Abstract
We investigate, through simulation, a system of aggregating particles in the free molecular regime that undergoes a crossover from ballistic to diffusive motion. As the aggregates grow, the aggregate mean free path becomes smaller and the motion between collisions becomes more diffusive. From growth kinetics, we find that when the ratio of the aggregate mean path to the mean aggregate nearest neighbor separation reaches of the order of unity, a crossover to diffusive motion occurs. This ratio, called the nearest neighbor Knudsen number, becomes an important parameter in understanding aerosol aggregation in the free molecular regime.
Copyright 2014 American Association for Aerosol Research
1. INTRODUCTION
From understanding climate change to nanomaterial synthesis, aggregation of aerosol particles is of great importance in both natural phenomena and our technology (Friedlander Citation1977; Dahneke Citation1983; Family and Landau Citation1984; Oh and Sorensen Citation1997). Aggregation of dispersed aerosol particles can occur under a host of different conditions. In the simplest case, aggregates are formed of approximately the same sized monomers (roughly spherical in shape) that come together under stochastic thermal forces (interactions with the medium) and bind permanently on contact via van der Waals forces. Even within such constraints, a number of particle/medium properties can be varied that highly affect the growth rate, particle morphologies, and resulting cluster size distributions of aggregating systems. In a gaseous medium the pressure, temperature, and molecular mass of the gas are determining factors. The initial particle volume fraction can also be adjusted over a large range, from the very dilute to very dense. The variation of these and other parameters leads to the limiting cases of either ballistic or diffusive type motion of particles between collisions with each other.
All particles dispersed into a medium experience drag forces. If the particles are dispersed in a dense medium, the paths of the medium molecules impinging on the surface of the dispersed particle will be severely affected by those leaving the surface. This produces a “stick” boundary condition at the particle surface in what is known as the continuum regime with a continuum, Stokes drag. Stokes drag is inversely proportional to the particle effective mobility radius, which is the geometric radius a if the particle is spherical, and the shear viscosity, which is a continuum property of the medium. If the particles are dispersed in a rarefied medium, the paths of the impinging medium molecules are essentially unaffected by those leaving the particle surface. This produces a “slip” boundary condition at the particle surface in what is known as the free molecular regime with an Epstein drag. Epstein drag is inversely proportional to the effective cross sectional area of the particle with a mobility radius squared functionality. The parameter that quantifies the continuum to free molecular regime change is the Knudsen number, Kn = mfp/a, where mfp is the mean free path of the medium molecules. When Kn → 0, the continuum regime holds, when Kn → ∞, the free molecular regime holds.
Another important parameter in classifying aggregate motion in a medium is the diffusional Knudsen number KnD, which is the ratio of the suspended particle's persistence length, la to some other characteristic length (the persistence is the stopping distance used to calculate the Stokes number (Kulkarni et al. Citation2011) when the velocity has the equipartion value). An aggregate's (we shall henceforth consider the suspended particles as aggregates) motion is taken to be on a straight line over a distance equal to its persistence length. When KnD → ∞ the persistence length dominates all other length scales and the motion is considered ballistic. When KnD → 0 the persistence length is small compared to the system's other length scales and the motion is considered diffusive. The crossover between these two limits occurs when . When the system is cluster dilute, the only characteristic length scale is the linear size of the aggregate (Pierce et al. Citation2006). Here, we use a definition of KnD similar to that used by Rogak and Flagan (1991) and Gopalakrishnan and Hogan (2011), in the dilute limit
[1]
where kB is the Boltzmann constant, T is temperature, μij is the reduced mass between an aggregate and its collision partner, Rg,i is the radius of gyration of the ith cluster and Γij is the reduced drag coefficient between these two aggregates defined as
were Γi is the drag coefficient of the ith cluster. The collision partner for a given cluster is assumed to be its nearest neighbor. This definition of KnD is equivalent to the ratio of the collision partners’ reduced persistence length to their combined size.
As the system becomes more crowded, the nearest neighbor separation Rnn becomes another important length scale (Pierce et al. Citation2006). If the persistence length of the aggregate la is large compared to the nearest neighbor separation then the movement of aggregates between collisions would be on a straight line, that is, ballistic motion. But if la is small compared to Rnn, the aggregate will have to travel many such la s, which follow each other randomly, before it collides with another aggregate; then the movement is diffusive. This is illustrated in the where in part (a), the light shaded cluster moves ballistically whereas in part (b) it moves diffusively. With this physical picture in mind, we introduce a new diffusional Knudsen number based on the nearest neighbor separation as a characteristic length scale:
[2]
FIG. 1. (a) Illustration of a ballistic collision where the light shaded cluster does not make one full persistence length before colliding with its neighbor. (b) Illustration of a diffusive collision where the light shaded cluster makes several persistence lengths before colliding.
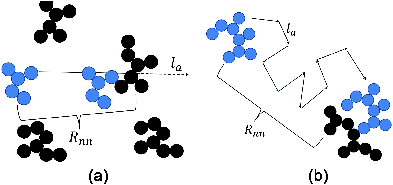
In this article, we present a simulation study of the aggregation of dispersed solid particles in the free molecular regime to demonstrate the effect of crossover between ballistic and Epstein diffusive type motion, a crossover that occurs due to cluster growth. Due to the evolving size of the aggregates or clusters, systems that initially aggregate in a ballistic fashion can cross over to Epstein diffusive as aggregation proceeds. The kinetics of aggregation, resulting cluster size distributions, and aggregate morphologies can all be affected by this crossover, and it is the results of these processes that this article addresses. We use two simulation methods to study this crossover: a direct integration of the Langevin equation (Mountain et al. Citation1986; Gopalakrishnan and Hogan Citation2011; Thajudeen et al. Citation2012) along with a simple and fast Monte Carlo aggregation algorithm that captures the essential physical characteristics of the crossover. The physical realization of this ballistic to diffusive crossover lies, for example, in highly sooting flames.
The rest of the article is organized as follows. In Section 2, we discuss both of our simulation algorithms. In Section 3, we describe the aggregation kinetics and how they are governed by the Smoluchowski equation. Next, Section 4 deals with the scaling of the aggregation kernel and the scaling of the aggregate mass distribution is discussed in Section 5. Results from our simulation studies are given in Section 6 and finally we give our concluding remarks in Section 7.
2. SIMULATION METHODS
Two simulation models, Langevin dynamics and Monte Carlo cluster-cluster aggregation, are used in this article. While Langevin dynamics simulations have been used in the past to describe aggregation in the free molecular regime (Nowakowski and Sitarski Citation1981; Mountain et al. Citation1986; Mulholland et al. Citation1988; Ranganathan and Hogan Citation2011), they are computationally expensive. We have developed a Monte-Carlo (MC) method for the crossover study similar to ones used in the past for continuum regimes (Pierce et al. Citation2006; Heinson et al. Citation2010). This MC method has the advantage of being computationally very efficient. Both simulations are constructed so that the crossover from ballistic to Epstein diffusive motion occurs as aggregation proceeded and the aggregate size increased. While the simulations differ in the mechanisms of aggregate movement, they share similar starting points. All simulations start with 100,000 monomers of radius a, diameter σ = 2a and mass mo that are placed at random in a 3D simulation box to obtain the desired volume fraction by adjusting the box size. If a monomer placed overlaps with another, it is given new set of random coordinates. Work presented here was done at volume fraction of . Periodic boundary conditions are in place to negate edge effects.
2.1. Langevin Dynamics
In this part, we integrate the Langevin equation for an aggregate:
[3]
where ma is the aggregate mass, Γ is the drag coefficient, R(t) the stochastic thermal force and r the position of a given aggregate center of mass. Aggregate mass can also be written as ma = Nmo where N is the number of monomers in an aggregate. The system is set to a constant temperature initially through the assignment of monomer velocities via the Maxwell-Boltzmann distribution. Solving EquationEquation (3)
[3] one finds: (Gunsteren and Berendsen Citation1982)
[4]
Here, the initial aggregate velocity is v(0) and the characteristic time,
is a measure of how long an aggregate will move along a ballistic trajectory before it feels the influence from the medium.
Integration of EquationEquation (4)[4] is done at time steps of Δt = 0.1 in reduced units of
. Reduced units of monomer mass mo = 1, monomer radius a = 0.5 and kBT = 1 are used to simplify the integration. When two aggregates collide they irreversibly stick, the total number of aggregates Nc is decremented by 1 and the new aggregate moves with a thermal velocity determined by equipartition.
Simulations presented here set out to model aerosol systems at low background gas densities often induced by high temperature and therefore are firmly in the free molecular drag regime. The free molecular drag coefficient is given as
[5]
In EquationEquation (5)
[5] , δ is the accommodation coefficient, P is pressure, mg is the mass of a gas medium molecule and Rm is mobility radius of the aggregate. Experiments and previous simulations have shown that the mobility radius for fractal aggregates is
[6]
where the mobility-mass exponent x = 0.46 (Wang and Sorensen Citation1999; Sorensen Citation2011). Now the drag coefficient becomes
[7]
The ballistic motion persists over a distance given by the product of the characteristic time, tc and thermal velocity
. Thus, the persistence length is written as
[8]
Notice that both the drag coefficient and persistence length have a power law dependence on N. Setting N = 1 yields the monomer persistence length
[9]
Rescaling EquationEquations (7)
[7] and Equation(8)
[8] by l0 provide a more compact from of Γ and la
[10]
[11]
From EquationEquation (10)
[10] it is clear that l0 and N define the drag coefficient and therefore are necessary to solve the Langevin equation. Thus, l0 along with fv and number of monomers, become a primary input in the simulation. All l0 values are given are in units of monomer diameter σ. Values of l0 = 1, 10, 50, 200, 10,000 were used in this work. We will show that when l0 = 1 the system acts as Epstein diffusion while l0 = 10,000 leads to purely ballistic motion.
2.2. Monte Carlo Cluster-Cluster Aggregation
Instead of solving the Langevin equation to move the aggregates, the Monte Carlo CCA method picks an aggregate at random, calculates a probability of movement Pm to determine if the aggregate moves, and then increments time by 1/Nc where Nc is the number of aggregates in the system including lone monomers. When the aggregate moves, it travels a distance of one monomer diameter σ. This model is standard for aerosol Monte-Carlo simulations in the continuum regime, where we differ is how Pm is calculated. In ballistic (BLCA) systems Pm is
proportional to the aggregate velocity and is set as (Pierce et al. Citation2006)
[12]
For Epstein diffusion the probability of movement is proportional to the aggregate's drag coefficient Γ and is set as (Pierce et al. Citation2006)
[13]
Our proposed crossover Pm must let an aggregate move ballistic on the scale of its persistence length la and diffusively on length scales larger than la. Also as an aggregate grows, its persistence length will decrease until la = 1 via EquationEquation (11)
[11] . Since in our simulation the minimum step size is one, at the la = 1 point, Pm must also crossover from ballistic to diffusive. Our proposed Pm is a linear combination of inverse of Pm,B and inverse Pm,D, that is, a harmonic sum of two probabilities.
[14]
Normalizing so monomers have Pm = 1 and crossover happens at la = 1 EquationEquation (14)[14] becomes
[15]
where 1 ≤ lo ≤ ∞.
We must note that the procedure for selecting Pm represented in EquationEquation (15)[15] is purely ad hoc. However, it captures the physics of the aggregate motion. We will test it by comparing to the Langevin result. EquationEquation (15)
[15] determines if an aggregate will move but does not say anything about the direction of movement. Since an aggregate must move on average a distance of la before it randomly changes direction due to the influence of the medium molecules, we calculate a probability of random direction change, Pr, that is checked every time an aggregate is moved. We chose to use the inverse of la for the probability of direction change:
[16]
As in EquationEquation (15)
[15] , Pr is purely ad hoc but still captures the physics of the system.
Care must be taken how time is incremented in this hybrid system where a cluster can move either ballistically or diffusively. The time, τD for an aggregate to move one monomer diameter by diffusion is given by the mean square displacement equation which yields
[17]
Comparing the time to move ballistically one monomer diameter
[18]
to τD and setting N = 1 and l0 = 1, the ratio of EquationEquation (17)
[17] to EquationEquation (18)
[18] is
[19]
In Monte Carlo simulations a cluster moves a distances of σ and time is incremented by 1/Nc but when the movement is diffusive the time scale needs to be normalized by EquationEquation (19)
[19] .
3. AGGREGATION KINETICS
The kinetics of aerosol aggregation are governed by the Smoluchowski equation, which describes how the number concentration of clusters of size N monomers, nN(t), changes with time (Friedlander Citation1977).
[20]
The aggregation kernel K(i,j) is the collision rate between aggregates made up of i monomers with aggregates of j monomers. K(i,j) is assumed to be a time-independent homogeneous function of particle size. To simplify the scaling we work under the assumption that the aggregation is between like-sized clusters, then the Smoluchowski equation becomes
[21]
Since K is homogeneous, one can write EquationEquation (21)[21] as
[22]
where λ is the degree of homogeneity, and K(1,1) is the kernel for a monomer–monomer collision. The aggregate size N changes with time and the concentration can be written as,
[23]
Then EquationEquation (22)
[22] becomes
[24]
Finally, integration yields
[25]
or
[26]
with a kinetic exponent, z as first seen by van Dongen and Ernst
[27]
and
[28]
Expanding EquationEquation (26)
[26] for small time t < t0 one finds that N(t) ∝ t. After this, small transient time, there is a transition when
, and then ultimately at large time when t > t0, the cluster size increases with power law N(t) ∝ tz. If the crossover happens during the linear transient regime, then information about the homogeneity will be absent. To avoid this below we simply graph average aggregate size
versus t0 = t; a process that by EquationEquation (26)
[26] linearizes a double logarithmic graph.
4. SCALING ANALYSIS OF THE AGGREGATION KERNEL
The previous section discussed how aggregation governed by the Smoluchowski equation yielded an average cluster size that increased with time via a power law with the kinetic exponent. Here, we present a simple scaling analysis that describes how the collision kernel and its homogeneity determine the kinetics for different regimes of motion. Beginning with the general statement that K is proportional to the colliding particles relative collision cross-sectional area, A and relative velocity, v, we write
[29]
4.1. Ballistic Regime
In the ballistic regime velocity is due to equipartition of energy and since
one finds from EquationEquation (29)
[29] :
[30]
Using the accepted value of fractal dimension,
, in the ballistic limit (Fry Citation2003), the homogeneity is found to be
and via EquationEquation (30)
[30] , hence the kinetic exponent is
.
4.2. Epstein Diffusion Regime
In the diffusion regime, the characteristic velocity is , where
is a characteristic diffusional length scale. In the dilute limit, the only length scale is
and from EquationEquation (29)
[29] one finds the homogeneity as
[31]
Aggregates in the Epstein diffusion regime have a fractal dimension of
which yields
and
. As the system becomes more crowded the relevant length scale becomes
. The nearest-neighbor separation is
where
is the number of clusters in the system. The total number of monomers,
is constant and equals
, thus
and the homogeneity is
[32]
In the Epstein limit, as the system becomes crowded, then, by EquationEquation (32)
[32] homogeneity is
and the kinetic exponent is
.
5. SCALING ANALYSIS OF THE CLUSTER SIZE DISTRIBUTION
As aggregation proceeds in the system, the aggregate size distribution develops a scaling form given by where n(N) is the number concentration of aggregates of size N, M1 is the 1st moment of the size distribution and s is the average size (van Dongen and Ernst Citation1985). The scaling variable is x = N/S and the scaled distribution function, ϕ(x) has the form
for large sizes (x > 1) (Oh and Sorensen Citation1997). Thus,
[33]
6. RESULTS
The aggregation kinetics for both Brownian dynamics and Monte Carlo simulations are shown in a–f. The graphs shown the inverse number of clusters versus t + t0 for various l0's. Since the number monomers remains constant,
is directly proportional to average cluster size. The constant t0 was calculated by EquationEquation (28)
[28] . The monomer aggregation kernel K(1,1) and z used in EquationEquation (28)
[28] were decided based on the initial KnD and Knn. Diffusive and nearest neighbor Knudsen numbers were found for each aggregate by EquationEquations (1)
[1] and Equation(2)
[2] , respectively, then averaged to obtain KnD and Knn for the system. For runs with l0 = 1 both KnD and Knn were less than unity and firmly in the diffusive regime, so values of diffusive K(1,1) and z were used. All other runs started with larger KnD and Knb and therefore ballistic values of K(1,1) and z were used to find t0. When l0 =1, the system is entirely diffusive due to both KnD and Knn being less than unity and yields a kinetic exponent of z = 0.8 for fv = 10−3 and 10−4 and z = 0.88 for the dense fv = 10−2. Both these values are consistent with predicted values of z = 0.73 for dilute Epstein diffusion and z = 0.88 for dense Epstein diffusion, respectively (Pierce et al. Citation2006).
FIG. 2. Inverse cluster count versus t + t0. t0 was found from EquationEquation (28)[28] . The lower dashed guide line has a kinetic exponent z = 0.80 − 0.88 and the upper guide line has z = 2.2. The points where Knn = 1 are marked and noted in the legend. The points where Knd = 1 are noted as stars in their respective runs. (a) Monte Carlo simulation at fv = 10−4; (b) Brownian Dynamics simulation at fv = 10−4; (c) Monte Carlo simulation at fv = 10−3; (d) Brownian Dynamics simulation at fv = 10−3; (e) Monte Carlo simulation at fv = 10−2; (f) Brownian Dynamics simulation at fv = 10−2. Notice that in (e) and (f) the Epstein diffusion exponent is z = 0.88 as expected when in the cluster dense regime. Also of note in (e) and (f) the trend has upward curvature do to the onset of gelation.
![FIG. 2. Inverse cluster count versus t + t0. t0 was found from EquationEquation (28)[28] . The lower dashed guide line has a kinetic exponent z = 0.80 − 0.88 and the upper guide line has z = 2.2. The points where Knn = 1 are marked and noted in the legend. The points where Knd = 1 are noted as stars in their respective runs. (a) Monte Carlo simulation at fv = 10−4; (b) Brownian Dynamics simulation at fv = 10−4; (c) Monte Carlo simulation at fv = 10−3; (d) Brownian Dynamics simulation at fv = 10−3; (e) Monte Carlo simulation at fv = 10−2; (f) Brownian Dynamics simulation at fv = 10−2. Notice that in (e) and (f) the Epstein diffusion exponent is z = 0.88 as expected when in the cluster dense regime. Also of note in (e) and (f) the trend has upward curvature do to the onset of gelation.](/cms/asset/e07a2f28-3718-44ae-842b-d7f1abf1c841/uast_a_922677_f0002_b.gif)
At large l0, 's parts all show for both types of simulation a kinetic exponent of z = 2.2 as expected for ballistic motion at all fv (Pierce et al. Citation2006). The intermediate values of l0 initially follow the ballistic track then evolve to the diffusive z exponents. For all intermediate l0 runs, the places where KnD = 1 are marked by a star and places where Knn = 1 are marked by symbols. While both Knudsen numbers fall within the crossover Knn does a better job of marking the beginning of the transition.
plots the aggregation kernel homogeneity λ versus either diffusional Knudsen number. By numerically finding z from the data in and using EquationEquation (27)[27] to find λ, we compare Knn to λ in Figure 3a and KnD to λ in Figure 3b. Closed symbols represent data from Monte Carlo simulations and open symbols are data from Brownian dynamics. All runs follow the same trend with a ballistic like upper limit of λ = 0.55 and an Epstein diffusional lower limit between λ = −0.36 for cluster dilute systems with fv = 10−4, 10−3 and λ = −0.14 for cluster dense systems with fv = 10−2. A crossover present when either Knudsen number is in the 0.1 to 10 range.
FIG. 3. (a) Aggregation kernel homogeneity λ versus nearest neighbor Knudsen number Knn for all Monte Carlo and Brownian Dynamic simulations. (b) The diffusive Knudsen number KnD versus homogeneity λ for all Monte Carlo and Brownian Dynamic simulations. Both measurements show similar behavior and provide a means of detecting the crossover.
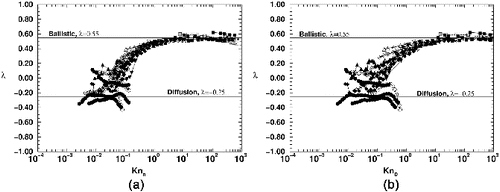
In a and b the size distributions at different times for the system at l0 = 50 and fv = 10−3 are shown for Brownian Dynamics and Monte Carlo simulations, respectively. The homogeneity is found from fitting EquationEquation (33)[33] . At early times when Knn > 1, the homogeneity is λ = 0.55 ± 0.1 consistent with ballistic motion, and at late time when Knn < 1, the system moves to λ = −0.4 ± 0.2 for the Brownian Dynamics runs and to λ = −0.3 ± 0.2 for the Monte Carlo runs, both of which are in the range of previous reported values of λ = −0.36 to −0.14 for Epstein diffusion (Pierce et al. Citation2006). The transition of λ can be seen more clearly by scaling EquationEquation (33)
[33] by
. and d show the rescaled mass distributions at different Knn values for runs of l0 = 50. Again as in and b, λ moves from a ballistic value to diffusive with changing Knn.
FIG. 4. Aggregate size distribution from Brownian Dynamics with l0 = 50 and fv = 10−3. Lines represent fits from EquationEquation (33)[33] . The system starts off with ballistic λ then enters an intermediate regime during the crossover and finally at late time and small Knn reaches a diffusive λ. (a) Size distribution from Monte Carlo simulations; (b) size distribution from Brownian Dynamics simulations; (c) rescaled data from (a), here the homogeneity λ transition from ballistic to diffusive can be clearly seen. The system starts with ballistic homogeneity of λ = 0.55 ± 0.1 then changes to λ = −0.3 ± 0.2, consistent to other values of λ reported for Epstein diffusion. (d) Rescaled data from (b), the system starts with ballistic homogeneity of λ = 0.55 ± 0.1 then changes to λ = −0.40 ± 0.2, consistent with Epstein diffusion.
![FIG. 4. Aggregate size distribution from Brownian Dynamics with l0 = 50 and fv = 10−3. Lines represent fits from EquationEquation (33)[33] . The system starts off with ballistic λ then enters an intermediate regime during the crossover and finally at late time and small Knn reaches a diffusive λ. (a) Size distribution from Monte Carlo simulations; (b) size distribution from Brownian Dynamics simulations; (c) rescaled data from (a), here the homogeneity λ transition from ballistic to diffusive can be clearly seen. The system starts with ballistic homogeneity of λ = 0.55 ± 0.1 then changes to λ = −0.3 ± 0.2, consistent to other values of λ reported for Epstein diffusion. (d) Rescaled data from (b), the system starts with ballistic homogeneity of λ = 0.55 ± 0.1 then changes to λ = −0.40 ± 0.2, consistent with Epstein diffusion.](/cms/asset/ca063c39-c4c1-47d9-844d-e9008fed6489/uast_a_922677_f0004_b.gif)
7. CONCLUDING REMARKS
We have performed simulations of the common yet previously unexplored aerosol situation in which the motion of the aggregates transforms from ballistic to Epstein diffusive while in the Free Molecular regime. Two algorithms were used. First, a slow but rigorous Brownian Dynamics method that solved for aggregate motion through the integration of the Langevin equation. Second, a less exact but faster Monte Carlo method that decides aggregate movement through use of an ad hoc probability of movement and probability of random walk. The probability of movement is a combination of the well-established probability of movements from ballistic and diffusive simulations and a probability of random walk insures aggregates on average move in a ballistic a distance of la. All simulations over all produced fractal aggregates of dimension 1.8 except for the very large l0 = 10,000 that yielded fractal dimension of 1.9. Both systems were in good agreement with regard to the time evolution of the nearest-neighbor and diffusion Knudsen number.
Volume fractions used went from the light to a dense
to highlight the importance of
as the dominant length scale in dense systems. To our surprise we found that at all volume fractions studied both nearest-neighbor separation and aggregate size were equally adequate at describing the crossover. In the future, simulations must be done with both denser and lighter systems to determine if both length scales do indeed remain markers of aggregation kinetics.
One physical situation in which the results of this article would apply is dense high temperature aerosols. Consider, for example, air at STP with a mean free path of 66 nm which means monomers of radius a = 10 nm would experience Epstein drag. Using an accommodation coefficient of δ = 1.36 for N2 gas yields by EquationEquation (9)[9] l0 = 30 nm. If these monomers had a volume fraction of fv = 10−6 then one finds Knn = 0.03, placing the system in the Epstein diffusion regime. As the aggregates grow from these initial conditions, the system would move to the continuum regime which is not accounted for in our simulations. Raising the temperature to that of typical flame experiments, T = 2100 K, the persistence length of the monomers grows to l0 = 210 nm yielding a Knn = 0.21, placing the system in the crossover regime between ballistic and diffusive motion, see . Raising the volume fraction in the flame to fv = 10−4 lowers the nearest neighbor separation which in turn gives a Knn = 1.00, in the middle of the ballistic to diffusive crossover. These latter conditions have been studied experimentally in the past 15 years (Sorensen et al. Citation1998, Citation2003; Kim et al. Citation2004, Citation2006; Chakrabarty et al. Citation2012). Finally, we remark that the reverse crossover from diffusive to ballistic aggregation can occur in dense systems near the gel point (Sorensen and Chakrabarti Citation2011).
FUNDING
This material is based upon work supported by the National Science Foundation under grant no. AGS 1261651.
REFERENCE
- Chakrabarty, R. K., Moosmuller, H., Garro, M. A., and Stipe, C. B. (2012). Observation of Superaggregates from a Reversed Gravity Low-Sooting Flame. Aerosol Sci. Technol., 46:I–Iii, doi:10.1080/02786826.2011.608389.
- Dahneke, B. (1983). Simple Kinetic Theory of Brownian Diffusion in Vapors and Aerosols, in Theory of Dispersed Multiphase Flow, R. E. Meyer, ed., Academic Press, New York, pp. 97–134.
- Family, F., and Landau, D. P., (1984). Kinetics of Aggregation, and Gelation. North-Holland, and Elsevier, Amsterdam.
- Friedlander, S. K. (1977). Smoke, Dust, and Haze: Fundamentals of Aerosol Dynamics. Wiley, Oxford University Press, New York.
- Fry, D., (2003). Aggregation in Dense Particulate Systems ( PhD diss.). Kansas State University.
- Fuchs, N. A. (1964). The Mechanics of Aerosols. Dover Publications.
- Gopalakrishnan, R., and Hogan, C. J. (2011) “Determination of the Transition Regime Collision Kernel from Mean First Passage Times. Aerosol Sci. Tech., 45:1499–1509.
- Gunsteren, W. F., and Berendsen, H. J. C. (1982) Algorithms for Brownian Dynamics. Mol. Phys., 45:637–647.
- Heinson, W. R., Sorensen, C. M., and Chakrabarti, A. (2010). Does Shape Anisotropy Control the Fractal Dimension in Diffusion-Limited Cluster-Cluster Aggregation? Aerosol Sci. Tech., 44:I–IV.
- Kim, W. G., Sorensen, C. M., and Chakrabarti, A. (2004). Universal Occurrence of Soot Aggregates with a Fractal Dimension of 2.6 in Heavily Sooting Laminar Diffusion Flames. Langmuir 20:3969–3973.
- Kim, W.G., Sorensen, C. M., Fry, D., and Amit Chakrabarti (2006). Soot Aggregates, Superaggregates and Gel-Like Networks in Laminar Diffusion Flames. J. Aerosol Sci., 37:386–401.
- Kulkarni, P., Baron, P. A., and Willeke, K. (2011) Fundamentals of Single Particle Transport, in Aerosol Measurement Principles, Techniques and Applications, P. Kulkarni, P. A. Baron, K. Willeke, eds., Wiley, Hoboken.
- Mountain, R. D., Mulholland, G. W., and Baum, H. (1986). Simulation of Aerosol Agglomeration in the Free Molecular and Continuum Flow Regimes. J. Coll. Interface Sci., 114:67–81.
- Mulholland, G. W., Samson, R. D., Mountain, R. D, and Ernst, M. H. (1988).Cluster Size Distribution for Free Molecular Agglomeration. Energy Fuels, 2:481–486.
- Nowakowski, B., and Sitarski, M. (1981). Brownian Coagulation of Aerosol Particles by Monte Carlo simulation. J. Coll. Interface Sci., 83:2196–2203.
- Oh, C., and Sorensen, C. M. (1997). Light Scattering Study of Fractal Cluster Aggregation Near the Free Molecular Regime. J. Aerosol Sci., 28:937–957.
- Pierce, F., Sorensen, C. M., and Chakrabarti, A. (2006). Computer Simulation of Diffusion-Limited Cluster-Cluster Aggregation with an Epstein Drag Force. Phys. Rev. E, 74:021411-1-021411-8.
- Rogak, S. N, and Flagan, R. C. (1991). Coagulation of Aerosol Agglomerates in the Transition Regime. J. Colloid Interface Sci., 151:203–221.
- Sorensen, C. M. (2011). The Mobility of Fractal Aggregates: A Review. Aerosol Sci. Tech., 45:755–769.
- Sorensen, C. M., and Chakrabarti, A. (2011). The Sol to Gel Transition in Irreversible Particulate Systems. Soft Matter, 7:2284–2296.
- Sorensen, C. M., Hageman, W. B., Rush, T. J., Huang, H., and Oh, C. (1998). Aerogelation in a Flame Soot Aerosol. Phys. Rev. Lett., 80:1782–1785.
- Sorensen, C. M., Kim, W., Fry, D., Chakrabarti, A. (2003). Observation of Soot Superaggregates with a Fractal Dimension of 2.6 in Laminar Acetylene/Air Diffusion Flames. Langmuir 19: 7560–7563.
- Thajudeen, T., Ranganathan, G., and Hogan, C. J. (2012). The Collision Rate of Nonspherical Particles and Aggregates for all Diffusive Knudsen Numbers. Aerosol Sci. Tech., 46:1174–1186.
- van Dongen, P. G. J., and Ernst, M. H. (1985). Dynamic Scaling in the Kinetics of Clustering. Phys. Rev. Lett., 54:1396–1399.
- Wang, G. M., and Sorensen, C. M. (1999). Diffusive Mobility of Fractal Aggregates Over the Entire Knudsen Number Range. Phys. Rev. E, 60:3036–3044