ABSTRACT
The structural characteristics of carbon nanoparticles synthesized by a spark discharge source were investigated by combined measurements of polydisperse number and Fuchs surface-area distributions against equivalent particle mobility and aerodynamic diameters. The number and Fuchs surface area distribution measurements were conducted by a scanning mobility particle sizer (SMPS) and a cascade epiphaniometer (CEPI), respectively. The CEPI-measured total Fuchs surface area and the Fuchs surface area calculated from SMPS number distributions were found to be in reasonable agreement (average absolute difference 28%), suggesting that the momentum-transfer diameter may be approximated by the mass-transfer diameter. The measured total Fuchs surface area of polydisperse distributions was reasonably reproduced by the Fuchs surface area of a monodisperse distribution (average absolute difference 26%). A fitting procedure was used to obtain the effective density mobility diameter relationship. The generated carbon aggregates, composed of primary particles of average diameter of 12.7 ± 2.5 nm, were determined to obey a fractal-like power law with a mass mobility fractal dimension and a prefactor
, in good agreement with theoretical calculations. Experimental measurements were compared to various theoretical models for the number of primary particles, the dynamic shape factors, and the effective density.
Copyright © 2016 American Association for Aerosol Research
EDITOR:
1. Introduction
Nanoparticles can be released into the air by several natural and anthropogenic sources. Fossil-fuel combustion and biomass burning comprise major sources of particulate matter within the urban atmosphere and the combustion-generated carbon-containing particles constitute a significant fraction of urban atmospheric aerosols. Nanotechnology deals with the production of nanoparticles generated to obtain improved composite materials with new properties and useful applications in various areas (Bhushan Citation2004; Strobel et al. Citation2006; Webster Citation2009; Trujillo-Reyes et al. Citation2014). Since nanomaterials are widely used in a variety of fields, concerns have also been raised regarding their environmental hazards and health risks (Brown et al. Citation2001; Oberdorster et al. Citation2007; Biskos and Schmidt-Ott Citation2012; Shang et al. Citation2014).
The physical and morphological characteristics of nanoparticles play an important role in a wide range of fields, strongly affecting their potential industrial and engineering applications, their environmental fate once released into the atmosphere, as well as their biological impact (Milanović et al. Citation2013; Pilou et al. Citation2015). Over the last decades, several studies have focused on the characterization of the structure and dynamic properties of aerosol nanoparticles with complex geometrical characteristics and self-similar structure (fractal-like aggregates/agglomerates). These studies aimed at the development and application of theoretical models and simulation techniques to parameterize nanoparticles properties, as well as the experimental determination of their physical and morphological parameters (Colbeck et al. Citation1989; Rogak et al. Citation1993; Virtanen et al. Citation2004; Sorensen Citation2011; Thajudeen et al. Citation2012; Mamakos et al. Citation2013; Melas et al. Citation2014b).
The physical properties of nonspherical nanoparticles composed of touching, nonoverlapping spherical monomers (e.g., soot particles) can be described by a number of parameters, including equivalent particle diameters. The equivalent diameters are used to model characteristic particle sizes of these nonspherical structures. The mobility of aerosol particles is of fundamental importance since it determines their transport, dispersion, and kinetics of their continued growth (Sorensen Citation2011). It can be expressed in terms of the equivalent mobility diameter , namely, the diameter of a spherical particle with the same mobility under the same flow conditions as the original particle. Another commonly used dynamic length is the equivalent aerodynamic diameter defined as the diameter of a standard density (
g/cm3) sphere that has the same gravitational settling velocity as the particle in question. Through combined measurements of the mobility and the aerodynamic diameters of nonspherical particles, additional information on particle morphology can be obtained, if the particle bulk density is known (DeCarlo et al. Citation2004).
Apart from their size, the unique properties of nanoparticles arise from their increased surface-to-volume ratio that enhances their reactivity. The surface area of nanoparticles is, thus, a key parameter in determining their properties and applications. Up to now, the available techniques for online and in situ measurements of particle surface area are based on the measurement of the mass transfer though the attachment rate of ions (active surface area) or radioactive atoms (Fuchs surface area) to the particle surface area. Either the active or Fuchs surface area of airborne nanoparticles defines the surface area that is available for interactions of the diffusing species (ions, lead atoms and gaseous species with sticking probability equal to unity) with the particles. The most commonly used instruments for real time active or Fuchs surface area measurements include the Diffusion Charger (DC, active surface area), the Epiphaniometer (EPI, Fuchs surface area) and more recently the Electrical Low Pressure Impactor (ELPI, active surface area) and the Cascade Epiphaniometer (CEPI, Fuchs surface area) (Gaggeler et al. Citation1989; Kasper et al. Citation2000; Marjamaki et al. Citation2000; Gini et al. Citation2013). The latter two instruments measure the surface area distribution of aerosol particles.
The instruments that are based on charging of aerosol particles through the diffusion of ions are most commonly used for particle (active) surface area measurements. However, questions arise about the way particle morphology affects charging efficiency and consequently, the instrument's response. Jung and Kittelson Citation(2005), who compared spherical particles and diesel agglomerates, observed variations in electrical charge from 15 to 17%. The diffusion of ions onto agglomerates was slightly larger than found onto spherical particle of the same mobility diameter. Ku and Maynard Citation(2005), using silver particles of morphologies that ranged from spherical to agglomerated particles, observed little difference in DC response for different particle shapes. Shin et al. Citation(2007) investigated the NSAM (Nanoparticle Surface Area Monitor, TSI Inc., Shoreview, MN, USA) response using NaCl and Ag agglomerates. The NSAM was developed to measure the nanoparticle surface area deposited in different regions of the human respiratory tract. Experimental data showed no significant dependence of calibration factors on particle material. While, more recently, Bau et al. Citation(2012) investigated the response of three instruments based on diffusion charging: LQ1-DC (Matter Engineering), NSAM (TSI), and AeroTrack 9000 (TSI). The results indicated that particle shape had a minor effect on the instruments' response. More specifically, for the LQ1-DC, they found that the active surface area was slightly greater for spherical particles than for quasi-fractal agglomerates, in contrast to theoretical predictions that the active surface area decreases as particles become more spherical. Based on the previous observations it can be concluded that although these devices can provide valuable information on the active surface area of airborne nanoparticles, care should be exercised related to uncertainties concerning charging efficiencies and electrostatic interactions.
On the other hand, the CEPI is a very sensitive instrument, with a low detection limit. Its principle of operation is based on the attachment of 211Pb atoms to the particle's surface area, which is not affected by electrostatic interaction (Rogak et al. Citation1991). However, its relatively slow response time (in the range of several minutes) and the signal saturation that occurs on large surface area concentrations, make its use for such studies very challenging. In this study, the surface area (concentration) distributions of carbon nanoparticle, generated by a spark discharge source, were measured directly by a CEPI. Then, a comparison was performed between the surface area calculated from the number distribution measurements and the surface area measured from CEPI to investigate whether the estimate of the active surface area of aggregate particles under the assumption of particle sphericity is a reasonable approach. Afterwards, the physical (surface area and effective density) and morphological parameters (Dm, Km, d1, Npp) of the produced polydisperse carbon nanoparticles were examined through combined measurements of their equivalent mobility and aerodynamic diameter distributions. These measurements were performed on the whole size range, instead of examining a specific narrow size range of the distribution by selecting monodisperse aerosols. The experimentally defined physical parameters of carbon aggregates were compared to empirical models and theoretical calculations that take into account the morphological characteristics of carbon aggregates.
2. Experimental procedure: Instrumentation and setup
2.1. Spark discharge generator
Carbon nanoparticles were synthesized by a spark discharge ionization source, b, (Fasmatech Science and Technology SA). Spark discharge ionization is a widely used technique to synthesize nanoparticles of uniform chemical composition in the gas phase (Biskos et al. Citation2008; Tabrizi et al. Citation2009; Meuller et al. Citation2012). The spark discharge source consists of a chamber which houses two opposing graphite electrodes, mounted at an adjustable distance, forming a gap of up to a few millimetres. The source is connected to a carrier inert gas of high-purity. The electrodes are connected to a high voltage supply (15 kV), in parallel to a capacitor which is charged by a constant current source continuously, increasing the voltage established between the two electrodes. When the capacitor reaches a sufficient voltage (gas breakdown voltage), a spark discharge occurs across the gap, ablating electrode material in the gas phase. The process of spark discharge is initiated by gas breakdown and formation of a conductive plasma channel. The spark lasts a few microseconds and reaches a local temperature of typically 20.000 K. The evaporated material is subjected to rapid cooling initially governed by adiabatic expansion and radiation and below the evaporation temperature by thermal conduction to the surrounding gas, producing high number concentrations of primary particles of a few nm in diameter. Further growth of these particles, due to coalescence or agglomeration, results in the production of nanostructured aggregates. The particle size and mass concentration of the produced nanoparticles can vary by adjusting the carrier gas flow rate, the spark energy and the spark frequency. A schematic diagram of the RCL circuit of the source is shown in a.
2.2. Cascade epiphaniometer (CEPI)
The Fuchs surface area distributions of the generated carbon nanoparticles were measured by a cascade epiphaniometer (Gini et al. Citation2013). The CEPI consists of a Gottingen online α-impactor (Sarad GmbH) combined with a 227Ac radioactive source (attachment chamber). The working principle of the CEPI depends on measuring the attachment rate of neutral 211Pb atoms onto the surface of the aerosol particles by counting the α-decay events of its daughter, 211Bi. The 211Pb atoms are produced at a constant rate by the decay of a short-lived radon isotope (219Rn) emitted from the long-lived artificial actinium source (227Ac), which is placed in the attachment chamber of the CEPI.
The aerosol stream was first drawn through the attachment chamber and then the 211Pb atoms attached to aerosol particles were transported to the impaction section through a diffusion trap which is attached to the entrance of the impactor, in order to minimize the number of the unattached atoms in the aerosol stream. The number of 211Pb atoms which were attached onto the particles was directly measured by Passivated-Implanted-Planar-Silicon-detectors (PIPS detectors), mounted below the impaction foils of each impactor stage. Mylar (Polyethylene Terephthalate) films of 3.5 μm thickness were used as impaction foils for each impactor stage, whereas PTFE filters of 2 μm in pore size were used below the last stage, as backup filters. Aerosol particles were deposited onto each stage according to their aerodynamic diameter. The aerodynamic cutoff diameters of CEPI range between 52 nm and 2 μm, at an operating flow rate of 11 lpm. The inversion algorithm described by Gini et al. Citation(2013) was applied in the raw data of CEPI in order to obtain the Fuchs surface area distribution of aerosol particles in real time. Since, the CEPI provides the size-fractionated surface area the total surface area of aerosol nanoparticle can be obtained by summing the instrument response of each impaction stage.
2.3. Scanning mobility particle sizer (SMPS)
The number distributions of the generated carbon nanoparticles were measured by a TSI scanning mobility particle sizer (SMPS). The SMPS consists of a condensation particle counter (CPC 3776, TSI) and an electrostatic classifier (EC 3080, TSI).
2.4. Experimental setup
The experimental set up used to generate and characterize carbon nanoparticles is presented in . Carbon nanoparticles were produced by the spark discharge ionization source. The generated particles were diluted with pure N2 and carried into a mixing chamber (100 lt) where they grew in size due to coagulation. A dry and filtered secondary (particle free) air flow (10 lt/min), forming a closed loop, was also circulated in the mixing chamber, leading to further dilution. Following, the mixed and homogeneous stream was drawn into the monitoring systems which consist of an SMPS and a CEPI. The SMPS system consists of an electrostatic classifier (EC 3080, TSI Inc.), incorporated with a 85Kr neutralizer, and a condensation particle counter (CPC 3776, TSI Inc.), probing the number-weighed mobility diameter distributions of the carbon nanoparticles. The CEPI was operated at an inlet flow of 11 lpm divided into two streams; the aerosol flow was 1.3 lpm, whereas for the additional 9.7 lpm dried and filtered air was drawn from a separate inlet (Gini et al. Citation2013). Occasionally, samples for SEM analysis were collected on a 47 mm Cyclopore membrane filter (Whatman, 1 nm pore size), placed in a custom-made filter holder which operated in parallel to the other instruments, at a flow rate of 1.3 lpm.
Given the limited size resolution of CEPI, carbon nanoparticles properties were examined using polydisperse aerosols. The range of variation of the produced polydisperse particle size distributions (i.e., number concentration and geometric mean diameter (GMD) of the number distributions) was achieved by varying the nanoparticles aging time, while the operating spark discharge voltage and the carrier gas flow were kept stable during the experiments.
3. Theoretical background: Nonspherical aggregates
3.1. Fuchs surface area
The particle Fuchs surface area was introduced by Pandis et al. Citation(1991) to interpret the signal of the epiphaniometer. They defined it as a dimensionless quantity, expressed through the coagulation coefficient between lead atoms and particles. It may be interpreted physically as the fraction of the geometric surface of a particle that is available for interactions with the surrounding gas, i.e., the effective particle surface area where mass and momentum exchange processes occur. In this study, based on the fact that the particle mobility is inversely proportional to the mass transfer coefficient (Siegmann and Siegmann Citation2000; Heitbrink et al. Citation2009), the Fuchs surface area of a single aggregate viewed as a virtual sphere of mobility diameter is calculated via (Equation (S7) in the online supplementary information [SI]):
[1] where
is the dimensionless momentum scattering coefficient, herein taken to be
(Zhang et al. Citation2012),
the gas mean free path,
the particle Knudsen number defined with respect to the equivalent mobility diameter, and
the corresponding Cunningham slip correction factor. The Fuchs surface area as defined by Equation (Equation1
[1] ) is valid in any flow regime, and it is proportional to the nondimensionalized Fuchs surface area (Pandis et al. Citation1991). It may be considered proportional to the geometric surface area for particle sizes lower than 100 nm (Gäggeler et al. Citation1989).
By combing the calculated Fuchs surface area of a single (virtual spherical) particle with the number concentration in each size bin as obtained from the SMPS-measured number distribution, the Fuchs surface area for a size bin of mean mobility diameter
is
[2]
The total surface area () is obtained by summing over the size intervals.
3.2. Number of primary particles
The theoretical determination of the number of primary particles in a fractal-like aggregate is essential for the calculation of numerous aggregate properties, including their effective density and mobility. In particular, the Aerosol Instrument Manager (AIM) software used to invert raw SMPS data relies on a theoretical estimate of the number of primary particles and their diameter to obtain the aggregate mobility diameter. Several approaches have been suggested to estimate
.
Experimental measurements and numerical simulations of synthetic fractal-like aggregates composed of nonoverlapping, point-contact, equal-sized spheres (monomers) suggest that scaling-like laws relate the number of primary particles to the diameter of gyration [3] and to the mobility diameter (Schmidt-Ott Citation1988)
[4] where the fractal dimension is
, the mobility fractal dimension is
, the fractal prefactor
, the mobility fractal prefactor
, and the diameter of the identical monomers is
. The diameter of gyration describes the mass distribution around the aggregates center of mass: it is one of the most frequently used characteristic geometric length scales associated with power-law aggregates. The fractal dimension is a descriptor of an aggregate's large-scale structure, whereas the fractal prefactor is a local-structure indicator (Melas et al. Citation2014a).
In addition to the determination from the scaling laws, the number of primary particles can be calculated as suggested by Lall and Friedlander Citation(2006). According to the Lall and Friedlander (L&F) model the number and diameter of the spherical primary particles that compose an aggregate and its mobility diameter are related through[5] where
is the dimensionless drag force, a constant that depends on the orientation of the particle. For aggregates that align parallel to the electric field in a differential mobility analyzer (DMA) or electrostatic analyzer (EC), and consequently parallel to the aggregate motion,
. The L&F model is valid for low fractal dimension aggregates,
, composed of uniform, spherical primary particles in the free molecule size range (corresponding, roughly, to
).
Sorensen Citation(2011), in the comprehensive review of the mobility of fractal aggregates generated primarily via Diffusion Limited Cluster Aggregation (DLCA aggregates), summarized experimental measurements and theoretical investigations. The morphological characteristics of DLCA aggregates are described by the pair . He concluded that, for relatively small aggregates and irrespective of the gas Knudsen number, the number of primary particles can be related to the mobility diameter by:
[6]
An alternative way to relate mobility properties to the number of primarily particles is described in the following subsection where an iterative scheme, based on equivalent diameters (the adjusted-sphere and continuum-regime mobility diameters), is proposed.
3.2.1. Equivalent mobility diameters
The adjusted-sphere method (ASM) provides a computational tool to determine the mobility diameter of nonspherical particles across the entire (momentum) transfer regime (Dahneke Citation1973). According to the ASM, a virtual adjusted sphere is introduced whose diameter is constant and independent of flow conditions (as specified by the gas mean free path). It is defined as a sphere that has the same slip factor as the irregularly shaped object across the entire transition regime. It can be shown (Melas et al. Citation2014b, Citation2015) that the adjusted-sphere diameter, the mobility diameter at an arbitrary gas Knudsen number, and the continuum-regime mobility diameter
are interrelated across the entire transition regime via
[7]
Furthermore, empirical expressions for the adjusted-sphere diameter and , which reproduce accurately the mobility properties of synthetic aggregates, have been proposed (Melas et al. Citation2014a,Citationb). The proposed expression for the adjusted-sphere diameter is
[8] and the expression for the continuum-regime mobility diameter is
[9]
Equation (Equation7[7] ), in conjunction with the two empirical expressions, may be used to infer properties of fractal-like aggregate. The number of primary particles may be estimated if the morphological characteristics of the aggregates, as described by the fractal-law parameters
, are considered known. For a given mobility diameter of an aggregate the number of primary particle may be determined iteratively. Specifically, an initial guess is made for
, the corresponding diameter of gyration is determined from the scaling law, the adjusted sphere-sphere and continuum mobility diameters are calculated from the empirical expression, and the validity of Equation (Equation7
[7] ) is tested. If the equality is not valid, the number of primary particles is modified, and the procedure is repeated till Equation (Equation7
[7] ) is satisfied.
This iterative procedure gives the number of primary particles an aggregate is composed off, if the mobility diameter and the morphological characteristics of the aggregate are known. Alternatively, the procedure may be performed in reverse order. For example, provided that is given by the Mobility Scaling Law, Equation (Equation4
[4] ), the diameter of gyration and the two equivalent radii may be calculated. The solution of Equation (Equation7
[7] ), then, gives the mobility diameter of the aggregated composed of the specified number of primary particles. We shall use both procedures in Section 4.
3.3. Effective density and particle morphology
The effective density differs from the particle (bulk) density when the particle contains internal void spaces. Various definitions of effective density are available in literature, depending on the methodology employed to determine it (DeCarlo et al. Citation2004). A frequently used definition is obtained by relating different equivalent particle diameters, in particular, the aerodynamic (da) and mobility diameter. The particle effective density based on the aerodynamic diameter , and the mobility and aerodynamic diameters are related through an expression that accounts for irregularities in particle shape and internal voids within the particle via (Maricq and Xu Citation2004; Kelly and McMurry Citation1992):
[10] where
is the slip correction factor with respect to the aerodynamic Knudsen number, and
the unit density (1 g/cm3). The slip correction factors are calculated at the CEPI stage pressure where the majority of the particles were collected.
In this study, the effective density of arbitrarily-shaped carbon nanoparticles was experimentally determined by parallel measurements of size distributions using two instruments: the SMPS that measures number distributions as a function of equivalent mobility diameters and the CEPI that measures Fuchs surface-area distributions as a function of aerodynamic diameters. First, the SMPS-measured number distributions are converted to surface-area distributions as a function of mobility diameters, , via the theoretical expression for the Fuchs surface area, Equation (Equation1
[1] ). The effective density is obtained iteratively via Equation (Equation10
[10] ), an iterative procedure that requires that the calculated surface-area distribution based on the mobility diameter
match the measured surface-area distribution
as a function of the aerodynamic diameter. The optimum effective density is determined by minimizing the difference (root mean square error [RMSE] in %) between measured and calculated size distributions via the Nelder–Mead simplex algorithm
[11] where
is the number of size bins of the CEPI size distribution. The optimization was implemented in MATLAB via the nonlinear curve- and data-fitting function lsqcurvefit (available in the Optimization Toolbox).
In the case of fractal-like aggregates, the effective density is also related to the equivalent mobility diameter via (Equation (S10) in the SI)[12]
The two definitions for the effective density are considered equivalent, , for the particle-size range under investigation (Mamakos et al. Citation2013; Schmid et al. Citation2007). Therefore, the mobility fractal dimension and prefactor can be calculated by plotting the experimentally determined effective density
against the mobility diameter.
3.4. Dynamic shape factor
The dynamic shape factor is a correction factor that accounts for the effect of shape on particle motion. It is defined as the ratio of the resistance force (typically the drag force) on the nonspherical particle to the resistance force on its equivalent volume sphere of diameter
when both move at the same relative velocity with respect to the gas (Hinds Citation1999). The equivalent volume diameter is the diameter of a virtual sphere that has the same volume as the volume of the irregularly shaped particle,
[13]
For spherical particles the dynamic shape factor is unity, whereas for nonspherical particles it is almost always greater than 1. It is related to the equivalent mobility diameter via (Schmid et al. Citation2007):[14] where
is the equivalent volume Knudsen number. If the effective density
and the bulk particle density
are known, it may be obtained from:
[15] a relationship that arises by combining Equations (Equation12
[12] ) and (Equation13
[13] ).
4. Results and discussion
4.1. Primary particle diameter: SEM image analysis
Additional information on the structure of the produced nanoparticles and the average size of the primary particles was obtained by scanning electron microscopy (SEM). The generated-by-spark discharging carbon nanoparticles were collected on Cyclopore membrane filters (Whatman, 1 nm pore size) and examined by low-voltage scanning electron microscopy (field emission gun scanning electron microscope [FEGSE-JSM7401F], JEOL). To minimize the strong charging effects that occur on insulating samples, Pt metallization of the surface was performed using the standard sputtering technique to deposit no more than 3 nm of Pt. This way, improved image quality was achieved combined with a minimum effect on the morphology of the sample. A total of 100 primary particles were analyzed using the ImageJ software. The repeated measurements from SEM images allowed the calculation of the primary particle diameter with reasonable accuracy despite the observed necking. The carbon nanoparticles were composed of primary particles of mean diameter ().
4.2. Fuchs surface area of carbon aggregates: Combined SMPS-CEPI measurements
The number (concentration) distributions of the generated nanoparticles were measured by a SMPS unit. They were obtained by inverting the raw data using the AIM (Version 8.0) software (TSI Inc.) that incorporates a theoretical expression for the aggregate drag and a charging efficiency correction. The most widely accepted charging theory for spherical particles is the Fuchs model. However, aggregate nanoparticles acquire different number of charges than spherical particles of the same mobility diameter. Aggregates become more charged because the charge may be distributed over larger areas in the agglomerate compared to a spherical particle of the same mobility size (Jung and Kittelson Citation2005; Rogak and Flagan Citation1992). The AIM software uses the charging efficiency model proposed by Wen et al. Citation(1984) to determine the aggregate charge distribution as a function the mobility diameter. The software also considers the drag force that aggregates experience in the electric field. The L&F model proposed by Lall and Friedlander Citation(2006) is used, according to which the drag force can be expressed in terms of the number and the diameter of primary particles, an expression that leads to Equation (Equation5[5] ).
The L&F model is valid under well-specified assumptions (idealized chain aggregates). Therefore, uncertainties in the determination of the equivalent mobility diameter may arise due to the distribution of sizes of the primary particles, the formation of necks between the primary particles, the assumption that aggregates form straight chains, as well as the alignment of the particles in the DMA. In the SI, the data analyses presented in the following sections were repeated using the AIM inversion algorithm option for spherical particles. Since the generated carbon nanoparticles are neither spheres nor “idealized chains,” we surmise that the true number distributions would lie in between these two extremes.
The generated polydisperse distributions were fitted to unimodal lognormal distributions with Geometric Mean Diameters (GMD) between 27 nm and 113 nm. The geometric standard deviations () were in the range 1.42 and 1.77, with a mean of 1.60. compares the total Fuchs surface area calculated from the polydisperse SMPS-measured number distributions,
, via Equation (Equation1
[1] ), to the total Fuchs surface area measured by CEPI,
. An average absolute difference of 28% (±20%) between the measured and calculated surface areas was observed for distributions with GMDs between 27 nm and 113 nm. The per-particle normalized experimental surface areas (from CEPI) as derived from the polydisperse distributions and the theoretically calculated per particle Fuchs surface areas of spherical particles against the particle mobility diameters are shown in . The comparisons shown in the two figures suggest that the attachment rate of neutral species onto aggregates is comparable to that of spherical particles with equal mobility diameters at least for particles in the transition regime, confirming earlier studies (Rogak et al. Citation1991; Shi et al. Citation2001). The observed differences may be attributed to several factors: particle morphology that influences the charge distribution of aggregates, the drag force acting on arbitrarily shaped particles, aggregate orientation in the electrostatic classifier under the influence of the electric field, primary particle diameter polydispersity and the relatively low time resolution of CEPI affecting measurements with high variability of the number concentrations.
Figure 4. Comparison of the SMPS-calculated total Fuchs surface area with the CEPI-measured total Fuchs surface area.
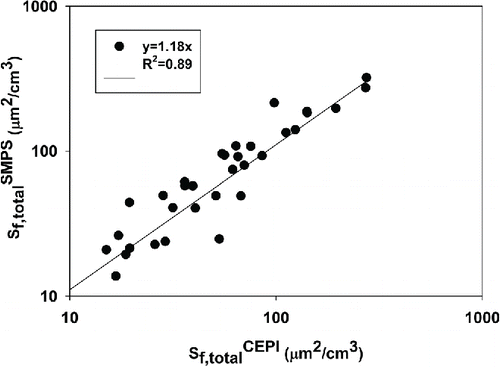
Figure 5. Comparison of theoretical and measured Fuchs surface area per particle. The normalized Fuchs surface area was obtained by dividing the CEPI response by total number concentration obtained from SMPS measurements.
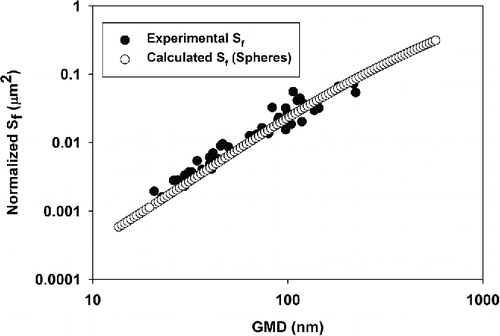
This comparison provides support to the use of the equivalent mobility diameter as the characteristic length scale to determine the Fuchs surface area. It implies that the mass-transfer mobility diameter (a quantity that determines the active surface area measured by the CEPI) may be taken to be approximately equal to the momentum-transfer diameter (a quantity that determines stress transfer and hence the drag a particle feels and eventually the mobility of a nonspherical structure, as measured by the SMPS), at least in the experimental size range under investigation, in agreement with the experimental results of Rogak et al. Citation(1991).
We have also to point out that the analysis of the SEM image showed that necking exists between the primary particles. The effect of necking has to be taken into consideration when the particle surface area is calculated solely by the surface area of the primary particle (Svensson et al. Citation2015). However, in this study the Fuchs surface area was determined experimentally through the equivalent mobility diameter which is a function of particle size and shape.
Additionally, we investigated whether properties of polydisperse aerosols can be reproduced by monodisperse aerosols of diameters equal to the lognormally fitted GMDs and total particle number concentration equal to the total aggregate number concentration (). We compared the total CEPI-measured Fuchs surface area
to the Fuchs surface area determined from the SMPS number distribution [
] assuming that the polydisperse aerosol may be represented by a monodisperse aerosol. The results are shown in . Inspection of the Figure shows that the CEPI-measured Fuchs surface area, corresponding to a polydisperse aerosol, is well correlated (coefficient of determination
) with the calculated total surface of an equivalent monodisperse aerosol. The average absolute difference between calculated and measured Fuchs surface area is 26 ± 22%. The parameters of the monodisperse aerosol were determined from the fit of the polydisperse size distribution to a unimodal lognormal distribution. In addition to the total surface area, the shape factor was calculated at the GMD and the
limits of a typical polydisperse distribution: the results, shown in the SI, suggest that the approximation is reasonable. We may conclude that some physical and morphological characteristics of a unimodal polydisperse aerosol may be approximated by the properties of a monodisperse aerosol, of diameter the GMD of the original distribution.
4.3. Structural characteristics of carbon aggregates: Combined SMPS-CEPI measurements
Several studies have examined the morphology and effective density of fractal-like aggregates (e.g., fresh soot particles), formed by diffusion-limited cluster aggregation (DLCA). One of the most commonly used techniques is the Tandem DMA-ELPI set-up. Maricq and Xu Citation(2004) employed that method to measure the effective density of soot particles from premixed flames and motor vehicle exhaust to show that their effective density ranged from 1.2 g/cm3 to <0.3 g/cm3, for particle sizes between 30 nm and 300 nm. Ristimaki et al. Citation(2002) presented another on-line method for simultaneous size distribution and effective density measurements, based on parallel measurements of the size distributions by SMPS and ELPI. The effective density can also be determined using the DMA-APM (aerosol particle mass analyzer) or the DMA-CPMA (Couette Centrifugal Particle Mass analyzer) instruments (McMurry et al. Citation2002; Shapiro et al. Citation2012; Johnson et al. Citation2014). Even though DMA-APM and DMA-CPMA have high enough resolution to distinguish externally mixed particles, measurement uncertainties are associated with particle-shape-dependent charging processes for both instruments, electrostatic interactions between ions and particles, and low signal to noise ratio due to the narrow transfer function of the DMA. Moreover, since the effective density is obtained from particle mass measurements (APM/CPMA), a small error in the mobility diameter would lead to a much larger error in the effective density calculation as the mass depends on (McMurry et al. Citation2002; Johnson et al. Citation2013).
Up to recently, aggregate morphological characteristics have been examined using monodisperse, size-selected aerosol particles. In this study, carbon nanoparticles were investigated through parallel measurements of whole size distributions. We used the methodology described in the theoretical background, Section 3.3, Equations (Equation10[10] ) and (Equation11
[11] ), to determine the average effective density of the carbon nanoparticles as a function of the GMDs of the polydisperse aerosols. The experimentally-determined effective density, as well as the predictions of various theoretical models, is presented in . The aerodynamic-mobility effective density, normalized by the bulk material density, is shown as a function of the geometric mean diameter of the number distributions measured by the SMPS, normalized by the primary particle diameter. It can be seen that the effective density decreases as the geometric mean diameter increases, ranging between 1.31 g/cm3 and 0.35 g/cm3. We estimate the uncertainty in the experimentally determined effective density to be lower than 20%, an estimate that is comparable to other techniques. Specifically, the uncertainty was determined by test measurements of artificially generated aerosols of known properties and nearly spherical shape: DEHS (Di-Ethyl-Hexyl-Sebacat), NaCl, and (NH4)2SO4. Parallel measurements of the generated aerosols were conducted by the CEPI and the SMPS unit, under relative humidity controlled conditions (Gini et al. Citation2013). The experimental errors (average absolute difference between
and
) was found to be 7.9% for DEHS, 18.9% for NaCl and 14.9% for (NH4)2SO4.
Figure 7. Experimental and calculated effective density (normalized by the bulk particle density) as a function of the geometric mean mobility diameter (normalized by the primary particle diameter). Symbols are described in the main text.
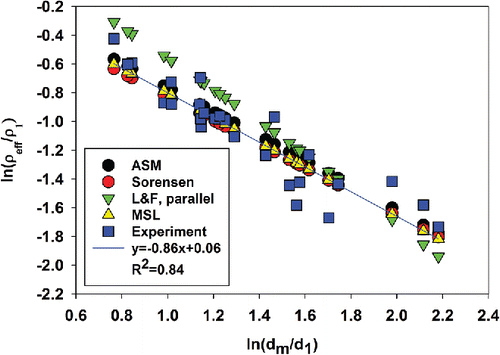
By plotting the effective density against the GMDs the average mass-mobility fractal dimension was determined to be and the average mobility prefactor
, for material density
g/cm3. The mass-mobility fractal dimension is similar to literature-reported values for soot aggregates generated by different sources and measured using different methodologies. For example, Mamakos et al. Citation(2013) found that the mass-mobility exponent for soot particles generated by a mini-CAST varied between 2.1 and 2.31. Maricq and Xu Citation(2004) determined the mass-mobility fractal dimension for flame-generated soot to be 2.15 ± 0.1, whereas a higher fractal dimension was found for diesel exhaust aggregates. Rissler et al. Citation(2013) found that the mass-mobility relationship for soot aggregates could be described by 2.3 (± 0.1) for sources with a volatile mass fraction lower than 10% and primary particle sizes 11–29 nm (diesel engines, flame soot generator and tapered candle).
It should be stressed that the experimentally determined mass-mobility fractal dimension and prefactor, defined by Equation (Equation4[4] ), differ from the fractal dimension and prefactor, Equation (Equation3
[3] ), although they are related. Melas et al. Citation(2014b) studied their relationship for different (gas) Knudsen numbers (
). They concluded that the pair
depends on the pair
, with a weak dependence on the Knudsen number. They argued that DLCA aggregates, characterized by
, have
when
at atmospheric pressure and
at lower pressures as the (gas) Knudsen number tends to infinity. Both predictions are in reasonable agreement with the scaling-law parameters obtained from the measured effective density, although the theoretically calculated prefactor is consistently slightly larger. This comparison, along with the general consensus that the formation of soot aggregates is a diffusion limited process, suggests that the formed carbon aggregates are DLCA aggregates. Theoretical predictions are also shown in : black circles are the predictions of the Adjusted-Sphere Method (ASM); red circles are predictions of the Sorensen Citation(2011) model; and inverted green triangles are predictions of the L&F model. All theoretical predictions are based on a calculation of the number of primary particles: the ASM uses the iterative procedure described in Section 3.2.1, Sorensen the empirical expression Equation (Equation6
[6] ), and L&F Equation (Equation5
[5] ). The effective density is subsequently calculated via Equation (Equation12
[12] ). The yellow triangles are predictions of Mobility Scaling Law (MSL, Equation (Equation4
[4] )) with the mobility fractal dimension
and prefactor
as experimentally determined by the coupled SMPS-CEPI measurements: as such, it is expected that they lie on the least-squares fit (solid line). Although the scatter of the experimental data is significant (maximum relative deviation from the best-fit line 26%), calculated effective densities and densities predicted by the ASM and Sorensen are in reasonable agreement with the experimental data (for the size range under investigation). The L&F predictions, however, seem to over predict significantly the effective density at low mobility diameters, eventually under predicting it as the mobility diameter increase, suggesting an incorrect slope. The average absolute difference between the experimental and the calculated effective densities is approximately 12% (ASM), 11% (Sorensen), and 22% (L&F).
Since the number of primary particles is an essential ingredient in the calculation of the effective density we present the theoretically predicted number of primary particles in . The experimental numbers (black diamonds) were obtained by inverting Equation (Equation12[12] ), given the experimentally determined effective density. The theoretical predictions were determined as described in the previous paragraph. In agreement with the results shown in , the ASM, Sorensen and MSL predictions are in excellent agreement, the L&F predictions deviating more than other model predictions from the experimental data as the mobility diameter increases. In general, the difference between the calculated and the experimentally-determined number of primary particles increases as the mobility diameter increases.
Figure 8. Calculated number of primary particles as a function of the geometric mean mobility diameter of the SMPS number distributions. The primary-particle diameter was 12.7 nm. Symbols are described in the main text.
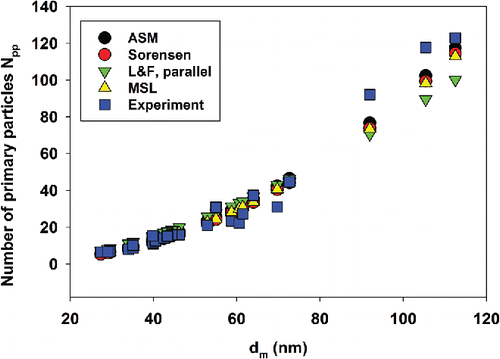
The measured and calculated dynamic shape factor of the generated aggregates as a function of the geometric mean diameter of the number distribution measured by the SMPS are shown in . The size range (equivalent mobility diameter) is between 27 nm and 113 nm. The experimental values were determined from the equivalent volume diameter as calculated by combining the measured effective density with the particle mobility diameter, Equation (Equation15[15] ). Then, the dynamic shape factor of the carbon nanoparticles was determined, without any assumption about particles morphology (experimental χ) via Equation (Equation14
[14] ). The same equation was also used to determine the theoretical predictions except that the equivalent volume diameter was obtained from the number of primary particles, Equation (Equation13
[13] ). The equivalent volume diameter was also calculated via the particle mobility diameter and the number of primary particles
that compose an aggregate. The average absolute difference between measured and predicted dynamic shape factors is approximately
for the ASM, Sorensen, and MSL predictions, whereas it is 12% for the L&F predictions.
4.4. Calculated mobility diameter: Aconsistency analysis
We emphasize that in this study morphological characteristics of carbon aggregates were determined by comparing CEPI measurements of polydisperse aerosols with the size distributions obtained from SMPS. The CEPI-measured Fuchs surface-area distributions were obtained without invoking any assumptions on the morphology of the nanoparticles. On the other hand, the inversion of SMPS raw data requires an assumption about particle morphology. The inversion software allows for two alternatives (extreme cases): spherical particles or idealised aggregates. The resulting user choice may add additional uncertainty on the estimate of the experimentally determined mobility diameter. As such, it is important to provide estimates of the associated uncertainties.
We investigated the effect of the inversion algorithm on the calculation of the Fuchs surface area and the effective density. In Section S5 of the SI, we summarize the calculations for SMPS-determined equivalent mobility diameters using the charge correction for spherical particles (we, also, show two characteristic number distributions). We found that the absolute difference between experimentally measured and calculated total Fuchs surface area is 33 ± 25%, a difference similar to that found when the ideal aggregates correction was used (26 ± 22%, Section 4.2). Predicted particle morphology, as specified by the mass mobility fractal dimension and prefactor, was also found to be slight different. The effective density dependence on the mobility diameter (spherical particle charge correction) is described by , values that have to be contrasted to
that were determined using the ideal chain aggregate correction. As mentioned earlier, Section 4.3, the ordered pair obtained with the multiple charge correction for ideal aggregate is in better agreement with theoretical calculations.
Lastly, we performed a consistency analysis of two models: the L&F model, which is used in the AIM inversion algorithm and the Mobility Scaling Law (MSL) whose parameters where determined from the fit to the experimental effective density, Equation (Equation12[12] ). The consistency test is based on a reference mobility diameter. For a given reference aggregate mobility diameter (and a monomer diameter) the expected number of primary particles in the aggregate is calculated either by the L&F model, Equation (Equation5
[5] ), or via the mass-mobility scaling law, Equation (Equation4
[4] ). If
is known, and since the aggregates are DLCA aggregates, the various characteristic scales
,
,
and
(and the corresponding Knudsen numbers and slip correction factors) may be calculated via Equations (Equation3
[3] ), (Equation13
[13] ), (Equation9
[9] ), and (Equation8
[8] ), respectively. Then, the calculated aggregate mobility diameter can be obtained by solving the nonlinear equation, Equation (Equation7
[7] ), according to the ASM, and described in Section 3.2.1. presents the comparison of reference and predicted mobility diameters for the two
calculations.
Figure 10. Comparison between reference mobility diameter (taken to be the GMD of the SMPS number distributions) and calculated mobility diameters.
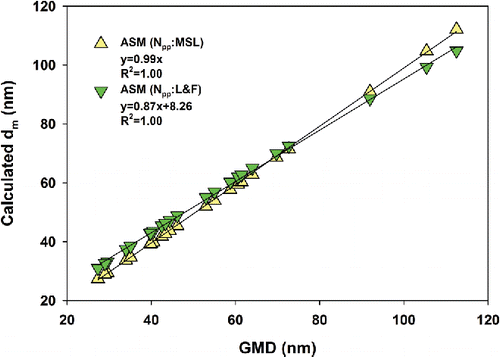
We note that when the number of primary particles is calculated using the mobility scaling law the resulting mobility diameters, obtained via the adjusted-sphere method for power-law aggregates, reproduce rather accurately the reference mobility diameters. Discrepancies are observed when is calculated using the L&F model. This is expected as differences between experimental data and L&F predictions were already noted in –. More importantly, the origin of these discrepancies is that the spark-discharge generated aggregates are not the idealized chain aggregates assumed in the L&F model, as was noted for flame synthesized aggregates/agglomerates (Thajudeen et al. Citation2015). shows the percentage difference between calculated and reference diameters as a function of the number of primary particles. When the number of primary particles is calculated under the assumption of idealized chain aggregates, the estimate of the mobility diameter may be in error between –10% and 7% for
, namely, in the size range between 27 nm and 113 nm. When the number of primary particles is calculated by the ASM, the error is lower than 2%. In the size range between 10 nm and 100 nm, an overestimation of particle mobility diameter by 10% may lead to an overestimation of the respective Fuchs surface area by
, as calculated by Equation (Equation1
[1] ).
5. Conclusions
In this study, the physical properties of carbon nanoparticles produced by a spark discharge ionization source were investigated by parallel measurements of their Fuchs surface-area distributions by a cascade epiphaniometer (CEPI) and their number distribution by a scanning mobility particle sizer (SMPS). The CEPI measures particle Fuchs surface area (concentration) distributions via the mass transfer of lead atoms onto the surface of aerosol particles, a process which is independent of material properties and electrostatic interactions. The CEPI offers an alternative technique to the physical characterization of aerosol particles since the most widely used techniques for online particle characterization of aerosol particles rely heavily on electrostatic properties and the charge distribution of aerosol particles.
The SMPS is a widely used instrument for number-distribution measurements, but an assumption is required about particle morphology that affects, inter alia, the charging efficiency, particle orientation, and electrostatic forces between particles and ions etc. The conversion of raw data into particle number distributions requires that the user choose between two extreme cases: spherical particles or idealised aggregates. This choice adds an uncertainty on the mobility diameter estimate. We found that both approaches give reasonable estimates of the total Fuchs surface area of carbon aggregates (calculated absolute differences of the order of 30%), and of their morphology. Specifically, structural characteristics of the aggregates as described by the mass mobility fractal dimension and prefactor, were determined to be under the assumption of spherical particles and
when the multiple charge correction for idealized aggregates was used. We note that the experimentally determined
with the charge inversion algorithm for aggregates agrees slightly better with the theoretical prediction
, at atmospheric pressure, for diffusion limited cluster aggregates (Melas et al. Citation2014b).
Calculated, via the SMPS number distributions, and measured, via the CEPI, Fuchs surface areas were in good agreement (within 28%, average absolute difference) for aggregates in the size range between 27 nm and 113 nm. This is in agreement with previous measurements (Rogak et al. Citation1991) that the mass transfer rate is approximately independent of particle morphology for particles with the same mobility, at least under the experimental conditions studied.
Through parallel CEPI-SMPS measurements the effective density of the generated carbon particles was determined to be between 1.31 and 0.35 g/cm3. The measured effective density was compared to predictions of various theoretical models. The agreement was very good with the predictions of the Adjusted-Sphere-Method and the empirical correlation of Sorensen Citation(2011), being worse for the L&F model that assumes idealized chain aggregates.
The morphological and physical properties of the spark-discharge generated carbon aggregates were determined using polydisperse particle distributions, instead of mononodisperse distributions of specific mobility diameter aerosols. The results revealed that some characteristics of a unimodal polydisperse aerosol (total Fuchs surface area) may be adequately approximated (average absolute difference 26%) by the properties of an equivalent monodisperse aerosol, with particle size equal to the GMD of the original distribution.
Funding
This work has been co-funded by two sources: (1) EnTeC FP7 Capacities program (REGPOT-2012-2013-1, FP7, ID: 316173) and (2) 11SYN_5_1861/DE_SPARK_NANO_GEN, implemented in the framework of the Action “Cooperation 2011 - Partnerships of Production and Research. Institutions in Focused Research and Technology” of the Operational Programme “Competitiveness and Entrepreneurship” (OPCE II, Action's Beneficiary: General Secretariat for Research and Technology - MIA-RTDI), and is co-financed by the European Regional Development Fund (ERDF) and the Greek State.
UAST_1134763_Supplemental_File.zip
Download Zip (185 KB)References
- Bau, S., Witschger, O., Gensdarmes, F., and Thomas, D. (2012). Evaluating Three Direct-reading Instruments Based on Diffusion Charging to Measure Surface Area Concentrations in Polydisperse Nanoaerosols in Molecular and Transition Regimes. J. Nanopart. Res., 14:1217.
- Biskos, G., and Schmidt-Ott, A. (2012). Airborne Engineered Nanoparticles: Potential Risks and Monitoring Challenges for Assessing Their Impacts on Children. Paediatr. Respir. Rev., 13:79–83.
- Biskos, G., Vons, V., Yurteri, C. U., and Schmidt-Ott, A. (2008). Generation and Sizing of Particles for Aerosol-Based Nanotechnology. KONA Powder Part J., 26:13–35.
- Bhushan, B. (2004). Springer Handbook of Nanotechnology. Springer, NY.
- Brown, D, Wilson, M, MacNee, W, Stone, V, and Donaldson, K. (2001). Size-Dependent Pro Inflammatory Effects of Ultrafine Polystyrene Particles: A Role for Surface Area and Oxidative Stress in the Enhanced Activity of Ultrafines. Toxicol. Appl. Pharm., 175:191–199.
- Colbeck, I., Eleftheriadis, K., and Simons, S. (1989). The Dynamics and Structure of Smoke Aerosols. J. Aerosol. Sci., 20:875–878.
- Dahneke, B. E. (1973). Slip Correction Factors for Non-Spherical Objects – III The Form of the General Law. J. Aerosol. Sci., 4:163–170.
- DeCarlo, P., Slowik, J. G., Worsnop, D. R., Davidovits, P., and Jimenez, J. L. (2004). Particle Morphology and Density Characterization by Combined Mobility and Aerodynamic Diameter Measurements. Part1: Theory. Aerosol Sci. Technol., 38:1185–1205.
- Gaggeler, H. W., Baltensperger, U., Emmenegger, N., Jost, D. T., Schmidt, O. H., Haller, P., and Hofmann, M. (1989). The Epiphaniometer, a New Device for Continuous Aerosol Monitoring. J. Aerosol. Sci., 20:557–564.
- Gini, M. I., Helmis, C., and Eleftheriadis, K. (2013). “Cascade Epiphaniometer: An Instrument for Aerosol “Fuchs” Surface Area Size Distribution Measurements”. J. Aerosol. Sci., 63:87–102.
- Heitbrink, W. A., Evans, D. E., Ku, B. K., Maynard, A. D., Slavin, T. J., and Peters, T. M. (2009). Relationship Among Particle Number, Surface Area, and Respirable Mass Concentrations in Automotive Engine Manufacturing. J. Occup. Environ. Hyg., 6:19–31.
- Hinds, W. C. (1999). Aerosol Technology. Properties, Behavior, and Measurement of Airborne Particles. Second ed., John Wiley & Sons, Inc.
- Johnson, T. J., Olfert, J. S., Cabot, R., Treacy, C., Yurteri, C. U., Dickens, C., McAughey, J., and Symonds, J. P. R. (2014). Steady-State Measurement of the Effective Particle Density of Cigarette Smoke. J. Aerosol. Sci., 75:9–16.
- Johnson, T. J., Symonds, J. P. R., and Olfert, J. S. (2013). Mass Mobility Measurements Using a Centrifugal Particle Mass Analyzer and Differential Mobility Spectrometer. Aerosol Sci. Technol., 47(11):1215–1225.
- Jung, H., and Kittelson, D. B. (2005). Characterization of Aerosol Surface Instruments in Transition Regime. Aerosol Sci. Technol., 39:902–911.
- Kasper, M., Matter, U., and Burtscher, H. (2000). NanoMet: On-Line Characterization of Nanoparticle Size and Composition. Society of Automotive Engineers Technical Paper: 2000-01-1998.
- Kelly, W. P., and McMurry, P. H. (1992). Measurement of Particle Density by Inertial Classification of Differential Mobility Analyser-Generated Monodisperse Aerosol. Aerosol Sci. Technol., 17:199–212.
- Ku, B. K., and Maynard, A. D. (2005). Comparing Aerosol Surface-area Measurements of Monodisperse Ultrafine Silver Agglomerates by Mobility Analysis, Transmission Electron Microscopy and Diffusion Charging. J. Aerosol. Sci., 36:1108–1124.
- Lall, A. A., and Friedlander, S. K. (2006). On-Line Measurement of Ultrafine Aggregate Surface Area and Volume Distributions by Electrical Mobility Analysis: 1. Theoretical Analysis. J. Aerosol. Sci., 37(3):260–271.
- Mamakos, A., Khalek, I., Giannelli, R., and Spears, M. (2013). Characterization of Combustion Aerosol Produced by a Mini-CAST and Treated in a Catalytic Stripper. Aerosol Sci. Technol., 47:927–936.
- Maricq, M. M., and Xu, N. (2004). The Effective Density and Fractal Dimension of Soot Particles from Premixed Flames and Motor Vehicle Exhaust. J. Aerosol. Sci., 35:1251–1274.
- Marjamaki, M., Keskinen, J., Chen, D.-R., and Pui, D. Y. H. (2000). Performance and Evaluation of the Electrical Low-Pressure Impactor (ELPI). J. Aerosol. Sci., 31(2):249–261.
- McMurry, P. H., Wang, X., Park, K., and Ehara, K. (2002). The Relationship between Mass and Mobility for Atmospheric Particles: A New Technique for Measuring Particle Density. Aerosol Sci. Technol., 36(2):227–238.
- Melas, A. D., Isella, L., Konstandopoulos, A. G., and Drossinos, Y. (2014a). Morphology and Mobility of Synthesized Colloidal Aggregates. J. Colloid Interface Sci., 417:27–36.
- Melas, A. D., Isella, L., Konstandopoulos, A. G., and Drossinos, Y. (2014b). Friction Coefficient and Mobility Radius of Fractal-like Aggregates in the Transition Regime. Aerosol Sci. Technol., 48(12):1320–1331.
- Melas, A. D., Isella, L., Konstandopoulos, A. G., and Drossinos, Y. (2015). A Methodology to Calculate the Friction Coefficient in the Transition Regime: Application to straight chains. J. Aerosol. Sci., 82:40–50.
- Milanović, M., Moshopoulou, E. G., Stamopoulos, D., Devlin, E., Giannakopoulos, K. P., Kontos, A. G., Eleftheriadis, K., Gini, M. I., and Nikolić, L. M. (2013). Structure and Magnetic Properties of Zn1-xInxFe2O4 and ZnYxFe2-xO4 Nanoparticles Prepared by Coprecipitation. Ceram. Int., 39:3235–3242.
- Meuller, B. O., Messing, M. E., Engberg, D. L. J., Jansson, A. M., Johansson, L. I. M., Norlén, S. M., Tureson, N., and Deppert, K (2012). Review of Spark Discharge Generators for Production of Nanoparticle Aerosols. Aerosol Sci. Technol., 46(11):1256–1270.
- Oberdorster, G, Stone, V, and Donaldson, K. (2007). Toxicology of Nanoparticles: A Historical Perspective. Nanotoxicology, 1:2–25.
- Pandis, S. N., Baltensperger, U., Wolfenbarger, K. J., and Seinfeld, J. H. (1991). Inversion of Aerosol Data from the Epiphaniometer. J. Aerosol. Sci., 22(4):417–428.
- Pilou, M, Mavrofrydi, O, Housiadas, C, Eleftheriadis, K, and Papazafiri, P. (2015). Computational Modeling as Part of Alternative Testing Strategies in the Respiratory and Cardiovascular Systems: Inhaled Nanoparticle Dose Modeling Based on Representative Aerosol Measurements and Corresponding Toxicological Analysis. Nanotoxicology, 9(S1):106–115.
- Rissler, J., Messing, M. E., Malik, A. I., Nilsson, P. T., Nordin, E. Z., Bohgard, M., Sanati, M., and Pagels, J. H. (2013). Effective Density Characterization of Soot Agglomerates from Various Sources and Comparison to Aggregation Theory. Aerosol Sci. Technol., 47:792–805.
- Ristimaki, J., Virtanen, A., Marjamaki, M., Rostedt, A., and Keskinen, J. (2002). On-line Measurement of Size Distribution and Effective Density of Submicron Aerosol Particles. J. Aerosol. Sci., 33:1541–1557.
- Rogak, S. N., Baltensperger, U., and Flagan, R. C. (1991). Measurement of Mass-Transfer to Agglomerate Aerosols. Aerosol Sci. Technol., 14(4):447–458.
- Rogak, S. N., and Flagan, R. C. (1992). Bipolar Diffusion Charging of Spheres and Agglomerates Aerosol Particles. J. Aerosol. Sci., 23:693.
- Rogak, S. N., Flagan, R. C., and Nguyen, H. V. (1993). The Mobility and Structure of Aerosol Agglomerates. Aerosol Sci. Technol., 18(1):25–47.
- Schmidt-Ott, A. (1988). New Approaches to in Situ Characterization of Ultrafine Agglomerates. Aerosol Sci. Technol., 19(5):553–563.
- Schmid, O., Karg, E., Hagen, D. E., Whitefield, P. D., and Ferron, G. A. (2007). On the Effective Density of Non-Spherical Particles Derived from Combined Measurements of Aerodynamic and Mobility Equivalent Size. J. Aerosol. Sci., 38(4):431–443.
- Shang, L., Nienhaus, K., and Nienhaus, G. U. (2014). Engineered Nanoparticles Interacting with Cells: Size Matters. J. Nanobiotechnology, 12:5.
- Shapiro, M., Vainshtein, P., Dutcher, D., Emery, M., Stolzenburg, M., Kittelson, D. B., and McMurry, P. H. (2012). Characterization of Agglomerates by Simultaneous Measurement of Mobility, Vacuum Aerodynamic Diameter and Mass. J. Aerosol. Sci., 44:24–45.
- Shi, J. P., Harrison, R. M., and Evans, D. (2001). Comparison of Ambient Particle Surface Area Measurement by Epiphaniometer and SMPS/APS. Atmos, Environ., 35(35):6193–6200.
- Shin, W. G., Pui, D. Y. H., Fissan, H., Neumann, S., and Trampe, A. (2007). Calibration and Numerical Simulation of Nanoparticle Surface Area Monitor (TSI Model 3550 NSAM). J. Nanopart. Res., 9:61–69.
- Siegmann, K., and Siegmann, H. (2000). Fast and Reliable “in situ” Evaluation of Particles and their Surfaces with Special Reference to Diesel Exhaust. Society of Automotive Engineers, Technical Paper 2000-01-1995.
- Sorensen, C. M. (2011). The Mobility of Fractal Aggregates: A Review. Aerosol Sci. Technol., 45(7):765–779.
- Ströbel, R., Garche, J., Moseley, P. T., Jörissen, L., and Wolf, G. (2006). Hydrogen Storage by Carbon Materials. J. Power Sources, 159(2):781--801.
- Svensson, C. R., Ludvigsson, L., Meuller, B. O., Eggersdorfer, M. L., Deppert, K., Bohgard, M., Pagels, J. H., Messing, M. E., and Rissler, J. (2015). Characteristics of Airborne Gold Aggregates Generated by Spark Discharge and High Temperature Evaporation Furnace: Mass–Mobility Relationship and Surface Area. J. Aerosol. Sci., 87:38–52.
- Tabrizi, N. S., Ullmann, M., Vons, V. A., Lafont, U., and Schmidt-Ott, A. (2009). Generation of Nanoparticles by Spark Discharge. J. Nanopart. Res., 11:315–332.
- Thajudeen, T., Gopalakrishnan, R., and Hogan, C. J., Jr. (2012). The Collision Rate of Nonspherical Particles and Aggregates for All Diffusive Knudsen Numbers. Aerosol Sci. Technol., 46 (11):1174--1186.
- Thajudeen, T., Jeo, S., and Hogan, C. J., Jr. (2015). The Mobilities of Flame Synthesized Aggregates/Agglomerates in the Transition Regime. J. Aerosol. Sci., 80:45–57.
- Trujillo-Reyes, J., Peralta-Videa, J. R., and Gardea-Torresdey, J. L. (2014). Supported and Unsupported Nanomaterials for Water and Soil Remediation: Are They a Useful Solution for Worldwide Pollution?. J. Hazard Mater., 280:487–503.
- Virtanen, A. K. K., Ristimaki, J. M., Vaaraslahti, K. M., and Keskinen, J. (2004). Effect of Engine Load on Diesel Soot Particles. Environ. Sci. Technology, 38(9):2551–2556.
- Webster, T. (2009). Safety of Nanoparticles. From Manufacturing to Medical Applications. Springer, NY.
- Wen, H. Y., Reischl, G. P., and Kasper, G. (1984). Bipolar Diffusion Charging of Fibrous Aerosol Particles- I. Charging Theory. J. Aerosol. Sci., 15:89–101.
- Zhang, C., Thajudeen, T., Larriba, C., Schwartzentruber, T. E., and Hogan, C. J., Jr. (2012). Determination of the Scalar Friction Factor for Nonspherical Particles and Aggregates Across the Entire Knudsen Number Range by Direct Simulation Monte Carlo (DSMC). Aerosol Sci. Technol., 46:1065–1078.