ABSTRACT
We introduce a new method to estimate the mass concentration of particulate organic carbon (POC) collected on quartz filters, demonstrating it using quartz-filter samples collected in greater Pittsburgh. This method combines thermal-optical organic carbon and elemental carbon (OC/EC) analysis and the volatility basis set (VBS) to quantify the mass concentration of semi-volatile POC on the filters. The dataset includes ambient samples collected at a number of sites in both summer and winter as well as samples from a highway tunnel. As a reference we use the two-filter bare-Quartz minus Quartz-Behind-Teflon (Q-QBT) approach to estimate the adsorbed gaseous fraction of organic carbon (OC), finding a substantial fraction in both the gas and particle phases under all conditions. In the new method we use OC fractions measured during different temperature stages of the OC/EC analysis for the single bare-quartz (BQ) filter in combination with partitioning theory to predict the volatility distributions of the measured OC, which we describe with the VBS. The effective volatility bins are consistent for data from both ambient samples and primary organic aerosol (POA)-enriched tunnel samples. Consequently, with the VBS model and total OC fractions measured over different heating stages, particulate OC can be determined by using the BQ filter alone. This method can thus be applied to all quartz filter-based OC/EC analyses to estimate the POC concentration without using backup filters.
© 2016 American Association for Aerosol Research
EDITOR:
Introduction
Aerosols cause serious negative effects on human health and also affect climate on regional and global scales through their ability to absorb and scatter solar radiation and to influence the properties of clouds (Forster et al. Citation2007). It is known that aerosols are comprised of a wide variety of materials, with organic species typically comprising nearly half of the particle aerosol mass (Zhang et al. Citation2007). Organic aerosols (OAs) are part of a complex system linking emissions, chemical transformations, and phase partitioning. The atmospheric lifetimes and characteristics of different organic species are strongly affected by their volatilities because their oxidation rates depend largely on the phase in which they reside.
Numerous techniques exist to measure the carbonaceous fraction of fine particle matter (PM2.5). One approach is to split total carbon (TC) between organic carbon (OC) and elemental carbon (EC), as their chemical, physical, and optical characteristics are distinct. Since (non-refractory) OC is much more volatile but less light absorbing than (refractory) EC, OC and EC can be separated by thermal-optical methods. For several decades, thermally derived carbon fractions including OC and EC have been reported for the Interagency Monitoring of Protected Visual Environments (IMPROVE) network, which was established by the National Park Service. Both archived and future network samples represent a rich reservoir of information regarding the carbonaceous fraction of PM2.5.
It has long been recognized that OC measurements employing quartz fiber filters are subject to artifacts related to desorption of semi-volatile compounds from collected particles and adsorption of organic vapors to the quartz fibers. Because of the importance of network data for assessing regulatory compliance with the PM2.5, extensive effort has been dedicated to constraining and correcting for sampling artifacts in order to determine the correct particulate-matter OC level (Turpin et al. Citation2000). These sampling artifacts arise from adsorption of OC vapors on quartz filters (positive artifact) and evaporation of semi-volatile OC constituents from particles on filters (negative artifact). Various methods have been applied to account for these artifacts, including multiple front and backup filters with different composition (quartz and Teflon) and denuders (Subramanian et al. Citation2004).
The temperature protocols used in OC/EC analysis include several stages of heating, and typically a portion of OC known as an “OC fraction” evolves from the filters during each stage. Also, during the heating process, some OC is known to pyrolyze, forming refractory light-absorbing carbon that can be confused with EC. Previous studies of OC/EC analysis have been mostly focused on determining the effect of sampling artifacts for particles of OC and EC, the pyrolyzed carbon (PC) split, and measurement differences between various temperature protocols. The OC fractions have received less attention. Some studies have been conducted with the OC fractions, for example, OC fractions were used in a source identification study in St. Louis supersite to analyze the emission factors, finding that the third fraction (OC3) accounts for the largest portion of total OC in the St. Louis area (Lee et al. Citation2006); however, there has been little effort focused on analyzing the gas-particle partitioning of OC fractions obtained from OC/EC measurements.
The vapor fraction associated with OC samples has long been regarded as a positive artifact obscuring the particulate organic carbon (POC) signal but it has generally not been considered as a useful measurement of semi-volatile OC vapors. We find that we can interpret particle-phase and gas-phase OC constrained by a standard analysis of OC collected on bare-Quartz (BQ) and Quartz Behind Teflon (QBT) filter pairs with an empirical volatility basis set (VBS) (Donahue et al. Citation2006). The OC/EC analysis does not provide us with molecular information regarding the composition of the OC fractions; however, the VBS model does not require that information. Consequently, the VBS model can provide an estimate of both the POC concentrations and volatility distribution as well as an alternative way to correct the artifact. The VBS model we use here does not involve any molecular specificity, and the effective saturation concentrations we assign are purely empirical. Though several previous studies have shown that gas-particle partitioning data can be derived from aggregate OC/EC measurements using quartz filters (Presto et al. Citation2012; May et al. Citation2013), we are not aware of previous work using the partitioning of individual OC fractions from the OC/EC analysis to correct or interpret filter-sampling artifacts. Here we present a new method to estimate the POC collected on quartz filters using partitioning theory.
In this study, we analyze the gas-particle partitioning of OC fractions by employing the IMPROVE_A temperature protocol and determine effective saturation concentrations appropriate for the protocol using the VBS model. Here we focus on an analysis of vapor and particle OC concentrations and the effective volatility of individual OC fractions for an ensemble of filters collected in Pittsburgh. Our major goal is to demonstrate that POC concentrations can be derived using BQ filters alone. We investigate the partitioning of these OC fractions by casting both the ambient and tunnel quartz-filter samples onto a VBS model. We then predict partitioning using the empirical VBS volatility distribution and compare those predictions to the observed partitioning, both for seasonal averages and for individual filters. The main implication of this work is that we can accomplish the artifact correction typically requiring multiple filter configurations with a single BQ filter sample. The second implication is that we gain information about semi-volatile partitioning from that analysis that converts the “artifact” into a signal related to the ambient OC volatility distribution.
Methods
Sample collection and analysis
We collected 213 ambient samples at a variety of sites in the greater Pittsburgh area in the summer and winter of 2013, using the CAPS Mobile Air Quality Lab. We classified the sampling sites based on three properties (Upland or Valley, near or far from traffic, and near or far from point sources). Also, in the winter of 2013 we collected 18 samples at the Fort Pitt tunnel (a tunnel containing a major highway connecting downtown Pittsburgh to southern suburbs and the airport).
We drew ambient air through a stainless-steel probe with an inner diameter of 5 mm and then through a cyclone to select fine particles. A sharp-cut PM2.5 cyclone was upstream of two lines: one with a filter pack holding a single quartz filter, and one with a filter pack holding a Teflon filter followed by a quartz filter. The upstream quartz filter is called Bare Quartz, and the downstream quartz filter is called Quartz Behind Teflon. This setup is similar to protocols used in previous studies (McDow and Huntzicker Citation1990; Turpin et al. Citation1994; Subramanian et al. Citation2004). Analysis of these filter pairs often involves assuming that the Teflon filter traps PM but the OC gas adsorption on it is negligible. Further assumptions are that the upstream Teflon filter loses minimal POC via evaporation (the negative artifact) and that it adsorbs minimal gas-phase OC. If those assumptions hold, the QBT filter is exposed to the same organic vapor as the BQ filter. Thus, any OC measured on the QBT filter constrains the gaseous organics adsorbed by any front quartz filter, including the BQ filter. Hence, POC can be estimated as BQ OC minus QBT OC (Q-QBT). This procedure can be applied to the individual OC fractions as well as the aggregate total OC signal.
The sampling duration in our study was 1 h for each ambient sample and 45 min for each tunnel sample. We pre-baked all quartz-fiber filters at 550°C for at least 4 h to remove organic impurities and then stored them in aluminum foil before sampling. We used the Teflon filters as provided by the manufacturer without any pretreatment. We loaded or unloaded all filters in a laminar flow hood. We used stainless-steel filter packs and a constant sample flow rate, controlled by a mass flow controller (MFC) at 46 slpm. Samples covered an area with a 38 mm diameter. We collected handling blanks before sampling every day. All of the handling blank values for quartz filters were lower than the EPA speciation-network specification of 1 μg cm−2 and also below the instrument detection limit for OC (0.2 μg-C cm−2). Following sampling we stored the filters in a refrigerator to prevent the evaporation of volatile components.
We analyzed a punch from each filter for OC and EC using a Sunset Laboratories OC/EC analyzer and the IMPROVE_A Thermal Optical Reflectance (TOR) protocol (Chow et al. Citation2007). The temperature profile of IMPROVE_A protocol is given in . We manually calibrated the instrument prior to use with sucrose standards and known quantities of CH4. We provide full details of the sampling and analysis protocol in the online supplementary information (SI).
Table 1. The IMPROVE_A protocol used for analyzing the quartz filters.
Ideally, OC evolves by thermal desorption in an inert He atmosphere over four temperature stages (fractions OC1–OC4), and EC evolves following oxidation in the oxidizing He–O2 atmosphere over three temperature stages (fractions EC1–EC3). However, during heating in an O2-free environment, some of OC chars to become light-absorbing, less-volatile refractory carbon, and when O2 is added, this char combusts along with the original EC collected on the filter's surface. This amount of char is often called pyrolyzed organic carbon, or PC. PC can absorb light and requires an oxidizing atmosphere to evolve off of the filter. A common assumption is that the absorptivity and combustion characteristics of PC are similar to those of EC. The quantity of PC can then be determined from the transmission of laser light through the filter. During pyrolysis, the laser transmittance will drop. During EC combustion, when transmittance returns to the pre-analysis value, one can assume that the detected carbon signal is equivalent to the PC mass and the remaining signal from light-absorbing carbon can be attributed to EC. This generally occurs during EC1. It is not necessary that all PC is less refractory than EC but rather that the characteristics of PC and EC are similar. The PC is thus functionally a portion of EC1. This produces OC concentrations for four desorption temperatures (OC fractions OC1, OC2, OC3, and OC4) and in addition a concentration for light-absorbing PC, which we assign as a fifth OC fraction. This PC fraction is empirically the least volatile, but our conclusions are not sensitive to any assumptions we make about PC volatility because it is not a major component of the OC.
Previous studies have shown that analysis of the QBT signal can provide a credible estimate of any sampling artifacts for short-duration sampling (Turpin et al. Citation2000; Subramanian et al. Citation2004). Consequently, we used the QBT OC signal to estimate the vapor OC collected on our quartz filters. In our study, any negative artifacts were probably small because the evaporation of collected POC was minimized by the short sampling interval. Volatilization from the Teflon filters was also probably small. Both the front and the backup quartz-fiber filters will absorb some organic vapors throughout their thickness during sampling; these organic vapors organics constitute the positive artifact and we treat them as gas-phase OC in our VBS model. We conclude that the Q-QBT approach can provide a reasonable estimate of both the particle-phase OC and the positive “artifact” for measurements such as ours with short sampling periods.
There are two other major methods to account for positive sampling artifacts. One popular approach is to place an organic denuder upstream of the quartz filter to remove organic gases from the incoming air stream by diffusion to a sorbent (Eatough et al. Citation2003). Another approach is to use a backup quartz filter behind the BQ filter in a single filter pack (the Q-QBQ approach). The POC concentration can be estimated as the BQ OC signal minus the QBQ OC signal (Chow et al. Citation2010). Similar to the Q-QBT approach, the Q-QBQ approach relies on an assumption that the QBQ filter is exposed to the same organic vapor concentration as the front quartz filter. However, the backup QBQ filter may adsorb less gaseous organic matter than the front quartz filter, especially at low exposure, if the front quartz filter is not in equilibrium with the sample air stream. The front filter would thus deplete the air stream of organic vapors (Kirchstetter et al. Citation2001; Mader and Pankow Citation2001; Chen et al. Citation2010). As a result, the QBQ OC level is usually lower than a comparable QBT filter for low exposure. The Q-QBQ approach is thus not ideal for short-duration studies such as ours. However, at high exposure the Q-QBQ approach provides robust estimates of the POC concentration (Hart and Pankow Citation1994) while the Q-QBT approach may overestimate the positive artifact (Turpin et al. Citation1994; Kim et al. Citation2001; Subramanian et al. Citation2004) because of volatilization of semi-volatile organics from the front Teflon filter (part of the negative artifact). Consequently, for long sampling periods (>3 h), the Q-QBQ approach provides a more reasonable estimate of the positive artifacts on the front quartz filter while the Q-QBT approach only provides an upper limit of positive artifacts.
Volatility analysis
The effective saturation concentration (Ci*) of an organic compound is expressed by converting its saturation vapor pressure into mass concentration units and multiplying by the appropriate activity coefficient for the organic mixture; this is also the inverse of the partitioning coefficient () (Pankow Citation1994). A simplifying assumption is that the activity coefficient will be constant and thus Ci* for a given compound will also be constant. If we project concentrations of OAs onto a basis set of saturation concentrations, we obtain the volatility distribution of the organic material. The condensed-phase organic-aerosol concentration, COA, and the equilibrium partitioning fractions ξi are described by (Donahue et al. Citation2006, Citation2011):
[1]
These two equations show how the fraction of compound i found in the condensed phase at equilibrium, or the partitioning fraction ξi and the total OA concentration COA can be calculated in a given circumstance. For instance, a constituent with will have half of its mass in the condensed phase while a more volatile constituent with
will only have 10% mass in the condensed phase.
Since the mass concentration of OAs (including H, O, N, etc.) cannot be obtained directly from OC/EC analysis, it is conventional to use the OM/OC ratio to convert the concentration of OC into the concentration of OAs. We can include this directly into the partitioning equation. Here we assume that OM/OC is constant; as a result, Equation (Equation1[1] ) becomes
[2] where XPi is particle fraction in OC fraction i, which can be measured by OC/EC analysis. In this way, we can use the VBS approach to estimate the Ci* value for each OC fraction.
Volatility classes of organics
Here we shall refer to some volatility ranges defined previously (Donahue et al. Citation2009). Organic compounds, in order of increasing volatility, can be defined as:
Extremely low volatility organic compounds (ELVOC, C* < 3 × 10−4 μg m−3): These compounds are almost completely in the particle phase under any ambient conditions.
Low volatility organic compounds (LVOC, 3 × 10−4 < C* < 0.3 μg m−3): These compounds are mostly in the particle phase in the atmosphere.
Semi-volatile organic compounds (SVOC, 0.3 < C* < 300 μg m−3): These compounds can be in either condensed phase or gas phase but often have mass fractions in both depending a lot on ambient conditions.
Intermediate volatility organic compounds (IVOC, 300 < C* < 3 × 106 μg m−3): These compounds reside almost exclusively in the gas phase under atmospheric conditions, but their numbers are untold and they are hard to measure. However, they have a very high potential to generate lower volatility products after reaction.
Volatile organic compounds (VOC, C* > 3 × 106 μg m−3): These compounds are always represented in models either explicitly or as lumped species.
Data processing
Semi-volatile organics have historically been regarded as a source of bias in OC/EC filter samples, and the BQ, QBT sampling strategy was developed to help correct for this bias, under the assumption that, for the most part, OC collected on QBT filter is related to semi-volatile vapor adsorption to the quartz filter (Hart and Pankow Citation1994). We are interested in treating the partitioning information as a signal rather than a source of bias. Shrivastava et al. (Citation2006) proposed that BQ and QBT filter pairs can provide an estimate of gas-particle partitioning for semi-volatile primary organic aerosol (POA) emissions. The premise is that the BQ filter captures the semi-volatile OC (particle and vapor) while the QBT filter captures only semi-volatile vapors that adsorb to the quartz. The key assumption is that vapor adsorption to the two quartz filters is the same and that evaporation from particles on the front filters is either minimal or identical. If we assume the BQ filter is saturated, then we can define the particle fraction as:[3]
Accordingly, the vapor fraction is:[4]
These two equations can only be used to provide the partitioning data for OC that is collected on quartz fibers since it is known that quartz is not highly efficient at capturing organic vapors, especially IVOCs and other organics with higher volatility.
The particle fraction, Xp, can also be calculated from partitioning theory as it corresponds to the summation term. In this study, we apply this method to all of our ambient and tunnel samples in order to estimate gas-particle partitioning for SVOCs. We subsequently interpret these data in the context of the VBS, starting with ensemble average partitioning based on the OC fractions and ending with sample-by-sample variation in partitioning driven by dilution.
It is known that the QBT filter, which we interpret as vapor, overcorrects for the positive artifact. The QBT may overestimate the OC vapor concentration, possibly because of volatilization from organic particulate matter collected on the Teflon filter; however, this negative bias is relatively small and even negligible in our short-time samples, as discussed above.
Results and discussion
Seasonal summary of OC statistics
In , we show the concentration distribution of vapor (QBT) and particulate (BQ-QBT) OC concentrations for three subsets: summer 2013, winter 2013, and the tunnel samples. In all cases, there is a substantial fraction of OC in both phases. The seasonal difference of vapor OC and particulate OC is small, but both vapor and particulate OC concentrations were higher in the summer. The overall POC concentrations in summer 2013 and winter 2013 vary from −0.99 to 19.19 µg-C m−3 with an average of 2.13 µg-C m−3 and the QBT OC in the same sampling group range from −0.05 to 8.78 µg-C m−3 with an average of 2.87 µg-C m−3. This seasonal pattern agrees with results from the Pittsburgh Air Quality Study (PAQS). For all three sampling groups, the QBT OC is about 55% of the BQ OC. The magnitude of the artifacts from this study is consistent with many previous studies as well. For instance, McDow and Huntzicker (Citation1990) found QBT OC to be 50% of the BQ OC for low OC values in Portland, Oregon. Kim et al. (Citation2001) also reported the QBT OC, varying from 0.53 to 4.97 µg-C m−3, at about 30% of the BQ OC during sampling in Rubidoux, California. Eatough et al. (Citation2003) found an average QBT OC of 15.16 µg-C m−3 in Southern Africa, which is 47% of BQ OC. Olson and Norris also showed that QBT OC, with an average concentration of 3.5 µg-C m−3, averaged 66% of BQ OC in Research Triangle Park, North Carolina (Olson and Norris Citation2005). Finally, the negative bias caused by evaporation of particle-phase organics on the Teflon filter causes occasional negative particle phase OC shown in . This result is similar to early findings (Chow et al. Citation1996).
Patterns of carbonaceous fractions
In , we show averages for BQ OC and QBT OC that evolved at each Helium temperature step for all ambient samples. The vapor fraction is large for OC1, OC2, and OC3 because those OC fractions are comparatively more volatile. However, the vapor fraction is relatively small in OC4 because it is less volatile. In general, the particle fraction of OC increases progressively from OC1 to OC4, consistent with the temperature profile; there is thus a clear qualitative relationship between the temperature step and OC volatility, consistent with the temperature profile. Finally, the vapor fraction in PC is the lowest of all; thus, we conclude that PC broadly represents the least volatile fraction of the OC (very little of the organic material that passes through the Teflon filter pyrolyzes during the OC/EC analysis for any of the conditions covered by this study). Similar to the PAQS study (Subramanian et al. Citation2004), the mass concentration of QBT OC mainly occurs in the first three temperature steps (OC1, OC2, and OC3) while the OC4 and PC signals on the QBT filter are very low.
Group-based variation and seasonal variation of OC fractions
Because there is a clear volatility signature on the filters, it is reasonable to expect differences in volatility for samples obtained in different locations with different loadings and different volatility distributions. Based on earlier work (Donahue et al. Citation2011) as well as other ambient samples (Huffman et al. Citation2009), we hypothesize that filters collected close to sources should have larger total OC and thus a larger particle fraction in OC for any given OC stage, while filters in urban background locations should show a smaller particle fraction in each OC stage.
In we show the average OC concentrations for four sampling-site groups for summer (a,b) and winter (c,d), arranged inversely from their proximity to sources (upland, near traffic, near both traffic and point sources, and in tunnel). The panels show the total OC (a,c) and the vapor-phase OC (b,d) in each OC fraction for each location group. By far the highest OC concentrations are from tunnel samples, and, consistent with our hypothesis, they have the smallest fraction of vapor-phase OC on each stage because the tunnel samples contain a higher fraction of fresh semi-volatile primary OC. The other samples also conform broadly to our hypothesis, with a slight but positive relationship between OC loading and proximity to sources. The summertime increase in OC is most evident in the total OC levels in fractions OC2 and OC3, suggesting that production or emission of semi-volatile OC is higher in summertime. also clearly show that with increasing distance from emission sources, the dilution effect tends to drive POC repartitioning to the gas phase as the tunnel classification has the smallest vapor OC fractions for all four classifications during the winter.
Figure 3. Comparison of OC concentrations in ambient and tunnel samples for different sampling locations. (a) Total OC concentrations of different fractions in winter. (b) Vapor OC concentration of different fractions in winter. (c) Total OC concentrations of different fractions in summer. (d) Vapor OC concentration of different fractions in summer.
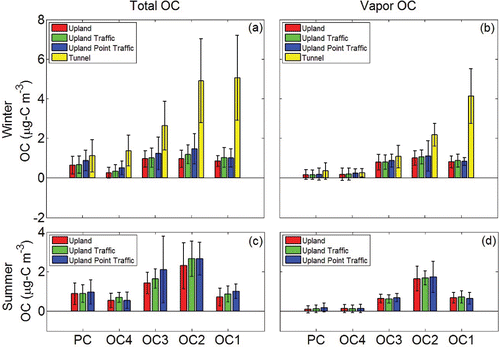
Derived volatility distributions
It is clear that the OC fractions display semi-volatile behavior qualitatively, with the OC evolving at the lowest temperature (OC1) being mostly in the vapor phase and the OC evolving at the highest temperature (OC4) being mostly in the condensed phase. In addition, to simplify our method, we treat PC as a low-volatility carbon fraction with specific volatility rather than consider it as a mixture that contains OC associated with all the temperature stages; this is supported by the very low QBT (vapor) signal in the PC fraction. To explore the partitioning behavior of OC collected on quartz filters, we use the VBS approach. It is fair to assume that the organics collected on the BQ and QBT filters have the same composition and volatility because both BQ and QBT filters are exposed to the same organic vapors. The OC/EC analysis cannot give us information about specific species collected on quartz filters. However, we can fit the partitioning with a VBS, which can be used without knowing the specific composition of organics, to derive a predicted volatility distribution and predict POC concentration on quartz filters. It is not even necessary that a given molecule evolves with only one OC fraction, but only that in general lower volatility organics evolve more at higher temperature stages. So by combining VBS and BQ-QBT approach, we can derive empirical volatility bins for each OC fraction.
In , we show the average partitioning behavior for all ambient filters as a histogram arranged from the least volatile (PC) to the most volatile (OC1) fraction. The bars indicate OC loading (total OC in µg-C m−3 is the total bar height for each fraction, while particle-phase OC is the filled in (green) bar height). The various fractions clearly obey the general partitioning behavior predicted by Equation (Equation2[2] ), with very little vapor for PC and OC4 and very little POC for OC2 and OC1.
Figure 4. (a) Gas-particle partitioning of OC fractions from all ambient samples, with total loadings shown with full bars and the particle phase portion with filled bars. The total loading for each OC fraction is given by the average mass concentration of that OC fraction on quartz filters. Error bars indicate the standard deviation of particle fraction for each volatility bin. (b) Volatility distribution of a predicted loading of the average organic aerosol concentration in all ambient samples with COA = 3.41 μg m−3 calculated from Equation (Equation2[2] ) based on predicted saturation concentration values. Compounds are distributed according to their saturation concentration.
![Figure 4. (a) Gas-particle partitioning of OC fractions from all ambient samples, with total loadings shown with full bars and the particle phase portion with filled bars. The total loading for each OC fraction is given by the average mass concentration of that OC fraction on quartz filters. Error bars indicate the standard deviation of particle fraction for each volatility bin. (b) Volatility distribution of a predicted loading of the average organic aerosol concentration in all ambient samples with COA = 3.41 μg m−3 calculated from Equation (Equation2[2] ) based on predicted saturation concentration values. Compounds are distributed according to their saturation concentration.](/cms/asset/a4f3326e-7159-4dbc-8bc7-79190071f934/uast_a_1158778_f0004_oc.gif)
The question is whether we can describe the observed partitioning behavior quantitatively. To try, we shall assume that each OC fraction corresponds to a specific effective saturation concentration, C*. It is very likely that the actual evolution temperature of organics from the quartz fiber filters in the OC/EC oven reflects desorption rather than evaporation from the original organic particles, but it is nonetheless reasonable to assume that on average the desorption temperature is related to volatility. To apply Equation (Equation2[2] ) to these data, we need to know the organic mass (OM) rather than OC for each sample. For this we estimate an organic-mass-to-OC ratio of 1.6 (Turpin and Lim Citation2001). From the average particle-phase OC of 2.13 µg-C m−3 we calculate an average particle-phase OM of 3.41 µg m−3.
In our study, the quartz filters collected mostly LVOCs and SVOCs (with substantial fractions in each phase), and we have five separate volatility fractions. It is evident from that several of the fractions show significant semi-volatile behavior (on average) and that the central fraction (OC3) is roughly 50:50 partitioned between the gas and condensed phases. Based on Equation (Equation1[1] ), this occurs when COA = C*, so we assume that the C* value for OC3 is roughly 4 μg m−3. For the rest of the fractions, we shall assume half-decade spacing as follows:
This VBS distribution is completely empirical. It is also very likely that this effective C* range is narrower than the true volatility distribution, but the effective distribution can still be useful, and we can quantitatively test whether it predicts the observed artifacts and partitioning behavior.
In we show the predicted partitioning for the aggregate ambient dataset based on the empirical volatility distribution and the BQ samples alone, using the VBS approach. The predicted partitioning for each fraction compares favorably with the observed partitioning shown in . The predicted total particle-phase OC is 2.39 μg m−3, which compares favorably with the observed value 2.13 μg m−3. Meanwhile the differences between measured POC and predicted POC are all within one standard deviation. The standard deviation of the mean should be even smaller as our dataset contains 213 samples, which means the particle concentrations of each fraction are stable, so this C* set can describe the partitioning behavior collected on quartz filters well. This is in fact well within the likely uncertainty range expected from the various positive and negative artifact corrections. This suggests that the simple BQ total OC measurement could be used, along with a VBS analysis, to recover the empirical volatility distribution of both the total OC and the particle-phase OC for a given sample, simplifying the task of predicting overall partitioning behavior for OC fractions defined by IMPROVE_A protocol and adding value to the conventional analysis method.
The VBS we employ here spans a narrow volatility range; based on the observed partitioning, most of the fractions appear to fall in the SVOC range. PC has the lowest effective saturation concentration at 10−0.4 μg m−3, but it is almost certain that this comprises all LVOC and ELVOC with volatility ≤10−0.4 μg m−3. If individual compounds evolve over several OC fractions, it is also possible that recovering the true volatility distribution would require deconvolution of the “OC fraction response function” to individual compounds. This would have the effect of rendering the true volatility distribution broader than the empirical distribution we use here. That, however, is left for future work.
We further assume that most IVOCs are not retained on quartz filters, but rather only the SVOCs corresponding to the narrow volatility range of our empirical VBS. There are many volatility bins with higher C* to the right of the range shown in that are not included in the basis set we employ, and evidently those organics are mostly in the gas phase. It is of course possible that some fraction of the OC1 signal comprises more volatile species that adsorb to the quartz filters, but it is equally possible that a portion of the vapors and particle-phase OC associated with OC1 is lost from the filters due to evaporation.
Figure 5. Seasonal variation of observed partitioning of all samples in (a) summer ambient, (c) winter ambient, and (e) tunnel, with total loadings shown with full bars and the particle phase portion with filled bars. The predicted partitioning for all samples are shown for (b) summer ambient, (d) winter ambient, and (f) tunnel. Error bars indicate the standard deviation of particle fraction for each volatility bin.
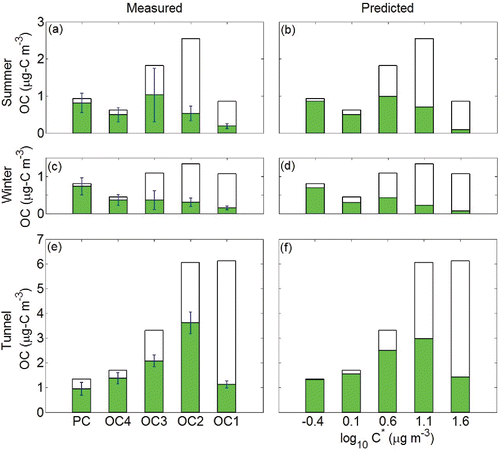
It is one thing for the empirical VBS to reproduce the OC fractions for the complete dataset. However, there are two more challenging tests for this relationship. The first is to assess the three major groupings separately (Summer, Winter, and Tunnel), and the second is to assess each individual filter, where partitioning should reflect the specific OC level for that sample. show that the VBS treatment reasonably reproduces the seasonal partitioning behavior. The total OC concentration was higher in summer than in winter. The difference between predicted particle-phase OC concentrations and measured concentrations for ambient samples is 0.24 μg m−3 in summer and 0.05 μg m−3 in winter, respectively. The higher summer concentrations are found mostly in OC2 and OC3. The difference between the observed and predicted particle fractions are all within one standard deviation, so we conclude that the partitioning observed on quartz filters can be described by our model.
A sterner test is the tunnel data set, which showed both much higher total OC and much higher volatility in general with high vapor content in OC1 and OC2. For the tunnel samples we apply an OM/OC of 1.24, consistent with fresh POA (Dallmann et al. Citation2014). show that our model again describes the observed partitioning reasonably well, with a predicted average particle-phase OC of 9.69 µg-C m−3 compared to the observed 8.48 µg-C m−3. The vehicular POC sampled in the tunnel is clearly semi-volatile in addition to being concentrated, with comparatively more material in volatile OC fractions, especially OC1 and OC2. This difference is strong evidence that aerosols in tunnel samples are more volatile than ambient aerosols due to the high concentration of fresh vehicular emissions in the tunnel.
The basis set we used for the tunnel data is the same as the one we used in ambient samples, with a lower OM/OC. With that difference, the effective saturation concentration that we assigned for the five OC fractions also reproduces the gas-particle partitioning in tunnel samples. The differences between observations and predictions are within one standard deviation, meaning the volatility bins used in this study are appropriate to describe OC fractions in IMPROVE_A temperature protocol.
PC has long been regarded merely as artifact of OC, but in this study we assigned PC a specific C* value and treat it as a low-volatility fraction. Empirically, it has been shown in many previous studies that PC is the least volatile fraction among the five fractions of OC as most of PC is in condensed phase (Watson et al. Citation2009; Zhu et al. Citation2010). However, some PC has been observed throughout all stages in the IMPROVE_A protocol, implying that PC can arise from a mixture of organic compounds with a wide range of volatility. Regardless, in our data, the PC formed during analysis of the QBT filters was negligible, suggesting that almost none of the vapors reaching the QBT filters was pyrolyzable. Thus, we conclude that most of the PC formed in our study arose from very low volatility organics.
It has also been shown that PC defined by different temperature protocols can differ significantly (Subramanian et al. Citation2006). Therefore, another issue associated with PC involves the choice of the charring correction method—transmittance or reflectance. Substantial charring takes place within the filter and so the drop of filter transmittance in the inert mode is larger than the drop of reflectance, which is dominated by charring only of the near-surface organics (Chow et al. Citation2004; Chen et al. Citation2010). Consequently, the PC concentration determined by the TOT (thermal/optical transmittance) method is ∼15% higher than PC determined by the TOR method. This difference can be very significant for EC determination, when the OC:EC ratio is large. For our analysis of OC partitioning, this represents a small correction to a relatively small portion of the total OC, and so it is not significant.
Effect of temperature dependence and dilution
So far, we have addressed average behavior for different seasons as well as the traffic tunnel. Next we shall use the VBS model to examine the effects of particle-phase OC concentration (COC) on the phase partitioning of individual samples. Semi-volatile combustion emissions enter the atmosphere and get mixed with background air quickly. Since ambient air is cooler and has much lower SVOC concentration, this mixing cools and dilutes the emissions, which causes repartitioning between the gas and particle phases. First cooling drives nucleation and condensation but measurements by using dilution samplers suggest that a large fraction of this POA may subsequently evaporate during isothermal dilution (Grieshop et al. Citation2009).
Changing the concentration of exhaust to drive a repartitioning between gas and particle phases is a standard approach to determine the volatility distribution of POA. This has been accomplished with dilution samplers (Lipsky and Robinson Citation2006), thermodenuders (Huffman et al. Citation2008; Huffman et al. Citation2009), and smog chambers (Grieshop et al. Citation2007; Ranjan et al. Citation2012). The data are then fit into VBS model using absorptive partitioning theory to determine the volatility distribution and particle fractions.
In this context, we can analyze the individual samples, including both ambient and traffic tunnel filters. is a partitioning plot showing the particle fraction versus particle-phase OC concentration (COC) for all individual ambient and tunnel samples. Points in the far upper right would correspond to high-concentration conditions dominated by fresh vehicular emissions. Dilution by ambient air drives the data diagonally down and to the left as total OC drops and partitioning progressively favors the gas phase. The upper-middle portion contains our tunnel samples, where emissions are diluted, but OA is still elevated. The left-hand portion contains ambient samples over a range of conditions.
Figure 6. Compilation of partitioning data for all samples and partitioning prediction for all samples using the VBS approach. Particle fraction versus organic carbon concentration is shown. The summer ambient data are shown with diamonds (red), the winter ambient data are shown with squares (green), and the tunnel data are shown with circles (blue). The lines are partitioning predictions for corresponding sampling groups. The circles represent averages over selected concentration ranges for these three sampling groups. Error bars indicate the standard error in the mean for those averages.
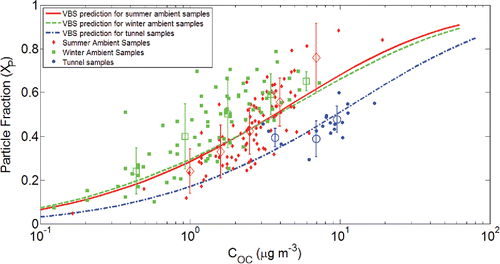
The individual data points are scattered but clearly follow a trend of decreasing particle fraction with decreasing COC. There are also clear differences between the three different groups (summer ambient, winter ambient, and tunnel). Consequently, we also show in the average COC and particle fraction for data points over selected COC ranges. These average points are shown with error bars indicating the standard error in the mean for each point. After this averaging, the partitioning behavior of the samples is clearly evident.
The dashed curves in depict the predicted equilibrium partitioning for a volatility distribution based on the averaged ambient data (i.e., ). It is significant that all samples from different groups follow the predicted partitioning behavior. This indicates that there was a consistent relationship between particle fraction and COC. The tunnel samples were collected in a POA-enriched environment, so they tend to have larger OA concentrations. However, the ambient samples, which have lower COC, are more scattered since they are aged and diluted in air. The VBS prediction curves slightly underestimate the particle fraction in summer ambient samples at high COC and underestimate the particle fraction in winter ambient samples by ∼0.05. This may be due to a lower temperature in our sampling van during the winter. The predicted partitioning behavior nicely describes the tunnel samples; they are shifted to the right compared to the ambient samples because of the lower OM/OC.
As expected, the particle fraction in both tunnel and ambient samples decreases monotonically with decreasing COC, which means that the particles evaporate as the air becomes diluted. For instance, in summer ambient samples, reducing COC from 10 to 1 μg-C m−3 would cause the particle fraction to shift from ∼0.6 to ∼0.2. As a result, at low COC, most of the organics are in the gas phase, but at high COC, the majority of the organics are in the condensed phase. On average, the summer ambient samples have higher COC values and larger particle fractions. On the other hand, the winter ambient samples tend to have smaller COC values and smaller particle fractions.
The samples analyzed here clearly and consistently show the signatures of semi-volatile phase partitioning. Averaged together, the OC fractions evolving at different temperatures suggest a volatility distribution that we can fit with an empirical five-bin VBS consisting of bins separated by ½ of an order of magnitude in volatility at 300 K, ranging from 0.1 to 30 µg m−3 saturation concentration. The observed partitioning based on the conventional Q-QBT analysis agrees well with the inferred partitioning derived from partitioning theory based on the BQ OC fraction data alone, suggesting that volatility bins can be used to correct positive sampling artifacts on single-filter BQ samples.
Partitioning behavior in ambient air can be affected significantly by temperature. For the VBS model, as suggested by Donahue et al. (Citation2006), a temperature change of 20 K results in a one-decade shift in the volatility bins (which are defined at 300 K). However, shows that an ambient temperature difference of 30 K (the average summer–winter temperature difference) is only associated with a change of ∼15% in the observed particle fraction. The principal reason is that the temperature of the filter packs inside our mobile lab changed only modestly between summer and winter.
It is also possible that OM/OC variation influenced the analysis. As shown in , the major difference between VBS predictions of ambient samples and tunnel samples was different assumed OM/OC ratios. For a given COC, a lower OM/OC ratio would lead to a lower particle fraction. So when applying this VBS model to other datasets, it is crucial to choose an appropriate OM/OC ratio as OM/OC ratio can vary significantly from one place to another, or from season to season.
Application to other datasets
To test the conclusions we have drawn from the Pittsburgh data, we analyzed several other datasets, and compared our model with results from the Q-QBQ approach for artifact correction.
A research campaign was conducted in Xueshan Tunnel, Taiwan, from October 2008 to July 2009 to measure the positive sampling artifacts of OC fractions for PM2.5 in a tunnel environment and the concentrations of each OC fractions were reported (Zhu et al. Citation2012). In this campaign, the Q-QBQ method was used to account for the positive artifact. The sampling interval varied from 3 to 6.5 h, resulting in relatively high filter exposure. We analyzed these data using the same VBS model described above and present the results in . Similar to our tunnel samples, we used an OM/OC ratio of 1.24 for the VBS calculation. Our VBS analysis of OC fraction data from the BQ filters alone reproduces the positive sampling artifacts and POC concentrations deduced by the Q-QBQ method with high fidelity. The average measured POC concentration is 24.18 μg-C m−3 while the POC concentration we calculated using the VBS model is 24.52 μg-C m−3. This result shows that our model works nicely for estimating POC concentrations in a POA-dominated tunnel environment, even for a long sampling period.
Figure 7. (a) Gas-particle partitioning of OC fractions from Xueshan Tunnel, Taiwan, with total loadings shown with full bars and the particle phase portion with filled bars. (b) The predicted partitioning behavior using same VBS model for this dataset. Similar to our tunnel samples, an OM/OC ratio of 1.24 was used in this model.
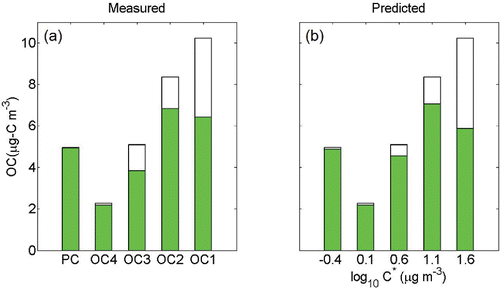
We analyze literature data from a field campaign in summer 1999 at the Fire and Mountain Ecology (FME) site and present the results in (Watson et al. Citation2009). In that study, both QBQ and QBT filters were used to estimate the positive artifact for 24-h samples. As with earlier findings, the Q-QBT overcorrected the artifact for these 24-h samples; in some OC fractions, the QBT OC signals were even higher than the BQ OC signals. For all OC fractions, the concentrations on the QBT filters were almost twice those on the QBQ filters (Watson et al. Citation2009). So here we use average QBQ OC and BQ OC concentration to fit in our model. For this modeling, we use an OM/OC ratio of 1.6. The particle fraction derived from Q-QBQ is 64%, which compares favorably with our calculated particle fraction of 60%. This result in turn shows that our model works nicely for ambient samples with longer sampling periods.
Figure 8. (a) Gas-particle partitioning of OC fractions from the Fire and Mountain Ecology site, with total loadings shown with full bars and the condensed phase portion with filled bars. (b) The predicted partitioning for this dataset. Similar to our ambient samples, an OM/OC ratio of 1.6 was used.

We have shown that our VBS model can be applied to short-duration filter samples by comparing our model results with the Q-QBT approach, which has the smallest biases for short-duration samples, but we have also shown that the model can be applied to long-duration samples by comparing our model results with the Q-QBQ approach, which has smaller biases for long-duration samples. For all these comparisons, the differences between the measured particle fraction and predicted particle faction were all within 10%, which shows that the VBS model we used is robust for estimating POC concentrations using only a BQ filter and the associated OC fractions.
Conclusions
We have demonstrated a new technique to estimate POC and semi-volatile OC phase partitioning for samples collected on quartz filters using thermo-optical OC/EC analysis and an empirical VBS model. Our major conclusions are as follows:
The OC fraction data from a BQ filter sample correlate with the volatility of sample organic constituents. We confirm this with conventional backup filter analyses and use the correlation to constrain a VBS model that successfully reproduces the conventional Q-QBT artifact correction while also providing information about the volatility distribution of the sample.
PC, though formed to an extent during all temperature stages, can be considered the least volatile organic fraction according to our volatility analysis as the particle fraction of PC is the highest among all five organic fractions; there is very little PC on the QBT filter.
By plotting particle fraction as a function of POC concentration for individual samples, we show that the particle fraction falls systematically as the total POC decreases. This decline follows the predictions of the VBS model quantitatively, confirming that the particles tend to evaporate when emissions are diluted by air, that is, moving away from source, and consequently lead to a repartitioning in gas and condensed phase and a smaller particle fraction.
By applying our VBS model to other datasets, we successfully reproduce the POC concentration derived by conventional methods using single-filter BQ data. This confirms that our empirical model works for both short-duration samples corrected by the Q-QBT approach and long-duration samples corrected by the Q-QBQ approach. Our single-filter VBS method can be directly applied to quartz filter data in general, and may be more robust than a somewhat arbitrary choice of Q-QBT for short-duration samples and Q-QBQ for long-duration samples.
Our data and model presented here provide a largely consistent picture of gas–particle partitioning on quartz filters. To extend our findings, a thorough analysis of all types of organic compounds would need combining the filter samples with other techniques. However, the data discussed here indicate that this shortcoming does not appear to be problematic from the perspective of predicting gas–particle partitioning of SVOCs under most atmospheric conditions.
In sum, it is convenient and beneficial to use this method for correcting positive sampling artifacts on quartz filters. We have shown that this new method can be easily applied to existing source data and historical filter samples. Moreover, based on our application of this empirical model to various datasets, the volatility distribution derived from this method can be directly used for analysis of a large number of quartz filter samples obtained under various sampling conditions.
supplemental_material.zip
Download Zip (169.1 KB)References
- Chen, S.-C., Tsai, C.-J., Huang, C.-Y., Chen, H.-D., Chen, S.-J., Lin, C.-C., Tsai, J.-H., Chou, C. C. K., Lung, S. C. C., Huang, W.-R., Roam, G.-D., Wu, W.-Y., Smolik, J., and Dzumbova, L. (2010). Chemical Mass Closure and Chemical Characteristics of Ambient Ultrafine Particles and Other PM Fractions. Aerosol Sci. Technol., 44:713–723.
- Chow, J. C., Watson, J. G., Chen, L.-W. A., Arnott, W. P., Moosmüller, H., and Fung, K. (2004). Equivalence of Elemental Carbon by Thermal/Optical Reflectance and Transmittance with Different Temperature Protocols. Environ. Sci. Technol., 38:4414–4422.
- Chow, J. C., Watson, J. G., Chen, L. W. A., Chang, M. C. O., Robinson, N. F., Trimble, D., and Kohl, S. (2007). The IMPROVE_A Temperature Protocol for Thermal/Optical Carbon Analysis: Maintaining Consistency with a Long-Term Database. J. Air Waste Manage. Assoc., 57:1014–1023.
- Chow, J. C., Watson, J. G., Chen, L.-W., Rice, J., and Frank, N. H. (2010). Quantification of PM 2.5 Organic Carbon Sampling Artifacts in US Networks. Atmos. Chem. Phys., 10:5223–5239.
- Chow, J. C., Watson, J. G., Lu, Z., Lowenthal, D. H., Frazier, C. A., Solomon, P. A., Thuillier, R. H., and Magliano, K. (1996). Descriptive Analysis of PM 2.5 and PM 10 at Regionally Representative Locations during SJVAQS/AUSPEX. Atmos. Environ., 30:2079–2112.
- Dallmann, T. R., Onasch, T. B., Kirchstetter, T. W., Worton, D. R., Fortner, E. C., Herndon, S. C., Wood, E. C., Franklin, J. P., Worsnop, D. R., Goldstein, A. H., and Harley, R. A. (2014). Characterization of Particulate Matter Emissions from On-Road Gasoline and Diesel Vehicles using a Soot Particle Aerosol Mass Spectrometer. Atmos. Chem. Phys., 14:7585–7599.
- Donahue, N. M., Epstein, S. A., Pandis, S. N., and Robinson, A. L. (2011). A Two-Dimensional Volatility Basis Set: 1. Organic-Aerosol Mixing Thermodynamics. Atmos. Chem. Phys., 11:3303–3318.
- Donahue, N., Robinson, A., Stanier, C., and Pandis, S. (2006). Coupled Partitioning, Dilution, and Chemical Aging of Semivolatile Organics. Environ. Sci.Technol., 40:2635–2643.
- Donahue, N. M., Robinson, A. L., and Pandis, S. N. (2009). Atmospheric Organic Particulate Matter: From Smoke to Secondary Organic Aerosol. Atmos. Environ., 43:94–106.
- Eatough, D. J., Long, R. W., Modey, W. K., Eatough, N. L. (2003). Semi-Volatile Secondary Organic Aerosol in Urban Atmospheres: Meeting a Measurement Challenge. Atmos. Environ., 37:1277–1292.
- Forster, P., Ramaswamy, V., Artaxo, P., Berntsen, T., Betts, R., Fahey, D.W., Haywood, J., Lean, J., Lowe, D.C., Myhre, G., Nganga, J., Prinn, R., Raga, G., Schulz, M. and Van Dorland, R. (2007). Changes in Atmospheric Constituents and in Radiative Forcing. In: Climate Change 2007: The Physical Science Basis. Contribution of Working Group I to the Fourth Assessment Report of the Intergovernmental Panel on Climate Change, S. Solomon, D. Qin, M. Manning, Z. Chen, M. Marquis, K.B. Averyt, M. Tignor and H. L. Miller, eds., Cambridge University Press, Cambridge, United Kingdom and New York, NY, USA.
- Grieshop, A. P., Donahue, N. M., and Robinson, A. L. (2007). Is the Gas-Particle Partitioning in Alpha-Pinene Secondary Organic Aerosol Reversible? Geophys. Res. Lett., 34:DOI: 10.1029/2007GL029987.
- Grieshop, A. P., Miracolo, M. A., Donahue, N. M., and Robinson, A. L. (2009). Constraining the Volatility Distribution and Gas-Particle Partitioning of Combustion Aerosols Using Isothermal Dilution and Thermodenuder Measurements. Environ. Sci. Technol., 43:4750–4756.
- Hart, K. M., and Pankow, J. F. (1994). High-Volume Air Sampler for Particle and Gas Sampling. 2. Use of Backup Filters to Correct for the Adsorption of Gas-Phase Polycyclic Aromatic Hydrocarbons to the Front Filter. Environ. Sci. Technol., 28:655–661.
- Huffman, J., Docherty, K., Aiken, A., Cubison, M., Ulbrich, I., DeCarlo, P., Sueper, D., Jayne, J., Worsnop, D., and Ziemann, P. (2009). Chemically-Resolved Aerosol Volatility Measurements from Two Megacity Field Studies. Atmos. Chem. Phys., 9:7161–7182.
- Huffman, J. A., Ziemann, P. J., Jayne, J. T., Worsnop, D. R., and Jimenez, J. L. (2008). Development and Characterization of a Fast-Stepping/Scanning Thermodenuder for Chemically-Resolved Aerosol Volatility Measurements. Aerosol Sci. Technol., 42:395–407.
- Kim, B. M., Cassmassi, J., Hogo, H., and Zeldin, M. D. (2001). Positive Organic Carbon Artifacts on Filter Medium During PM2.5 Sampling in the South Coast Air Basin. Aerosol Sci. Technol., 34:35–41.
- Kirchstetter, T. W., Corrigan, C. E., and Novakov, T. (2001). Laboratory and Field Investigation of the Adsorption of Gaseous Organic Compounds onto Quartz Filters. Atmos. Environ., 35:1663–1671.
- Lee, J. H., Hopke, P. K., and Turner, J. R. (2006). Source Identification of Airborne PM2.5 at the St. Louis-Midwest Supersite. J. Geophys. Res., 111:DOI: 10.1029/2005JD006329.
- Lipsky, E. M., and Robinson, A. L. (2006). Effects of Dilution on Fine Particle Mass and Partitioning of Semivolatile Organics in Diesel Exhaust and Wood Smoke. Environ. Sci. Technol., 40:155–162.
- Mader, B. T., and Pankow, J. F. (2001). Gas/Solid Partitioning of Semivolatile Organic Compounds (SOCs) to Air Filters. 3. An Analysis of Gas Adsorption Artifacts in Measurements of Atmospheric SOCs and Organic Carbon (OC) When using Teflon Membrane Filters and Quartz Fiber Filters. Environ. Sci. Technol., 35:3422–3432.
- May, A. A., Presto, A. A., Hennigan, C. J., Nguyen, N. T., Gordon, T. D., and Robinson, A. L. (2013). Gas-Particle Partitioning of Primary Organic Aerosol Emissions: (1) Gasoline Vehicle Exhaust. Atmos. Environ., 77:128–139.
- McDow, S. R., and Huntzicker, J. J. (1990). Vapor Adsorption Artifact in the Sampling of Organic Aerosol: Face Velocity Effects. Atmos. Environ. Part A. General Top., 24:2563–2571.
- Olson, D. A., and Norris, G. A. (2005). Sampling Artifacts in Measurement of Elemental and Organic Carbon: Low-Volume Sampling in Indoor and Outdoor Environments. Atmos. Environ., 39:5437–5445.
- Pankow, J. F. (1994). An Absorption Model of Gas/Particle Partitioning of Organic Compounds in the Atmosphere. Atmos. Environ., 28:185–188.
- Presto, A. A., Hennigan, C. J., Nguyen, N. T., and Robinson, A. L. (2012). Determination of Volatility Distributions of Primary Organic Aerosol Emissions from Internal Combustion Engines Using Thermal Desorption Gas Chromatography Mass Spectrometry. Aerosol Sci. Technol., 46:1129–1139.
- Ranjan, M., Presto, A. A., May, A. A., and Robinson, A. L. (2012). Temperature Dependence of Gas–Particle Partitioning of Primary Organic Aerosol Emissions from a Small Diesel Engine. Aerosol Sci. Technol., 46:13–21.
- Shrivastava, M. K., Lipsky, E. M., Stanier, C. O., Robinson, A. L. (2006). Modeling Semivolatile Organic Aerosol Mass Emissions from Combustion Systems. Environ. Sci. Technol., 40:2671–2677.
- Subramanian, R., Khlystov, A. Y., Cabada, J. C., and Robinson, A. L. (2004). Positive and Negative Artifacts in Particulate Organic Carbon Measurements with Denuded and Undenuded Sampler Configurations Special Issue of Aerosol Science and Technology on Findings from the Fine Particulate Matter Supersites Program. Aerosol Sci. Technol., 38:27–48.
- Subramanian, R., Khlystov, A. Y., and Robinson, A. L. (2006). Effect of Peak Inert-Mode Temperature on Elemental Carbon Measured Using Thermal-Optical Analysis. Aerosol Sci. Technol., 40:763–780.
- Turpin, B. J., Huntzicker, J. J., and Hering, S. V. (1994). Investigation of Organic Aerosol Sampling Artifacts in the Los Angeles Basin. Atmos. Environ., 28:3061–3071.
- Turpin, B. J., and Lim, H.-J. (2001). Species Contributions to PM2.5 Mass Concentrations: Revisiting Common Assumptions for Estimating Organic Mass. Aerosol Sci. Technol., 35:602–610.
- Turpin, B. J., Saxena, P., and Andrews, E. (2000). Measuring and Simulating Particulate Organics in the Atmosphere: Problems and Prospects. Atmos. Environ., 34:2983–3013.
- Watson, J. G., Chow, J. C., Chen, L. W. A., and Frank, N. H. (2009). Methods to Assess Carbonaceous Aerosol Sampling Artifacts for IMPROVE and Other Long-Term Networks. J. Air Waste Manage. Assoc., 59:898–911.
- Zhang, Q., Jimenez, J., Canagaratna, M., Allan, J., Coe, H., Ulbrich, I., Alfarra, M., Takami, A., Middlebrook, A., and Sun, Y. (2007). Ubiquity and Dominance of Oxygenated Species in Organic Aerosols in Anthropogenically-Influenced Northern Hemisphere Midlatitudes. Geophys. Res. Lett., 34:DOI: 10.1029/2007GL029979.
- Zhu, C.-S., Chen, C.-C., Cao, J.-J., Tsai, C.-J., Chou, C. C. K., Liu, S.-C., and Roam, G.-D. (2010). Characterization of Carbon Fractions for Atmospheric Fine Particles and Nanoparticles in a Highway Tunnel. Atmos. Environ., 44:2668–2673.
- Zhu, C.-S., Tsai, C.-J., Chen, S.-C., Cao, J.-J., and Roam, G.-D. (2012). Positive Sampling Artifacts of Organic Carbon Fractions for Fine Particles and Nanoparticles in a Tunnel Environment. Atmos. Environ., 54:225–230.