ABSTRACT
In this article, we address a fundamental question regarding computational fluid dynamics (CFD) modeling within lung airways: does the inhaled volume during imaging have a significant effect on CFD computations of aerosol deposition? High resolution computed tomography (HRCT) images taken at mean lung volume (MLV) and at total lung capacity (TLC) obtained as part of a previous study of ventilation and aerosol deposition using positron emission tomography (PET) in challenged asthmatics were utilized to construct two airway models for each subject, and the differences in CFD calculated deposition metrics were subsequently quantified. These models included all the airway generations that could be rendered from the HRCT images. CFD calculations for three inhalation flow rates and four monodisperse aerosol sizes used images at MLV and at TLC from 24 volunteer subjects. Both large scale and detailed measures of particle deposition distribution were used in the analysis. The influence of lung volume during imaging is to increase airway dimensions in realistic models and thus reduce flow velocity and deposition due to impaction in the upper airways as calculated by CFD. However, large-scale deposition measures are confounded when the TLC models include deeper generations in the lung that increase the total airway deposition. These trends are modulated by the flow and particle characteristics of the aerosol, making consistent quantifiable differences between MLV and TLC difficult to predict unless both models consider the same anatomical airways.
© 2017 American Association for Aerosol Research
EDITOR:
Introduction
Computational fluid dynamics (CFD) advances over the last twenty years, in particular the availability of powerful commercial packages, have spurred the study of aerosol dosimetry using a technique in which aerosol transport and deposition are calculated in realistic three dimensional (3D) models of the respiratory tract (Longest and Holbrook Citation2012). For example, a very limited survey of the range of published studies includes an early paper on the effects of laryngeal position on deposition in the trachea (Katz et al. Citation1999), a study of models to simulate complex laminar-turbulent transitional flows (Stapleton et al. Citation2000), a study of deposition in central airway bifurcations (Balásházy et al. Citation2003), a computational model of airflow in the upper 17 generations of the human respiratory tract (Gemci et al. Citation2008), a simulation using CFD compared to analytical models of particle deposition in a 16-generation airway model (Zhang et al. Citation2009), the evaluation of an inhaler using a concurrent CFD and in vitro approach (Longest and Hindle Citation2009), the validation of CFD models with in vivo measurements (De Backer et al. Citation2010), a study to provide the computational and experimental foundation for developing 3D/CFD extended airway models in laboratory animals and humans (Corley et al. Citation2012), CFD incorporated into a multiscale modeling approach (Lin et al. Citation2013), an investigation of flow patterns in the upper human airway including oral and nasal inhalation by experimental measurements and CFD (Phuong and Ito Citation2015), and a simulation of the phase change and deposition of inhaled semi-volatile liquid droplets in the nasal passages of rats and humans (Schroeter et al. Citation2016).
In spite of this progress there are several fundamental challenges remaining for the utilization of CFD in realistic airway models obtained from imaging. For example, in general, inhalation therapy takes places when the patient is sitting or standing erect, while virtually all lung imaging is performed with the patient lying supine or prone (see Chan et al. Citation2014 for a rare exception). It has been documented that lung volumes (Ibanez and Raurich Citation1982) and potentially airway geometries, ventilation and aerosol deposition are affected by body position (Baskin et al. Citation1990; Grönkvist et al. Citation2002; Petersson et al. Citation2007; Chan et al. Citation2014). Thus, it is still to be determined if and when this uncertainty in morphology will significantly affect CFD modeling results (Friedman et al. Citation2011). Another challenge arises from the fact that airway morphology is not static during the breathing cycle. For example, glottal motion during inhalation using nasofibroscopy indicates the potential for significant effects on flow resistance and deposition that could not be simulated using a static airway model (Scheinherr et al. Citation2015).
In this article, we address whether the volume of the lungs during HRCT image acquisition can have significant effects on CFD computations of aerosol deposition derived for those images. It is inherent, if not explicitly stated, in the vast majority of CFD studies that the static airway model is representative of a particular lung volume. For example, the well-known Weibel lung model was based on measurements of a single adult lung cast estimated to be ¾ total lung capacity (TLC) following a complex procedure and calculation (Weibel Citation1963). Herein we are able to utilize high resolution computed tomography (HRCT) images taken at mean breathing lung volume (MLV) and at TLC, taking advantage of a previous study of ventilation and aerosol deposition using positron emission tomography (PET) in challenged asthmatics (Greenblatt et al. Citation2015a,Citationb, Citation2016). Two airway models were constructed for each subject, and the differences in CFD calculated deposition metrics could be quantified. The goal was to provide guidance on the uncertainty arising due to lung volume in CFD studies using realistic airway models.
Methods
The Methods cover three general subdivisions: the HRCT image acquisition from the previous study, the CFD simulations, and the data analysis.
HRCT acquisition
HRCT acquisition has been described previously (Greenblatt et al. Citation2015a). The imaging protocol was completed at Massachusetts General Hospital with IRB approval (Application No. 2007P000493). HRCT imaging data at two lung volumes was available from 24 volunteers with mild intermittent or mild persistent asthma as defined by the NIH Global Initiative for Asthma (Bateman et al. Citation2008). During an initial screening visit the concentration of methacholine (MCh) required to cause a 20% drop in the subject's forced expiratory volume in 1 second (FEV1) was determined; that is the PC20 dose. On a second visit the PC20 was administered with five deep breaths of MCh aerosolized by a DeVilbiss nebulizer and Rosenthal dosimeter (model 646, DeVilbiss Healthcare, Somerset, PA, USA) with the subject in the supine position in the PET-CT camera. HRCT images obtained at MLV approximately 5 min after bronchoconstriction and at TLC approximately 30 min after the MCh challenge are used herein. To monitor lung volume and guide its value during imaging, a real time trace of instantaneous volume was obtained with an inductance plethysmograph (Somno Star PT, Sensor Medics Corp, Yorba Linda, CA, USA) and presented to the subject through video goggles. When imaging the lung at MLV with HRCT, the subject was instructed to stop breathing and hold his/her breath when the trace reached a line defining their MLV (previously determined during a 30-s window of spontaneous breathing before the scan).
Full chest HRCT scans were acquired in the supine position during a breath hold (12 sec) at the specified lung volume (MLV or TLC). The scanner was used in a helical mode to acquire 64 slices per rotation with 0.6 mm collimation, and a pitch of 1 mm. The energy settings were 120 kV peak, and 80 mA. Image reconstruction was done using the B31F kernel with a 0.75 mm slice thickness (0.5 mm slice increment and 0.25 mm overlap). Reconstruction of the image with a reduced field of view resulted in typical voxel dimensions of 0.6 × 0.6 × 0.5 mm.
Computational fluid dynamics
The first step in creating a CFD model using a realistic airway model was segmentation of the 64 HRCT image slices into a 3D closed-surface tube system within which the gas flows and on which the aerosol particles deposit. The software used for this process was Pulmonary Workstation 2 (PW2) (VIDA Diagnostics, Coralville, IA, USA) as described in a previous paper (Montesantos et al. Citation2013). Preparation of the geometry for meshing that included smoothing, visual examination for “holes,” the creation and definition of orthogonal entrances and exits, and conversion into CFD ready format (stereolithic, stl) was performed using the open source software Blender v2.5 (Blender Foundation, Amsterdam, The Netherlands). If the volume of the model changed during the smoothing process, it was rescaled manually to match the original geometry.
The realistic airway geometries were then meshed using FLUENT MESHING (ANSYS, Canonsburg, PA, USA), and the flow was simulated using the finite volume solver FLUENT (ANSYS, USA). A hybrid mesh with prism wall layers transitioned to core pyramid cells was used. Within FLUENT, second-order-accurate discretization schemes were used for all terms. Pressure-velocity coupling was achieved using the SIMPLE algorithm, and the transitional k-kl-ω model, a three-equation eddy-viscosity model for laminar and turbulent kinetic energies (k and kl, respectively), as well as an inverse turbulent time scale (ω), was incorporated. The model is based on two transport equations, one for intermittency and one for the transition onset criteria in terms of momentum thickness Reynolds number. The transport equations are intended for the implementation of correlation-based models into general-purpose CFD methods (Menter et al. Citation2006). The theoretical framework and correlation constants for the turbulence model are beyond the scope of this article but are provided in the FLUENT Theory Guide (ANSYS Citation2011). This model has been found to be effective in low Reynolds number transitional flows (Zhang and Kleinstreuer Citation2003; Longest and Vinchurkar Citation2007), including the predominantly laminar cases. Gravity was oriented to assume the erect position. The boundary conditions included no-slip and no-penetration at the walls, a prescribed steady uniform inlet velocity at the trachea, and exits at uniform pressure with classic outflow conditions on downstream derivatives.
The convergence criteria were based on the volume flow rate in the main bronchi and the five lobar flow rates. The simulation was considered converged when the variation of each of these seven monitored values fell below 0.5% over 1000 iterations following methodology described by Van Ertbruggen et al. Citation(2005).
A mesh refinement study found that flow variables and deposition results were converged with cell volumes of ∼0.6 mm. The number of cells varied significantly between subjects and lung volume; the average number of volume elements for all the MLV and TLC models was 4 million (0.9 million) and 6 million (2 million), respectively. The standard deviations are in parentheses. The mesh included thinner boundary layer cells, six deep that had a base surface of ∼0.4 mm.
Particle deposition simulations were performed as an uncoupled post processing step to the flow calculations (Sandeau et al. Citation2010). Ten particles were introduced at each boundary cell (e.g., 6 × 104 particles for H147) with an initial condition of velocity equal to the flow at the entrance to the trachea. The correction for anisotropy proposed by Matida et al. Citation(2004) was implemented using a user-defined function.
Data analysis
The current study considers three inhalation flow rates (15, 30, 60 L/min) and four monodisperse aerosol sizes (1, 3, 5, 10 µm), or 12 test cases for each of the images from 24 volunteers for a total of 288 calculations of particle deposition distribution at MLV and an additional set of 288 calculations at TLC. The large-scale quantitative measures used are right lung deposition fraction, left lung deposition fraction, and the total fraction of particles leaving all exits of the model (penetration fraction). Generations 2 and 3 of the right upper lobe (lobar and segmental level) were selected for a more localized investigation of particle deposition. This region was selected primarily for its relatively homogeneous structural characteristics across subjects, i.e., its moderate inter-subject structural variability, along with the fact that the relevant airways could be easily segmented and matched for the entire population participating in this study. Furthermore, the right upper lobe and, more specifically, the right apical segmental bronchus has traditionally been a site of medical-imaging biomarker research for a variety of lung diseases (Chae et al. Citation2011; Gupta et al. Citation2014), especially since this region appears to be more sensitive to emphysema and certain types of COPD (Bakker et al. Citation2012; Diaz et al. Citation2012). A final comparison of large-scale measures was made using a pseudo cut TLC model. That is, deposition in the generations of the TLC model that do not exist in the MLV model were transferred to the penetration fraction, thus mimicking the case in which CFD calculations are performed in the TLC model geometry that had been reduced to match the depth in generation of the MLV model.
The paired Student t-test in Excel (Microsoft, Redmond, WA, USA) was used to compare data between MLV and TLC deposition results. Statistical significance was assumed for p < 0.05.
Results
Airway model
shows the lung envelopes and segmented airways from the HRCT images from subject H147. The key features for comparison are the large volume increase (137% between MLV and TLC), the depth of the rendered airway trees (i.e., the number of exits, 46 versus 70), and the differences in diameter of individual airways between MLV and TLC (e.g., 7.61 versus 9.75 mm of the lobar root to the Right Upper Lobe). Characteristic data on each of the 24 subjects and the air volume measured with PW2 from the HRCT images are given in . On average, the TLC is almost twice the volume of MLV (86% difference), but the spread of values is significant, ranging from as little as 17% (H175) to over 200% (H170).
Figure 1. Lung envelopes and airways segmented from HRCT images at MLV (left) and TLC (right) for subject H147.
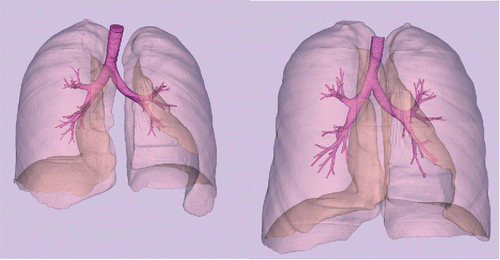
Table 1. Subject data and air volume from imaging. NA is not applicable. UA is unavailable.
Quantitative characteristics of the CFD airway geometry models are given in . Here the geometry volumes and number of CFD cells used show less variation than overall lung volumes, though there is more variation in the depth of the models in terms of the number of distinguishable exits. In one case (H137) where the geometry volume increase in TLC compared to MLV was small (10%), there were fewer exits identified (39 versus 41). Presumably this variation was due to the relatively poor quality of the TLC image, which was degraded for an extraneous reason.
Table 2. Characteristics of the computational fluid dynamics geometry models.
CFD
shows the airway geometry model for the same subject at MLV and TLC, and markers of particle deposition based on CFD calculations with an inhalation flow rate of 30 L/min and 5 micron particles. CFD results over all of the subjects for the average large-scale measures, right and left deposition fractions, and the penetration fraction exiting the models are given in and shown graphically in . The local measures of right upper lobe deposition fraction are given in . The trends in the results follow the known understanding of deposition (Martonen and Katz Citation1993a,Citationb); that is, the greater the flow and larger the particle size the greater the possibility of deposition by impaction in the upper airways. For the large-scale results, only the right deposition fraction for the highest flow rate (60 L/min) and for the largest particle size (10 µm) reaches statistical significance. However, for the local deposition in the right upper lobe there is consistently more deposition in the MLV model (except for the 60 L/min, 10 µm case where they are essentially the same); but there is only statistical significance for two cases. gives the results of average large-scale deposition fractions for each test case where the deposition fractions for the TLC models are “cut” to match the anatomic depth of the MLV model. These data are shown graphically in . Here the effect of only the increased size of the airways is apparent, and thus there is significantly less deposition in the TLC models. These results are statistically significant for all but three cases. gives the deposition results for the single subject H147.
Figure 2. Airway geometry models based on imaging at MLV (left) and TLC (right) for subject H147. Note that the images are at slightly different orientation based on the position of the subject when imaged. Particle deposition results are for 5 µm particles with 30 L/min inhalation flow rate.
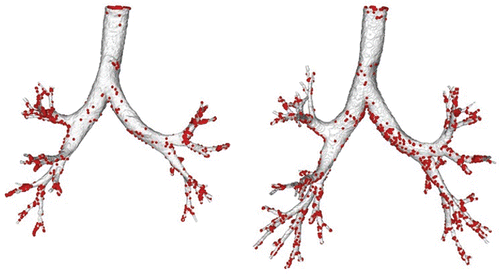
Figure 3. Comparison of MLV vs. TLC data points given in to the identity line for (a) right lung deposition fraction, (b) left lung deposition fraction, and (c) penetration fraction.
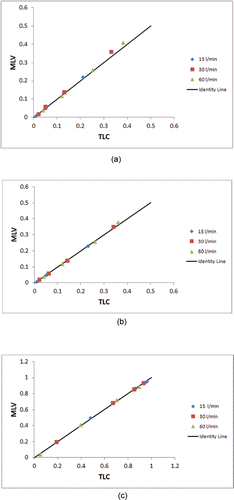
Figure 4. Comparison of MLV vs. the “cut” TLC data points given in to the identity line for (a) right lung deposition fraction, (b) left lung deposition fraction, and (c) penetration fraction.
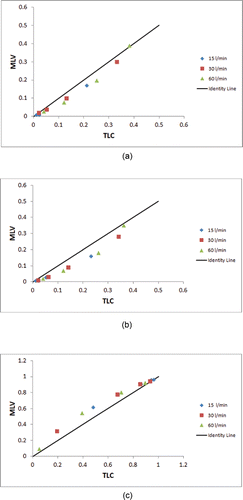
Table 3. Computational fluid dynamics results of average large-scale deposition fractions for each test case.
Table 4. Computational fluid dynamics results of average local deposition fractions in the right upper lobe for each test case.
Table 5. Computational fluid dynamics results of average large-scale deposition fractions for each test case where the deposition fractions for the TLC models are “cut” to match the MLV depth.
Table 6. Computational fluid dynamics results of deposition for subject H147.
Discussion and conclusion
In this study, CFD simulations were performed utilizing a database of HRCT images taken at MLV and TLC from 24 asthmatic subjects after methacholine challenge in realistic human airway models, thus giving the opportunity to assess the impact of lung volume at imaging on CFD results.
The volume data given in illustrate the substantial intersubject variability of MLV relative to TLC observed in this study. This variability is also expressed in the CFD geometry model volumes given in , which exhibit on average a 30% difference between MLV and TLC.
Mechanistically, deposition within the limits of the models should decrease with increased airway dimensions (i.e., at TLC) due to lower velocity flow at the same flow rate in turn resulting in less impaction. On the other hand, the increased depth (i.e., increased number of exits or generations present) of the TLC model yields an increased opportunity for deposition. This behavior is evident in the trends of the large-scale deposition data given in . In addition, the statistical results could be interpreted as supporting the concept that the volume at imaging had no significant effect on the large-scale CFD deposition results. However, this interpretation is misleading because the local deposition patterns were different and, in general, larger airway dimensions result in slower flow and less local deposition in that airway for TLC than MLV. Even so, the combination of morphological differences and physical parameters of flow and particle size interacted to necessitate interpretation of comparisons on a case-by-case basis. The local deposition measures in the Right Upper Lobe, presented in , confirm that for the same anatomical airways the deposition in the TLC model was less than that in the MLV model, but the p values do not reach statistical significance. The most consistent result is for the “cut” TLC model where deposition is lower than in the MLV model, and thus more penetration occurs with high significance statistically. provides ample quantitative evidence that for the case of subject H147 (also shown in ) the difference in CFD deposition results between the two models is considerable.
We believe the study presented herein is unique, but of note is the study by De Backer et al. Citation(2010). In their study, an airway model based on an HRCT image taken at TLC was prepared for CFD flow simulations. The TLC image and a second HRCT image at functional residual capacity were used to determine downstream flow boundary conditions for the CFD simulations by comparing regional volume measurements between the two images, thus using the static images to provide guidance for the dynamic regional flow distribution. Nevertheless, in that study with 6 mild asthmatics, a quantitative assessment on CFD results of the effect of lung volume was not provided.
Our study was limited by having only one type of subject, i.e., challenged asthmatics. Also the CFD analysis was limited to simple boundary conditions of uniform flow at the entrance to the trachea when in fact the deposition there is typically affected by the turbulent jet developed by flow through the glottal aperture (Sandeau et al. Citation2010; Scheinherr et al. Citation2015). Another simplification is the use of uniform pressure at the exits that will not recover the true ventilation distribution (Greenblatt et al. Citation2015a). The impact of these limiting assumptions is difficult to state precisely. However, we expect that as ventilation and deposition heterogeneity increase (e.g., the presence of deposition hotspots) the effects of lung volume would be more pronounced. Thus, the diseased airways would be more affected than healthy ones and the use of uniform pressure boundary conditions would tend to reduce the potential effect. On the other hand, if extra-thoracic airways were included in the model they would tend to filter the largest particles that are most likely to deposit in the upper tracheobronchial (TB) airways. Thus the results for 10 µm particles are probably overstated in that we would not expect many of them to pass through to the TB region.
This article considers an essential shortcoming of using a single static airway model to assess the flow and deposition in a dynamic system. Thus, a logical development with enhanced imaging techniques of much greater bandwidth is 4D-CT: that is, the use of several images taken in time through the breathing cycle (Colby et al. Citation2014; Min et al. Citation2014; Brennan et al. Citation2015). Of note is the work of Lin et al. Citation(2013), which uses multiple images and multiscale modeling to provide downstream boundary conditions at the exits of 3D CFD models of the central airways. In fact, in a very recent paper that has appeared since the current article was submitted, Miyawaki et al. Citation(2016) consider this issue by comparing a static and dynamic model of a single healthy subject. They found differences in lobar deposition and emphasized the potential for increased differences associated with heterogeneous flow patterns.
In conclusion, the influence of elevated lung volume during imaging is to increase airway dimensions in realistic models and thus reduce flow velocity and deposition due to impaction in the upper airways as calculated by CFD. However, unless the same anatomical airways are considered in both models, measures of large-scale deposition may be confounded because the TLC models include deeper generations in the lung that increase the total deposition within the model. These trends are modulated by the flow and particle characteristics of the aerosol, making consistent quantifiable differences between MLV and TLC difficult to predict when models do not include the same anatomical airways. Thus it is a matter for the analyst and the particular goals of his/her study to determine if the errors introduced by the static model will have an impact on the conclusions following their results. We believe the current article can help him/her make that judgment by giving data on both the changes in lung morphology involved and their effect on particle deposition. That is, lung volume should be explicitly considered when using deposition models, and this article gives some insight into the quantification of assumptions. Furthermore, while this study has focused on in silico CFD models, the conclusions that follow also apply to other mechanistic based in silico models (Asgharian, Hofmann, and Bergmann Citation2001; Martonen and Katz Citation1993) and realistic in vitro models (Delvadia et al. Citation2012; Rochefort et al. Citation2007).
References
- ANSYS, FLUENT. (2011). 14.0 Theory Guide. ANSYS Inc., Section 4.4.2., pp. 62–65.
- Asgharian, B., Hofmann, W., and Bergmann, R. (2001). Particle Deposition in a Multiple-Path Model of the Human Lung. Aerosol Sci. Technol., 34(4):332–339. doi:10.1080/02786820119122
- Bakker, M. E., Stolk, J., Reiber, J. H. C., and Stoel, B. C. (2012). Influence of Inspiration Level on Bronchial Lumen Measurements with Computed Tomography. Respir. Med., 106(5):677–686.
- Balásházy, I., Farkas, Á., Szöke, I., and Hofmann, W. (2003). Simulation of Airflow, Aerosol Deposition and Clearance in Central Human Airways. In International Austrian-Israeli Technion Symposia, Particulate Matter and Health, Vienna, Austrian Academy of Sciences.
- Baskin, M. I., Abd, A. G., and Ilowite, J. S. (1990). Regional Deposition of Aerosolized Pentamidine: Effects of Body Position and Breathing Pattern. Annal. Internal Med., 113(9):677–683.
- Bateman, E. D. 1., Hurd, S. S., Barnes, P. J., Bousquet, J., Drazen, J. M., FitzGerald, M., Gibson, P., et al. (2008). Global Strategy for Asthma Management and Prevention: GINA Executive Summary. Eur. Respir. J., 31(1):143–178.
- Brennan, D., Schubert, L., Diot, Q., Castillo, R., Castillo, E., Guerrero, T., Martel, M. K., et al. (2015). Clinical Validation of 4-Dimensional Computed Tomography Ventilation with Pulmonary Function Test Data. Int. J. Radiat. Oncol., Biol., Phys., 92(2):423–429. doi:10.1016/j.ijrobp.2015.01.019
- Chae, E. J., Kim, T.-B., Cho, Y. S., Park, C.-S., Seo, J. B., Kim, N., and Moon, H.-B. (2011). Airway Measurement for Airway Remodeling Defined by Post-Bronchodilator FEV1/FVC in Asthma: Investigation Using Inspiration-Expiration Computed Tomography. Allergy, Asthma Immunol. Res., 3(2):111–117.
- Chan, H.-F., Tawhai, M. H., Levin, D. L., Bartholmai, B. B., and Clark, A. R. (2014). Supine to Upright Lung Mechanics: Do Changes in Lung Shape Influence Lung Tissue Deformation? In Engineering in Medicine and Biology Society (EMBC), 2014 36th Annual International Conference of the IEEE, 832–35. IEEE, New York. http://ieeexplore.ieee.org/xpls/abs_all.jsp?arnumber=6943720
- Colby, S. M., Einstein, D. R., Corley, R. A., Fessler, J. A., and Minard, K. R. (2014). Development and Testing of 4D MRI for Measuring Regional Lung Mechanics with High Spatiotemporal Resolution. Am. J. Respir. Crit. Care Med., 189:A4318.
- Corley, R. A., Kabilan, S., Kuprat, A. P., Carson, J. P., Minard, K. R., Jacob, R. E., Timchalk, C., et al. (2012). Comparative Computational Modeling of Airflows and Vapor Dosimetry in the Respiratory Tracts of Rat, Monkey, and Human. Toxicol. Sci., 128 (2):500–516.
- De Backer, J. W., Vos, W. G., Vinchurkar, S. C., Claes, R., Drollmann, A., Wulfrank, D., Parizel, P. M., Germonpré, P., and De Backer, W. (2010). Validation of Computational Fluid Dynamics in CT-Based Airway Models with SPECT/CT 1. Radiology, 257(3):854–862.
- Delvadia, R. R., Longest, P. W., and Byron, P. R. (2012). In Vitro Tests for Aerosol Deposition. I: Scaling a Physical Model of the Upper Airways to Predict Drug Deposition Variation in Normal Humans. J. Aerosol Med. Pulmonary Drug Del., 25(1):32–40.
- Diaz, A. A., Come, C. E., Ross, J. C., Estãšpar, R. S. J., Han, M. K., Loring, S. H., Silverman, E. K., and Washko, G. R. (2012). Association between Airway Caliber Changes with Lung Inflation and Emphysema Assessed by Volumetric CT Scan in Subjects with COPD. CHEST J., 141(3):736–744.
- Friedman, K., Katz, I., Pichelin, M., Martin, A., Caillibotte, G., Conway, J., Fleming, J., Kroneberg, P., and Muellinger, B. (2011). Erect vs. Supine: A Physical Analysis of Aerosol Deposition in the Lungs Based on Results from Human Subject Experiments. In J. Aerosol Med. Pulmonary Drug Del., 24:162–65.
- Gemci, T., Ponyavin, V., Chen, Y., Chen, H., and Collins, R. (2008). Computational Model of Airflow in Upper 17 Generations of Human Respiratory Tract. J. Biomech., 41(9):2047–2054.
- Greenblatt, E. E., Winkler, T., Harris, R. S., Kelly, V. J., Kone, M., Katz, I., Martin, A., Caillibotte, G., Hess, D. R., and Venegas, J. G. (2016). Regional Ventilation and Aerosol Deposition with Helium-Oxygen in Bronchoconstricted Asthmatic Lungs. J. Aerosol Med. Pulmonary Drug Del., 29(3):260–272.
- Greenblatt, E. E., Winkler, T., Harris, R. S., Kelly, V. J., Kone, M., Katz, I., Martin, A. R., Caillibotte, G., and Venegas, J. (2015a). What Causes Uneven Aerosol Deposition in the Bronchoconstricted Lung? A Quantitative Imaging Study. J. Aerosol Med. Pulmonary Drug Del., 29(3):260–272.
- Greenblatt, E. E., Winkler, T., Harris, R. S., Kelly, V. J., Kone, M., and Venegas, J. (2015b). Analysis of Three-Dimensional Aerosol Deposition in Pharmacologically Relevant Terms: Beyond Black or White ROIs. J. Aerosol Med. Pulmonary Drug Del., 28(2): 116–129.
- Grönkvist, M. J., Emery, M. J., and Gustafsson, P. M. (2002). Mechanisms of Ventilation Inhomogeneity during Vital Capacity Breaths Standing and Supine. Respir. Physiol., 129(3):345–355.
- Gupta, S., Hartley, R., Khan, U. T., Singapuri, A., Hargadon, B., Monteiro, W., Pavord, I. D., et al. (2014). Quantitative Computed Tomography–derived Clusters: Redefining Airway Remodeling in Asthmatic Patients. J. Allergy Clin. Immunol., 133(3):729–738.
- Ibanez, J., and Raurich, J. M. (1982). Normal Values of Functional Residual Capacity in the Sitting and Supine Positions. Intensive Care Med., 8(4):173–177.
- Katz, I. M., Davis, B. M., and Martonen, T. B. (1999). A Numerical Study of Particle Motion within the Human Larynx and Trachea. J. Aerosol Sci., 30(2):173–183.
- Lin, C.-L., Tawhai, M. H., and Hoffman, E. A. (2013). Multiscale Image-Based Modeling and Simulation of Gas Flow and Particle Transport in the Human Lungs. Wiley Interdiscip. Rev.: System. Biol. Med., 5(5):643–655.
- Longest, P. W., and Hindle, M. (2009). Evaluation of the Respimat Soft Mist Inhaler Using a Concurrent CFD and in Vitro Approach. J. Aerosol Med. Pulmonary Drug Del., 22(2):99–112.
- Longest, P. W., and Holbrook, L. T. (2012). In Silico Models of Aerosol Delivery to the Respiratory Tract—Development and Applications. Adv. Drug Del. Rev., 64(4):296–311.
- Longest, P. W., and Vinchurkar, S. (2007). Validating CFD Predictions of Respiratory Aerosol Deposition: Effects of Upstream Transition and Turbulence. J. Biomech., 40(2):305–316.
- Martonen, T. B., and Katz, I. (1993a). Deposition Patterns of Polydisperse Aerosols within Human Lungs. J. Aerosol Med., 6(4): 251–274.
- Martonen, T. B., and Katz, I. M. (1993b). Deposition Patterns of Aerosolized Drugs within Human Lungs: Effects of Ventilatory Parameters. Pharmaceut. Res., 10(6):871–878.
- Matida, E. A., Finlay, W. H., Lange, C. F., and Grgic, B. (2004). Improved Numerical Simulation of Aerosol Deposition in an Idealized Mouth–throat. J. Aerosol Sci., 35(1):1–19.
- Menter, F. R., Langtry, R. B., Likki, S. R., Suzen, Y. B., Huang, P. G., and Völker, S. (2006). A Correlation-Based Transition Model Using Local Variables—Part I: Model Formulation. J. Turbomach., 128(3): 413–422.
- Min, Y., Neylon, J., Shah, A., Meeks, S., Lee, P., Kupelian, P., and Santhanam, A. P. (2014). 4D-CT Lung Registration Using Anatomy-Based Multi-Level Multi-Resolution Optical Flow Analysis and Thin-Plate Splines. Int. J. Computer Assisted Radiol. Surg., 9(5):875–889. doi:10.1007/s11548-013-0975-7.
- Miyawaki, S., Hoffman, E. A., and Lin, C.-L. (2016). Effect of Static vs. Dynamic Imaging on Particle Transport in CT-Based Numerical Models of Human Central Airways. J. Aerosol Sci., 100:129–39. doi:10.1016/j.jaerosci.2016.07.006.
- Montesantos, S., Katz, I., Fleming, J., Majoral, C., Pichelin, M., Dubau, C., Piednoir, B., Conway, J., Texereau, J., and Caillibotte, G. (2013). Airway Morphology from High Resolution Computed Tomography in Healthy Subjects and Patients with Moderate Persistent Asthma. Anatom. Record, 296(6):852–866.
- Petersson, J., Rohdin, M., Sánchez-Crespo, A., Nyrén, S., Jacobsson, H., Larsson, S. A., Lindahl, S. G. E., et al. (2007). Posture Primarily Affects Lung Tissue Distribution with Minor Effect on Blood Flow and Ventilation. Respir. Physiol. Neurobiol., 156(3):293–303.
- Phuong, N. L., and Ito, K. (2015). Investigation of Flow Pattern in Upper Human Airway Including Oral and Nasal Inhalation by PIV and CFD. Build. Environ., 94:504–515.
- de Rochefort, L., Vial, L., Fodil, R., Maître, X., Louis, B., Isabey, D., Caillibotte, G., et al. (2007). In Vitro Validation of Computational Fluid Dynamic Simulation in Human Proximal Airways with Hyperpolarized 3He Magnetic Resonance Phase-Contrast Velocimetry. J. Appl. Physiol., 102(5):2012–2023. doi:10.1152/japplphysiol.01610.2005
- Sandeau, J., Katz, I., Fodil, R., Louis, B., Apiou-Sbirlea, G., Caillibotte, G., and Isabey, D. (2010). CFD Simulation of Particle Deposition in a Reconstructed Human Oral Extrathoracic Airway for Air and Helium–oxygen Mixtures. J. Aerosol Sci., 41(3):281–294.
- Scheinherr, A., Bailly, L., Boiron, O., Lagier, A., Legou, T., Pichelin, M., Caillibotte, G., and Giovanni, A. (2015). Realistic Glottal Motion and Airflow Rate during Human Breathing. Med. Eng. Phys., 37(9):829–839.
- Schroeter, J. D, Asgharian, B., Price, O. T., Kimbell, J. S., Kromidas, L., and Singal, M. (2016). Simulation of the Phase Change and Deposition of Inhaled Semi-Volatile Liquid Droplets in the Nasal Passages of Rats and Humans. J. Aerosol Sci., 95(May): 15–29.
- Stapleton, K.-W., Guentsch, E., Hoskinson, M. K., and Finlay, W. H. (2000). On the Suitability of K–ε Turbulence Modeling for Aerosol Deposition in the Mouth and Throat: A Comparison with Experiment. J. Aerosol Sci., 31(6):739–749.
- Van Ertbruggen, C., Hirsch, C., and Paiva, M. (2005). Anatomically Based Three-Dimensional Model of Airways to Simulate Flow and Particle Transport Using Computational Fluid Dynamics. J. Appl. Physiol., 98(3):970–980.
- Weibel, E. R. (1963). Material and Methods of Preparation for Morphometric Studies of the Lung. In Morphometry of the Human Lung, 39–51. Springer, Berlin Heidelberg. http://link.springer.com/chapter/10.1007/978-3-642-87553-3_4
- Zhang, Z., and Kleinstreuer, C. (2003). Low-Reynolds-Number Turbulent Flows in Locally Constricted Conduits: A Comparison Study. AIAA J., 41(5):831–840.
- Zhang, Z., Kleinstreuer, C., and Kim, C. S. (2009). Comparison of Analytical and CFD Models with Regard to Micron Particle Deposition in a Human 16-Generation Tracheobronchial Airway Model. J. Aerosol Sci., 40(1):16–28.