ABSTRACT
The Fourier-transform infrared (FT-IR) spectra of ambient fine aerosols were used with partial least-squares (PLS) regression to accurately, inexpensively, and nondestructively predict organic carbon (OC) on polytetrafluoroethylene (PTFE) filters in the U.S. Environmental Protection Agencies' Chemical Speciation Network (CSN). Recently, a similar FT-IR method was used for OC determination in the rural United States Interagency Monitoring of PROtected Visual Environments network, with the present work extending the method to urban aerosols with low mass loadings. In the present study, FT-IR spectra were calibrated to collocated thermal/optical reflectance (TOR) OC measurements following numerical processing with a second derivative filter, backward Monte Carlo unimportant variable elimination, and a quadratic discriminant analysis-PLS vapor correction routine. After processing and vapor correcting spectra, the number of model components (latent variables) were reduced from thirty-five to three with only the first PLS component patently predicting OC. The two lesser components modeled PTFE and inorganic interference remaining in the spectra. A wavenumber ranking procedure— using both the variable importance in projection and bootstrapped confidence intervals— underscored the primacy of aliphatic C-H stretches and carbonyl vibrations for OC prediction. Aliphatic deformations, amines, organonitrate, carboxylate, and aromatic vibrations were also valuable for OC quantification. This study demonstrates that PLS models quantifying TOR OC are explicable in terms of organic functional group absorption after judiciously processing FT-IR spectra.
Copyright © 2016 American Association for Aerosol Research
EDITOR:
1. Introduction
In the United States, the Chemical Speciation Network (CSN) collects, analyzes, and speciates particulate matter (PM) from urban and suburban sites while the Interagency Monitoring to PROtect Visual Environments network (IMRPOVE) monitors PM at rural sites (Malm et al. Citation2011; Solomon et al. Citation2014). Speciated fine particulate matter measurements (<2.5µm diameter, 50% cut point; PM2.5) quantify visibility degradation in pristine areas (Malm and Hand Citation2007; Watson Citation2002), aid in apportioning source contributions to particulate pollution (Baumann et al. Citation2008; Chen et al. Citation2010; Chow et al. Citation2015), constrain atmospheric aerosol models (Turpin et al. Citation2000; Dubovik et al. Citation2002; Carlton et al. Citation2008), and are associated with an array of adverse health effects (Pope III and Dockery Citation2006).
The CSN and IMPROVE networks analyze PM to determine the mass of inorganic ions including sulfate (SO42−) and nitrate (NO3−), elements related to soil, sea salt, and anthropogenic sources as well as organic and elemental carbon (OC and EC). Both networks measure OC and EC using the thermal optical reflectance (TOR) method (Chow et al. Citation1993; Chow et al. Citation2007). The IMPROVE_A TOR protocol operationally defines OC as the total fraction of carbonaceous material volatilized from a quartz fiber filter below 580°C in the absence of oxygen. Refractory carbon (EC) evolves from quartz sampling filters at higher temperatures in the presence of oxygen. While the TOR method reliably fractionates carbonaceous material by exploiting the volatility and then oxidation of condensed phase aerosols, the method is time consuming, costly, and destroys the aerosol.
Researchers have thus sought non-destructive, fast, and inexpensive methods for characterizing the carbon constituent of PM2.5 collected on filter media. Fourier-transform infrared (FT-IR) spectroscopy is a method well-suited to resolve mid-infrared absorption bands associated with organic and inorganic aerosol constituents bound to porous media (Allen et al. Citation1994; Boer et al. Citation2007; Griffiths and De Haseth Citation2007). Given the complexity of ambient PM2.5 spectra, carefully chosen FT-IR absorption bands measured from model compounds and mixtures have been used to quantify organic functional groups (Maria et al. Citation2002; Russell Citation2003; Takahama et al. Citation2013; George et al. Citation2015). Absorption bands are deliberately chosen to estimate the moles of a functional group present in an ambient sample with combinatorial and/or mole balance arguments then used to quantify OC and EC.
Alternatively, a multivariate partial least squares (PLS) regression considers each channel in an FT-IR spectrum—as opposed to preselected absorption bands—as potentially predictive of OC and EC, relying on the algorithm to determine the best combination of features to use for future (blind) predictions (Wold et al. Citation1983; Geladi and Kowalski Citation1986; Wold et al. Citation2001; Næs et al. Citation2002). The PLS determination of aerosol species bound to polytetrafluoroethylene (PTFE) filters has been demonstrated for IMPROVE network samples (Ruthenburg et al. Citation2014; Dillner and Takahama Citation2015a, Citation2015b; Reggente et al. Citation2015). Specifically, a PLS regression finds the best linear combinations of absorption features in the FT-IR calibration spectra, projects those features onto a low-dimensional subspace spanned by orthogonal components, and develops a calibration within this subspace. Although many PLS algorithms are used today (Trygg and Wold Citation2002; Rosipal and Krämer Citation2006), the nonlinear iterative partial least squares (NIPALS) algorithm was used in the present study to determine TOR OC in the CSN. Two fundamental calibration equations are derived from the NIPALS algorithm as[1]
[2]
Here, the calibration spectra, , are decomposed into the product of two matrices: the PLS orthogonal scores,
, and transposed loadings,
. The column-vectors that comprise the scores and loadings matrices, denoted
and
, are derived by directly referencing the TOR OC measurements (
). Companion plots (e.g., plotting
vs. wavenumber) may therefore aid in interpreting which functional groups are most important for modeling OC. The error matrix,
, represents all unmodeled variability in
including additive noise and non-interfering (but redundant) FT-IR absorption. For calibration, PLS weights
according to the regression coefficients (
) to estimate OC or EC in calibration samples—with model errors (
) conforming to most least-squares regression assumptions (Krämer and Sugiyama Citation2011; Williams et al. Citation2013).
Routine determination of OC or EC in field samples benefits from transforming regression coefficients back into absorbance units (AU) according to[3] yielding the following prediction equation (with “T” denoting prediction samples)
[4]
Although the PLS loading weights, , are discussed in detail elsewhere (Wold et al. Citation2001), we may simply consider
in (Equation3
[3] ) as a linear operator that changes the basis of the regression coefficients from the orthogonal component-space back into the original FT-IR measurement-space. The quantity of OC or EC are readily predicted in field samples using (Equation4
[4] ) with the magnitude of prediction errors (
), in theory, identically distributed to calibration errors (
).
A major advantage of PLS over traditional methods is that it simultaneously handles predictor collinearity—inherent and excessive to absorption spectra—while generating parameterizations of the original variables referred to as components, latent variables, or factors. Since the components are derived by referencing the TOR OC measurements directly, OC infrared absorption bands may be archetypically rendered onto the components in a manner analogous to using positive matrix factorization (PMF) for aerosol source apportionment (Lee et al. Citation1999; Kim et al. Citation2003; Jimenez et al. Citation2009a; Russell et al. Citation2011). However, the signal-to-noise ratio, interference from non-carbonaceous mixed aerosol species, and absorption from the PTFE filter itself complicates a factor interpretation by vastly increasing the number and modifying the character of the components used for calibration. Model interpretation therefore depends critically upon the scaling, transformation, predominance of interferences, and quality of the FT-IR spectra.
One year of samples from seven sites in the IMPROVE network were used to calibrate the FT-IR spectra of PM2.5 collected on PTFE filters to TOR OC and EC determined from quartz filters (Dillner and Takahama Citation2015a, Citation2015b). Inspection of the FT-IR spectra indicated significant interference from the strong C-F absorption of PTFE (∼1400–1000 cm−1, <650 cm−1) (Liang and Krimm Citation1956; Starkweather Jr et al. Citation1985; Quarti et al. Citation2013). A large, gradually sloping baseline above 1400 cm−1 also dominated the FT-IR spectra and was likely related to the scattering of infrared radiation by the PTFE substrate (Presser et al. Citation2014). A specific aim of the study was to compare the prediction capabilities of PLS when the FT-IR spectra were pretreated to remove PTFE interference. Three analysis schemes were proposed. First, pretreatment was limited to the removing interpolated data points, the result of zero-filling interferograms. These “raw” spectra contained 2784 absorption channels. Second, the raw FT-IR spectra were baseline corrected using a polynomial fitting procedure to remove the baseline above 1500 cm−1 and minimize water vapor and carbon dioxide absorption (Takahama et al. Citation2013). A third scheme truncated raw spectra below 1500 cm−1 thereby completely removing PTFE bands from the calibration problem. Irrespective of spectral pretreatment, a many-component PLS calibration accurately predicted independent TOR OC and EC test samples with an error, bias, precision and minimum detection limit (MDL) equivalent or better than collocated (replicate) TOR measurements.
The spatial and temporal sensitivity of the calibrations developed from the 2011 IMPROVE samples were next evaluated (Reggente et al. Citation2015). TOR OC and EC were predicted accurately in IMPROVE samples collected in the 2013 sampling year for the same sites (OC: R2 = 0.97; EC: R2 = 0.95). Predictions were also viable for other IMPROVE sites not included the calibration (OC: R2 = 0.89; EC: R2 = 0.87). However, TOR OC and EC calibrations showed more spatial (geographic) sensitivity according to diminished R2 and higher testing-sample error. These sensitivities were ultimately connected, in one instance, to mixed agricultural and urban emissions (Fresno, CA) and, in another instance, to high urban mass loadings coupled with unique dust and shipping emissions (South Korea). Alternative calibrations were developed for these sites after quantifying their deviance with respect to the 2011 samples using a squared Mahalanobis distance metric. Fresno and Korea samples were better predicted by an alternative calibration (OC: R2 = 0.96; EC: 0.66 ≤ R2 ≤ 0.93) suggesting that including spectra from all sites in the calibration or assigning FT-IR spectra to alternative calibrations may be warranted in certain cases.
Identifying which functional groups were used for OC and EC prediction was intractable for the large (many component) PLS models developed in Dillner and Takahama (Citation2015a, Citation2015b). In Takahama et al. Citation(2016) sparse PLS and elastic net regression were applied to both raw and baseline corrected spectra to determine an optimal subset of wavenumbers for OC and EC quantification; optimal in the sense that prediction errors are reduced and/or model interpretation was facilitated. In many instances, the sparse methods reduced the numbers of predictors required for calibration by an order of magnitude and reduced the number of components (latent variables) by more than half. Summative measures of predictor importance (e.g., the variable importance in projection, VIP) as well as the sign of PLS regression coefficients (±) aided in revealing which absorption features were vital to predicting TOR OC and EC by the sparse methods (Chong and Jun Citation2005b). The most accurate and parsimonious PLS solutions relied on aromatic, amide, and ester functional groups vibrations. Alkanes, alkenes, and carbonyl vibrations were also used for the TOR OC models with EC predictions occasionally requiring the use of ether bands.
Rather than extrapolate existing IMPROVE calibrations to CSN samples—a process known as calibration transfer (Feudale et al. Citation2002; Chen et al. Citation2011)—the present study develops new calibrations to determine OC. Three particular challenges prevent a straightforward calibration transfer including: the noted chemical diversity of urban fine aerosols (see Reggente et al. Citation2015 discussion above), significantly greater PTFE interference in CSN spectra, and a lower areal density of PM2.5 on CSN filters (µg/cm2). First, the proximity of samplers to urban emission sources and extent of atmospheric aging likely modify the average infrared absorption behavior of OC species to the extent that rural-IMPROVE calibrations may not predict urban-CSN OC with good accuracy and low bias (Pöschl Citation2005; Kroll and Seinfeld Citation2008; Jimenez et al. Citation2009a). Furthermore, the FT-IR spectra of two Fresno, CA samples illustrate dramatic differences in both PTFE and PM2.5 absorption, singularly preventing a simple calibration transfer (). Here, the thicker CSN PTFE filter (∼40 µm vs. ∼30 µm) shows considerably greater absorption and scattering. In addition, a much larger deposition area (11.3 cm2 vs. 3.5 cm2) and lower total volume of air sampled in the CSN (9.6 m3 vs. 32.8 m3 over 24 hours) reduces the quantity of aerosol within the infrared beam path. For collocated measurements, the areal density of CSN samples is nominally lower by a factor of ∼11, proportionally reducing the average absorption cross-section of PM2.5 (AU/cm2). However, this reduction in areal density represents an upper estimate since a higher concentration of OC in urban aerosols tends to reduce differences in total OC mass between the two networks. In addition, the greater face velocity in IMPROVE samplers (108 cm/s vs. 10 cm/s) (McDow and Huntzicker Citation1990; McDade et al. Citation2009)), longer field latency in the IMPROVE network (7 days vs. as short as 48 hours for the CSN), and differences in shipping and storage (Dillner et al., Citation2009; Solomon et al., Citation2014) complicates linking mass distribution differences to specific sampling artifacts. Ultimately, the lower signal-to- (PTFE) background ratio of CSN spectra may altogether prevent determining OC using the FT-IR method. Therefore the first aim of this study is to prove that the FT-IR method is not restricted to the IMPROVE network.
Figure 1. FT-IR spectra of a heavily-loaded Fresno, CA samples (PM2.5 = 78.6 µg/m3, TOR OC = 13.5 µg/m3) acquired on 12/15/2013 from the CSN and IMPROVE network. The IMPROVE spectrum shows less scattering (broad baseline above 1400 cm−1), lower PTFE absorption, and greater absorption of PM2.5 than the collocated CSN spectrum.
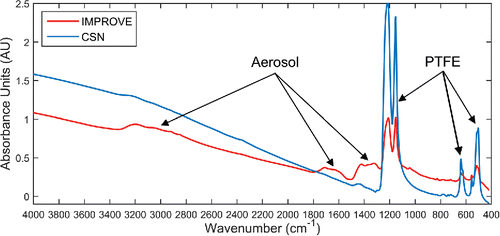
Once the FT-IR method is proven for CSN samples the functional groups considered indispensable for OC quantification are evaluated. As demonstrated by Takahama et al. Citation(2016), numerically processing spectra by baseline correction and wavenumber selection yields parsimonious PLS solutions less encumbered by matrix interferences that are easier to interpret. Alternative approaches are used in the present study to aid interpretation and include transforming raw into second derivative spectra to suppress baseline interferences, eliminating wavenumbers weakly correlated to OC using backward Monte Carlo unimportant variable elimination (BMCUVE) (Weakley et al. Citation2014), and using a principal component decomposition with a quadratic discriminant analysis (QDA) to remove water vapor interference from derivative spectra (Cowe and McNicol Citation1985; Nieuwoudt et al. Citation2004; Dixon and Brereton Citation2009). Interference from strongly absorbing PM constituents (e.g., ammonium salts) and PTFE are also investigated at each stage of spectral manipulation to ascertain their presence and impact on OC determination. Finally, the most parsimonious and interference-free model is interpreted to advance a fundamental understanding of those OC functional groups comprising urban PM2.5 in the CSN.
2. Method
2.1. CSN aerosol sampling and analysis
One year of samples, collected in 2013 at ten CSN sites were used in this study (). Birmingham, AL, Cleveland, OH, Elizabeth, NJ, Fresno, CA, Salt Lake City, UT, have high PM concentrations that do not meet U.S. National Ambient Air Quality Standard (NAAQS) for PM2.5 while Boston, MA, E. Providence, NJ, Phoenix, AZ, Seattle, WA and Washington, DC have lower concentration that are in attainment for NAAQS (https://www3.epa.gov/airquality/particlepollution/designations/2006standards/state.htm). Each site has a unique set of sources of organic matter and meteorological conditions which impact the composition of the aerosol. For example, the Elizabeth NJ site is located next to a refinery and the New Jersey Turnpike and Fresno, CA is surrounded by agriculture and experiences extended atmospheric inversions during the winter.
Each site has a one-day-in-three sampling schedule and two sites (Cleveland, OH and Boston, MA) have collocated 1-in-6 sampling. Samples are collected midnight to midnight local time. Samples collected on PTFE filters (Whatman PM2.5 membranes, 47 mm) using a SASS sampler (MetOne, Grant's Pass, OR, 6.7 liters per minute) are used for gravimetric and elemental analysis in the CSN network and for FT-IR analysis in the project. Samples are shipped and stored cold (4°C)
2.1.1. TOR OC measurements
In the CSN, OC and EC are measured using the TOR method on quartz filters (Pall, 25 mm) collected with a URG-3000N sampler flow controlled to maintain 22.8 liters per minute. In TOR analysis, a small portion of the filter is heated using a prescribed temperature ramp following the IMPROVE_A protocol (Chow et al. Citation2007). Speciating carbon according to the TOR protocol is complicated by the pyrolysis of organic carbon (OP) occurring prior to the oxidation and evolution of EC from the sampling filters. If left uncorrected, OP contributes a false-positive artifact to the EC and consequently underestimates OC on the quartz filters. Thus, OP is demarcated, defined, and removed from EC and added to OC when the reflectance of a He-Ne laser off the sampling filter returns to its base value (Chow et al. Citation1993). Total OC constitutes the sum of OP and each volatile OC fraction (OC = OC1+OC2+OC3+OC4+OP).
Samples and blank TOR data were obtained from the US Environmental Protection Agency's Air Quality System (AQS) database on July 27, 2015 (http://aqsdr1.epa.gov/aqsweb/aqstmp/airdata/). EPA is in the process of adopting a gas-phase artifact correction protocol, already implemented by IMPROVE, to remove the positive artifact from non-PM species adsorbed onto field samples (http://vista.cira.colostate.edu/improve/Data/QA_QC/Advisory/da0032/da0032_OC_artifact.pdf). Artifact correction subtracts the median monthly field blank OC values from each ambient sample. In this study, the OC measurements were field blank corrected using the median monthly values estimated from the 10 CSN sites.
2.1.2. Collocated sampling
Collocated CSN, IMPROVE, and Southeastern Aerosol Research and Characterization (SEARCH) samples were used to evaluate the quality of TOR data in two ways: to remove substandard TOR samples as well as evaluate the precision of the TOR and FT-IR predictions. First, collocated TOR data were used to identify whether systematic deviations (drift) between collocated samplers were present. Visual inspection of collocated TOR OC scatter plots was used to ascertain the quality of the TOR data. A linear regression confirmed the presence of significant drift between collocated samplers, requiring the removal of a sampling site from further consideration. Regardless of cause, drift was considered present and significant if the slope and intercept of the regression was significantly different than one and/or the intercept was non-zero (i.e., ; α = 0.01). Cleveland, OH and Boston, MA sites were evaluated for drift using collocated CSN data. The Boston samplers did not show significant drift upon inspection and according to the regression analysis. However, the Cleveland replicates showed significant drift (
; α < 0.01). The maintenance record from the CSN database provided evidence as to which Cleveland sampler was likely compromised. This allowed the appropriate Cleveland data set to be retained for PLS calibration and the other collocated set removed.
Phoenix, AZ, Fresno, CA, Birmingham, AL, and Seattle, WA had parallel TOR measurements from the IMPROVE network which used very similar samplers and an identical TOR protocol. Regression analyses indicated that gas-phase artifact correction was likely effective for all sites, as indicated by a zero intercept for each site (Malm et al. Citation2011). Phoenix, Fresno, and Seattle sites showed regression slopes always deviating positively from one (i.e., ; α < 0.01), to about the same degree. Furthermore, CSN samples from these three sites contained, on average, 11% ± 2.5% higher OC mass concentration than IMPROVE samples. These results conformed (within error) to observations made by Rattigan et al. Citation(2011) for collocated sampling at an urban New York site as well as a laboratory study of collocated samples stored at temperatures to mimic IMPROVE and CSN conditions (Dillner et al. Citation2009).
Birmingham samples exhibited significant drift ( which could not be explained by the retention of semi-volatiles, i.e., IMPROVE samples actually contained more OC than CSN samples at the Birmingham site. The Birmingham site also had parallel TOR measurements from the SEARCH network. The SEARCH and IMPROVE TOR measurements agreed closely, suggesting, that the CSN Birmingham measurements were unreliable. Birmingham data were therefore excluded from PLS modeling. Due to removing the Birmingham and one Cleveland dataset, the total number of samples decreased from 1045 to 927 with 30 field blanks (see Section S1 of the online supplemental information [SI] for details).
2.2. FT-IR analysis
PTFE samples and field blanks were brought to room temperature from cold storage prior to taking an FT-IR spectrum using the Bruker Tensor 27 spectrometer (Bruker Optics, Billerica, MA). No other sample preparation was performed. Filters were analyzed in transmission mode (4000 cm−1 to 420 cm−1) at 4 cm−1 nominal resolution with a liquid nitrogen cooled mercury-cadmium-telluride detector. Absorbance spectra were calculated by taking the ratio of a filter spectrum against a reference spectrum of the empty sample compartment. Compressed laboratory air was scrubbed of water vapor and carbon dioxide and circulated within the optical compartments of the instrument (PureGas LLC, Broomfield, CO). The sampling compartment was purged for 4 minutes prior to acquiring a filter or reference spectrum to remove carbon dioxide or water vapor that entered the system during a sample change. Additional details on FT-IR analysis can be found in Ruthenburg et al. Citation2014.
2.3. PLS calibration and model selection
2.3.1. Data partitioning
Samples from the 9 remaining CSN sites (N = 927) were sorted by site and sampling date (Dillner and Takahama Citation2015a, Citation2015b). Every third sample from each site was placed in the test set with the remaining allocated to the calibration set with the exception of 51 collocated Boston, MA samples which were partitioned one-to-one. This resulted in a nearly 2-to-1 division of samples into either calibration (Nc = 598) or test (NT = 329) samples. Ordering samples by site and date prior to partitioning constitutes a form of stratified sampling that attempts to maintain a similar OC mass distribution of samples (μg/m3) between the sets while also spanning the same seasonal variations in aerosol composition.
2.3.2. Design matrices for multivariate analysis
The FT-IR spectra are stacked into two matrices after stratified sampling with rows corresponding to spectra and columns to their absorption measurements (indexed by wavenumber). In general, any -by-p design matrix may be expanded as
[5] with
indicating the jth absorption measurement corresponding to the ith FT-IR spectrum for the kth design matrix. For this study, each design matrix contained
total spectra, subscripted according to affiliation with either PLS calibration (k = c) or model testing (k = T). Stratified sampling therefore generated a calibration matrix,
, containing 598 rows and 2784 columns and the testing matrix,
, containing 329 and 2784 columns. The design matrix was expanded in (Equation5
[5] ) as p column-vectors to better illustrate that the total number of predictors used by PLS was initially very large (p = 2784).
2.3.3. FT-IR spectral processing
Spectra processing—including derivative transformation and wavenumber selection—has a large impact on which information the PLS algorithm projects onto the PLS components. Processing FT-IR spectra may either completely remove unwanted absorption from the calibration problem or modify the character of the FT-IR absorption such that the PLS algorithm consigns unwanted variability to the residual matrix (see in (Equation1
[1] )). With extraneous information removed or suppressed, the PLS calibration is likely more interpretable in terms of OC functional groups.
summarizes the core processing methods used to construct calibrations from the raw, processed, and vapor-corrected and processed (VCP) spectra. For the raw calibration (I), untreated spectra are passed directly to PLS without any attempt to suppress baseline or remove background interferences. Processed spectra are developed by transforming raw into second derivative spectra followed by BMCUVE wavenumber optimization (II). PLS calibration is embedded as a subroutine in the BMCUVE program and is therefore represented as a single block in the flow chart (BMUCVE+PLS). The VCP model (III) is developed from the same processed spectra in (II) except that the water vapor contamination is identified, isolated, and removed using a QDA-PLS method following principal component analysis (PCA). Section 2.3.4 (below) discusses the use of PCA in identifying interferences in the FT-IR spectra while Section S2 in the SI provides a derivation of the QDA-PLS correction procedure.
Figure 3. Flow chart illustrating the three model development procedures used in this study. Note that vapor correction (III) does not modify the quantity of predictors (wavenumbers) used for calibration, only the character of the absorption indexed on those wavenumbers.
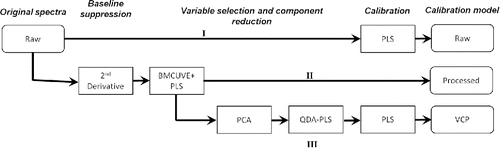
Processing FT-IR spectra constituted first transforming the raw into second derivative spectra followed by reducing the number of predictors (columns) in using the BMCUVE algorithm. First, second-derivative spectra were generated by applying a symmetric, second-order, 21-point, Savitsky-Golay filter to the raw spectra (Savitzky and Golay Citation1964). Derivative transformation aimed to suppress the broad baseline in the raw spectra. Filtering removed 10 channels at the beginning and end of each spectrum reducing the total number of predictors to 2764, the spectral range to 3986–433 cm−1, and design matrices by 20 predictors.
The BMCUVE algorithm was next employed to objectively remove baseline, background, or otherwise redundant OC absorption from the second derivative spectra (Weakley et al. Citation2014). As a randomized wrapper method (Saeys et al. Citation2007), BMCUVE interacts with the PLS regression to iteratively prune wavenumbers from until a best subset to use for OC calibration is identified, usually yielding much smaller design matrices (
2784). BMCUVE begins by considering the 2764 predictors in
as viable for calibration, estimates the number of PLS components necessary to predict OC, applies reliability criteria to rank each predictor's importance in regression, and then removes a fixed fraction of unreliable predictors (Centner et al. Citation1996; Cai et al. Citation2008). The process of estimating components, ranking predictor importance, and removing unreliable variables is repeated until a best subset of predictors is identified according some goodness-of-fit criterion. Ultimately, the best PLS model—optimized in terms of both PLS components and predictors—was chosen according to a minimized root-mean-squared-error of cross-validation (RMSECV) with the estimated number of components later refined using the Wold R criterion (Li et al. Citation2002). Further details concerning the Monte Carlo estimation of reliability criteria, specifying the appropriate resampling parameters, and defining the algorithm's stopping criteria are developed in Weakley et al. Citation2014. The net result of second derivative transformation and BMCUVE will be referred to as processing.
2.3.4. Exploring and removing interferences from the spectral matrices
A principal component analysis (PCA) was performed on the raw and processed calibration spectra to explore the extent of baseline, background, and inorganic interferences in the FT-IR spectra (Cowe and McNicol Citation1985; Nieuwoudt et al. Citation2004). Similar to PLS, a PCA decomposes into a matrix of scores and loadings (see (Equation1
[1] )) but unlike PLS, which develops components according to the joint covariation between predictors and response (
and
), PCA defines orthogonal components according to the directions of maximal variation within
(Yamamoto et al. Citation2009; Abdi and Williams Citation2010). PCA thus provides a clearer picture of the systematic absorption variations in the CSN spectra, uninfluenced by the TOR OC response vector. Weakley et al. Citation(2012) provide additional illustrations of using principal components to assess systematic variations in spectral matrices. To avoid confusion when discussing PCA, principal component scores are denoted “PC” and subscripted according to their association with a given component.
Although the FT-IR instrument and the sample compartment were purged of water vapor and carbon dioxide small amounts of these constituents were identified by PCA in processed spectra. A quadratic discriminant analysis (QDA) coupled with an auxiliary PLS regression removed this interference from the processed spectra in order to prevent the strong vapor absorption lines from influencing the interpretation of OC functional groups. Processed spectra free from water vapor contamination will be referred to as vapor-corrected and processed (VCP) spectra.
2.3.5. PLS component selection and outlier identification
Either raw, processed, or VCP spectra were used for TOR OC calibration and prediction. Every design matrix, regardless of the extent of processing, was standardized to unit variance prior to calibration and prediction and TOR OC response vectors () were mean centered (Helland Citation1988; Bocklitz et al. Citation2011). Wold's R criterion was applied after 5-fold cross-validation to identify the correct number of components to use in each calibration (Li et al Citation2002). Wold's R criterion is calculated as
where MSECV denotes the mean-squared error of cross-validation and a indexes the current PLS component used in the cross-validation. Wold's R assumes that a local minimum on an MSECV plot corresponds to the true size of the regression subspace, therefore preventing overfitting the PLS model. For this study, the first A components scoring less than 0.95 on Wold's R were accepted for calibration (Krzanowski Citation1983). Further details on the use of Wold's R criterion for model selection can be found in Section S3 of the SI.
Calibration outliers were identified using standardized calibration residuals and leverage statistics (Isaksson and Næs Citation1988). Details concerning outlier identification and removal can be found in the SI (Section S4). Eighteen spectra were found to be outlying when raw spectra were used for calibration (Nc = 580). Nine outliers were identified for the processed and VCP models (Nc = 589).
2.4. Method validation using figures of merit
The three FT-IR models were validated using the prediction equations developed in (Equation4[4] ). The performance of each method was compared on seven figures of merit. Five figures used every test sample in their calculation including the coefficient of determination (R2), median bias (μg/m3), median absolute error (μg/m3), and normalized median absolute deviation or MAD (%). The MAD is a robust and bias-corrected goodness-of-fit measure that parses the bias contribution from error. The concentration-normalized MAD was calculated as
[6] where
represents the median of an absolute quantity,
the difference between the FT-IR and TOR measurements for the ith sample,
a vector comparing all FT-IR and TOR measurements, and
the ith TOR OC measurement. The performance of each FT-IR model was also judged by comparing to the same metrics for collocated TOR data to assess TOR analysis and aerosol sampling error. Specifically, 51 sample-pairs from the Boston site were used to develop collocated TOR metrics.
The minimum detection limit (MDL) was estimated from field blank spectra as three times the standard deviation of model-predicted test set blanks (NB = 11). The percent of samples below the MDL were also used to qualify the performance of each model. Precision was calculated using collocated Boston test sample-pairs (Ncol = 26) in the following expression:[7] where
represents the difference between the ith predicted sample-pair using either the FT-IR or TOR method.
Bootstrapping-by-residuals were used to develop 95% confidence intervals for bias, MAD, MDL, and precision (Efron and Tibshirani Citation1993; Zhang and Garcia-Munoz Citation2009). Specifically, percentile-based bootstrapped confidence intervals were developed using 7500 bootstrap replicates for each metric. Confidence intervals were used to test the null hypothesis that a figure of merit estimated by the FT-IR method was not significantly different than one estimated by the TOR method (Gardner and Altman Citation1986). The null hypothesis (FT-IR = TOR) was not rejected when confidence intervals overlapped and was rejected (FT-IR ≠ TOR) when confidence intervals did not overlap.
2.5. FT-IR variable importance and infrared band interpretation
Wavenumbers were assigned to infrared functional groups for the calibration showing the best performance on the seven figures of merit. First, the variable importance in projection (VIP) was used to indicate which wavenumbers in the FT-IR spectra were most critical to predicting OC. The VIP succinctly expresses the total analyte variation “explained” by the PLS components as distributed on each predictor used for calibration. Although this is only one way to conceive the VIP, a higher VIP score indicates that a predictor, and its corresponding wavenumber, is relatively more important to the calibration than others. Formally[8] where
is the variable importance in projection of the jth predictor (column) from
, p are the total number of predictors in
,
the percent of y-variance explained by the ath PLS component, and
is the normalized loading weight value (
) for the ath component indexed on the jth predictor (see Section S5 in the SI for a restatement of VIP in terms of
). Heuristic thresholds have been employed to determine whether a predictor is considered sufficiently “important” to a PLS model (Chong and Jun Citation2005a). The customary VIP > 1 threshold was adequate for our purposes.
The VIP provides a convenient measure of a wavenumber's importance to the calibration and, in principle, the value of that wavenumber in OC prediction. Having been derived using only calibration samples, the VIP does not consider the uncertainty in estimating the PLS parameters. Failing to consider the sensitivity of the calibration to noisy perturbations—particularly from PTFE baseline and background—may lead to reduced confidence in assigning infrared absorption to functional group vibrations. Therefore, a measure of predictor “stability” complimented the calculation of the VIP to screen unreliable predictors from the interpretation problem (Mehmood et al. Citation2012).
Stability was considered by calculating 95% bias-corrected and accelerated bootstrapped confidence intervals (BCIs) for each regression coefficient. If the BCI did not capture zero this indicated that the regression coefficient was plausibly non-zero and its associated predictor sufficiently stable (α = 0.05). Taken together, a predictor showing a > 1 (important) and regression coefficient not equal to zero (stable) was considered safely interpretable. Predictors failing to meet these two conditions were removed from interpretation (not the model). The consecutive application of VIP estimation, followed by BCI calculations, disregarding predictors, and then sorting according to VIP will be referred to herein as VIP-BCI ranking.
Infrared bands were assigned to wavenumbers considered interpretable by VIP-BCI ranking. Mayo et al. Citation(2004) and Shurvell Citation(2002) provided most of the theoretical and reference material used to assign VIP-BCI features to specific infrared bands. Plausible band assignments were based on the following considerations: theoretical infrared activity, functional group abundance in primary and secondary organic aerosols (POAs and SOAs), the sensitivity of the vibration to local chemical environment, multiple vibrations identified by VIP-BCI corresponding to the same functional group (e.g., stretches and bends), and visual confirmation of the bands presence on a blank-corrected second derivative spectrum. Band assignments were generally considered “tentative” according to the proximity of a feature to the very strong C-F stretches of PTFE (1400–1000 cm−1) and band resolution. Bands known to reside at or near ammonium, nitrate, sulfate, and other inorganics were also assigned with caution.
2.6. Chemometric and statistics software
Basic data manipulation and PCA was performed using the Matlab™ base, statistics, and signal processing toolboxes (2015a, The MathWorks, Inc., Natick, MA, United States). The NIPALS estimator was used for PLS calibration, prediction, and regression coefficient estimation (Wold et al. Citation2001). NIPALS, cross-validation, VIP, the basic MCUVE architecture were garnered from the libPLS package (v1.9, Changsha Nice City, China). BMCUVE and other diagnostic tools were written by the authors.
3. Results and discussion
summarizes TOR OC predictions for the raw, processed, and VCP calibrations as compared to collocated TOR sampling. Despite the fact that the CSN filters contained lower OC areal density than 2011 IMPROVE samples, the FT-IR method was closely reproduced here. Considerable PTFE and ammonium salt absorption prevented identifying the functional groups pertinent to predicting OC using raw spectra Section 3.1).
Table 1. Figures of merit for the FT-IR OC prediction using raw, processed, and VCP test spectra. TOR OC collocated sampling provides a basis for comparison.
Predicting OC using the FT-IR method slightly improved following spectral processing (Section 3.2). Enhancement was attributed to increased signal-to-baseline ratio (SBR) and the removal of redundant absorption from the FT-IR spectra. Processing helped reveal the presence of water vapor in the FT-IR spectra which was removed by QDA-PLS (Section 3.3). Although all three models performed equivalently to TOR sampling (Section 3.4), processing and vapor correcting spectra removed thirty-two PLS components from the calibration. This simplified the model's latent structure considerably. Specifically, the first PLS component in the VCP calibration unambiguously explained TOR OC variability in the spectra with the two remaining components likely modeling small interferences from PTFE and ammonium nitrate (Section 3.5). Organic functional group vibrations were then readily identified and many confidently assigned using VIP-BCI ranking (Section 3.6).
3.1. Prediction of TOR OC using raw spectra
illustrates that the FT-IR method predicts TOR OC with good accuracy and small bias using the raw spectra (see for figures of merit). Thirty-five components were required for calibration. Precision and MDL were comparable to collocated TOR sampling with 3.7% of test samples residing below the FT-IR MDL and 2.7% below the TOR empirical MDL. It was anticipated a priori that collocated TOR sampling would significantly outperform the FT-IR method, with the former considering errors from only one filter type (quartz) and analytical method (TOR). In addition, nine sites were used by the FT-IR method to estimate R2, bias, error, and MAD; whereas, only one site (Boston) was used to estimate TOR metrics (see Section 2.1.2 for details). Taken together, and demonstrate that the FT-IR method does not add significant error to the estimation of OC. Further comparing to illustrates that variation in OC composition may explain the lower R2 observed in FT-IR OC prediction, likely resulting from predicting OC in nine CSN sites (a) as opposed to one (b). While TOR and FT-IR MDL and precision are more comparable, caution is advised when inferring any differences considering that only eleven field blanks and twenty six collocated Boston samples were used in their calculation. Overall, the good precision and accuracy of the FT-IR method in the absence of spectral pretreatment is noteworthy.
Figure 4. TOR measured versus FT-IR predicted OC using raw spectra (a). The 26 collocated Boston samples are plotted (b) to illustrate dispersion attributable to TOR sampling.
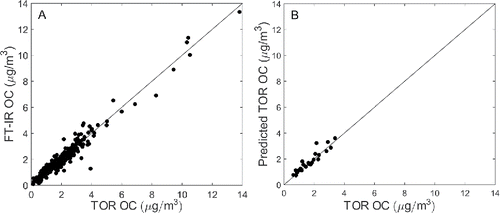
This FT-IR model showed comparable results to Dillner and Takahama Citation(2015a) where TOR OC was determined on IMPROVE PTFE filters. CSN filters contained an estimated ∼6.5 times lower areal density relative to those used in the IMPROVE study (see Section S6 in the SI for details). While only a rough estimate, this value appears more reasonable than what might be expected for collocated samplers (∼11). Furthermore, these results confirm that the considerable PTFE scattering and absorption in raw CSN spectra (e.g., ) does not negatively affect TOR OC quantification.
A clear connection between TOR OC and infrared absorption was anticipated upon examining the first few PLS components. Substantial PTFE interference and, to a lesser extent, interference from inorganic species (e.g., N-H bends from ammonium cations) prevented assigning regression parameters to any particular functional group vibrations. Exploring interferences in with PCA revealed that most of the variability in the raw spectra was explained by a single component (90.3%), unrelated to any particular absorption band. shows that projecting spectra onto the first and third principal components demonstrates no correlation between PC1 and TOR OC, implying that the dominant variations in
are unrelated to the TOR OC response (
). Rather, changes in baseline and PTFE background explain the distribution of FT-IR spectra on PC1, with two spectra illustrating extreme (±) instances of these fluctuations (). The second principal component explained a smaller fraction of total variability in
(6.2%) by mapping fluctuations in PTFE absorption bands and nuanced baseline variation above 1400 cm−1 (see Section S7 in the SI for PC2 interpretation). Taken together, we can infer that approximately 96% of the variability in the raw spectra is related to extinction by the filter substrate.
Figure 5. Calibration spectra projected on the first and third principal components (a). Spectra are pseudo-colored according to the mass of ammonium determined in each sample using ion chromatography (NH4+, μg/m3). Spectra showing extreme behavior on PC1 (vertical dashes, a) are also plotted to confirm that most variability in the raw spectra are related to non-specific substrate extinction (b).
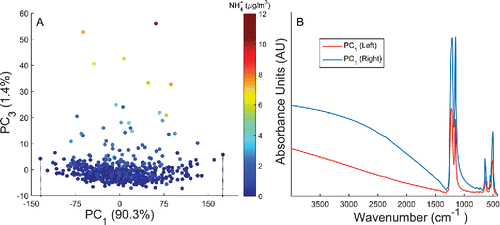
The third principal component (PC3) was neither strongly correlated to TOR OC nor PTFE, explaining 1.4% of the spectral variations. illustrates that most spectra cluster between ±10 standard deviations on PC3 with six samples located much higher than the majority (>30). Pseudo-coloring each spectrum according to their ammonium ion content (NH4+) indicated that PC3 was strongly correlated to the absorption of ammonium salts with the six samples containing an uncharacteristically high ammonium concentration relative to the rest of the samples. This suggested that PC3 was very close to a pure “ammonium” factor in raw calibration spectra. Overall, ∼97% of absorption variations in the raw CSN spectra were not readily linked to changes in TOR OC concentration. This provided strong evidence that most of the thirty-five PLS components were modeling matrix interferences as opposed to TOR OC response.
3.2. Predicting TOR OC from processed spectra
Transforming raw into second derivative spectra and optimizing the calibration using BMCUVE resulted in a parsimonious PLS calibration using only 375 predictors and four components. illustrates an apparent improvement in FT-IR OC prediction according to figures of merit (with the exception of the slight increase in bias). However, only MAD improved significantly after processing (α < 0.05).
illustrates the dual role of derivative transformation and BMCUVE in removing substrate absorption from the PLS problem. First, we see that the characteristically broad baseline present in the raw spectra shows near-zero absorption after transformation. The band-pass characteristics of the second derivative filter ensured this result, although to the detriment of the broad X-H stretches above 3100 cm−1 (Russell et al. Citation2011). Derivative filtering consequently reduced the total infrared signal by many orders of magnitude but lead to the amplification of narrow infrared bands relative to baseline. This increased signal-to-baseline ratio (SBR) was the major reason that many components were removed from the calibration after processing. In fact, derivative transformation alone was responsible for eliminating twenty-nine components from the PLS model (Section S3, Figure S7 in the SI).
Figure 6. Second derivative spectrum of an ambient CSN filter sample. Wavenumbers selected by BMCUVE are denoted (bullets). The spectral range was reduced to better visualize the main analytical region used for TOR OC calibration. Sixty-six wavenumbers used by the calibration below 1350 cm−1 and above 3350 cm−1 are not displayed.
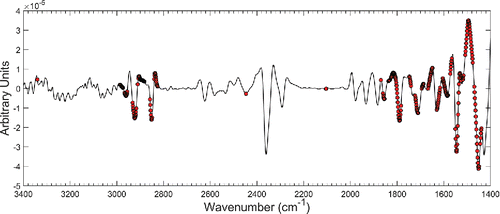
The increase in SBR was quantified using the CO2 anti-symmetric stretch of carbon dioxide at ∼2360 cm−1 (Ingle Jr. and Crouch Citation1988). Numerically integrating this spectral line suggested an average SBR for the second derivative spectra on the order of 101. The raw spectra, on the other hand, showed an average SBR on the order of 10−2. This 1000-fold increase in SBR was therefore strongly associated with reducing PLS model complexity (see Section S8 in the SI for calculation methodology).
Derivative transformation was only the first step in processing FT-IR spectra. BMCUVE fine-tuned the calibration by eliminating wavenumbers corresponding to PTFE and those considered unreliable for OC prediction. Of the 2764 predictors in the derivative spectra (p), only 375 were considered optimal by BMCUVE. In total, derivative transformation was responsible for removing 29 components from the calibration problem with BMCUVE removing two more. Although comprehensive band assignment will follow in Section 3.5, we see in that the wavenumbers selected by BMCUVE were mostly concentrated between 3000–2800 cm−1 and 1850 cm–1400 cm−1, regions known to contain organic functional group vibrations (Shurvell Citation2002). Wavenumbers selected outside these regions were usually needed to account for residual baseline, PTFE, and water vapor contamination in the infrared spectra (e.g., 2500–2000 cm−1, >3500 cm−1).
3.3. Ambient water vapor correction and OC prediction
An exploratory PCA confirmed that processing removed a substantial proportion of PTFE interference from the spectra. Comparing to , we see that absorption variations that dominated the processed spectra were very different from those dominating the raw spectra. Specifically, the first component explains only 26.7% of the spectral variation in the processed FT-IR spectra. Pseudo-coloring samples according to OC mass concentration (μg/m3) shows that PC1 is no longer correlated to PTFE extinction but is now affiliated with OC absorption. A slight misalignment of the OC color map with the PC1 axis illustrates that OC concentration is imperfectly correlated with PC1.
Figure 7. Fresno and Elizabeth spectra projected onto the first and third principal components pseudo-colored according to OC mass concentration (a). A boundary determined from QDA separates the Fresno (upper) and Elizabeth (lower) sites. The lower QDA boundary was used as the dependent variable with PC1 and PC3 scores as independent variables in an auxiliary PLS regression (b). Regression and projection into the PLS scores () isolated water vapor and OC absorption onto distinct components (see Section S2 in the SI for QDA-PLS notation).
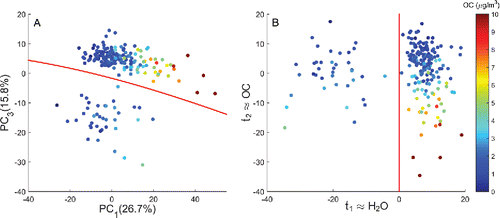
Regression diagnostics revealed that PC2 explained a nearly equal proportion of spectral variation, showing some connection to TOR OC (20.1%; see Section S9 of the SI). In addition, PC3 no longer spanned ammonium absorption indicating that processing mitigated its impact on the calibration. Instead, we found that Elizabeth, NJ samples clustered inordinately low on PC3 relative to the other eight sites. The distribution of Elizabeth samples on PC3 was not connected to OC and EC nor OC/EC and OC/NH4+ but rather spanned the feature-space of water vapor absorption. This suggested that excess water vapor remained in the infrared sampling compartment after purging (Section S9, Figure S20 of the SI). Consequently, Elizabeth samples contained measurably higher vapor interference than spectra from the other sites with the amplification of water vapor vibrations between 4000–3500 cm−1 and 2000–1400 cm−1 an unintended consequence of derivative transformation.
Because many OC functional groups reside between 1850–1400 cm−1, we hypothesized that a direct consequence of water vapor interference was the inclusion of an additional component in the FT-IR model. As demonstrated in the raw FT-IR model, retaining additional components to account for interferences does not necessarily degrade OC prediction. However, removing interference from the FT-IR spectra is preferable in the interest of functional group identification and band assignment. shows that it was possible to confine the water vapor interference to the first QDA-PLS component () with the critically important OC signal remaining intact on the second QDA-PLS scores (
). With water vapor relegated to a single component, the infrared spectral matrices were reconstructed without the component included. PLS calibration using the resulting VCP spectra utilized the same wavenumbers as the processed model () albeit with a revised set of regression coefficients.
showed that three PLS components were required for OC prediction after vapor correction. This provided evidence that water vapor was both a true interference (“factor”) in the processed spectra and subsequently removed from the model by the QDA-PLS routine. More importantly, figures of merit confirmed that the VCP spectra remained viable for OC prediction.
3.4. Uncertainties in figures of merit
Confidence intervals calculated for MAD suggested no true difference between TOR and FT-IR methods, as shown in . Notably, comparing the width of the confidence intervals shows that the spectral processing (including vapor correction) reduces test set uncertainty relative to TOR sampling. The contrary is true for the raw-spectra calibration. We see that the width of the confidence intervals calculated for the raw model is approximately on the order of those calculated from TOR sampling in spite of the former using significantly more test samples (329 vs. 26). Greater uncertainty in the raw FT-IR predictions was due to the loss of statistical power and increased error variance resulting from estimating thirty-five components.
3.5. Latent structure interpretation for the VCP model
shows the first PLS component loadings plotted against wavenumber for the VCP model. Wavenumbers considered “safely interpretable” by VIP-BCI ranking are also indicated. The visual association between the first PLS component and the top-ranked wavenumbers was surprising considering the reported complexity of urban aerosols (Rogge et al. Citation1993; Kroll and Seinfeld Citation2008; Jimenez et al. Citation2009a; Zhang et al. Citation2015), i.e., we assumed that OC absorption would be distributed ambiguously across multiple components thereby complicating model interpretation using simple loading plots. illustrates why visualization was possible: the first PLS component explains 88.1% of the total variance in the TOR response. Specifically, the high percentage of explained y-variance for component 1 controls the calculation of the VIP making the VIP-BCI metric directly proportional to the first normalized loading weights ( in (Equation8
[8] )) and by extension the first PLS loadings. Conceptually, we see that the first PLS component therefore constitutes the only true predictive “OC factor” for this regression problem. The remaining PLS components model a mixture of substrate extinction and ammonium salt interference (see Section S10 in the SI for details).
Figure 9. The first PLS component loadings () plotted against wavenumber for the VCP model (a). The VIP-BCI procedure identified an approximate reliability threshold at ±0.04 with the highest ranked features labeled (bullets). Direct correspondence between
and VIP-BCI reliability is understood by considering that nearly all variation in the TOR OC analyte vector (
) was explained by the first PLS component,
(b).
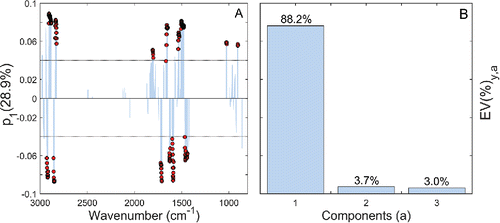
3.6. Wavenumber interpretation for the VCP model
displays band assignments for the VCP model. Confident assignments include the methylene (CH2) symmetric and antisymmetric stretches between 2929–2826 cm−1. Wavenumbers spanning 1723–1710 cm−1—which actually contains a doublet feature centered at 1710 cm−1 and 1720 cm−1—are assigned to multiple carbonyl stretching vibrations from ketones, aldehydes, and/or organic acids (Shurvell Citation2002; Mayo et al. Citation2004). Carbonyl and alkane functional groups have been used to directly estimate OC using the Beer-Lambert law, leaving little doubt as to their efficacy in TOR OC prediction by PLS (Blando et al. Citation2001; Takahama et al. Citation2013; Ruthenburg et al. Citation2014). Other confident assignments include the methyl (CH3) deformations at 1462 cm−1 and methylene (CH2) scissoring mode at ∼1444 cm−1 which, interestingly, were picked out by the VIP-BCI criteria in spite of the positive interference from the broad (and very strong) ammonium deformations at ∼1425 cm−1 (Allen et al. Citation1994; Boer et al. Citation2007).
Figure 10. A blank-corrected second derivative spectrum with the 154 VIP-BCI ranked features labeled.
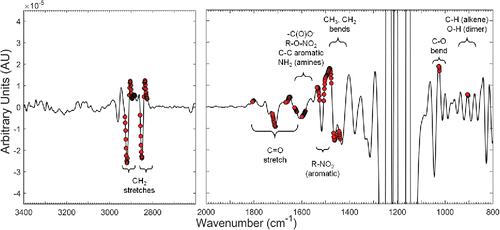
Reference spectra from humic-like substances (HULIS) extracted from ambient fine aerosols closely align with many of the assignments in including the C-H stretches above 2883 cm−1, a characteristically broad carbonyl stretch near 1725 cm−1, C-H deformations centered at 1461 cm−1, and the C=O stretch of conjugated carbonyl moieties at 1631 cm−1 (Havers et al. Citation1998; Zappoli et al. Citation1999; Duarte et al. Citation2007). The C-O functionality of polysaccharides was assigned to ∼1060 cm−1 in the spectra of HULIS with this band resolvable in at ∼1040 cm−1 (Graber and Rudich Citation2006). A general assignment of ether, ester, lactone, and anhydride C-O deformations to the 1027–1023 cm−1 range was made considering its off-center position. Although what is extracted and nominated “HULIS” is protocol-dependent, HULIS extracts are typically free from inorganic species with chemical functionality similar to the water soluble organic carbon (Polidori et al. Citation2008) and can comprise a significant portion of OC mass in ambient fine aerosols (Reemtsma et al. Citation2006; Duarte et al. Citation2007; Jimenez et al. Citation2009b). In this way, the good match between the major functional groups from HULIS and those wavenumbers identified by VIP-BCI confirms that the VCP calibration is mostly using organic functional groups for the determination of TOR OC in CSN samples.
Multiple assignments between the narrow 1631–1620 cm−1 region are possible including the aforementioned C=O stretch of conjugated species, the antisymmetric NO2 stretch of organonitrates, and NH2 deformations from primary amines (Shurvell Citation2002; Presto et al. Citation2005; Liu et al. Citation2012). The H-O-H deformations from “particulate water strongly bound to salt hydrates” has also been shown to absorb here in ambient fine aerosols (Allen et al. Citation1994). If present, this vibration is likely a very minor contributor to the absorption in this region due to the dry condition of the CSN filters and theoretically weak H-O-H bends of hydrated salts (Miller and Wilkins Citation1952).
Plausible assignments include C=C olefinic and amide C=O stretches to 1668–1648 cm−1 (Fiegland et al. Citation2005). A triple assignment of NH2 deformations from primary amines, carboxylate antisymmetric stretches (−C(O)O−), and possibly C-C aromatic stretches are all reasonable assignments between 1601–1585 cm−1 (Shurvell Citation2002; Hay and Myneni Citation2007). The 906–904 cm−1 range can be assigned to the very infrared active C-H out-of-plane bending vibrations of vinyl and vinylidene groups centered at ∼910 cm−1 and ∼890 cm−1, respectively (Mayo et al. Citation2004). This provides secondary support for the presence and usefulness of olefin functional groups for OC determination. The considerable abundance of carboxylic acids in fine aerosols also supports an assignment of O-H “dimer bends” to the same wavenumber range.
Less certain assignments include the N=O antisymmetric stretch from nitroaromatic compounds centered near 1531 cm−1, the assignment of lactones and anhydrides (C=O) at ∼1805 cm−1, and most assignments corresponding to the large range spanning 1508–1476 cm−1 (not labeled). Further discussion of these vibrations, including their VIP scores, can be found in Section S12 of the SI.
4. Conclusions
Urban fine aerosols were collected on PTFE filters in the U. S. Environmental Protection Agencies' Chemical Speciation Network (CSN) and analyzed by inexpensive and nondestructive FT-IR spectroscopy. The multivariate PLS determination of TOR OC was first validated by reproducing Dillner and Takahama's Citation(2015a) IMPROVE protocol. In spite of containing much lower aerosol infrared absorption and substantially greater interference from the PTFE filters (), TOR OC was determined using raw (untreated) FT-IR spectra with good accuracy, precision, and low bias. The FT-IR method is therefore extendible to TOR OC determination in the CSN urban sampling network.
The raw FT-IR calibration exhibited significant complexity preventing a clear assessment of the functional groups used for OC prediction. To address this, raw spectra were subjected to two processing regimes in the interest of simplifying the calibration and elucidating the OC functional groups used for calibration. The first step in both processing protocols involved transforming absorption into second derivative spectra. Derivative spectra showed a dramatic 1000-fold increase in signal-to-baseline ratio after transformation, a result of suppressing most PTFE scattering above 1400 cm−1. Derivative transformation eliminated twenty-nine PLS components from the calibration, proving to be the most crucial step in reducing PLS model complexity.
The second step in processing used backward Monte Carlo unimportant variable elimination (BMCUVE) to refine the calibration. Two additional PLS components were removed after BMCUVE with only 375 of the original 2784 variables needed for OC prediction. Calibrations developed from these “processed” spectra showed a statistically significant improvement in average OC prediction according to median absolute deviation (MAD).
Processing helped reveal otherwise undetectable water vapor interference in the processed spectra on principal component plots. Vapor correction using the first and third principal components in a combined quadratic discriminant analysis and PLS routine removed vapor interference at no cost to prediction. Most importantly, this final “vapor corrected and processed” (VCP) model contained only three components, which were meaningfully interpreted in terms of OC functional group vibrations.
The VCP model relied chiefly on the first PLS component for prediction implying that the infrared absorption of OC in ambient fine aerosols may exhibit predictable behavior in geographically and compositionally diverse urban samples. Specifically, the first component singularly correlated OC absorption to the TOR OC response, with PLS parameters describing some mixture-average of OC functional group vibrations. The remaining two supplemental components showed little correlation to TOR OC, adjusting regression coefficients to likely account for PTFE and ammonium salt absorption in the FT-IR spectra. A variable ranking procedure (VIP-BCI) confirmed the factor interpretation indicating aliphatic C-H stretches and bends, a diverse array of C = O stretches, vibrations corresponding to carboxylic acids (e.g., carboxylate anions, dimers), olefinic C = C vibrations, organonitrate vibrations, as well as amines and aromatic ring stretches as germane for prediction. Overall, the systematic treatment of CSN spectra provided a straightforward assessment of functional group absorption used by PLS regression to predict OC in urban CSN samples.
UAST_1217389_Supplementary_file.zip
Download Zip (1.2 MB)Acknowledgments
Thanks to S.M. Raffuse for the map of the CSN sites and to the RTI International team for managing CSN.
Funding
The authors acknowledge funding from U.S. EPA and the IMPROVE program (National Park Service cooperative agreement P11AC91045) and EPFL funding.
References
- Abdi, H., and Williams, L. J. (2010). Principal Component Analysis. Wiley Interdisciplinary Rev.: Comput. Statist., 2:433–459.
- Allen, D. T., Palen, E. J., Haimov, M. I., Hering, S. V., and Young, J. R. (1994). Fourier Transform Infrared Spectroscopy of Aerosol Collected in a Low Pressure Impactor (LPI/FTIR): Method Development and Field Calibration. Aerosol Sci. Technol., 21:325–342.
- Baumann, K., Jayanty, R. K. M., and Flanagan, J. B. (2008). Fine Particulate Matter Source Apportionment for the Chemical Speciation Trends Network Site at Birmingham, Alabama, Using Positive Matrix Factorization. J. Air Waste Manag. Assoc., 58:27–44.
- Blando, J. D., Porcja, R. J., and Turpin, B. J. (2001). Issues in the Quantitation of Functional Groups by FTIR Spectroscopic Analysis of Impactor-Collected Aerosol Samples. Aerosol Sci. Technol., 35:899–908.
- Bocklitz, T., Walter, A., Hartmann, K., Rosch, P., and Popp, J. (2011). How to Pre-Process Raman Spectra for Reliable and Stable Models? Anal. Chim. Acta, 704:47–56.
- Boer, G. J., Sokolik, I. N., and Martin, S. T. (2007). Infrared Optical Constants of Aqueous Sulfate–Nitrate–Ammonium Multi-Component Tropospheric Aerosols from Attenuated Total Reflectance Measurements—Part I: Results and Analysis of Spectral Absorbing Features. J. Quant. Spectrosc. Radiat. Transfer, 108:17–38.
- Cai, W., Li, Y., and Shao, X. (2008). A Variable Selection Method Based on Uninformative Variable Elimination for Multivariate Calibration of Near-Infrared Spectra. Chemom. Intell. Lab. Syst. 90:188–194.
- Carlton, A. G., Turpin, B. J., Altieri, K. E., Seitzinger, S. P., Mathur, R., Roselle, S. J., and Weber, R. J. (2008). CMAQ Model Performance Enhanced When in-Cloud Secondary Organic Aerosol is Included: Comparisons of Organic Carbon Predictions with Measurements. Environ. Sci. Technol., 42:8798–8802.
- Centner, V., Massart, D.-L., de Noord, O. E., de Jong, S., Vandeginste, B. M., and Sterna, C. (1996). Elimination of Uninformative Variables for Multivariate Calibration. Anal. Chem., 68:3851–3858.
- Chen, Z.-P., Li, L.-M., Yu, R.-Q., Littlejohn, D., Nordon, A., Morris, J., Dann, A. S., Jeffkins, P. A., Richardson, M. D., and Stimpson, S. L. (2011). Systematic Prediction Error Correction: A Novel Strategy for Maintaining the Predictive Abilities of Multivariate Calibration Models. Analyst, 136:98–106.
- Chen, L.-W. A., Watson, J. G., Chow, J. C., DuBois, D. W., and Herschberger, L. (2010). Chemical Mass Balance Source Apportionment for Combined PM 2.5 Measurements from US Non-Urban and Urban Long-Term Networks. Atmos. Environ., 44:4908–4918.
- Chong, I.-G., and Jun, C.-H. (2005a). Performance of Some Variable Selection Methods When Multicollinearity is Present. Chem. Intel. Laboratory Syst., 78:103–112.
- Chong, I.-G., and Jun, C.-H. (2005b). Performance of Some Variable Selection Methods When Multicollinearity is Present. Chemometr. Intelligent Laboratory Syst., 78:103–112.
- Chow, J., Lowenthal, D., Chen, L. W. A., Wang, X., and Watson, J. (2015). Mass Reconstruction Methods for PM2.5: A Review. Air Qual. Atmos. Health, 8:243–263.
- Chow, J. C., Watson, J. G., Chen, L.-W. A., Chang, M. O., Robinson, N. F., Trimble, D., and Kohl, S. (2007). The IMPROVE_A Temperature Protocol for Thermal/Optical Carbon Analysis: Maintaining Consistency with a Long-Term Database. J. Air Waste Manag. Assoc., 57:1014–1023.
- Chow, J. C., Watson, J. G., Pritchett, L. C., Pierson, W. R., Frazier, C. A., and Purcell, R. G. (1993). The DRI Thermal/Optical Reflectance Carbon Analysis System: Description, Evaluation and Applications in US Air Quality Studies. Atmos. Environ. Part A. General Topics, 27:1185–1201.
- Cowe, I. A., and McNicol, J. W. (1985). The Use of Principal Components in the Analysis of Near-Infrared Spectra. Appl. Spectrosc., 39:257–266.
- Dillner, A. M., Phuah, C. H., and Turner, J. R. (2009). Effects of Post-Sampling Conditions on Ambient Carbon Aerosol Filter Measurements. Atmos. Environ., 43:5937–5943.
- Dillner, A. M., and Takahama, S. (2015a). Predicting Ambient Aerosol Thermal-Optical Reflectance (TOR) Measurements from Infrared Spectra: Organic Carbon. Atmos. Measur. Tech., 8:1097–1109.
- Dillner, A. M., and Takahama, S. (2015b). Predicting Ambient Aerosol Thermal-Optical Reflectance Measurements from Infrared Spectra: Elemental Carbon. Atmos. Measur. Techn., 8:4013–4023.
- Dixon, S. J., and Brereton, R. G. (2009). Comparison of Performance of Five Common Classifiers Represented as Boundary Methods: Euclidean Distance to Centroids, Linear Discriminant Analysis, Quadratic Discriminant Analysis, Learning Vector Quantization and Support Vector Machines, as Dependent on Data Structure. Chemometr. Intell. Lab. Syst., 95:1–17.
- Duarte, R. M. B. O., Santos, E. B. H., Pio, C. A., and Duarte, A. C. (2007). Comparison of Structural Features of Water-Soluble Organic Matter from Atmospheric Aerosols with Those of Aquatic Humic Substances. Atmos. Environ., 41:8100–8113.
- Dubovik, O., Holben, B., Eck, T. F., Smirnov, A., Kaufman, Y. J., King, M. D., Tanré, D., and Slutsker, I. (2002). Variability of Absorption and Optical Properties of Key Aerosol Types Observed in Worldwide Locations. J. Atmos. Sci., 59:590–608.
- Efron, B., and Tibshirani, R. (1993). An Introduction to the Bootstrap. Chapman & Hall, Boca Raton, Florida.
- Feudale, R. N., Woody, N. A., Tan, H., Myles, A. J., Brown, S. D., and Ferré, J. (2002). Transfer of Multivariate Calibration Models: A Review. Chemometr. Intell. Lab. Syst., 64:181–192.
- Fiegland, L. R., McCorn Saint Fleur, M., and Morris, J. R. (2005). Reactions of C C-Terminated Self-Assembled Monolayers with Gas-Phase Ozone. Langmuir, 21:2660–2661.
- Gardner, M. J., and Altman, D. G. (1986). Confidence Intervals Rather Than P Values: Estimation Rather Than Hypothesis Testing. BMJ, 292:746–750.
- Geladi, P., and Kowalski, B. R. (1986). Partial Least-Squares Regression: A Tutorial. Anal. Chim. Acta, 185:1–17.
- George, K. M., Ruthenburg, T. C., Smith, J., Yu, L., Zhang, Q., Anastasio, C., and Dillner, A. M. (2015). FT-IR Quantification of the Carbonyl Functional Group in Aqueous-Phase Secondary Organic Aerosol from Phenols. Atmos. Environ., 100:230–237.
- Graber, E., and Rudich, Y. (2006). Atmospheric HULIS: How Humic-Like are They? A Comprehensive and Critical Review. Atmos. Chem. Phys., 6:729–753.
- Griffiths, P. R., and De Haseth, J. A. (2007). Fourier Transform Infrared Spectrometry. John Wiley & Sons, Hoboken, New Jersey.
- Havers, N., Burba, P., Lambert, J., and Klockow, D. (1998). Spectroscopic Characterization of Humic-Like Substances in Airborne Particulate Matter. J. Atmos. Chem., 29:45–54.
- Hay, M. B., and Myneni, S. C. (2007). Structural Environments of Carboxyl Groups in Natural Organic Molecules from Terrestrial Systems. Part 1: Infrared Spectroscopy. Geochim. Cosmochim. Acta, 71:3518–3532.
- Helland, I. S. (1988). On the Structure of Partial Least Squares Regression. Commun. Stat. Simulat. Comput., 17:581–607.
- Ingle Jr, J. D., and Crouch, S. R. (1988). Spectrochemical Analysis. Prentice Hall College Book Division, Old Tappan, New Jersey.
- Isaksson, T., and Næs, T. (1988). The Effect of Multiplicative Scatter Correction (MSC) and Linearity Improvement in NIR Spectroscopy. Appl. Spectrosc., 42:1273–1284.
- Jimenez, J., Canagaratna, M., Donahue, N., Prevot, A., Zhang, Q., Kroll, J., DeCarlo, P., Allan, J., Coe, H., and Ng, N. (2009a). Evolution of Organic Aerosols in the Atmosphere. Science, 326:1525–1529.
- Jimenez, J. L., Canagaratna, M. R., Donahue, N. M., Prevot, A. S. H., Zhang, Q., Kroll, J. H., DeCarlo, P. F., Allan, J. D., Coe, H., Ng, N. L., Aiken, A. C., Docherty, K. S., Ulbrich, I. M., Grieshop, A. P., Robinson, A. L., Duplissy, J., Smith, J. D., Wilson, K. R., Lanz, V. A., Hueglin, C., Sun, Y. L., Tian, J., Laaksonen, A., Raatikainen, T., Rautiainen, J., Vaattovaara, P., Ehn, M., Kulmala, M., Tomlinson, J. M., Collins, D. R., Cubison, M. J., Dunlea, J., Huffman, J. A., Onasch, T. B., Alfarra, M. R., Williams, P. I., Bower, K., Kondo, Y., Schneider, J., Drewnick, F., Borrmann, S., Weimer, S., Demerjian, K., Salcedo, D., Cottrell, L., Griffin, R., Takami, A., Miyoshi, T., Hatakeyama, S., Shimono, A., Sun, J. Y., Zhang, Y. M., Dzepina, K., Kimmel, J. R., Sueper, D., Jayne, J. T., Herndon, S. C., Trimborn, A. M., Williams, L. R., Wood, E. C., Middlebrook, A. M., Kolb, C. E., Baltensperger, U., and Worsnop, D. R. (2009b). Evolution of Organic Aerosols in the Atmosphere. Science, 326:1525–1529.
- Kim, E., Hopke, P. K., and Edgerton, E. S. (2003). Source Identification of Atlanta Aerosol by Positive Matrix Factorization. J. Air Waste Manag. Assoc., 53:731–739.
- Kroll, J. H., and Seinfeld, J. H. (2008). Chemistry of Secondary Organic Aerosol: Formation and Evolution of Low-Volatility Organics in the Atmosphere. Atmos. Environ., 42:3593–3624.
- Krzanowski, W. J. (1983). Cross-Validatory Choice in Principal Component Analysis; Some Sampling Results. J. Statist. Comput. Simulat., 18:299–314.
- Krämer, N., and Sugiyama, M. (2011). The Degrees of Freedom of Partial Least Squares Regression. J. Amer. Statist. Assoc., 106:697–705.
- Lee, E., Chan, C. K., and Paatero, P. (1999). Application of Positive Matrix Factorization in Source Apportionment of Particulate Pollutants in Hong Kong. Atmos. Environ., 33:3201–3212.
- Liang, C., and Krimm, S. (1956). Infrared Spectra of High Polymers. III. Polytetrafluoroethylene and Polychlorotrifluoroethylene. J. Chem. Phys., 25:563–571.
- Li, B., Morris, J., and Martin, E. B. (2002). Model Selection for Partial Least Squares Regression. Chemometr. Intell. Lab. Syst., 64:79–89.
- Liu, S., Shilling, J. E., Song, C., Hiranuma, N., Zaveri, R. A., and Russell, L. M. (2012). Hydrolysis of Organonitrate Functional Groups in Aerosol Particles. Aerosol Sci. Technol., 46:1359–1369.
- Malm, W. C., and Hand, J. L. (2007). An Examination of the Physical and Optical Properties of Aerosols Collected in the IMPROVE Program. Atmos. Environ., 41:3407–3427.
- Malm, W. C., Schichtel, B. A., and Pitchford, M. L. (2011). Uncertainties in PM2.5 Gravimetric and Speciation Measurements and What We Can Learn from Them. J. Air Waste Manag. Assoc., 61:1131–1149.
- Maria, S. F., Russell, L. M., Turpin, B. J., and Porcja, R. J. (2002). FTIR Measurements of Functional Groups and Organic Mass in Aerosol Samples Over the Caribbean. Atmos. Environ., 36:5185–5196.
- Mayo, D. W., Miller, F. A., and Hannah, R. W. (2004). Course Notes on the Interpretation of Infrared and Raman Spectra. John Wiley & Sons, Hoboken, New Jersey.
- McDade, C. E., Dillner, A. M., and Indresand, H. (2009). Particulate Matter Sample Deposit Geometry and Effective Filter Face Velocities. J. Air Waste Manag. Assoc., 59:1045–1048.
- McDow, S. R., and Huntzicker, J. J. (1990). Vapor Adsorption Artifact in the Sampling of Organic Aerosol: Face Velocity Effects. Atmos. Environ. Part A. General Topics, 24:2563–2571.
- Mehmood, T., Liland, K. H., Snipen, L., and Sæbø, S. (2012). A Review of Variable Selection Methods in Partial Least Squares Regression. Chemometr. Intell. Lab. Syst., 118:62–69.
- Miller, F. A., and Wilkins, C. H. (1952). Infrared Spectra and Characteristic Frequencies of Inorganic Ions. Anal. Chem., 24:1253–1294.
- Nieuwoudt, H. H., Prior, B. A., Pretorius, I. S., Manley, M., and Bauer, F. F. (2004). Principal Component Analysis Applied to Fourier Transform Infrared Spectroscopy for the Design of Calibration Sets for Glycerol Prediction Models in Wine and for the Detection and Classification of Outlier Samples. J. Agric. Food. Chem., 52:3726–3735.
- Næs, T., Isaksson, T., Fearn, T., and Davies, T. (2002). An User-friendly Guide to Multivariate Calibration and Classification. Nir Publications, Chichester, West Sussex.
- Polidori, A., Turpin, B. J., Davidson, C. I., Rodenburg, L. A., and Maimone, F. (2008). Organic PM 2.5: Fractionation by Polarity, FTIR Spectroscopy, and OM/OC Ratio for the Pittsburgh Aerosol. Aerosol Sci. Technol., 42:233–246.
- Pope III, C. A., and Dockery, D. W. (2006). Health Effects of Fine Particulate Air Pollution: Lines That Connect. J. Air Waste Manag. Assoc., 56:709–742.
- Presser, C., Conny, J. M., and Nazarian, A. (2014). Filter Material Effects on Particle Absorption Optical Properties. Aerosol Sci. Technol., 48:515–529.
- Presto, A. A., Huff Hartz, K. E., and Donahue, N. M. (2005). Secondary Organic Aerosol Production from Terpene Ozonolysis. 2. Effect of NO x Concentration. Environ. Sci. Technol., 39:7046–7054.
- Pöschl, U. (2005). Atmospheric Aerosols: Composition, Transformation, Climate and Health Effects. Angew. Chem. Int. Ed., 44:7520–7540.
- Quarti, C., Milani, A., and Castiglioni, C. (2013). Ab Initio Calculation of the IR Spectrum of PTFE: Helical Symmetry and Defects. J. Phys. Chem. B, 117:706–718.
- Rattigan, O. V., Felton, H. D., Bae, M.-S., Schwab, J. J., and Demerjian, K. L. (2011). Comparison of Long-Term PM 2.5 Carbon Measurements at an Urban and Rural Location in New York. Atmos. Environ., 45:3228–3236.
- Reemtsma, T., These, A., Venkatachari, P., Xia, X., Hopke, P. K., Springer, A., and Linscheid, M. (2006). Identification of Fulvic Acids and Sulfated and Nitrated Analogues in Atmospheric Aerosol by Electrospray Ionization Fourier Transform Ion Cyclotron Resonance Mass Spectrometry. Anal. Chem., 78:8299–8304.
- Reggente, M., Dillner, A. M., and Takahama, S. (2015). Predicting Ambient Aerosol Thermal-Optical Reflectance (TOR) Measurements from Infrared Spectra: Extending the Predictions to Different Years and Different Sites. Atmos. Measur. Techn. Discuss., 8:12433–12474.
- Rogge, W. F., Mazurek, M. A., Hildemann, L. M., Cass, G. R., and Simoneit, B. R. T. (1993). Quantification of Urban Organic Aerosols at a Molecular Level: Identification, Abundance and Seasonal Variation. Atmos. Environ. Part A. General Topics, 27:1309–1330.
- Rosipal, R., and Krämer, N. (2006). Overview and Recent Advances in Partial Least Squares, in Subspace, Latent Structure and Feature Selection, Springer, Berlin, Heidelberg, 34–51.
- Russell, L. M. (2003). Aerosol Organic-Mass-to-Organic-Carbon Ratio Measurements. Environ. Sci. Technol., 37:2982–2987.
- Russell, L. M., Bahadur, R., and Ziemann, P. J. (2011). Identifying Organic Aerosol Sources by Comparing Functional Group Composition in Chamber and Atmospheric Particles. Proc. Natl. Acad. Sci. U.S.A., 108:3516–3521.
- Ruthenburg, T. C., Perlin, P. C., Liu, V., McDade, C. E., and Dillner, A. M. (2014). Determination of Organic Matter and Organic Matter to Organic Carbon Ratios by Infrared Spectroscopy with Application to Selected Sites in the IMPROVE Network. Atmos. Environ., 86:47–57.
- Saeys, Y., Inza, I., and Larrañaga, P. (2007). A Review of Feature Selection Techniques in Bioinformatics. Bioinformatics, 23:2507–2517.
- Savitzky, A., and Golay, M. J. E. (1964). Smoothing and Differentiation of Data by Simplified Least Squares Procedures. Anal. Chem., 36:1627–1639.
- Shurvell, H. (2002). Spectra–Structure Correlations in the Mid-and Far-Infrared. Handbook of Vibrational Spectroscopy, 3:1783–1816.
- Solomon, P. A., Crumpler, D., Flanagan, J. B., Jayanty, R. K. M., Rickman, E. E., and McDade, C. E. (2014). U.S. National PM2.5 Chemical Speciation Monitoring Networks—CSN and IMPROVE: Description of networks. J. Air Waste Manag. Assoc., 64:1410–1438.
- Starkweather Jr, H. W., Ferguson, R. C., Chase, D. B., and Minor, J. M. (1985). Infrared Spectra of Amorphous and Crystalline Poly (tetrafluoroethylene). Macromolecules, 18:1684–1686.
- Takahama, S., Johnson, A., and Russell, L. M. (2013). Quantification of Carboxylic and Carbonyl Functional Groups in Organic Aerosol Infrared Absorbance Spectra. Aerosol Sci. Technol., 47:310–325.
- Takahama, S., Ruggeri, G., and Dillner, A. M. (2016). Analysis of Functional Groups in Atmospheric Aerosols by Infrared Spectroscopy: Sparse Methods for Statistical Selection of Relevant Absorption Bands. Atmos. Meas. Tech. Discuss., 2016:1–42.
- Trygg, J., and Wold, S. (2002). Orthogonal Projections to Latent Structures (O-PLS). J. Chemom., 16:119–128.
- Turpin, B. J., Saxena, P., and Andrews, E. (2000). Measuring and Simulating Particulate Organics in the Atmosphere: Problems and Prospects. Atmos. Environ., 34:2983–3013.
- Watson, J. G. (2002). Visibility: Science and Regulation. J. Air Waste Manag. Assoc., 52:628–713.
- Weakley, A., Miller, A., Griffiths, P., and Bayman, S. (2014). Quantifying Silica in Filter-Deposited Mine Dusts using Infrared Spectra and Partial Least Squares Regression. Anal. Bioanal. Chem., 406:4715–4724.
- Weakley, A. T., Warwick, P. C., Bitterwolf, T. E., and Aston, D. E. (2012). Multivariate Analysis of Micro-Raman Spectra of Thermoplastic Polyurethane Blends using Principal Component Analysis and Principal Component Regression. Appl. Spectrosc., 66:1269–1278.
- Williams, M. N., Grajales, C. A. G., and Kurkiewicz, D. (2013). Assumptions of Multiple Regression: Correcting Two Misconceptions. Pract. Assessment, Res. Evaluat.on, 18:2.
- Wold, S., Martens, H., and Wold, H. (1983). The Multivariate Calibration Problem in Chemistry Solved by the PLS Method, in Matrix Pencils, B. Kågström and A. Ruhe, eds., Springer, Berlin Heidelberg, pp. 286–293.
- Wold, S., Sjöström, M., and Eriksson, L. (2001). PLS-Regression: A Basic Tool of Chemometrics. Chemometr. Intell. Lab. Syst., 58:109–130.
- Yamamoto, H., Yamaji, H., Abe, Y., Harada, K., Waluyo, D., Fukusaki, E., Kondo, A., Ohno, H., and Fukuda, H. (2009). Dimensionality Reduction for Metabolome Data using PCA, PLS, OPLS, and RFDA with Differential Penalties to Latent Variables. Chemometr. Intell. Lab. Syst., 98:136–142.
- Zappoli, S., Andracchio, A., Fuzzi, S., Facchini, M., Gelencser, A., Kiss, G., Krivacsy, Z., Molnar, A., Meszaros, E., and Hansson, H.-C. (1999). Inorganic, Organic and Macromolecular Components of Fine Aerosol in Different Areas of Europe in Relation to Their Water Solubility. Atmos. Environ., 33:2733–2743.
- Zhang, L., and Garcia-Munoz, S. (2009). A Comparison of Different Methods to Estimate Prediction Uncertainty using Partial Least Squares (PLS): A practitioner's Perspective. Chemometr. Intell. Lab. Syst., 97:152–158.
- Zhang, R., Wang, G., Guo, S., Zamora, M. L., Ying, Q., Lin, Y., Wang, W., Hu, M., and Wang, Y. (2015). Formation of Urban Fine Particulate Matter. Chem. Rev., 115:3803–3855.