Abstract
We used quantum chemical formation free energies of hydrated sulfuric acid-containing molecular clusters and a dynamic model to simulate a flow tube measurement, and determined the effective diffusion coefficient of sulfuric acid as a function of relative humidity. This type of measurement was performed by Hanson and Eisele, who presented and applied a fitting method to obtain equilibrium constants K1 and K2 for the formation of sulfuric acid mono- and dihydrates, respectively, from the experimentally determined diffusion coefficients. The fit is derived assuming that only H2SO4 molecules hydrated by up to two water molecules are present. To study the sensitivity of the results to this assumption, we implemented the same fit to the modeled diffusion coefficient data, computed including also larger H2SO4 hydrates with more than two waters. We show that according to quantum chemical equilibrium constants, the larger hydrates are likely to be present in nonnegligible amounts, which affects the effective diffusion coefficient. This results in the fitted value obtained for K1 being lower and for K2 being higher than the actual values. The results are further altered if contaminant base molecules, such as amines, capable of binding to H2SO4 molecules, are able to enter the system, for example, with the water vapor. The magnitude and direction of the effect of the contaminants depends not only on the contaminant concentration, but also on the H2SO4 concentration and on the hygroscopicity of the H2SO4–base clusters.
Copyright 2014 American Association for Aerosol Research
1. INTRODUCTION
Sulfuric acid has been shown to be the main compound driving atmospheric new particle formation (Weber et al. Citation1997; Kuang et al. Citation2008). The role of other species has been the topic of intensive research: for instance, atmospheric bases, organic compounds, and ions have been suggested to enhance particle formation and growth by stabilizing acid-containing molecular clusters (Zhang et al. Citation2004; Yu Citation2006; Erupe et al. Citation2011; Kirkby et al. Citation2011; Yu et al. Citation2012). Especially strong bases, such as amines, may be able to bind strongly to even single sulfuric acid molecules (Kurtén et al. Citation2008; Loukonen et al. Citation2010; Almeida et al. Citation2013). Whenever water vapor is present at sufficient concentrations, acid molecules and clusters are likely to be hydrated. It is at the moment uncertain to which degree acid–base clusters are hydrated at moderate RH, and how strongly hydration affects their formation energetics. In any case, it is clear that in the absence of stronger bases, for example in the free troposphere, water plays a very important role in enhancing the clustering of sulfuric acid. However, the equilibrium constants for hydrate formation, or equivalently the hydrate distribution, are difficult to predict for molecules and very small clusters.
Equilibrium constants of H2SO4–water clusters have been previously calculated with the classical liquid droplet model by, for example, Jaecker-Voirol et al. (Citation1987) and Noppel et al. (Citation2002). However, the droplet model that describes a macroscopic substance is not valid for clusters consisting of only a few molecules (Noppel et al. Citation2002). The most accurate and reliable theoretical method to study the thermodynamics of small molecular clusters is quantum chemistry (see, for example, the review by Kurtén and Vehkamäki [Citation2008]). Although in general quantitative results given by different quantum chemical methods may differ, the qualitative predictions agree (Leverentz et al. Citation2013, and references therein, and Kupiainen-Määttä et al. Citation2013 for method comparisons). Equilibrium constants for cluster formation, or the corresponding cluster formation free energies (EquationEquation (4)[4] ), can be experimentally determined with a chemical composition measurement. However, the composition measurement is performed with mass spectrometry, which can only be applied to charged clusters. The Gibbs free energies of formation of charged H2SO4 hydrates have been measured by, for instance, Froyd and Lovejoy (Citation2003a, Citation2003b), and Sorokin et al. (Citation2006). Experimental determination of the formation free energies of electrically neutral clusters is very challenging, as their composition cannot be directly measured. A method to measure the hydration free energies of the H2SO4 molecule, based on the effect of hydrate formation on the diffusivity of H2SO4 vapor, was presented by Hanson and Eisele (Citation2000).
Hanson and Eisele (Citation2000) obtained experimental values for the equilibrium constants for the formation of sulfuric acid mono- and dihydrates using the following approach: the effective diffusion coefficient of H2SO4 vapor at different relative humidities (RHs) was determined by measuring the wall loss rate coefficient in a cylindrical laminar flow tube (see also the study by Hanson (Citation2005) that reports similar results, but with fewer data points). The gas phase acid concentration [H2SO4] was measured with a chemical ionization mass spectrometer (SCIMS; Eisele and Tanner Citation1993) which detects also the acid hydrates, and therefore the measured wall loss rate coefficient is the effective loss rate of the sum of all the species H2SO4•(H2O)n with n = 0–nmax, where nmax is the maximum number of water molecules bound to the acid molecule. The hydrate distribution can be assumed to be in equilibrium throughout the flow tube as the collision and evaporation rates of water molecules are orders of magnitude higher than the rates of the diffusion transport processes. The effective diffusion coefficient Deff was obtained from the effective wall loss rate coefficient kw,eff assuming diffusion-limited wall loss (Brown Citation1978):
[1] where r is the radius of the flow tube. Finally, Deff was converted to the pressure-independent diffusion coefficient pDeff by multiplying by the total pressure in the flow tube.
The equilibrium constants were obtained by assuming that H2SO4 can be hydrated by up to nmax = 2 water molecules, in which case pDeff at RH, calculated as a weighted average over the equilibrium hydrate distribution (Jaecker-Voirol et al. Citation1987), is given as
[2] where pDn is the diffusion coefficient of H2SO4•(H2O)n and Kn is the equilibrium constant for the formation of H2SO4•(H2O)n from H2SO4•(H2O)n−1 and H2O, which can be converted to the standard reference pressure of Pref = 1 atm as
[3] where
is the saturation vapor pressure of water (see Wexler Citation1976 for a parameterization for
). The equilibrium constant is related to the change in the Gibbs free energy as
[4] where ΔGref(i) is the Gibbs free energy of formation of cluster i from monomers at reference pressure Pref and temperature T, and kB is the Boltzmann constant.
The experimental procedure described above is a straightforward method to estimate the K1 and K2 constants, provided that only the H2SO4•(H2O)0-2 species affect the diffusion measurement and that values for the diffusion coefficient of each molecule and cluster species (pDn) are known (see Section 2.3). Hanson and Eisele (Citation2000) also tested the effect of extending EquationEquation (2)[2] to include the third hydrate H2SO4•(H2O)3, but the K1 and K2 values reported in the study are obtained by including only the first and second hydrates. The work by Hanson and Eisele (Citation2000) is to our knowledge the only study presenting experimental equilibrium constants for electrically neutral sulfuric acid hydrates, which makes the results very important benchmarking parameters for theoretical work, for example, quantum chemistry or liquid droplet model.
Table 1 Clusters included in the systems studied using the B3LYP/CBSB7//RICC2/aug-cc-pV(T+d)Z cluster energies. The number of water molecules in the cluster is indicated in parenthesis. Additional clusters, that were included only in the simulations where the system was extended to clusters containing up to two H2SO4 and two base molecules, are marked with an asterisk; otherwise only clusters containing up to one H2SO4 and one base molecule were included
In this work, we use quantum chemical equilibrium constants for H2SO4 hydrates and small acid-containing clusters to study how the presence of larger hydrates and other compounds affects the measurement result. First, we examine the effect of including H2SO4 hydrates containing more than two water molecules. Second, we assess the sensitivity of this type of experiment to the potential presence of base molecules that may form stable clusters with H2SO4, as these types of molecules have been observed in the CLOUD chamber experiments at background-level concentrations (Kirkby et al. Citation2011). We apply the fitting method of Hanson and Eisele (Citation2000) to the modeled diffusion coefficient data, and compare the values of the K1 and K2 constants obtained from the fit to the actual values that were used to generate the data. We emphasize that the main purpose of this study is not a direct comparison of absolute experimental and quantum-chemical equilibrium constants (such comparisons have been done for example by Kurtén et al. (Citation2007) and Nadykto and Yu (Citation2007) for a large variety of different methods). Instead, we focus on the relative effect of H2SO4•X clusters (where X is anything else than H2O or (H2O)2) on the K1 and K2 values obtained using EquationEquation (2)[2] . The results provide insights into the directions and magnitudes of the changes that the presence of such clusters may cause in the measurable diffusion coefficient and the fitted K1 and K2 parameters.
2. METHODS
We calculated theoretical diffusion coefficients for sulfuric acid and representative base contaminant molecules and small acid–base clusters and their hydrates. The effective diffusion coefficient of each molecule or cluster at different RHs was calculated as a weighted average over the equilibrium hydrate distribution. The hydrate distributions were computed from the Gibbs free energies of hydration obtained from quantum chemical calculations (as in Kurtén et al. Citation2007 and Henschel et al. Citation2014; see also the study by Marti et al. [Citation1997], who calculated the effective diffusion coefficient of H2SO4 in a similar manner using classical thermodynamics as in Kulmala et al. Citation1991 for determining the Gibbs free energies and hydrate distributions). The saturation vapor pressure of water was calculated with the temperature-dependent parameterization derived by Wexler (Citation1976). We used the calculated diffusion coefficients and a dynamic cluster model (McGrath et al. Citation2012) to generate a set of “measurable” H2SO4 diffusion coefficient data as a function of RH in different conditions (see Sections 2.1 and 2.4). We applied the fitting method used by Hanson and Eisele (Citation2000), which is based on the assumption that there exist only H2SO4•(H2O)0-2 clusters (EquationEquation (2)[2] ), to obtain the equilibrium constants K1, fit and K2, fit from the generated data, computed including also species other than H2SO4•(H2O)0-2. We then compared the fitted values to the actual input values K1, input and K2, input to determine the effect that the presence of the other species may have on the experimentally fitted parameters. A flowchart illustrating the procedure is presented in .
2.1. Modeled Molecules and Clusters
First, we considered a system consisting solely of acid monomers and their hydrates H2SO4•(H2O)n, n = 0−nmax, and studied the effect of including hydrates containing more than nmax = 2 water molecules in the calculation. Then, we assumed that there exists a background concentration of base molecules B and its hydrates that can collide with H2SO4•(H2O)n to form H2SO4•B clusters and their hydrates. In the case of the base-contaminated system, we focused on dimethylamine (DMA; (CH3)2NH) as a representative base compound, with additional simulations performed using ammonia (NH3), monomethylamine (MMA; CH3NH2) or trimethylamine (TMA; (CH3)3N). Finally, we studied the effect of formation of larger clusters by including (H2SO4)2 clusters and their hydrates in the system with no bases. For the system containing H2SO4 and DMA, we tested the effect of incorporating B2, H2SO4•B2, (H2SO4)2•B, and (H2SO4)2•B2 clusters, as well as their hydrates. A summary of the examined systems is presented in . To avoid unnecessary computational burden, the free energies of hydration that are not previously published by Henschel et al. (Citation2014) (i.e., mainly the hydrates containing MMA or TMA) were not computed up to a fixed number of water molecules. Instead, the hydration energies were first computed for the first hydration steps, and if the formation of these small hydrates proved to be unfavorable, larger hydrates were omitted. Hydrates of clusters that are in any case very unstable and therefore not abundant (those consisting of only two base molecules or two bases and one acid) were also omitted.
2.2. Quantum Chemical Data
The equilibrium hydrate distribution calculations and dynamic simulations were mainly performed using quantum-chemical Gibbs free energies of formation of the clusters calculated with a multi-step method proposed by Ortega et al. (Citation2012), that combines B3LYP/CBSB7 optimized geometry and frequency calculations with RI-CC2/aug-cc-pV(T+d)Z single point energy calculations (Ortega et al. Citation2012, and references therein). Thermochemical data computed with the B3LYP/CBSB7//RICC2/aug-cc-pV(T+d)Z method for the unhydrated sulfuric acid–ammonia and sulfuric acid–DMA clusters are published in our previous work (Ortega et al. Citation2012), and the data for the hydrated clusters, as well as for clusters containing MMA or TMA, are given in the online supplementary information (SI).
For the system containing only H2SO4•(H2O)n species, we used also Gibbs free energies computed at different quantum-chemical levels of theory by Kurtén et al. (Citation2007), Nadykto and Yu (Citation2007) and Temelso et al. (Citation2012). Kurtén et al. (Citation2007) used the MP2/aug-cc-pV(D+d)Z level for optimization and frequency calculations, and performed higher-level calculations at the MP2/aug-cc-pV(T+d)Z and MP4/aug-cc-pV(D+d)Z levels to obtain the single-point energy, denoted with ΔE0,3-step (Kurtén et al. Citation2007), as a combination of the MP2/aug-cc-pV(D+d)Z and MP4/aug-cc-pV(D+d)Z energies and a basis set limit energy extrapolated from the two MP2 energies (for more detailed descriptions of the methods, see the section ``Method validation'' in Kurtén et al. Citation2007). Vibrational anharmonicity was taken into account with scaling factors (the ‘‘sf2’’ scaling factor set, see Kurtén et al. Citation2007). Nadykto and Yu (Citation2007) used the PW91PW91/6-311++G(3df,3pd) level of theory. Temelso et al. (Citation2012) computed thermodynamic corrections at the RI-MP2/aug-cc-pVDZ level and the single-point energy by extrapolating RI-MP2/aug-cc-pVDZ, RI-MP2/aug-cc-pVTZ and RI-MP2/aug-cc-pVQZ energies to the complete basis set limit, denoted as CBS (Temelso et al. Citation2012), and also used anharmonic scaling factors (for more details, see Temelso et al. Citation2012). All the thermochemical data used in this study were computed at 298.15 K.
2.3. Diffusion Coefficients
The diffusion coefficients of molecules and clusters in nitrogen gas were calculated as in the Enskog–Chapman theory (Chapman and Cowling Citation1952), according to which the first approximation for the diffusion coefficient of two vapor species, in this case molecule or cluster i and nitrogen N2, is
[5] where ri and mi are the radius and mass of species i, and
and
those of the nitrogen molecule, respectively. Ctot is the total vapor concentration which can be obtained from the ideal gas law and total vapor pressure Ptot as Ctot = Ptot/(kBT). The radius of the nitrogen molecule
was taken to be 1.85 Å (Haynes Citation2014), which is obtained from the viscosity of pure nitrogen gas
according to the kinetic gas theory as (Chapman and Cowling Citation1952)
[6] The radii of other molecules and clusters were calculated from the molecular masses and liquid densities of the pure compounds assuming spherical clusters and ideal mixing (the used densities were 1830 kg m−3 for H2SO4, 680 kg m−3 for DMA, 696 kg m−3 for NH3, 656 kg m−3 for MMA, 627 kg m−3 for TMA, and 997 kg m−3 for H2O; Haynes Citation2014). This approach gives the value of 0.096 atm cm2 s−1 at 298.15 K for the diffusion coefficient of the unhydrated sulfuric acid molecule, which is in agreement with the experimental values 0.094 atm cm2 s−1 (±7%) at 298 K (Hanson and Eisele Citation2000), 0.11 atm cm2 s−1 (±20%) at 295 K (Lovejoy and Hanson Citation1996) and 0.088 atm cm2 s−1 (±2%) at 303 K (Pöschl et al. 1998). The values obtained for the diffusion coefficients of the sulfuric acid mono- and dihydrates (87 and 78% of the value for the dry H2SO4 molecule, respectively) also agree closely with those used by Hanson and Eisele (Citation2000) (85 and 76% of the dry value), who used contemporary quantum chemical hydrate structures to estimate the collision cross sections with the nitrogen molecule. The calculated diffusion coefficients of all the molecules and clusters included in the dynamic simulations are given in the SI.
2.4. Dynamic Simulations
The effective diffusion coefficients pDeff for the system containing only the H2SO4•(H2O)n species were obtained directly by taking the weighted average of the diffusion coefficients of the hydrates. For the systems containing also molecular clusters, we modeled the dynamics of the systems with the Atmospheric Cluster Dynamics Code (ACDC; McGrath et al. 2012; see Olenius et al. 2013 for a description of the current version) to obtain the measurable diffusion coefficients. “Measurable” refers to determining the effective diffusion coefficient from the simulation data with the same method that was used to obtain it in the flow tube measurement (Section 2.4.2).
The time development of the cluster concentrations in conditions relevant to a flow tube experiment is given as
[7] where Ci is the concentration of cluster or molecule i, βi,j is the collision rate coefficient between clusters i and j, γk→i,j is the evaporation rate coefficient of cluster k fragmenting into clusters i and j, and kwall,i is the wall loss rate coefficient for cluster i. The collision coefficients were calculated according to the kinetic gas theory (Chapman and Cowling Citation1952) assuming that all collisions stick, and the evaporation coefficients were determined from the quantum chemical Gibbs free energies of formation according to the condition of detailed balance (for more detailed descriptions of calculating the rate coefficients; McGrath et al. Citation2012 and Ortega et al. Citation2012). The wall loss rate coefficients were obtained from the calculated diffusion coefficients (EquationEquation (5)
[5] ) according to EquationEquation (1)
[1] . All the collision, evaporation, and wall loss rate coefficients were calculated as weighted averages over the equilibrium hydrate distributions (Jaecker-Voirol et al. Citation1987) as suggested by Paasonen et al. (Citation2012) (Almeida et al. Citation2013). In other words, all the H2SO4 and base molecules and H2SO4–base clusters are explicitly treated in the model, and water is implicitly taken into account by assuming equilibrium hydrate distributions for all the molecules and clusters. All the species except for water are assumed to be lost on the wall of the instrument at the diffusion limit. Including water explicitly in the model is in practice computationally impossible due to the approximately ten orders of magnitude difference in the characteristic collision and evaporation frequencies of water compared to those of all other compounds. This results from the extremely high concentration of water with respect to the other species, which is also the reason why the timescale of equilibration of the hydrate distributions can be assumed to be much faster than the timescales of the other kinetic processes, and why the wall loss of water can be neglected. As new particle formation is not assumed to occur in a diffusion measurement, clusters were not allowed to grow out of the simulated system. This was done by disabling collisions leading to clusters outside the system. Additional test simulations performed with different boundary conditions, described in the SI, showed in practice no changes in the results.
2.4.1. Simulated Conditions
The simulations were run by setting initial concentrations for H2SO4 and base monomers, and integrating the time development of the cluster concentrations for selected residence times (Section 2.4.2). As the acid concentration measured with SCIMS is likely to include contributions from H2SO4 molecules clustered with base molecules, both dry and hydrated, in addition to single H2SO4 molecules and its hydrates (Kurtén et al. Citation2011; Kupiainen-Määttä et al. Citation2013), the measurable final acid concentration was defined as the sum of all clusters containing one H2SO4 molecule and 0–2 base molecules. The initial acid monomer concentration at the beginning of the flow tube was set to 3 × 107, 3 × 108, and 3 × 109 cm−3, as the typical initial concentration in the experiment was reported to be (0.3–3) × 109 cm−3, with lower concentrations down to approximately 3×107 cm−3 at high RHs. The initial base concentration was first set to 2.5 × 107 cm−3 and 2.5 × 108 cm−3 (corresponding to typical atmospheric mixing ratios of 1 and 10 ppt, respectively) for all the RHs. However, a more realistic approach may be to assume that the contaminant base molecules enter the system with the water vapor, in which case the base concentration is proportional to the RH. Therefore, the initial base concentration [base]init was set to be linearly proportional to RH (in percent) as
[8] where [base]ref was set to 2.5×106 cm−3 (corresponding to a mixing ratio of 0.1 ppt in the atmosphere) to produce background level concentration values of a few ppt. For ammonia, also higher reference concentration [base]ref values of 2.5 × 107 cm−3 and even 2.5 × 108 cm−3 were tested, since atmospheric ammonia concentrations may be orders of magnitude higher than amine concentrations. All the simulations were performed at the standard temperature of 298.15 K and total pressure of 620 Torr. It must be noted that the pressure affects only the calculated diffusion coefficients (EquationEquation (5)
[5] ), as the evaporation rate coefficients, calculated from the quantum chemical Gibbs free energies of formation via detailed balance, are independent of it (Ortega et al. Citation2012).
2.4.2. Determining the Measurable pDeff from the Simulations
To estimate the effect of varying conditions in a realistic way, the effective diffusion coefficient pDeff was determined from the measurable wall loss coefficient (EquationEquation (1)[1] ) following the experimental procedure. Hanson and Eisele (Citation2000) measured the acid concentration [H2SO4] with an SCIMS at different sample residence times by varying the position of the acid source. Representative results are presented in in the study by Hanson and Eisele (Citation2000), which shows ln([H2SO4]/ [H2SO4]ref)) as a function of the distance z between the acid source and the SCIMS at a known flow speed v. Here, [H2SO4] is the measured acid concentration and [H2SO4]ref = 108 cm−3 is a reference concentration. The effective acid wall loss coefficient kwall (cm−1) was then obtained as the slope of a linear fit to the (z, ln([H2SO4]/[H2SO4]ref))—data by assuming that the acid concentration decays exponentially. Finally, kwall was converted from cm−1 to s−1 by multiplying by the flow velocity v, and pDeff was obtained from kwall according to EquationEquation (1)
[1] .
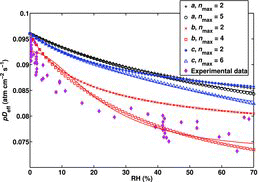
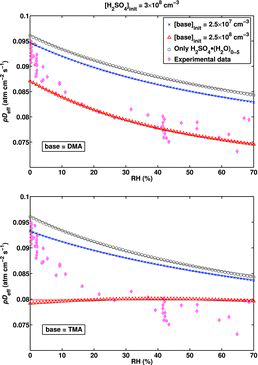
In this study, ACDC was used to simulate the time evolution of the molecule and cluster concentrations as the sample proceeds through the flow tube. The measurable final acid concentration, as defined in Section 2.4.1, was recorded at residence times t of 20, 27, 34, and 41 s, corresponding to the distances z in in the study by Hanson and Eisele (Citation2000). The simulated acid concentration was then presented as in by Hanson and Eisele (Citation2000) in (t, ln([H2SO4]/[H2SO4]ref))-coordinates. As in the experiment, a linear fit was applied to the data, and the effective wall loss coefficient kwall (s−1) was obtained as the slope of the fit. Again, pDeff was calculated as in EquationEquation (1)[1] . A demonstrative figure of the determination of kwall from the simulation data in representative conditions is presented in the SI.
3. RESULTS AND DISCUSSION
Theoretical effective diffusion coefficients as a function of RH in the studied conditions are presented in –. The data are depicted as separate markers, and the fits to the data according to EquationEquation (2)[2] are depicted as lines. Unless otherwise stated, the theoretical data are obtained using the B3LYP/CBSB7//RICC2/aug-cc-pV(T+d)Z cluster energies. The experimental data measured by Hanson and Eisele (Citation2000) are also shown for reference. The equilibrium constants K1 and K2 obtained from the fits, and their changes with respect to the actual input values are presented in . Note that following the convention of the results reported by Hanson and Eisele (Citation2000), the K constants are not at the standard state of 1 atm, but can be converted to it by multiplying by a factor of 100 × 1 atm/
, where
is the saturation vapor pressure of water (see Wexler Citation1976 for a parameterization for
). The fitting procedure and the goodness of the fits are briefly discussed in the SI. However, it must be noted that as the function given by EquationEquation (2)
[2] is not suited for the simulated data in all the studied cases, the quantitative results for the K constants should be examined with some caution. Moreover, as the absolute K values in any case depend on the quantum chemical dataset, the focus of the results is on the qualitative effects and the directions of the changes in the K constants.
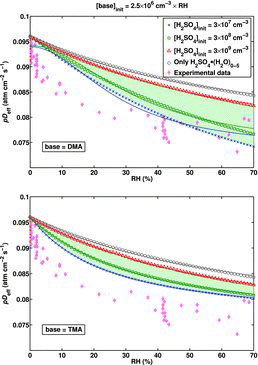
![Figure 5 Effective diffusion coefficient as a function of RH for the H2SO4–DMA system extended to include larger clusters, calculated using the B3LYP/CBSB7//RICC2/aug-cc-pV(T+d)Z Gibbs free energies. The shaded (green) area presents the spread of the results for pDeff as the initial H2SO4 concentration varies between 3 × 107 cm−3 and 3 × 109 cm−3. The upper limit of the shaded area corresponds to [H2SO4]init = 4 × 108 cm−3.](/cms/asset/9638d4be-d95e-4484-8d18-de2cc4e8d65d/uast_a_903556_f0005_oc.jpg)
3.1. Effect of Enabling Further Hydration
shows the effective diffusion coefficient for the system containing only single sulfuric acid molecules and its hydrates with the hydrate distribution determined from quantum-chemical Gibbs free energies calculated with different levels of theory, assuming that the H2SO4 molecule can be hydrated by up to nmax = 2 or nmax = 5 (or nmax = 4 in the case of the MP2/aug-cc-pV(D+d)Z//ΔE0,3-step data, for which the hydrate containing five waters had not been calculated, and nmax = 6 in the case of the RI-MP2/aug-cc-pVDZ//RI-MP2/CBS data, for which the hydrate containing six waters had also been calculated) water molecules. For figure clarity and the reason explained below, results obtained with the PW91PW91/6-311++G(3df,3pd) data are not shown in the figure. While the magnitude of the effect of including hydrates with more than two waters is dependent on the quantum chemical method, all the methods predict similar trends: as the formation of the larger hydrates is allowed, the value obtained for K1 decreases and the value for K2 increases. The changes are the largest in the case of the high-level method MP2/aug-cc-pV(D+d)Z//ΔE0,3-step, for which K1 decreases by two orders of magnitude and K2 increases by an order of magnitude, as shown in . For the B3LYP/CBSB7//RICC2/aug-cc-pV(T+d)Z and RI-MP2/aug-cc-pVDZ//RI-MP2/CBS methods, the changes are less than an order of magnitude. It has to be noted that the changes due to further hydration () are larger for K1 for the MP2/aug-cc-pV(D+d)Z//ΔE0,3-step method, and considerably larger for K2 for all the methods, than the error estimates of the experimental values K1 = 0.13 ± 0.06 (ΔK1 = ±46%) and K2 = 0.016 ± 0.006 (ΔK2 = ±38%) calculated by Hanson and Eisele (Citation2000).
As can be seen in , the MP2/aug-cc-pV(D+d)Z//ΔE0,3-step method predicts in general more hydration than the two other methods, for which the pDeff values are higher. The lower pDeff values obtained with this high-level method agree well with the measurements. Anyhow, conclusions of the presence of larger hydrates in the measurement cannot be drawn due to the scatter in the experimental data. The qualitative predictions regarding the larger hydrates agree: according to all three quantum chemical methods, the inclusion of the fourth hydrate nmax = 4 still affects the calculated pDeff compared to allowing hydration by up to three waters. According to the RI-MP2/aug-cc-pVDZ//RI-MP2/CBS and B3LYP/CBSB7//RICC2/aug-cc-pV(T+d)Z methods, increasing nmax from four to five (and also from five to six in the case of the RI-MP2/aug-cc-pVDZ//RI-MP2/CBS method for which the data for the sixth hydrate was available) does not in practice have any effect, indicating that the addition of the fifth water is not favorable at the studied temperature and RH values. The PW91PW91/6-311++G(3df,3pd) data include hydrates containing up to only three waters, and therefore the results obtained with the data were excluded from , as they might be altered by the inclusion of hydrates with four or more waters. The qualitative changes in K1 and K2 due to the incorporation of the third hydrate are similar to the results obtained with the other methods: K1 decreases and K2 increases, the changes in both constants being approximately an order of magnitude.
To test whether taking into account the larger hydrates affects the K1 and K2 obtained from the original experimental data, we extended the fit (EquationEquation (2)[2] ) to include hydrates with up to four waters, and applied the fit to the experimental data. Since the values obtained for the fitting parameters Kn with the nonlinear least-squares procedure may depend on the initial guesses given for them, different initial values were tested. The obtained Kn values are presented and discussed in more detail in the SI. Setting the initial value for K3 to be lower than that for K2 resulted in K1 and K2 values close to those obtained by including in the fit hydrates with up to only two waters; setting the initial value for K3 to be higher than that for K2 gave different K1 and K2 values with essentially the same goodness of fit. Therefore, neglecting the larger hydrates n = 3–4 in the case that their K coefficients are actually higher or comparable to those of the smaller hydrates n = 1–2 may distort the values obtained for K1 and K2. If the larger hydrates are unstable and their K coefficients are low, the experimental data can in principle be treated by including hydrates with up to only two waters; however, it cannot be inferred from the experimental data if this is the case.
3.2. Effect of Base Contaminants
Out of the base compounds studied in this work, DMA and TMA proved to be capable of having a significant effect at background-level concentrations. NH3 concentrations of a few hundred ppt or MMA concentrations of a few ppt have no effect at the studied acid concentration range, since the fraction of H2SO4 clustered with the base in the NH3 and MMA systems is negligible. This is due to the evaporation rate of the clusters being significantly higher than the corresponding collision rate. For example, the evaporation rate of the H2SO4•NH3 dimer is 3.2 × 104 s−1, and at an NH3 concentration of 2.5 × 109 cm−3, the collision rate of an H2SO4 molecule with an NH3 molecule is only 1.2 s−1. Corresponding evaporation and collision rates for the H2SO4•MMA dimer at an MMA concentration of 2.5 × 108 cm−3 are 4.7 × 10 s−1 and 1.2 × 10−1 s−1, respectively. DMA and TMA, on the other hand, are able to cluster with sulfuric acid efficiently. The essential difference between these two amines is the degree of clustering with H2SO4 as a function of RH. Although hydration increases the collision rates as the collision cross-sections increase, water molecules have a different effect on the stability of the H2SO4•DMA and H2SO4•TMA clusters: H2SO4•DMA is somewhat further stabilized, that is, its effective evaporation rate decreases, whereas H2SO4•TMA becomes less stable by the addition of water, and thus remains mainly unhydrated also at high RH. This can be explained by the different structures of these two alkylamines. As the number of methyl groups is two for DMA and three for TMA, DMA is capable of forming two hydrogen bonds, whereas TMA can form only one bond. Thus, in the case of H2SO4•TMA clusters, the water molecules can bind only to H2SO4 molecules, but in the case of H2SO4•DMA clusters, they can bind to both H2SO4 and amine molecules.
Table 2 Equilibrium constants K1 and K2 obtained from the fits (EquationEquation (2)
[2] ) to the theoretical effective diffusion coefficients presented in Figures 2–5, and their changes ΔK1 and ΔK2 with respect to the actual input values, defined as ΔKn = (Kn,fit−Kn,input)/Kn,input, for the systems where the changes in the simulation result were significant (note that the constants are not at the standard state of 1 atm). 95% confidence bounds for the absolute values Kn are stated in the parenthesis. N/A indicates that the fit could not be unambiguously applied because the initial acid and base concentrations as a function of RH are not known; Kn corresponding to specific concentrations are given in the square brackets
The effect of DMA and TMA at varying RH-independent amine concentration and initial sulfuric acid concentration of [H2SO4]init = 3 × 108 cm−3 is presented in . In the case of DMA (top panel), the effect on the slope of the (RH, pDeff)-curve (and thus also on the K constants) is insignificant, but the values of pDeff are systematically decreased. For TMA (bottom panel), the slope becomes gentler as the amine concentration increases and may approach zero (or even change sign) at high enough TMA concentration. This is explained by the H2SO4•TMA clusters becoming less stable as RH increases (see above), resulting in a smaller fraction of H2SO4 being clustered with the contaminant. This in turn increases the diffusivity of the H2SO4 species that can be detected by SCIMS, and consequently the measurable pDeff is increased. It must be noted that even if the slope is unaffected by the contaminant, as in the case of DMA, the apparent diffusion coefficient of the unhydrated sulfuric acid molecule at RH = 0% obtained from the measurement decreases if H2SO4 is actually clustered with the contaminant in dry conditions.
However, as there is no clear physical reason for the contaminant concentration to be constant throughout the experiments, and the water vapor is the most likely source of contaminants, we will from now on concentrate on the cases where the base concentration is proportional to RH as in EquationEquation (8)[8] . shows that both DMA and TMA have a significant effect on the slope of the pDeff curve at an RH-dependent amine concentration. Again, the slope is steeper at higher RH for DMA (top panel) compared to TMA (bottom panel), since as opposed to DMA, increase in RH actually decreases the degree of clustering in the case of TMA, but the assumption that the base concentration increases with RH compensates for the de-stabilizing effect of water on the H2SO4•TMA clusters.
A very important parameter affecting the pDeff values in the presence of base contaminants is the initial acid concentration [H2SO4]init, as illustrated in . Decreasing [H2SO4]init increases the fraction of H2SO4 clustered with the base, which correspondingly decreases the pDeff values. The effect on the slope depends also on the hygroscopicity of the H2SO4•base clusters, as discussed above. From the experimental point of view, the variation of the initial acid concentration during the experiment, for instance along the RH axis as in the measurement by Hanson and Eisele (Citation2000), on which the [H2SO4]init values used in the simulations are based, is a noteworthy issue: it may cause considerable extra scattering of the measurement data along the pDeff axis, and distort the slope of the curve. If [H2SO4]init varies between 3×107 cm−3 and 3 × 109 cm−3, the measured pDeff values may be anywhere in the shaded areas of . On the other hand, Hanson and Eisele (Citation2000) solved the [H2SO4]init from a fit to the measured final acid concentration as a function of residence time as described in Section 2.4.2. If the measurable acid concentration does not decay exponentially as it was assumed, the deduced initial concentration is incorrect. We tested the magnitude of this effect by solving the [H2SO4]init from the simulation data in the same manner, and found that in all the studied conditions, the difference between the true [H2SO4]init used as an input in the simulation and the one obtained from the fit was minor compared to the overall range in which the input concentration was varied; the difference compared to the true [H2SO4]init was found to be approximately 30% at its largest. As obtaining the modeled pDeff curve, and consequently applying the fitting method, requires more accurate knowledge on the [H2SO4]init as a function of RH than what is reported in the study by Hanson and Eisele (Citation2000), the K coefficients could not be determined in the case of a base-contaminated system. We provide only a couple of estimates of the effect of an RH-dependent amine concentration if [H2SO4]init is constant throughout the RH axis (): at [H2SO4]init = 3 × 108 cm−3 and [DMA]init = 2.5 × 106 cm−3 × RH, the changes in the K values obtained from the fit with respect to the actual values are ΔK1 = −94% and ΔK2 = 9159%. In the case of TMA, the corresponding changes are ΔK1 = 69% and ΔK2 = 183%, demonstrating that the magnitude and direction of the changes ΔKn depend on the identity of the contaminant species—and in addition also on the initial sulfuric acid concentration [H2SO4]init (even if it is constant), as different values are obtained at [H2SO4]init = 3 × 107 cm−3 and 3×109 cm−3 for both amines.
While different quantum chemical methods agree that amines bind to sulfuric acid more efficiently than ammonia (Kurtén et al. Citation2008; Nadykto et al. Citation2011; Ortega et al. Citation2012), according to some methods (Nadykto et al. Citation2011) the amine clusters are less stable than predicted by the B3LYP/CBSB7//RICC2/aug-cc-pV(T+d)Z method. In that case higher than merely background-level amine concentrations are required to produce a nonnegligible fraction of clustered H2SO4 molecules (see Kupiainen-Määttä et al. Citation2013 for a comparison of different quantum chemical methods regarding the formation of acid–DMA clusters). The results by Almeida et al. (Citation2013) show that the concentrations of clusters containing two H2SO4 molecules are significantly increased in the presence of DMA, indicating that DMA is capable of binding strongly to very small sulfuric acid clusters. However, until more definitive estimates on the stability of the H2SO4•amine dimers are available, we can state that the results presented in and provide predictions of the effect of amine contaminants in the case that the dimers are bound strongly enough to exist in sufficient amounts.
3.3. Effect of the Formation of Larger Clusters
Allowing the formation of H2SO4 dimers in the base-free system does not have an effect on the (RH, pDeff) curve compared to the system containing only hydrates with a single H2SO4 molecule, as the dimer, even when being stabilized by water to some degree, is not stable in the studied conditions. Adding an RH-dependent concentration of DMA and allowing the formation of clusters containing up to two H2SO4 and two DMA molecules may decrease or increase the measurable pDeff depending on the initial acid concentration (), which is explained in the following way: at [H2SO4]init = 3 × 107 cm−3, the acid concentration is too low for significant amounts of clusters containing two acids to be formed. H2SO4•DMA is the most abundant acid–DMA cluster, and has a decreasing effect on pDeff. At [H2SO4]init = 3 × 108 cm−3, two-acid clusters that are stabilized by DMA molecules, and thus acting as a sink for both acid and DMA, become more abundant. This effectively decreases the detectable acid concentration (that is, the sum of clusters containing one H2SO4 molecule), and the apparent wall loss coefficient increases. At [H2SO4]init = 3×109 cm−3, the absolute concentration of two-acid clusters is high enough for the major fraction of DMA molecules to be bound to them, and in practice only DMA-free H2SO4 molecules and its hydrates contribute to the detectable acid concentration. On the other hand, the relative concentration of the two-acid clusters is nonetheless low compared to the concentration of the H2SO4 hydrates, and consequently the effective diffusion coefficient is approximately equal to that of the system containing only H2SO4•(H2O)n species (). Since [H2SO4]init as a function of RH is not known, the K coefficients could not be determined as explained already in Section 3.2. The area where the results for pDeff are spread as the initial H2SO4 concentration varies between 3×107 cm−3 and 3 × 109 cm−3 is again presented as a shade (). To determine the upper limit of the shaded area in the case of the extended system, for which pDeff first increases and then decreases as [H2SO4]init increases, as explained above, we performed additional simulations at several [H2SO4]init between 3 × 107 cm−3 and 3 × 109 cm−3. Hence, the upper limit was found to be at [H2SO4]init = 4 × 108 cm−3, which corresponds to the highest pDeff values in the studied [H2SO4]init range.
4. CONCLUSIONS
We simulated the conditions of a flow tube measurement of the diffusion of sulfuric acid in humidified nitrogen performed by Hanson and Eisele (Citation2000), and determined the effective diffusion coefficient pDeff of H2SO4 as a function of RH as was done in the experiment. The simulations were performed using formation free energies of small hydrated clusters containing sulfuric acid computed with a quantum chemical multi-step method B3LYP/CBSB7//RICC2/aug-cc-pV(T+d)Z, cluster diffusion coefficients calculated according to the kinetic gas theory, and a dynamic collision-evaporation model. We applied the fitting method proposed by Hanson and Eisele (Citation2000) for obtaining the equilibrium constants K1 and K2 for the formation of the H2SO4 mono- and dihydrates, derived assuming that only the H2SO4•(H2O)0–2 clusters contribute to pDeff, and examined how the obtained values change in varying conditions where this assumption may not be valid.
The incorporation of H2SO4•(H2O)3–5 hydrates decreases the effective diffusion coefficient at higher RHs compared to the situation where only H2SO4•(H2O)0–2 species are assumed to be present, causing the fitted value obtained for K1 to decrease and for K2 to increase. We performed the calculations using several available sets of quantum chemical H2SO4 hydration energies, including data computed at higher levels of theory that are computationally too demanding for larger clusters, and found the qualitative results to be consistent with the predictions of the somewhat less accurate, but computationally affordable B3LYP/CBSB7//RICC2/aug-cc-pV(T+d)Z method.
The presence of a background-level concentration of a few ppt of contaminant base molecules, the most probable source of which is the water vapor, may have a significant effect on the measurable diffusion coefficient. According to the B3LYP/CBSB7//RICC2/aug-cc-pV(T+d)Z calculations, dimethylamine (DMA) and trimethylamine (TMA) are capable of forming stable acid–base dimers, which decreases the measurable diffusion coefficient given that also the H2SO4 molecules clustered with base are detected, as assumed in this study. If the clustered H2SO4 molecules would not be detected, the clustering would have the opposite effect on the measurement result, as the diffusion coefficient would in that case be overestimated. Ammonia and monomethylamine (MMA) were found to be incapable of binding to single H2SO4 molecules and thus have no effect on pDeff. The concentration of the contaminant molecules was assumed to be proportional to the RH, which steepens the slope of the (RH, pDeff)-curve for DMA throughout the RH axis, as water has a stabilizing effect on H2SO4•DMA dimers. H2SO4•TMA clusters are instead de-stabilized by water, resulting in the slope becoming less steep at higher RH. While extending the system to include clusters containing up to two H2SO4 molecules had no effect in the case of the base-free system, in the DMA-contaminated system the formation of the larger clusters may increase the measurable diffusion coefficient, as the larger clusters act as a sink for H2SO4 and DMA. On the other hand, at high enough acid concentration the fraction of two-acid clusters may be large enough to bind most of the DMA molecules, but small enough for the single H2SO4 hydrates to be the dominant species, resulting in approximately same pDeff values as in the H2SO4•(H2O)n system. To more profoundly understand the effect of bases on the diffusion coefficient deduced from experiments, a diffusion measurement similar to the one performed by Hanson and Eisele (Citation2000) should be repeated (1) with only sulfuric acid and water vapors, and (2) with sulfuric acid and water, and an intentionally added, known base concentration.
According to the results presented in this article, the K constants obtained with the fitting method of Hanson and Eisele (Citation2000) may vary up to approximately one or two orders of magnitude in the presence of species other than H2SO4•(H2O)0–2. If the experiment is contaminated by base molecules, the initial acid concentration [H2SO4]init is an important parameter affecting the measurable diffusion coefficient, as the degree of clustering depends on it. Variation of [H2SO4]init at different RH values may spread the measurement data along the pDeff axis, and thus have a prominent effect on the slope of the curve fitted to the data. While a better evaluation of the magnitude of this effect requires estimates of the initial acid concentration—as well as the base concentration—as a function of RH, this study provides qualitative predictions of uncertainties due to experimental conditions and the assumption regarding the degree of hydration of H2SO4.
ACKNOWLEDGEMENTS
We thank the CSC–IT Center for Science in Espoo, Finland, for computing time.
FUNDING
We thank ERC projects 257360-MOCAPAF and 27463-ATMNUCLE, Vilho, Yrjö and Kalle Väisälä Foundation and Academy of Finland projects No. 135054 and No. 266388 for funding.
SUPPLEMENTAL MATERIAL
Supplemental data for this article can be accessed on the .
Olenius_Diffusion_SI.zip
Download Zip (491.7 KB)REFERENCES
- Almeida, J., Schobesberger, S., Kürten, A., Ortega, I.K., Kupiainen-Määttä, O., Praplan, A.P., et al. (2013). Molecular Understanding of Sulphuric Acid-Amine Particle Nucleation in the Atmosphere. Nature, 502:359–363.
- Brown, R.L. (1978). Tubular Flow Reactors with First-Order Kinetics. J. Res. Natl. Bur. Stand. (US), 83:1–8.
- Chapman, S., and Cowling, T.G. (1952). The Mathematical Theory of Non-Uniform Gases. Cambridge University Press, Cambridge.
- Eisele, F.L., and Tanner, D.J. (1993). Measurement of the Gas Phase Concentration of H2SO4 and Methane Sulfonic Acid and Estimates of H2SO4 Production and Loss in the Atmosphere. J. Geophys. Res., 98:9001–9010.
- Erupe, M.E., Viggiano, A.A., and Lee, S.-H. (2011). The Effect of Trimethylamine on Atmospheric Nucleation Involving H2SO4. Atmos. Chem. Phys., 11:4767–4775.
- Froyd, K.D., and Lovejoy, E.R. (2003a). Experimental Thermodynamics of Cluster Ions Composed of H2SO4 and H2O. 1. Positive ions. J. Phys. Chem. A, 107:9800–9811.
- Froyd, K.D., and Lovejoy, E.R. (2003b). Experimental Thermodynamics of Cluster Ions Composed of H2SO4 and H2O. 2. Measurements and ab Initio Structures of Negative Ions. J. Phys. Chem. A. 107:9812–9824.
- Hanson, D.R. (2005). Mass Accommodation of H2SO4 and CH3SO3H on Water-Sulfuric Acid Solutions from 6% to 97% RH. J. Phys. Chem. A, 109:6919–6927.
- Hanson, D.R., and Eisele, F. (2000). Diffusion of H2SO4 in Humidified Nitrogen: Hydrated H2SO4. J. Phys. Chem. A, 104:1715–1719.
- Haynes, W.M., ed. (2014). CRC Handbook of Chemistry and Physics,94th ed. (Internet Version). CRC Press/Taylor and Francis, Boca Raton, FL.
- Henschel, H., Acosta Navarro, J.C., Yli-Juuti, T., Kupiainen-Määttä, O., Olenius, T., Ortega, I.K., et al. (2014). Hydration of Atmospherically Relevant Molecular Clusters: Computational Chemistry and Classical Thermodynamics. J. Phys. Chem. A
- Jaecker-Voirol, A., Mirabel, P., and Reiss, H. (1987). Hydrates in Supersaturated Binary Sulfuric Acid-Water Vapor: A Reexamination. J. Chem. Phys., 87:4849–4852.
- Kirkby, J., Curtius, J., Almeida, J., Dunne, E., Duplissy, J., Ehrhart, S., et al. (2011). Role of Sulphuric Acid, Ammonia and Galactic Cosmic Rays in Atmospheric Aerosol Nucleation. Nature, 476:429–433.
- Kuang, C., McMurry, P.H., McCormick, A.V., and Eisele, F.L. (2008). Dependence of Nucleation Rates on Sulfuric Acid Vapor Concentration in Diverse Atmospheric Locations. J. Geophys. Res., 113:D10209.
- Kulmala, M., Lazaridis, M., Laaksonen, A., and Vesala, T. (1991). Extended Hydrates Interaction Model: Hydrate Formation and the Energetics of Binary Homogeneous Nucleation. J. Chem. Phys., 94:7411–7413.
- Kupiainen-Määttä, O., Olenius, T., Kurtén, T., and Vehkamäki, H. (2013). CIMS Sulfuric Acid Detection Efficiency Enhanced by Amines Due to Higher Dipole Moments: A Computational Study. J. Phys. Chem. A, 117:14109–14119.
- Kurtén, T., Loukonen, V., Vehkamäki, H., and Kulmala, M. (2008). Amines are Likely to Enhance Neutral and Ion-Induced Sulfuric Acid-Water Nucleation in the Atmosphere More Effectively Than Ammonia. Atmos. Chem. Phys., 8:4095–4103.
- Kurtén, T., Noppel, M., Vehkamäki, H., Salonen, M., and Kulmala, M. (2007). Quantum Chemical Studies of Hydrate Formation of H2SO4 and HSO4–. Boreal Environ. Res., 12:431–453.
- Kurtén, T., Petäjä, T., Smith, J., Ortega, I.K., Sipilä, M., Junninen, H., et al. (2011). The Effect of H2SO4–Amine Clustering on Chemical Ionization Mass Spectrometry (CIMS) Measurements of Gas-Phase Sulfuric Acid. Atmos. Chem. Phys., 11:3007–3019.
- Kurtén, T., and Vehkamäki, H. (2008). Investigating Atmospheric Sulfuric Acid-Water-Ammonia Particle Formation Using Quantum Chemistry. Adv. Quantum Chem., 55:407–427.
- Leverentz, H.R., Siepmann, J.I., Truhlar, D.G., Loukonen, V., and Vehkamäki, H. (2013). Energetics of Atmospherically Implicated Clusters Made of Sulfuric Acid, Ammonia, and Dimethyl Amine. J. Phys. Chem. A, 117:3819–3825.
- Loukonen, V., Kurtén, T., Ortega, I.K., Vehkamäki, H., Padua, A.A. H., Sellegri, K., et al. (2010). Enhancing Effect of Dimethylamine in Sulfuric Acid Nucleation in the Presence of Water: A Computational Study. Atmos. Chem. Phys., 10:4961–4974.
- Lovejoy, E.R., and Hanson, D.R. (1996). Kinetics and Products of the Reaction SO3 + NH3 + N2. J. Phys. Chem., 100:4459–4465.
- Marti, J.J., Jefferson, A., Cai, X.P., Richert, C., McMurry, P.H., and Eisele, F. (1997). H2SO4 Vapor Pressure of Sulfuric Acid and Ammonium Sulfate Solutions. J. Geophys. Res. D., 102:3725–3735.
- McGrath, M.J., Olenius, T., Ortega, I.K., Loukonen, V., Paasonen, P., Kurtén, T., et al. (2012). Atmospheric Cluster Dynamics Code: A Flexible Method for Solution of the Birth–Death Equations. Atmos. Chem. Phys., 12:2345–2355.
- Nadykto, A.B., and Yu, F. (2007). Strong Hydrogen Bonding Between Atmospheric Nucleation Precursors and Common Organics. Chem. Phys. Lett., 435:14–18.
- Nadykto, A.B., Yu, F., Jakovleva, M.V., Herb, J., and Xu, Y. (2011). Amines in the Earth's Atmosphere: A Density Functional Theory Study of the Thermochemistry of Pre-Nucleation Clusters. Entropy, 13:554–569.
- Noppel, M., Vehkamäki, H., and Kulmala, M. (2002). An Improved Model for Hydrate Formation in Sulfuric Acid–Water Nucleation. J. Chem. Phys., 116:218–228.
- Olenius, T., Kupiainen-Määttä, O., Ortega, I.K., Kurtén, T. and Vehkamäki, H. (2013). Free Energy Barrier in the Growth of Sulfuric Acid–Ammonia and Sulfuric Acid–Dimethylamine Clusters. J. Chem. Phys., 139:084312.
- Ortega, I.K., Kupiainen, O., Kurtén, T., Olenius, T., Wilkman, O., McGrath, M.J., et al. (2012). From Quantum Chemical Formation Free Energies to Evaporation Rates. Atmos. Chem. Phys., 12:225–235.
- Paasonen, P., Olenius, T., Kupiainen, O., Kurtén, T., Petäjä, T., Birmili, W., et al. (2012). On the Formation of Sulphuric Acid–Amine Clusters in Varying Atmospheric Conditions and its Influence on Atmospheric New Particle Formation. Atmos. Chem. Phys., 12:9113–9133.
- Sorokin, A., Arnold, F., and Wiedner, D. (2006). Formation and Growth of Sulfuric Acid-Water Cluster Ions: Experiments, Modelling, and Implications for Ion-Induced Aerosol Formation. Atmos. Environ., 40:2030–2045.
- Temelso, B., Morrell, T.E., Shields, R.M., Allodi, M.A., Wood, E.K., Kirschner, K.N., et al. (2012). Quantum Mechanical Study of Sulfuric Acid Hydration: Atmospheric Implications. J. Phys. Chem. A, 116:2209–2224.
- Weber, R.J., Marti, J.J., McMurry, P.H., Eisele, F.L., Tanner, D.J., and Jefferson, A. (1997). Measurements of New Particle Formation and Ultrafine Particle Growth Rates at a Clean Continental Site. J. Geophys. Res. D., 102:4375–4385.
- Wexler, A. (1976). Vapour Pressure Formulation for Water in Range 0 to 100 °C. J. Res. Natl. Bur. Stand., 80A:775–785.
- Yu, F. (2006). From Molecular Clusters to Nanoparticles: Second-Generation Ion-Mediated Nucleation Model. Atmos. Chem. Phys., 6:5193–5211.
- Yu, H., McGraw, R., and Lee, S.-H. (2012). Effects of Amines on Formation of Sub-3 nm Particles and Their Subsequent Growth. Geophys. Res. Lett., 39:L02807.
- Zhang, R., Suh, I., Zhao, J., Zhang, D., Fortner, E.C., Tie, X., et al. (2004). Atmospheric New Particle Formation Enhanced by Organic Acids. Science, 304:1487–1490.