Abstract
Nucleation rate is a very sensitive function of the temperature and vapor mole fraction. Analytical approximation schemes for the mean nucleation rate in turbulent flows are derived using Laplace’s approximation method. The schemes only require the derivative of the nucleation rate function and the probability density function (pdf) of the vapor mole fraction and/or temperature at the point of maximum nucleation rate. Based on the relation between the mole fraction and temperature, i.e., linearly correlated or not, different approximation schemes are developed. Numerical examples are constructed to investigate the accuracy of these approximation. In the examples, the pdfs of mole fraction and/or temperature in various turbulent flows are assumed to come from the beta distribution with five distinct forms. The mean nucleation rate of dibutyl phthalate (DBP) aerosol in these turbulent flows are calculated from the approximation schemes, and compared with exact numerical integration. The relative errors are less than 1% for cases when nucleation rate diminishes at the bounds of temperature fluctuations, and no more than 50% for all studied examples. Furthermore, the approximation schemes are not sensitive to the precise form of the pdfs. Hence, these developed approximation schemes can be used to estimate the mean nucleation rate in a broad range of turbulent flows conveniently.
Copyright 2014 American Association for Aerosol Research
1. INTRODUCTION
Aerosol nucleation is ubiquitous in both natural and industrial processes. Nucleation takes place under supersaturation condition, which can arise from chemical or physical processes. Physical processes producing supersaturation include adiabatic expansion or mixing with cool air or radiative or conductive cooling (Friedlander Citation2000). Turbulence is characterized by strong mixing, hence nucleation in turbulence is important and has received much attention (Lesniewski and Friedlander Citation1995; Chan et al. Citation2010a,Citationb; Zhou and Chan Citation2011; Fager et al. Citation2012). Due to the complexity of turbulence, a practical approach is to estimate the mean nucleation rate efficiently based on the fundamentals of turbulence, that is, easily obtainable information.
Nucleation rate is a sensitive function of temperature T and vapor mole fraction c, that is, . The mean nucleation rate can be computed as

However, there are two limitations in both of the approximations (Shaw Citation2004; Zhou and Chan Citation2011). One is that the integration domain in Equation (Equation2) is assumed to be large enough (termed as infinite bounds) that the nucleation rate is then intrinsically to be zero at the integration bounds. Usually, this assumption is satisfied. But if the temperature or vapor mole fraction fluctuates in a range (termed as finite bounds) that the nucleation rate does not vanish at the bounds of the studied range, then the approximations of Shaw (Citation2004) and Zhou and Chan (Citation2011) will introduce big errors. The other limitation is the assumption of Le=1, which is generally not true in practice.
In this study, new approximations are developed and introduced, which relieve the two limitations mentioned above, and are applicable to the cases of Le=1 and Le≠1, and of the infinite bounds and the finite bounds.
2. NUCLEATION RATE
From the classical nucleation theory, the nucleation rate satisfies (Friedlander Citation2000)


3. APPROXIMATIONS OF MEAN NUCLEATION RATE FOR
3.1. Infinite Bounds
From Equations Equation(2)–Equation(5), it is clear that the mean nucleation rate can be computed as

Long ago, Laplace (Citation1986) made the observation that the major contribution to the integral type of Equation (Equation6) should come from the neighborhoods of the point where the integrand obtains its greatest value. More precisely, assume f(T) obtains its minimum at T*, and T min<T*<T max, then (Wong Citation2001)
FIGURE 1 Quadratic approximation of the nucleation rate function
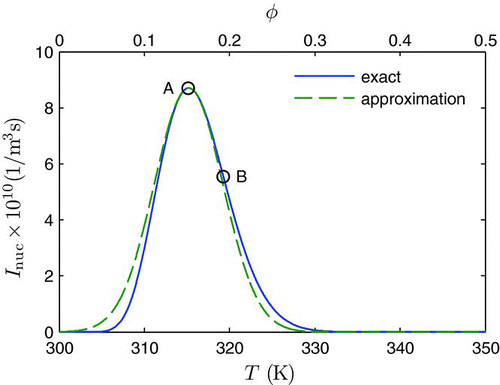
The first approximation is obtained by replacing h(x) with its quadratic expansion. The second approximation is based on the assumption that T min and T max are far away from T* and the exponential function nearly decays to zero beyond T min and T max. Therefore, it is permissible to extend the integral domain to infinity. The last step uses the Gaussian integral.
The applicability of the Laplace’s approximation (Equation (8)) relies on two prerequisites: (i) f(T) has a minimum inside the domain, and (ii) the integrand almost vanishes beyond the integration bounds. Fortunately, the prerequisites are usually satisfied when evaluating the mean nucleation rate in turbulence. The approximation of Equation (8) is slightly different from that of Shaw (Citation2004) (There is a typo of missing the absolute sign therein.)


3.2. Finite Bounds
If the nucleation rate function is far from zero at either bound of the domain, neither Equation (8) nor (Equation10) is a good approximation any longer (). First, the nucleation rate is assumed to be much larger than zero at the lower bound of temperature fluctuation T lower, which is not necessarily equal to T min (but T lower⩾T min) as discussed in the Introduction section. For this case, it is still possible to use the same quadratic expansion of Equation (8), and to follow the derivation of Equations (Equation9a) and (9b), but only to extend the upper bound to infinity and keep the lower bound intact. It is then straightforward to obtain
If the nucleation rate is much larger than zero at the upper bound of temperature fluctuation T upper (T upper⩽T max), the approximation can be obtained by replacing (T lower−T*) with (T*−T upper) in Equation (Equation11) due to the symmetry
The case for nonvanishing nucleation rate at both T lower and T upper can be obtained by the simple integration relation
The approximations of Equations (Equation11) and (Equation12) are based on the quadratic order Taylor expansion of f(T) at T*. If T lower or T upper is far way from T*, it is better to expand f(T) at T lower or T upper. Since T lower and T upper are not local minimum points, f′(T lower)≠0 and f′(T upper)≠0, then f(T) can be expanded to the linear order
3.3. Numerical Examples
Here, the nucleation of dibutyl phthalate (DBP) is investigated as numerical examples. DBP as condensate has been used in various experimental investigations (Higuchi and O’Konski Citation1960; Brock et al. Citation1986; Okuyama et al. Citation1987; Lesniewski and Friedlander Citation1998; Pyykönen et al. Citation2007), because it is a stable compound and does not need any cooling to observe nucleation. There are also numerical simulations (Garmory and Mastorakos Citation2008; Zhou and Chan Citation2011; Zhou et al. Citation2012) on DBP aerosol dynamics. Empirical equations for ρ
p
, , and σ are taken from Okuyama et al. (Citation1987), which are given in .
Physical properties of DBP, from Okuyama et al. (Citation1987)
The temperature and vapor mole fraction are set as T min=300 K, T max=400 K, c min=0 ppm, and c max=500 ppm, which correspond to the boundary conditions for a turbulent jet studied in the experiment (Lesniewski and Friedlander Citation1998) and the numerical simulations (Garmory and Mastorakos Citation2008; Zhou and Chan Citation2011).
To compute the mean nucleation rate in turbulence, it requires the pdf of the mixture fraction (a representative of temperature and vapor mole fraction). A popular model for the pdf is the beta distribution (Girimaji Citation1991)
Relative errors of the quadratic and linear approximations to the mean nucleation rate, with a linear correlation between T and c
It is useful to briefly discuss how to evaluate the derivatives in the Laplace’s approximations. Although the function f(x) is complex, its derivative can be obtained by symbolic computation software, or by finite difference approach. When using finite difference, attention should be paid to the choice of the spacing. For example, in the central difference scheme f′(x)=[f(x+l)−f(x−l)]/(2l), in order to optimize the truncation and roundoff errors, it is optimal (Press et al. Citation1992) to set l=ε1/3 xc , where ε is the machine precision, and xc is a “characteristic scale” of the function f(x), which is usually assumed xc =x (except near x=0).
gives the relative errors of the quadratic/linear approximations to the mean nucleation rate for various cases. Three temperature ranges are investigated, the infinite bounds case (300–400 K) and two finite bounds cases (315–400 K and 330–400 K). For each temperature range, the five beta pdfs shown in are used to evaluate the mean nucleation rate. The relative error is defined as , where the Exact value is obtained by direct numerical integration of Equation (Equation6). The relative errors using the quadratic and the linear approximations are compared. For the infinite bounds cases (300–400 K), the relative errors using the quadratic approximation of Equation (9c) are from 0.6 to 1.6% for all the pdfs considered here. The linear approximation is not applicable for the infinite bounds case, since the linear term in the Taylor expansion (8) vanishes. In the cases with temperature range 315–400 K, the relative errors using the quadratic approximation are from 7.9 to 16.1%. The relative errors using the linear approximation are much more than hundred percent. For the temperature range 330–400 K, the relative errors using the quadratic approximation are from 41.9 to 250.2%, which are around one order of magnitude higher than the linear approximation. A thumb of rule for choosing between the quadratic or the linear approximation is to compare the temperature bound with the inflection point (B in ). If the temperature bound exceeds the inflection point, the linear approximation performs much better. If the temperature bound is close to the maximum point (A in ), the quadratic approximation should be used. It is worth pointing out that the case for temperature range of 330–400 K is not very important in practice, because the value is not very high at the tail of the nucleation rate function. Hence, the quadrature approximation can generally be used.
FIGURE 3 The pdfs of a passive scalar (mixture fraction φ) in a turbulent mixing layer at three crosswise locations, corresponding to mean values of


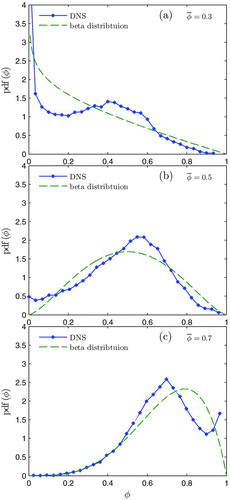
The pdf shape affects the approximation to some extent, but without clear trend. A general conclusion is that the precision of the approximation is not very sensitive to the pdf. In the Laplace’s approximation, the whole pdf over the domain is not required, but only the probability density at the point where the nucleation rate obtains its maximum. The maximum point can be determined from the given boundary conditions for a specific turbulent flow. Therefore, it is possible to approximate the mean nucleation rate in turbulence by estimating the probability density at the maximum nucleation point, which is very convenient and efficient. The error in the approximation is nearly proportional to the accuracy for estimating the probability density at the maximum point. This fact has also been observed by Shaw (Citation2004) in the analysis for the infinite bounds case.
To further clarify the application of the Laplace approximation scheme, a practical example is given to estimate the mean nucleation rate at a turbulent mixing layer. In the recent work of Zhou et al. (Citation2012), the pdf of a passive scalar (mixture fraction φ) is obtained by means of direct numerical simulation (DNS). Here, the mean nucleation rate evaluated from the pdf and the corresponding approximation using beta distribution is compared. Besides, the error in evaluating the mean nucleation rate by the Laplace approximation is also investigated. In , from the DNS at three crosswise locations with the corresponding beta distribution are shown. The beta distribution is determined from the mean (μ) and variance (σ2) of the pdf, as
4. APPROXIMATIONS OF MEAN NUCLEATION FOR
4.1. Infinite Bounds
Quite similar to the single integral of an exponential function, Laplace’s approximation is also developed for double integral (Hsu Citation1948)

However, Equation (Equation20) is generally not applicable to the approximation of the mean nucleation rate. Because the nucleation rate of Equation (Equation4a) is a monotonically decreasing function of T, and is a monotonically increasing function of c. In other words, nucleation prefers high vapor mole fraction and low vapor temperature. It is impossible to find a point (Tc
, cc
) inside the domain (Rectangular domain is assumed, and irregular domain is not considered here) at which f(T, c) obtains the minimum. To demonstrate this point more clearly, plots a surrogate function 1/f(T, c), which has the same monotonicity as the nucleation rate function e−f(T,c). The reason to use the surrogate function other than the original one is that e−f(T,c) variates so dramatically, hence it is difficult to show the characteristics clearly in the plot. Obviously, the nucleation rate obtains its maximum at the corner of the domain, (T, c)=(300, 500), when T and c are independent to each other. However, if T and c are linearly related to each other (the dashed line in the T–c plane), the nucleation rate function has a peak inside the domain, as shown in the continuous curve (the curve with dots is the projection onto a plane ). That is the case of Le=1 discussed in Section 3. Of course, the nucleation rate function may also have a peak or peaks if T and c are related in a more involved way than the linear relation discussed here, which should be studied on a case-by-case basis.
FIGURE 4 The surrogate function 1/f(T, c) for the nucleation rate, normalized by its maximum in the domain. If T and c are linearly correlated (dashed line in the T–c plane), f(T, c) is then the continuous curve shown. For clarity, the curve is projected to a plane of
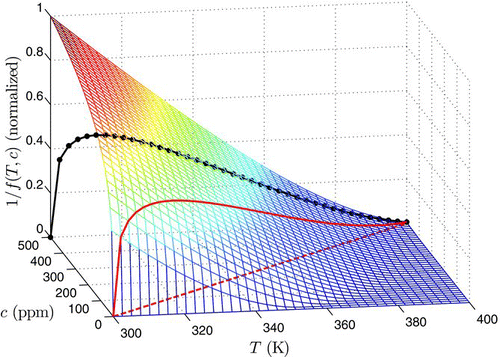
4.2. Finite Bounds
Although the simple quadratic order approximation of Equation (Equation20) is not applicable for nucleation, it is possible to obtain simple approximation from a linear order. Following the developed method of López and Pagola (Citation2008), the function f(T, c) can be approximated to first order


The first approximation is obtained by replacing f(T, c) with its linear approximation of Equation (Equation21). The second approximation is obtained through two steps: first step, by changing the variables (T−Tc )→T and (c−cc )→−c, and then extending the upper integral bound to infinity. The second step is carried out straightforwardly.
The approximation of Equation (Equation22) is applicable to a rectangular domain of integration (Tc , T max)×(c min, cc ), with the assumption that nucleation rate vanishes at the bounds T=T max or c=c min. If the nucleation rate is far from zero at the upper temperature or lower vapor mole fraction bounds, then it only requires to represent the domain of integration (Tc , T max)×(c min, cc ) as the subtraction of (T max, T ∞)×(0, c min) from (Tc , T max)×(0, cc ), and apply the approximation of Equation (Equation22) to the last two domains. Here, T ∞ denotes a high temperature at which the nucleation rate is zero.
4.3. Numerical Examples
The numerical examples shown in this section have similar setting with those in Section 3.3, except that the integral domain is two-dimensional instead of one-dimensional. Since it has shown that the Laplace approximation is not sensitive to the pdf in Section 3, here only the uniform pdf is considered for convenience.
shows the relative errors for three temperature and three vapor mole fraction ranges (total nine cases). Only linear approximation is constructed. Generally, the smaller the temperature range (or vapor mole fraction range) is, the smaller the relative error will be. The maximum error is less than 40% for T=300–400 K and c=0–500 ppm cases. Taking into account the substantial uncertainties in turbulence and the kinetic theory of nucleation, the approximation of the mean nucleation rate is quite satisfactory.
Relative errors of the linear approximation of the mean nucleation rate, with independent T and c
4.4. Discussion
The analytical approximation method derived here has advantage over numerical scheme on certain aspects. It is much easier to investigate the functional dependence of the mean nucleation rate on the pdf of temperature and vapor concentration. The analytical approximation method here may be used to solve some specific aerosol evolution problems, combined with asymptotic methods.
On the numerical aspect, the analytical approximation scheme is numerically far more efficient than direct numerical double integration in evaluating the mean nucleation rate, while sacrificing the numerical precision to certain extent. Although the computational resource becomes much cheaper, the numerical efficiency is still critical for some complex problems. One example is the DNS of aerosol evolution in turbulent flows. In a DNS, the grid size is required to resolve the Kolmogorov scale, which renders the number of grid cells proportional to Re 9/4 (where Re is the Reynolds number) (Pope Citation2000). However, due to the sensitivity of nucleation rate to the temperature, the nucleation layer might be still under-resolved at some fast mixing regions (Zhou et al. Citation2014). It is extremely expensive to reduce to the grid size in order to resolve the nucleation layer. A good workaround is to reconstruct the temperature T and vapor concentration c in a grid cell through an interpolation from neighbor grid cell values, and to evaluate the mean nucleation rate in the grid cell from the reconstructed field of T and c. The mean nucleation rate is evaluated as the nucleation rate function is convoluted with the distribution of T and c, which has exactly the same form as Equation (Equation20). Although numerical schemes can be used to carry out the double integration, it is very expensive to do so at every grid cell (usually tens of millions to even billions). The Laplace approximation scheme here (Equation (Equation22)) is far more efficient to evaluate the mean nucleation rate than the numerical double integration (of course, with mild sacrifice of its numerical precision).
5. CONCLUSION
Nucleation rate is a very sensitive function of temperature and vapor mole fraction. The mean nucleation rate in turbulence is the convolution integral of the nucleation rate function with the joint pdf of temperature and vapor mole fraction. If the temperature is linearly related to the vapor mole fraction (Le=1), the evaluation of mean nucleation rate is simplified to one-dimensional integration over the temperature fluctuation range in a turbulent flow. Depending on where the nucleation rate obtains its maximum, that is, inside the temperature range or at one end of the temperature range, two approximation equations are developed to evaluate the mean nucleation rate using Laplace’s method. These equations only require the derivative (either first or second order) of the nucleation rate function and the probability density at the point of maximum nucleation rate. Five pdfs from the beta distribution are used to model the pdfs of temperature in various turbulent flows, then the approximation equations are used to compute the mean nucleation rate of DBP aerosol for the five pdfs. The numerical examples show that the relative errors are around 1% when the nucleation rate approaches zero at both ends of the temperature range. For other cases, the relative errors are larger, but no more than 20% with the best adequate analytical approximation scheme (linear or quadratic). Taking into account the substantial uncertainties of the nucleation kinetic theory and turbulence, these approximation schemes are well acceptable.
If the temperature and vapor mole fraction are independent to each other (Le≠1), it is found that the nucleation rate function cannot obtain a local maximum inside the domain, but it can only obtain its maximum at the bound of the domain, corresponding to the minimum temperature and maximum vapor mole fraction. An approximation equation for the mean nucleation rate is also derived, and the numerical examples show that the approximation scheme is also accurate and efficient.
Hence, these developed approximation schemes can be used to estimate the mean nucleation rate in a broad range of turbulent flows conveniently.
FUNDING
This work was supported by the grants from the Mechanical Engineering Department and the Central Research Grant of The Hong Kong Polytechnic University (Project Nos. G-U918 and G-YK66).
REFERENCES
- Attili , A. and Bisetti , F. 2012 . Statistics and Scaling of Turbulence in a Spatially Developing Mixing Layer at Reλ = 250 . Phys. Fluids , 24 : 035109 Article No.
- Baurle , R. A. , Alexopoulos , G. A. and Hassan , H. A. 1994 . Assumed Joint Probability Density Function Approach for Supersonic Turbulent Combustion . J. Propul. Power , 10 : 473 – 484 .
- Brock , J. R. , Kuhn , P. J. and Zehavi , D. 1986 . Condensation Aerosol Formation and Growth in a Laminar Coaxial Jet: Experimental . J. Aerosol Sci. , 17 : 11 – 22 .
- Chan , T. L. , Liu , Y. H. and Chan , C. K. 2010a . Direct Quadrature Method of Moments for the Exhaust Particle Formation and Evolution in the Wake of the Studied Ground Vehicle . J. Aerosol Sci. , 41 : 553 – 568 .
- Chan , T. L. , Zhou , K. , Lin , J. Z. and Liu , C. H. 2010b . Vehicular Exhaust Gas-to-Nanoparticle Conversion and Concentration Distribution in the Vehicle Wake Region . Int. J. Nonlinear Sci. Numer. Simul. , 11 : 581 – 592 .
- Fager , A. J. , Liu , J. and Garrick , S. C. 2012 . Hybrid Simulations of Metal Particle Nucleation: A Priori and a Posteriori Analyses of the Effects of Unresolved Scalar Interactions on Nanoparticle Nucleation . Phys. Fluids , 24 : 075110 Article No.
- Friedlander , S. K. 2000 . Smoke, Dust, and Haze: Fundamentals of Aerosol Dynamics, 2nd ed. , New York : Oxford University Press .
- Garmory , A. and Mastorakos , E. 2008 . Aerosol Nucleation and Growth in a Turbulent Jet Using the Stochastic Fields Method . Chem. Eng. Sci. , 63 : 4079 – 4089 .
- Girimaji , S. S. 1991 . Assumed β-pdf Model for Turbulent Mixing: Validation and Extension to Multiple Scalar Mixing . Combust. Sci. Tech. , 78 : 177 – 196 .
- Givi , P. 1989 . Model-Free Simulations of Turbulent Reactive Flows . Prog. Energy Combust. Sci. , 15 : 1 – 107 .
- Higuchi , W. and O’Konski , C. 1960 . A Test of the Becker-Doering Theory of Nucleation Kinetics . J. Colloid Sci. , 15 : 14 – 49 .
- Hsu , L. C. 1948 . Approximations to a Class of Double Integrals of Functions of Large Numbers . Amer. J. Math. , 70 : 698 – 708 .
- Laplace , P. S. 1986 . Memoir on the Probability of the Causes of Events (translated from the original French) . Statist. Sci. , 1 : 364 – 378 .
- Lesniewski , T. and Friedlander , S. K. 1995 . The Effect of Turbulence on Rates of Particle Formation by Homogeneous Nucleation . Aerosol Sci. Technol. , 23 : 174 – 182 .
- Lesniewski , T. K. and Friedlander , S. K. 1998 . Particle Nucleation and Growth in a Free Turbulent Jet . Proc. R. Soc. Lond. A , 454 : 2477 – 2504 .
- López , J. L. and Pagola , P. J. 2008 . A Simplification of the Laplace Method for Double Integrals. Application to the Second Appell Function . Electron. Trans. Numer. Anal. , 30 : 224 – 236 .
- Okuyama , K. , Kousaka , Y. , Warren , D. R. , Flagan , R. C. and Seinfeld , J. H. 1987 . Homogeneous Nucleation by Continuous Mixing of High-Temperature Vapor with Room Temperature Gas . Aerosol Sci. Technol. , 6 : 15 – 27 .
- Pope , S. B. 2000 . Turbulent Flows , Cambridge : Cambridge University Press .
- Press , W. H. , Teukolsky , S. A. , Vetterling , W. T. and Flannery , B. P. 1992 . Numerical Recipes in Fortran 77: The Art of Scientific Computing, 2nd ed. , New York : Cambridge University Press .
- Pyykönen , J. , Miettinen , M. , Sippula , O. , Leskinen , A. , Raunemaa , T. and Jokiniemi , J. 2007 . Nucleation in a Perforated Tube Diluter . J. Aerosol Sci. , 38 : 172 – 191 .
- Shaw , B. D. 2004 . Asymptotic Evaluation of Probability Density Functions for Mean Aerosol Particle Formation Rates by Homogeneous Nucleation in Turbulent Gas Jets . J. Aerosol Sci. , 35 : 177 – 184 .
- Wong , R. 2001 . Asymptotic Approximations of Integrals , Philadelphia : SIAM: Society for Industrial and Applied Mathematics .
- Zhou , K. , Attili , A. , Alshaarawi , A. and Bisetti , F. 2014 . Simulation of Aerosol Nucleation and Growth in a Turbulent Mixing Layer . Phys. Fluids , under review
- Zhou , K. , Attili , A. and Bisetti , F. Direct Numerical Simulation of Aerosol Growth Processes in a Turbulent Mixing Layer . Seventh International Conference on Computational Fluid Dynamics (ICCFD7), Big Island, Hawaii, July 9–13. Paper No. 1504
- Zhou , K. and Chan , T. L. 2011 . Simulation of Homogeneous Particle Nucleation in a Free Turbulent Jet . Aerosol Sci. Technol. , 45 : 973 – 987 .