the HYSPLIT_4 Modeling System of Trajectories, Dispersion, and Deposition ” . In Aust. Meteor. Mag. Vol. 47 , 295 – 308 .
- Farmer , D. K. , Matsunaga , A. , Docherty , K. S. , Surratt , J. D. , Seinfeld , J. H. Ziemann , P. J. 2010 . Response of an Aerosol Mass Spectrometer to Organonitrates and Organosulfates and Implications for Atmospheric Chemistry . PNAS , 107 : 6670 – 6675 .
- Green , D. , Baker , T. and Fuller , G. 2008 . Volatile Correction Model (VCM) for PM10 Application to Hourly Time Resolution and AURN FDMS Purge Measurements , 64 England : King's College London .
- Hakola , H. , Hellén , H. , Hemmilä , M. , Rinne , J. and Kulmala , M. 2012 . In Situ Measurements of Volatile Organic Compounds in a Boreal Forest . Atmos. Chem. Phys. , 12 : 11665 – 1678 .
- Huffman , J. A. , Jayne , J. T. , Drewnick , F. , Aiken , A. C. , Onasch , T. Worsnop , D. R. 2005 . Design, Modeling, Optimization, and Experimental Tests of a Particle Beam Width Probe for the Aerodyne Aerosol Mass Spectrometer . Aerosol Sci. Technol. , 39 : 1143 – 1163 .
- IPCC . 2007 . Climate Change 2007: The Physical Scientific Basis , Cambridge, England : Cambridge University Press .
- Jayne , J. T. , Leard , D. C. , Zhang , X. , Davidovits , P. , Smith , K. A. Kolb , C. E. 2000 . Development of an Aerosol Mass Spectrometer for Size and Composition Analysis of Submicron Particles . Aerosol Sci. Technol. , 33 : 49 – 70 .
- Jimenez , J. L. , Canagaratna , M. R. , Donahue , N. M. , Prevot , A. S. H. , Zhang , Q. Kroll , J. H. 2009 . Evolution of Organic Aerosols in the Atmosphere . Science , 326 : 1525 – 1529 .
- Järvi , J. , Hannuniemi , H. , Hussein , T. , Junninen , H. , Aalto , P. P. Hillamo , R. 2009 . The Urban Measurement Station SMEAR III: Continuous Monitoring of Air Pollution and Surface-Atmosphere Interactions in Helsinki, Finland . Boreal Env. Res. , 14 ( suppl. A ) : 86 – 109 .
- Leck , C. , Larsson , U. , Bågander , L. E. , Johansson , S. and Hajdu , S. 1990 . Dimethyl Sulfide in the Baltic Sea: Annual Varability in Relation to Biological Activity . J. Geophys. Res. , 95 : 3353 – 3363 .
- LeClair , P. J. , Collet , J. L. and Mazzoleni , L. R. 2012 . Fragmentation Analysis of Water-Soluble Atmospheric Organic Matter using Ultrahigh-Resolution FT-ICR Mass Spectrometry . Environ. Sci. Technol. , 46 : 4312 – 4322 .
- Matthew , B. M. , Middlebrook , A. M. and Onasch , T. B. 2008 . Collection Efficiencies in an Aerodyne Aerosol Mass Spectrometer as a Function of Particle Phase for Laboratory Generated Aerosols . Aerosol Sci. Technol. , 42 : 884 – 898 .
- Mazzoleni , L. R. , Ehrmann , B. M. , Shen , X. , Marshall , A. G. and Collet , J. L. 2010 . Water-Soluble Atmospheric Organic Matter in Fog: Exact Masses and Chemical Formula Identification by Ultrahigh-Resolution Fourier Transform Ion Cyclotron Resonance Mass Spectrometry . Environ. Sci. Technol. , 44 : 3690 – 3697 .
- Middlebrook , A. M. , Bahreini , R. , Jimenez , J. L. and Canagaratna , M. R. 2012 . Evaluation of Composition-Dependent Collection Efficiencies for the Aerodyne Aerosol Mass Spectrometer using Field Data . Aerosol Sci. Technol. , 46 : 258 – 271 .
- Murphy , S. M. , Sorooshian , A. , Kroll , J. H. , Ng , N. L. , Chhabra , P. Tong , C. 2007 . Secondary Aerosol Formation from Atmospheric Reactions of Aliphatic Amines . Atmos. Chem. Phys. , 7 : 2313 – 2337 .
- Ng , N. L. , Canagaratna , M. R. , Zhang , Q. Jimenez , Tian , J. L. J. and Ulbrich . 2010 . Organic Aerosol Components Observed in Northern Hemispheric Datasets from Aerosol Mass Spectrometry . Atmos. Chem. Phys. , 10 : 4625 – 4641 .
- Nel , A. 2005 . Air Pollution-Related Illness: Effects of Particles . Science , 308 : 804 – 806 . NIST database at http://webbook.nist.gov/chemistry/ (accessed January 2009)
- Orsini , D. , Ma , Y. , Sullivan , A. , Sierau , B. , Baumann , K. and Weber , R. 2003 . Refinements to the Particle-into-Liquid Sampler (PILS) for Ground and Airborne Measurements of Water Soluble Aerosol Composition . Atmos. Environ. , 37 : 1243 – 1259 .
- Paatero , P. and Tapper , U. 1994 . Positive Matrix Factorization: A Non-Negative Factor Model with Optimal Utilization of Error Estimates of Data Values . Environmetrics , 5 : 111 – 126 .
- Pakkanen , T. A. , Kerminen , V.-M. , Korhonen , C. H. , Hillamo , R. , Aarnio , P. Koskentalo , T. 2001 . Use of Atmospheric Elemental Size Distributions in Estimating Aerosol Sources in the Helsinki Area . Atmos. Environ. , 35 : 5537 – 5551 .
- Pirinen , P. , Simola , H. , Aalto , J. , Kaukoranta , J. P. , Karlsson , P. and Ruuhela , R. 2012 . Tilastoja Suomen Ilmastosta 1981–2010 , 83 Finland : Finnish Meteorological Institute .
- Saarikoski , S. , Carbone , S. , Decesari , S. , Giulianelli , L. , Angelini , F. Canagaratna , M. 2012 . Chemical Characterization of Springtime Submicron Aerosol in Po Valley, Italy . Atmos. Chem. Phys. , 12 : 8401 – 8421 .
- Saarikoski , S. , Timonen , H. , Saarnio , K. , Aurela , M. , Järvi , L. Keronen , P. 2008 . Sources of Organic Carbon in PM1 in Helsinki Urban air . Atmos. Chem. Phys. , 8 : 6281 – 6295 .
- Saarnio , K. , Niemi , J. V. , Saarikoski , S. , Aurela , M. , Timonen , H. Teinilä , K. 2012 . Using Monosaccharide Anhydrides to Estimate the Impact of Wood Combustion on Fine Particles in the Helsinki Metropolitan Area . Boreal Env. Res. , 17 : 163 – 183 .
- Salcedo , D. , Onasch , T. B , Canagaratna , M. R. , Dzepina , K. , Huffman , J. A. Jayne , J. T. 2007 . Technical Note: Use of Beam Width Probe in an Aerosol Mass Spectrometer to Monitor Particle Collection Efficiency in the Field . Atmos. Chem. Phys. , 7 : 549 – 556 .
- Sand , M. , Berntsen , T. K. , Seland , O. and Kristjánsson , J. E. 2013 . Arctic Surface Temperature Change to Emissions of Black Carbon Within Arctic or Midlatitudes . J. Geophys. Res. , 118 : 7788 – 7798 .
- Seinfeld , J. H. and Pandis , S. N. 1998 . Atmospheric Chemistry and Physics: From Air Pollution to Climate Change , 1326 Hoboken, N. J. : John Wiley .
- Sellegri , K. , Hanke , M. , Umann , B. , Arnold , F. and Kulmala , M. 2005 . Measurements of Organic Gases During Aerosol Formation Events in the Boreal Forest Atmosphere During QUEST . Atmos. Chem. Phys. , 5 : 373 – 384 .
- Sillanpää , M. , Saarikoski , S. , Hillamo , R. , Pennanen , A. , Makkonen , U. Spolnik , Z. 2005 . Chemical Composition, Mass Distribution and Source Analysis of Long-Range Transported Wildfire Smokes in Helsinki . Sci. Total Environ. , 350 : 119 – 135 .
- Sofiev , M. , Siljamo , P. , Valkama , I. , Ilvonen , M. and Kukkonen , J. 2006 . A Dispersion Modelling System SILAM and Its Evaluation Against ETEX Data . Atmosph. Environ. , 40 : 674 – 685 .
- Sueper , D. 2008 . ToF-AMS High Resolution Analysis Software – Pika . Available at:http://cires.colorado.edu/jimenez-group/ToFAMSResources/ToFSoftware/PikaInfo/ [Accessed January 2012]
- Sun , Y.-L. , Zhang , Q. , Schwab , J. J. , Demerjian , K. L. , Chen , W.-N. Bae , M.-S. 2011 . Characterization of the Sources and Processes of Organic and Inorganic Aerosols in New York City with a High-Resolution Time-of-Flight Aerosol Mass Spectrometer . Atmos. Chem. Phys. , 11 : 1581 – 1602 .
- Timonen , H. , Carbone , S. , Aurela , M. , Saarnio , S. , Saarikoski , S. Nga , L. N. 2013 . Characteristics, Sources and Water-Solubility of Ambient Submicron Aerosol in Springtime in Helsinki, Finland . J. Aerosol Sci. , 56 : 61 – 77 .
- Timonen , H. , Saarikoski , S. K. , Aurela , M. , Saarnio , K. and Hillamo , R. E. 2008 . Water-Soluble Organic Carbon in Urban Aerosol: Concentrations, Size-Distributions and Contribution to Particulate Matter . Boreal Environ. Res. , 13 : 335 – 346 .
- Ulbrich , I. M. , Canagaratna , M. R. , Cubison , M. J. , Zhang , Q. , Ng , N. L. Aiken , A. C. 2012 . Three-Dimensional Factorization of Size-Resolved Organic Aerosol Mass Spectra from Mexico City . Atmos. Meas. Tech. , 5 : 195 – 224 .
- Ulbrich , I. M. , Canagaratna , M. R. , Zhang , Q. , Worsnop , D. R. and Jimenez , J. L. 2009 . Interpretation of Organics Components from Positive Matrix Factorization of Aerosol Mass Spectrometric Data . Atmos. Chem. Phys. , 9 : 2891 – 2918 .
- Winklmayr , W. , Wang , H.-C. and John , W. 1990 . Adaptation of the Twomey Algorithm to the Inversion of Cascade Impactor Data . Aerosol Sci. Technol. , 13 : 322 – 331 .
- Zhang , Q. , Jimenez , J. L. , Worsnop , D. R. and Canagaratna , M. 2007 . A Case Study of Urban Particle Acidity and Its Influence on Secondary Organic Aerosol . Environ. Sci. Technol. , 41 : 3213 – 3219 .