ABSTRACT
Aerosol dynamics models that describe the evolution of a particle distribution incorporate nucleation as a particle formation rate at a small size around a few nanometers in diameter. This rate is commonly obtained from molecular models that cover the distribution below the given formation size – although in reality the distribution of nanometer-sized particles cannot be unambiguously divided into separate sections of particle formation and growth. When incorporating nucleation, the distribution below the formation size is omitted, and the formation rate is assumed to be in a steady state. In addition, to reduce the modeled size range, the formation rate is often scaled to a larger size based on estimated growth and scavenging rates and the assumption that also the larger size is in a steady state. This work evaluates these assumptions by simulating sub-10 nm particle distributions in typical atmospheric conditions with an explicit molecular-resolution model. Particle formation is included either (1) dynamically, that is, the whole size range starting from single vapor molecules is modeled explicitly or (2) implicitly by using an input formation rate as is done in aerosol models. The results suggest that while each assumption can affect the outcome of new particle formation modeling, the most significant source of uncertainty affecting the formation rates and resulting nanoparticle concentrations is the steady-state assumption, which may lead to an overprediction of the concentrations by factors of approximately from two to even orders of magnitude. This can have implications for modeling and predicting atmospheric particle formation.
Copyright © 2017 American Association for Aerosol Research
EDITOR:
1. Introduction
Based on global-scale simulations and field observations, a comparable fraction of the climatically relevant aerosol particles originate from atmospheric vapors through gas-to-particle conversion, i.e., nucleation and condensation (Reddington et al. Citation2011; Kerminen et al. Citation2012). Understanding the physics and chemistry of atmospheric new particle formation (NPF) and representing them accurately in air quality and climate models is thus necessary for quantifying the role of aerosol particles in the Earth system.
The very first steps of NPF are incorporated in aerosol dynamics models as a formation rate of particles of ∼1–2 nm in diameter (Jung et al. Citation2006; Sihto et al. Citation2009). The formation rate depends on the ambient conditions such as the concentrations of condensable vapors and the temperature, and is obtained from molecular theories, or parameterizations based on modeling or measurements. Models providing the formation rate typically treat the particles—or molecular clusters—below a couple of nanometers with more accurate, discrete molecular-resolution approaches (see, e.g., McGrath et al. Citation2012, and references therein). These models explicitly describe the population dynamics by a set of discrete kinetic equations which accurately treat the molecular collisions and evaporations forming and growing the population (Lehtinen and Kulmala Citation2003; Holten and van Dongen Citation2009).
The same level of accuracy is not achievable in aerosol dynamics models, which instead rely on solving the continuous general dynamic equation (GDE, e.g., Ramabhadran et al. Citation1976; Kumar and Ramkrishna Citation1997; Chapter 13 in Seinfeld and Pandis Citation2006). When applying the continuous GDE, the formation and initial growth are considered as two separate processes, “nucleation and subsequent growth,” in which newly formed particles are introduced at a given formation size and then grown toward larger sizes with a separate model framework (Zhang et al. Citation1999). The dominating compounds and the relative roles of condensation, evaporation, and coagulation vary at different stages of the growth process (Ehn et al. Citation2014). Yet, there is no fundamental difference in the physics below and above the “formation size.” The reason for separating the process into two different stages is primarily mathematical: the continuous GDE does not consider the stochastic widening of the particle size distribution, which plays a major role in the dynamics of newly formed particle populations at the smallest sizes of a couple of nanometers (Holten and van Dongen Citation2009; Wang et al. Citation2013; Olenius et al. Citation2015).
Combining the results of molecular approaches with aerosol dynamics models by using the formation rate as an input variable involves certain assumptions or approximations. Assessing these assumptions for different atmospheric environments is important especially now that much effort is put to obtaining more accurate estimates for NPF rates in different conditions (Almeida et al. Citation2013; Riccobono et al. Citation2014; Glasoe et al. Citation2015), and climate and air-quality models aim at increasingly better description of NPF by implementing the state-of-the-art formation rate schemes (Riccobono et al. Citation2014; Baranizadeh et al. Citation2016; Croft et al. Citation2016).
In this work, we give a brief review of the principles of molecular cluster kinetics models used to determine initial formation rates, and list the main assumptions related to treating nucleation in aerosol dynamics models. The assumptions are assessed in different representative atmospheric conditions by simulations of nanoparticle populations with a discrete molecular-resolution model. The aim is to answer the following question: if an accurate particle formation model is combined with an accurate growth dynamics model, how large are the uncertainties that arise solely from simulating the different size ranges separately and combining them via the formation rate? Based on the simulated size distributions, we discuss the implications of the assumptions for NPF modeling. While the scope is on atmospheric environments, the results are relevant also for other applications, such as nucleation chamber experiments.
2. Particle formation, initial growth, and the “formation size” in the context of atmospheric NPF
Expressions such as “nucleation and subsequent growth” are used often in atmospheric NPF studies (Curtius Citation2006; Zhang et al. Citation2012). The terms imply that the conversion from vapor molecules to particles is physically a two-step process, in which particles first form at some specific size, and then begin to grow. To clarify these concepts, the main principles of molecular models that are generally used to describe the initial formation, as well as their application in aerosol dynamics models, are briefly reviewed below. The concepts are schematically presented in .
Figure 1. Schematic figure of the size-dependent particle formation (nucleation) rate described by a molecular model, and its incorporation in an aerosol dynamics model. The formation rates are qualitative, and demonstrate the differences of the different steady-state approaches (blue) to each other, as well as to the true dynamic formation rate in the case of a time-dependent situation (red hues). The solid (red-hued) lines illustrate an example behavior of the dynamic formation rate relative to the steady-state rate in a diurnal cycle where the vapor concentration Cvapor first increases, then stays at a maximum, and finally decreases. The relations of the rates may be different depending on the ambient conditions; e.g., the scaling approach may give values either lower or higher than the explicit steady-state solution.
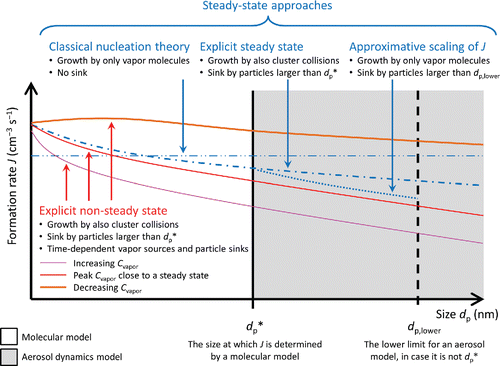
The conception of two separate stages partly originates from the classical nucleation theory (CNT) which emphasizes the role of the critical cluster, i.e., the molecular cluster size that has the maximum formation free energy. The fact that subcritical particles are unstable in the sense that they tend to evaporate faster than they grow has sometimes been interpreted to imply that the distribution below the critical size is negligible, and that new particles form directly at the critical size, making it the “formation size” (Ramabhadran et al. Citation1976; Duplissy et al. Citation2016). However, the relative instability of subcritical sizes does not mean that they cannot exist in significant amounts. Furthermore, it is not clear if atmospheric molecular systems actually exhibit a distinct, well-defined critical size (Olenius et al. Citation2013; Girshick Citation2014).
2.1. Main principles of the classical nucleation theory
The CNT (Becker and Döring Citation1935; Zeldovich Citation1943) is the most widely used theoretical approach to examine the formation of small liquid particles from vapors (for derivations and critical analyses; Ford Citation2004; Vehkamäki Citation2006; Merikanto et al. Citation2007). The original CNT is a combination of classical thermodynamics and simplified particle population kinetics. The thermodynamic aspect has generally been given more emphasis, and the main topic of interest has been identifying the critical cluster corresponding to the maximum Gibbs free energy of formation ΔG. The energetics have traditionally been derived from the liquid drop model, which gives a free-energy profile exhibiting a single maximum and no minima (Zhang et al. Citation2012).
The particle formation rate is derived from the kinetic equations governing the particle size distribution. The equations, here written for one component for simplicity, describe the formation and decay of each particle size i:
In Equation (Equation1), Ci is the concentration of a particle of i molecules, β1,i is the rate coefficient for the collision (i.e., attachment) of a vapor molecule to particle i, and γi → 1,i−1 is the rate coefficient for the evaporation of a molecule from particle i. The time derivative dCi/dt contains all collision and evaporation processes involving a particle and a vapor molecule that can form or destroy particle i, and the distribution is assumed to be in time-independent steady state dCi/dt = 0. The net particle flux from size i to the consecutive size i + 1, that is, the formation rate at size i + 1 is
[2]
Since no other kinetic processes are considered and a steady state is assumed, it follows directly that the net flux Ji → i+1 between two consecutive particle sizes is equal for all sizes i. This naturally applies also for particle formation from multiple compounds. The flux Ji → i+1 ≡ J is solved by expressing the evaporation rates γ via the Gibbs free energies of formation as γi → 1,i−1 ∝ exp[(ΔGi – ΔGi−1 – ΔG1)/(kBT)], where kB is the Boltzmann constant and T is the temperature, and summing the kinetic equations over all cluster sizes (Girshick and Chiu Citation1990). If the free-energy profile exhibits a global maximum as is the case with the liquid drop model, the sum is dominated by the maximum. Assuming also that the critical size is large enough, the sum can be approximated with a Gaussian integral, and solved to yield the final expression for J which thus contains the free energy ΔG* at the critical size (Vehkamäki Citation2006)[3]
This seemingly underlines the role of the critical cluster in the particle formation process, but it should be kept in mind that the formation rate given by EquationEquation (3)[3] is not specific to the critical size. From the perspective of the population dynamics, it is clear that the critical size is not in any special position in terms of the particle flux J (EquationEquation (2)
[2] ): while it is established that CNT gives the formation rate at the critical size (Curtius Citation2006; Zhang et al. Citation2012; Duplissy et al. Citation2016), it in fact gives the formation rate at all cluster sizes (thin dash-dot-dotted line in ).
2.2. Improved descriptions of the initial formation process
The use of classical bulk thermodynamics to describe the properties of clusters consisting of only a few molecules has often been criticized (e.g., Merikanto et al. Citation2007 and references therein), and more accurate thermodynamic approaches, namely, electronic structure methods have been proposed for the CNT framework (Merikanto et al. Citation2016). It must, however, be kept in mind that the final form of the CNT-derived formation rate J (Equation (Equation3[3] )) requires that the free-energy profile ΔG along the cluster growth pathway exhibits a distinct global maximum. This assumption stems from the liquid drop model, but for complex atomic systems, the qualitative-energy profile may be different (Olenius et al. Citation2013; Girshick Citation2014). If there exist more than one maxima of comparable heights, the summing procedure applied in CNT to yield J becomes inapplicable (Kupiainen-Määttä et al. Citation2014), and the formation rate must be solved numerically.
While efforts have been made to improve the description of the energetics in the CNT framework, the kinetic treatment has received less attention. For an improved treatment, the kinetic equations (EquationEquation (1)) need to be solved considering also the following kinetic processes or aspects not included in CNT:
Scavenging of the molecular clusters by external sinks: External sinks, such as larger aerosol particles or walls of a measurement chamber are generally present in atmospheric situations and experiments. The presence of sinks makes the formation rate dependent on the cluster size, and their effect is likely to be non-negligible at typical atmospheric vapor concentrations (Ehrhart and Curtius Citation2013; Kupiainen-Määttä et al. Citation2014; Malila et al. Citation2015; dash-dotted line in ).
Self-coagulation among the cluster population: EquationEquation (1)
considers solely collisions involving a cluster and a vapor molecule. However, when the relative role of cluster evaporation decreases due to high vapor concentrations or suppressed evaporation, cluster concentrations may become so high that also cluster self-coagulation needs to be taken into account (Rao and McMurry Citation1989; Vehkamäki et al. Citation2012; Lehtipalo et al. Citation2016; dash-dotted line in ).
Non-steady-state situations: CNT, as well as many other cluster formation schemes, considers time-independent conditions and is generally not applicable to non-steady-state situations. In the atmosphere, such situations can arise if ambient conditions change in timescales that are much shorter than the timescales in which the cluster population adapts to changes in the environment (Yu Citation2003; solid lines in ).
To summarize, the size-dependent cluster formation rate is affected by both the properties of the clustering vapors, and the external conditions such as scavenging sinks. Thermodynamic barriers shape the cluster distribution and thus the formation rates, as they determine the relative collision and evaporation frequencies. It needs to be emphasized, however, that the initial formation rate, generally referred to as the nucleation rate, is not a property of some specific critical cluster size, but a quantity defined for all sizes starting from the initial agglomerate of two molecules, regardless of whether there exists a critical cluster or not. In cases where there exists an unambiguous critical size, the physical nature of the clustering process does not undergo any fundamental change at this size (Holten and van Dongen Citation2009).
2.3. Incorporating particle formation in aerosol dynamics modeling
The newly formed particles are introduced in aerosol dynamics models by placing them at the “formation size.” The condensation and coagulation dynamics are modeled from this size upward with a conventional approach for discretizing the aerosol size distribution function (Zhang et al. Citation1999; Korhonen et al. Citation2003 and Citation2004; grey area in ). When using formation rates obtained from CNT, the initial “formation size” is generally taken to be the critical size (Jung et al. Citation2006; Sihto et al. Citation2009; Duplissy et al. Citation2016). However, as explained above, the “formation size” is in practice arbitrary, as long as the subsequent sizes can be assumed to be relatively stable so that they do not evaporate back to the “subformation” sizes in large amounts. Incorporating NPF via a formation rate involves the following assumptions or approximations:
(1) | As the distribution below the “formation size” (white area in ) is neglected, particles smaller than that size do not affect the distribution dynamics. They do not reduce the vapors by binding vapor molecules, or contribute to the growth of larger particles by coagulating with them. | ||||
(2) | The input formation rate is generally determined with nucleation or clustering theories that consider a steady-state situation (such as CNT or other cluster kinetics approaches; dash-dot-dotted and dash-dotted lines in ). The time in which the distribution of the smallest particles reaches a steady state is assumed to be short compared to the time scale of variations in ambient atmospheric conditions (Chapter 11.1 in Seinfeld and Pandis Citation2006), but this assumption may be sensitive to the sources and sinks of the vapors and particles (Warren and Seinfeld Citation1984; Yu Citation2003). | ||||
(3) | To reduce the modeled size range, the formation rate originally given for some small size dp,1 is sometimes extrapolated to a larger size dp,2 (Lee et al. Citation2013; Makkonen et al. Citation2014; dotted line in ). The most widely used formula for this is (Kerminen and Kulmala Citation2002; Lehtinen et al. Citation2007) |
A typical value for the parameter m is −1.6. The formula replaces the actual modeling of the growth between sizes dp,1 and dp,2 by an approximation for the size-dependent formation rate, based on the continuous GDE for a steady-state situation with no self-coagulation (Kerminen and Kulmala Citation2002; Lehtinen et al. Citation2007; for the effect of self-coagulation, see Anttila et al. Citation2010).
3. Sub-10 nm aerosol dynamics simulations using a molecular-resolution model
We now assess the effects of aforementioned assumptions 1–3 by simulating the time evolution of the distribution of nanometer-sized particles. The time development of the system mimics a diurnal cycle in an atmospheric environment. The simulations are performed with a discrete model that treats the size range explicitly molecule-by-molecule, starting from the initial molecular dimer. The discrete GDE for condensation and coagulation is similar to EquationEquation (1), but includes also collision and evaporation processes occurring between two particles, as well as other dynamic processes affecting the population:
[6]
In EquationEquation (6)[6] , Qi is an external source term that is most often relevant only for the vapor monomers, and Si is the loss rate coefficient corresponding to an external particle sink. Note that when j ≠ i−j, the collision and break-up fluxes βj,i−jCjCi−j and γi → j,i−jCi are equal for the smaller sizes j and i−j and for the larger size i. When j = i−j, the fluxes are from the point of view of size j, i.e., twice the fluxes from the point of view of size i.
The discrete GDE provides the physically accurate description of the dynamics of small particles (Lehtinen and Kulmala Citation2003; Korhonen et al. Citation2003; Holten and van Dongen Citation2009; Olenius et al. Citation2015), but its applications are restricted to relatively small model systems, since the number of kinetic equations becomes computationally unbearable as the modeled size range increases to particles consisting of thousands of molecules and/or multiple chemical compounds. It is used in this work for the whole simulated size range in order to study the interface between the distribution below and above the assumed formation size without the uncertainties related to the continuous GDE and discretization errors (Korhonen et al. Citation2003).
The simulations are performed as follows: first, an explicit simulation including all sizes is performed, and the formation rates at different sizes dp = 1.5, 3, 5, and 10 nm are saved. The formation rate J (dp, t) is defined as the net particle flux over the given size dp, i.e., the sum of the fluxes of processes in which two particles below dp collide to produce a particle at or above dp. The three assumptions listed in Section 2.3 are then tested one at a time:
(1) | To assess the effect of omitting the distribution below the “formation size,” the same conditions are simulated again, but now using the time-dependent formation rate J (dp, t), recorded in the explicit simulation, as a source term Q for size dp,input, set to either 1.5 or 3 nm. The rate equations are solved only for sizes ≥dp,input and the vapor monomer, the concentration of which is also reduced according to J (dp,input, t). | ||||
(2) | To investigate the steady-state assumption, the simulation is performed again with an input formation rate at size dp,input, now using the steady-state rate Jss (dp,input) corresponding to the simulation conditions at each moment. The values Jss (dp,input) are obtained by performing steady-state simulations for smaller systems containing particles up to the given sizes dp,input. The results are saved as look-up tables of steady-state rates as a function of vapor concentration and scavenging sink, from which Jss (dp,input) at specific conditions is obtained by interpolation. | ||||
(3) | Finally, to assess the scaling procedure, the input formation rate at dp,input is derived by extrapolating the steady-state rate Jss determined for a smaller size to dp,input as in EquationEquation (4) |
We use a simple model system described by parameters representative of typical atmospheric molecules involved in NPF (Almeida et al. Citation2013). The details of the simulation set-up are given in the online supplementary information (SI). The model substance is a quasiunary representation of particles that are formed by sulfuric acid-driven nucleation, with additional organic compounds condensing onto particles larger than 3 nm in mass diameter. The largest simulated size is approximately 11 nm. We consider three simulation cases corresponding to different atmospheric boundary layer environments: (Equation1) a remote, (Equation2
[2] ) an average, and (Equation3
[3] ) a polluted location (Table S1). The concentration of the vapor driving the particle formation, and the scavenging sink caused by a background population of large particles increase from case 1 to case 3.
4. Results and discussion
The simulation results are presented in . shows the true time evolution of the particle distribution for each case study, obtained from the explicit simulations with no input formation rate. and present the formation rates J (dp) and total concentrations C (≥dp), respectively, for different sizes dp when assumptions 1–3 are incrementally implemented in the simulations.
Figure 2. The true time evolution of the particle distribution in the explicit simulations with no input formation rate. Simulation conditions for each case study are found in Table S1. The particles are classified into size bins so that the bin boundaries are equally spaced along the logarithmic size axis. The lower mass boundary of bin n + 1 is Mn+1 = 1.5× Mn, and the lower boundary of the first bin is the mass of a dimer of the model substance.
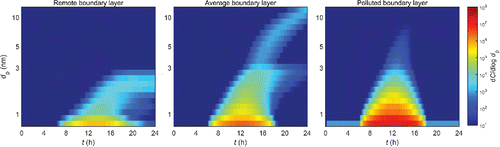
Figure 3. Formation rate J (dp) over different sizes dp for the three representative environments. Solid (black) lines: the true values obtained from the explicit simulation with no input formation rate. Dashed lines: simulation in which the time-dependent formation rate J (dp,input, t) obtained from the explicit simulation is used as input (indistinguishable from the solid lines except for the polluted case with dp,input = 1.5 nm). Dash-dotted lines: simulation in which the steady-state rate Jss (dp,input) is used as input. Dotted lines: simulations in which the input rate J (dp,input) is extrapolated from the steady-state rate Jss (dp) determined for a smaller size dp < dp,input (indistinguishable from the dash-dotted lines for the remote case). The input size is either 1.5 nm (blue lines) or 3 nm (red lines); for the dotted (red) lines, the initial size before the extrapolation is dp = 1.5 nm. The lighter solid (green) lines in the top panels show the vapor concentration Cvapor scaled by a factor of 10−6.
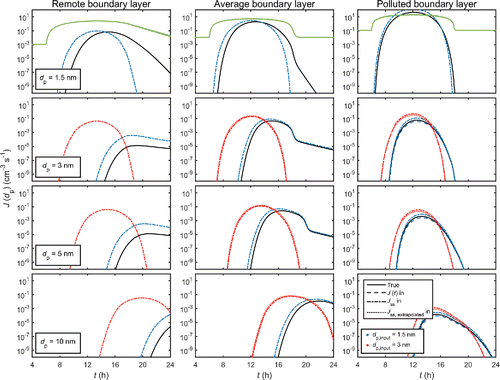
Figure 4. Total particle concentration C (≥dp) from sizes dp upward for the three representative environments. Explanations for the lines are as in .
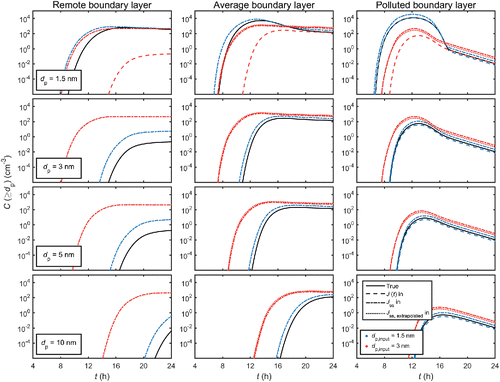
The magnitudes of the effects of the evaluated assumptions depend strongly on the ambient conditions. summarizes the effects on J (dp) and C (≥dp), characterized by the differences between the maxima of J and C in a given simulation case with or without the simplifying assumptions. When using an input formation rate, the time development of J and C is for the most part similar to the explicit simulation, but shifted along the time axis, and thus the differences are often much larger at a specific moment in time.
Table 1. Approximate effects of the assumptions made when using an input formation rate J (dp,input) in particle size distribution simulations. The effects are presented as the order of the ratios Jmax (dp)/Jtrue,max (dp) and Cmax (≥dp)/Ctrue,max (≥dp) in the highest values of the formation rates and concentrations compared to the explicit simulation (the two ratios are similar with the used accuracy), and the time shift in the appearance of new particles. The time shift is characterized by the difference t (Jtrue,max (dp,input)) − t (Jmax (dp,input)) between the times at which J (dp,input) exhibits its peak value in the explicit simulation and in the simulations using an input formation rate. Situations in which the effects are notable for, e.g., measurement comparisons are marked in bold, and situations in which they severely distort the results for, e.g., air-quality modeling are marked in italic.
4.1. Effect of omitting the distribution below the “formation size”
In and , the results of the simulations using the true time-dependent formation rate J (dp,input, t) obtained from the explicit simulation as input for size dp,input, and omitting the sizes below it are shown as dashed lines. The differences with respect to the explicit simulation, shown as black solid lines, are rather minor or even negligible. For input size dp,input = 1.5 nm (blue dashed lines) in the remote and average conditions, the formation rates and concentrations are slightly decreased compared to the exact results (; in and the dashed lines are indistinguishable from the solid lines). For the polluted environment, the underestimation is more significant although still small. In this case, the concentrations of the small sizes in the explicit simulation are high enough for these particles to contribute by coagulation to the formation and growth of sizes larger than 1.5 nm. When the input size is increased to 3 nm, the differences to the explicit simulation become negligible for all studied cases. This is due to the organic compounds participating in the growth from 3 nm onwards, reducing the roles of the particle-forming vapor and the small clusters in the growth process. Overall, the quantitative effects are rather small with respect to, for instance, typical measurement accuracies, but can still be non-negligible in the presence of vapors that efficiently form molecular clusters, increasing the concentrations of the “subformation” sizes.
4.2. Effect of the assumption of a steady-state formation rate
The assumption of a steady-state formation rate, depicted as dash-dotted lines in and , may have prominent effects on the modeled distribution. The larger the input size, the longer it takes for it to adapt to changes in the ambient conditions, and the more uncertain the steady-state assumption becomes. When using the steady-state formation rates Jss (dp,input), the newly formed particles appear too early at the given input size as the adaptation time is not considered, and the quantitative rates may differ significantly from the true dynamic rates J (dp,input, t) (). Increasing the input size more and more eventually leads to the time scale of approaching the steady state exceeding the time scale of variations in the vapor concentration to the extent that the steady-state assumption becomes completely invalid.
In addition to the input size, the applicability of the steady-state approach is affected by the time scales of the dynamic processes among the population. For the remote case, the collision and scavenging rate constants are low with respect to the rate at which the vapor concentration changes. When the vapor concentration starts to increase, the true formation rate follows the changes with a delay (solid lines in and ), and may not even reach the steady-state rate before the vapor concentration changes again. When using the steady-state rate, the formation rate is assumed to reflect the vapor concentration with no delay, and as a result the concentrations are overpredicted already when dp,input = 1.5 nm (blue dash-dotted lines). In the average and polluted environments, the collision processes occur at relatively higher frequencies, and the formation rate at 1.5 nm rapidly adapts to changes in the vapor concentration, resulting in notably less severe overprediction. For dp,input = 3 nm (red dash-dotted lines), the assumption that the formation rate instantaneously follows the vapor concentration becomes worse even for the polluted case. As an additional test, the conclusions on the steady-state assumption were verified by studying how far the explicitly simulated system is from a steady state (Figure S1 in the SI).
4.3. Effect of extrapolating the formation rate from a smaller size
The dotted lines (red) in and show the results for using the steady-state formation rate determined for 1.5 nm, and scaled to dp,input = 3 nm according to EquationEquation (4)[4] . Compared to the simulations using a steady-state rate determined directly at 3 nm (red dash-dotted lines), the additional uncertainties due to the extrapolation are not very large: the differences to the results of the explicit simulation are of the same order (). This is because the scaling formula is derived for a steady state situation, but due to its approximations, its result is likely to differ more or less from the exact solution for J at the extrapolated size (Olenius et al. Citation2015). Depending on the conditions, the extrapolated values may be either too low or too high, or the effect of the approximations may even be negligible, as in the remote boundary layer case. Concisely, applying the scaling procedure is similar to using the steady-state approach at the input size, but with some extra uncertainties in the steady-state formation rate.
4.4. Implications for NPF modeling
Based on the presented case studies, the steady-state assumption is by far the worst approximation made in describing the initial particle formation process, and can have implications for predicting atmospheric NPF and interpreting observations. The assumption generally tends to overpredict the nanoparticle concentrations and distort their temporal profiles in time-dependent situations, as the relaxation time required for the formation rate to adapt to changes in the ambient conditions is not considered. The relaxation time has been brought up in studies addressing situations with a constant vapor production rate by, e.g., Warren and Seinfeld (Citation1984) and Shi et al. (1990), who also showed that the presence of scavengers reduces the time lag. Yu (Citation2003) studied the dependence of the time lag on vapor concentration and particle size by simulating the build-up of a particle population at a fixed vapor concentration, and reported longer relaxation times at lower vapor concentrations.
For a diurnal cycle exhibiting a peak in the vapor concentration, neglecting the time lag results in a too early and likely also too strong formation event ( and ). If the input size is increased, the time lag may become so long that the use of steady-state formation rates leads to particle formation being predicted at times at which it in reality does not occur at all (e.g., red lines in the remote and average boundary layer cases in ). This limits applying a steady-state formation rate at larger sizes, whether the rate is determined by detailed molecular modeling or the common extrapolation procedure (EquationEquation (4)[4] ) which also corresponds to a steady state. The effects on the resulting particle concentrations may be notable on a global scale: a progressive overprediction of nanoparticle concentrations has been observed in a general circulation model when the input size was increased via the extrapolation formula (Lee et al. Citation2013). The optimal input size depends on how high uncertainties are considered acceptable: to avoid uncertainties higher than, for instance, a factor of two, the case studies suggest that the optimal input size for incorporating the formation rate is smaller than 3 nm. Compared to the explicit simulations, using steady-state formation rates at 1.5 nm results in uncertainties of factors between approximately 1.5 and 30 in the modeled nanoparticle concentrations, whereas increasing the input size to 3 nm leads to uncertainties of factors of 4–103. In case of small input sizes for which the absolute formation rates may not be significantly overestimated but mainly shifted further along the time axis, the steady-state approach could be improved by considering the time delay in the incorporation of the formation rate. Assessing the time shifts, however, requires that the ambient conditions affecting the particle growth and survival to the input size do not change significantly during the growth time, and should thus be applied with caution.
On the other hand, at higher vapor concentrations where the steady-state assumption becomes better, the omission of the distribution below the “formation size” can underpredict coagulational growth in case of strong clustering at these smallest sizes. Such clustering has been experimentally observed in atmospheric conditions (Lehtipalo et al. Citation2016). The effects may not be as prominent as those of the steady-state assumption, here an underprediction of down to a factor of 0.6 in the nanoparticle concentrations, but can be of importance when a detailed description of the growth dynamics is desired.
It must be noted that the simulations of this work represent formation events of 24 h, and in addition to the initial formation rates the eventual particle concentrations are affected also by the subsequent development of the ambient conditions. For instance, the survival and growth of particles that have not grown beyond a few nanometers during the first day depend on the evolution of the scavenging sinks and the availability of condensable vapors during the following days.
5. Conclusions
In aerosol dynamics models, the formation of particles from vapors is incorporated by feeding in the steady-state formation rate, determined by a molecular model or parameterization, at a small size around a few nanometers. As the formation and growth is physically an inseparable process, the size at which the formation rate is given is a matter of convention. The advantage of using an input size larger than ∼1–2 nm is that a wider size range is described accurately: stochastic effects that cannot be neglected for sizes of a few nanometers are inherently not described by aerosol dynamics models, while molecular-resolution models treat the dynamics of the smallest sizes correctly. A larger input size may also be required due to computational limitations. On the other hand, the steady-state assumption regarding the formation rate is expected to become increasingly worse as the input size is increased. In addition, the size range below the input size, excluded in the aerosol dynamics model broadens with increasing input size. In reality, the effect of the omitted “subformation” sizes on the dynamics of the larger particles via coagulation may be non-negligible.
According to the present molecular-resolution simulations of sub-10 nm particle populations, the fact that the distribution below the given formation size is omitted has minor effects on the modeled concentrations. The assumption of a steady-state formation rate, by contrast, may lead to significant distortions: the differences between the true dynamic, time-dependent formation rate and the steady-state rate may be prominent with respect to both the quantitative values, and the behavior as a function of time. The differences generally lead to an overprediction of the concentrations of nanometer-sized particles, and distort the temporal pattern of the NPF event. A similar effect arises from using a formation rate determined for a size smaller than the input size, and scaling it to the input size with commonly used extrapolation formulae. These problems are likely to become significant already for input sizes of or larger than approximately 3 nm, and for environments with low vapor and particle concentrations. The effects may be a notable issue when aiming to quantitatively accurate description of particle distributions, for instance, in measurement comparisons seeking to explain observations, or in climate and air quality studies involving atmospheric nanoparticle concentrations.
UAST_1262530_Supplementary_File.zip
Download Zip (479.1 KB)Acknowledgments
The authors thank Dr. Oona Kupiainen-Määttä for contributions to developing the ACDC program, and Dr. Jan Julin and Prof. Kari Lehtinen for useful comments on the manuscript.
Funding
ERC project 278277-ATMOGAIN and Formas project 2015-749 are gratefully acknowledged for funding.
References
- Almeida, J., Schobesberger, S., Kürten, A., Ortega, I. K., Kupiainen-Määttä, O., Praplan, A. P., Adamov, A., Amorim, A., Bianchi, F., Breitenlechner, M., David, A., Dommen, J., Donahue, N. M., Downard, A., Dunne, E., Duplissy, J., Ehrhart, S., Flagan, R. C., Franchin, A., Guida, R., Hakala, J., Hansel, A., Heinritzi, M., Henschel, H., Jokinen, T., Junninen, H., Kajos, M., Kangasluoma, J., Keskinen, H., Kupc, A., Kurtén, T., Kvashin, A. N., Laaksonen, A., Lehtipalo, K., Leiminger, M., Leppä, J., Loukonen, V., Makhmutov, V., Mathot, S., McGrath, M. J., Nieminen, T., Olenius, T., Onnela, A., Petäjä, T., Riccobono, F., Riipinen, I., Rissanen, M., Rondo, L., Ruuskanen, T., Santos, F. D., Sarnela, N., Schallhart, S., Schnitzhofer, R., Seinfeld, J. H., Simon, M., Sipilä, M., Stozhkov, Y., Stratmann, F., Tomé, A., Tröstl, J., Tsagkogeorgas, G., Vaattovaara, P., Viisanen, Y., Virtanen, A., Vrtala, A., Wagner, P. E., Weingartner, E., Wex, H., Williamson, C., Wimmer, D., Ye, P., Yli-Juuti, T., Carslaw, K. S., Kulmala, M., Curtius, J., Baltensperger, U., Worsnop, D. R., Vehkamäki, H., and Kirkby, J. (2013). Molecular Understanding of Sulphuric Acid-Amine Particle Nucleation in the Atmosphere. Nature, 502:359–363.
- Anttila, T., Kerminen, V.-M., and Lehtinen, K. E. J. (2010). Parameterizing the Formation Rate of New Particles: The Effect of Nuclei Self-Coagulation. J. Aerosol Sci., 41:621–636.
- Baranizadeh, E., Murphy, B. N., Julin, J., Falahat, S., Reddington, C. L., Arola, A., Ahlm, L., Mikkonen, S., Fountoukis, C., Patoulias, D., Minikin, A., Hamburger, T., Laaksonen, A., Pandis, S. N., Vehkamäki, H., Lehtinen, K. E. J., and Riipinen, I. (2016). Implementation of State-of-the-Art Ternary New-Particle Formation Scheme to the Regional Chemical Transport Model PMCAMx-UF in Europe. Geosci. Model Dev., 9:2741–2754, doi:10.5194/gmd-9-2741-2016.
- Becker, R., and Döring, W. (1935). Kinetische Behandlung der Keimbildung in übersättigen Dämpfen. Ann. Phys. (Leipzig), 24:719–752.
- Croft, B., Martin, R. V., Richard Leaitch, W., Tunved, P., Breider, T. J., D'Andrea, S. D., and Pierce, J. R. (2016). Processes Controlling the Annual Cycle of Arctic Aerosol Number and Size Distributions. Atmos. Chem. Phys., 16:3665–3682, 10.5194/acp-16-3665-2016.
- Curtius, J. (2006). Nucleation of Atmospheric Aerosol Particles. C. R. Phys., 7:1027–1045, 10.1016/j.crhy.2006.10.018.
- Duplissy, J., Merikanto, J., Franchin, A., Tsagkogeorgas, G., Kangasluoma, J., Wimmer, D., Vuollekoski, H., Schobesberger, S., Lehtipalo, K., Flagan, R. C., Brus, D., Donahue, N. M., Vehkamäki, H., Almeida, J., Amorim, A., Barmet, P., Bianchi, F., Breitenlechner, M., Dunne, E. M., Guida, R., Henschel, H., Junninen, H., Kirkby, J., Kürten, A., Kupc, A., Määttänen, A., Makhmutov, V., Mathot, S., Nieminen, T., Onnela, A., Praplan, A. P., Riccobono, F., Rondo, L., Steiner, G., Tome, A., Walther, H., Baltensperger, U., Carslaw, K. S., Dommen, J., Hansel, A., Petäjä, T., Sipilä, M., Stratmann, F., Vrtala, A., Wagner, P. E., Worsnop, D. R., Curtius, J., and Kulmala, M. (2016). Effect of Ions on Sulfuric Acid-Water Binary Particle Formation: 2. Experimental Data and Comparison with QC-Normalized Classical Nucleation Theory. J. Geophys. Res. Atmos., 121:1752–1775, 10.1002/2015JD023539.
- Ehn, M., Thornton, J. A., Kleist, E., Sipilä, M., Junninen, H., Pullinen, I., Springer, M., Rubach, F., Tillmann, R., Lee, B., Lopez-Hilfiker, F., Andres, S., Acir, I.-H., Rissanen, M., Jokinen, T., Schobesberger, S., Kangasluoma, J., Kontkanen, J., Nieminen, T., Kurtén, T., Nielsen, L. B., Jørgensen, S., Kjaergaard, H. G., Canagaratna, M., Maso, M. D., Berndt, T., Petäjä, T., Wahner, A., Kerminen, V.-M., Kulmala, M., Worsnop, D. R., Wildt, J., and Mentel, T. F. (2014). A Large Source of Low-Volatility Secondary Organic Aerosol. Nature, 506:476–479.
- Ehrhart, S., and Curtius, J. (2013). Influence of Aerosol Lifetime on the Interpretation of Nucleation Experiments with Respect to the First Nucleation Theorem. Atmos. Chem. Phys., 13:11465–11471.
- Ford, I. J. (2004). Statistical Mechanics of Nucleation: A Review. Proc. Inst. Mech. Eng. Part C J. Mech. Eng. Sci., 218:883–899, 10.1243/0954406041474183.
- Girshick, S. L. (2014). The Dependence of Homogeneous Nucleation Rate on Supersaturation. J. Chem. Phys., 141:024307, 10.1063/1.4887338.
- Girshick, S. L., and Chiu, C.-P. (1990). Kinetic Nucleation Theory: A New Expression for the Rate of Homogeneous Nucleation from an Ideal Supersaturated Vapor. J. Chem. Phys., 93:1273–1277.
- Glasoe, W. A., Volz, K., Panta, B., Freshour, N., Bachman, R., Hanson, D. R., McMurry, P. H., and Jen, C. (2015). Sulfuric Acid Nucleation: An Experimental Study of the Effect of Seven Bases. J. Geophys. Res. D Atmos., 120:1933–1950, 10.1002/2014JD022730.
- Holten, V., and Van Dongen, M. E. H. (2009). Comparison between Solutions of the General Dynamic Equation and the Kinetic Equation for Nucleation and Droplet Growth. J. Chem. Phys., 130: 014102, 10.1063/1.3054634.
- Jung, J., Adams, P. J., and Pandis, S. N. (2006). Simulating the Size Distribution and Chemical Composition of Ultrafine Particles During Nucleation Events. Atmos. Environ., 40:2248–2259, 10.1016/j.atmosenv.2005.09.082.
- Kerminen, V.-M., and Kulmala, M. (2002). Analytical Formulae Connecting the ‘Real’ and the ‘Apparent’ Nucleation Rate and the Nuclei Number Concentration for Atmospheric Nucleation Events. J. Aerosol Sci., 33:609–622.
- Kerminen, V.-M., Paramonov, M., Anttila, T., Riipinen, I., Fountoukis, C., Korhonen, H., Asmi, E., Laakso, L., Lihavainen, H., Swietlicki, E., Svenningsson, B., Asmi, A., Pandis, S. N., Kulmala, M., and Petäjä, T. (2012). Cloud Condensation Nuclei Production Associated with Atmospheric Nucleation: A Synthesis Based on Existing Literature and New Results. Atmos. Chem. Phys., 12:12037–12059.
- Korhonen, H., Lehtinen, K. E. J., and Kulmala, M. (2004). Multicomponent Aerosol Dynamics Model UHMA: Model Develoment and Validation. Atmos. Chem. Phys., 4:757–771.
- Korhonen, H., Lehtinen, K. E. J., Pirjola, L., Napari, I., Vehkamäki, H., Noppel, M., and Kulmala, M. (2003). Simulation of Atmospheric Nucleation Mode: A Comparison of Nucleation Models and Size Distribution Representations. J. Geophys. Res. D Atmos., 108:AAC 12-1–AAC 12-8.
- Kumar, S., and Ramkrishna, D. (1997). On the Solution of Population Balance Equations by Descretization--III. Nucleation, Growth, and Aggregation of Particles. Chemical Engineering Science, 52(24):4659–4679.
- Kupiainen-Määttä, O., Olenius, T., Korhonen, H., Malila, J., Dal Maso, M., Lehtinen, K., and Vehkamäki, H. (2014). Critical Cluster Size Cannot in Practice be Determined by Slope Analysis in Atmospherically Relevant Applications. J. Aerosol Sci., 77:127–144, 10.1016/j.jaerosci.2014.07.005.
- Lee, Y. H., Pierce, J. R., and Adams, P. J. (2013). Representation of Nucleation Mode Microphysics in a Global Aerosol Model with Sectional Microphysics. Geosci. Model Dev., 6:1221–1232, 10.5194/gmd-6-1221-2013.
- Lehtinen, K. E. J., Dal Maso, M., Kulmala, M., and Kerminen, V.-M. (2007). Estimating Nucleation Rates from Apparent Particle Formation Rates and Vice Versa: Revised Formulation of the Kerminen-KulmalaEquation. J. Aerosol Sci., 38:988–994.
- Lehtinen, K. E. J., and Kulmala, M. (2003). A model for Particle Formation and Growth in the Atmosphere with Molecular Resolution in Size. Atmos. Chem. Phys., 3:251–257.
- Lehtipalo, K., Rondo, L., Kontkanen, J., Schobesberger, S., Jokinen, T., Sarnela, N., Kürten, A., Ehrhart, S., Franchin, A., Nieminen, T., Riccobono, F., Sipilä, M., Yli-Juuti, T., Duplissy, J., Adamov, A., Ahlm, L., Almeida, J., Amorim, A., Bianchi, F., Breitenlechner, M., Dommen, J., Downard, A. J., Dunne, E. M., Flagan, R. C., Guida, R., Hakala, J., Hansel, A., Jud, W., Kangasluoma, J., Kerminen, V.-M., Keskinen, H., Kim, J., Kirkby, J., Kupc, A., Kupiainen-Määttä, O., Laaksonen, A., Lawler, M. J., Leiminger, M., Mathot, S., Olenius, T., Ortega, I. K., Onnela, A., Petäjä, T., Praplan, A., Rissanen, M. P., Ruuskanen, T., Santos, F. D., Schallhart, S., Schnitzhofer, R., Simon, M., Smith, J. N., Tröstl, J., Tsagkogeorgas, G., Tomé, A., Vaattovaara, P., Vehkamäki, H., Vrtala, A. E., Wagner, P. E., Williamson, C., Wimmer, D., Winkler, P. M., Virtanen, A., Donahue, N. M., Carslaw, K. S., Baltensperger, U., Riipinen, I., Curtius, J., Worsnop, D. R., and Kulmala, M. (2016). The Effect of Acid-Base Clustering and Ions on the Growth of Atmospheric Nano-Particles. Nat. Commun., 7:11594, 10.1038/ncomms11594.
- Makkonen, R., Seland, Ø., Kirkevåg, A., Iversen, T., and Kristjánsson, J. E. (2014). Evaluation of Aerosol Number Concentrations in NorESM with Improved Nucleation Parameterization. Atmos. Chem. Phys., 14:5127–5152, 10.5194/acp-14-5127-2014.
- Malila, J., McGraw, R., Laaksonen, A., and Lehtinen, K. E. J. (2015). Communication: Kinetics of Scavenging of Small, Nucleating Clusters: First Nucleation Theorem and Sum Rules. J. Chem. Phys., 142, 10.1063/1.4905213.
- McGrath, M. J., Olenius, T., Ortega, I. K., Loukonen, V., Paasonen, P., Kurtén, T., Kulmala, M., and Vehkamäki, H. (2010). Atmospheric Cluster Dynamics Code: A Flexible Method for Solution of the Birth-Death Equations. Atmospheric Chemistry and Physics, 12(5):2345–2355.
- Merikanto, J., Duplissy, J., Määttänen, A., Henschel, H., Donahue, N. M., Brus, D., Schobesberger, S., Kulmala, M., and Vehkamäki, H. (2016). Effect of Ions on Sulfuric Acid-Water Binary Particle Formation: 1. Theory for Kinetic- and Nucleation-Type Particle Formation and Atmospheric Implications. J. Geophys. Res. Atmos., 121:1736–1751, 10.1002/2015JD023538.
- Merikanto, J., Zapadinsky, E., Lauri, A., and Vehkamäki, H. (2007). Origin of the Failure of Classical Nucleation Theory: Incorrect Description of the Smallest Clusters. Phys. Rev. Lett., 98:145702, 10.1103/PhysRevLett.98.145702.
- Olenius, T., Kupiainen-Määttä, O., Lehtinen, K. E. J., and Vehkamäki, H. (2015). Extrapolating Particle Concentration along the Size Axis in the Nanometer Size Range Requires Discrete Rate Equations. J. Aerosol Sci., 90:1–13, 10.1016/j.jaerosci.2015.07.004.
- Olenius, T., Kupiainen-Määttä, O., Ortega, I. K., Kurtén, T., and Vehkamäki, H. (2013). Free Energy Barrier in the Growth of Sulfuric Acid-Ammonia and Sulfuric Acid-Dimethylamine Clusters. J. Chem. Phys., 139:084312, 10.1063/1.4819024.
- Ramabhadran, T. E., Peterson, T. W., and Seinfeld, J. H. (1976). Dynamics of Aerosol Coagulation and Condensation. AIChE J., 22:840–851.
- Rao, N. P., and McMurry, P. H. (1989). Nucleation and Growth of Aerosol in Chemically Reacting Systems. A theoretical study of the near-collision-controlled regime. Aerosol. Sci. Technol., 11:120–132.
- Reddington, C. L., Carslaw, K. S., Spracklen, D. V., Frontoso, M. G., Collins, L., Merikanto, J., Minikin, A., Hamburger, T., Coe, H., Kulmala, M., Aalto, P., Flentje, H., Plass-Dülmer, C., Birmili, W., Wiedensohler, A., Wehner, B., Tuch, T., Sonntag, A., O'Dowd, C. D., Jennings, S. G., Dupuy, R., Baltensperger, U., Weingartner, E., Hansson, H.-C., Tunved, P., Laj, P., Sellegri, K., Boulon, J., Putaud, J.-P., Gruening, C., Swietlicki, E., Roldin, P., Henzing, J. S., Moerman, M., Mihalopoulos, N., Kouvarakis, G., Ždímal, V., Zíková, N., Marinoni, A., Bonasoni, P., and Duchi, R. (2011). Primary Versus Secondary Contributions to Particle Number Concentrations in the European Boundary Layer. Atmos. Chem. Phys., 11:12007–12036, 10.5194/acp-11-12007-2011.
- Riccobono, F., Schobesberger, S., Scott, C. E., Dommen, J., Ortega, I. K., Rondo, L., Almeida, J., Amorim, A., Bianchi, F., Breitenlechner, M., David, A., Downard, A., Dunne, E. M., Duplissy, J., Ehrhart, S., Flagan, R. C., Franchin, A., Hansel, A., Junninen, H., Kajos, M., Keskinen, H., Kupc, A., Kürten, A., Kvashin, A. N., Laaksonen, A., Lehtipalo, K., Makhmutov, V., Mathot, S., Nieminen, T., Onnela, A., Petäjä, T., Praplan, A. P., Santos, F. D., Schallhart, S., Seinfeld, J. H., Sipilä, M., Spracklen, D. V., Stozhkov, Y., Stratmann, F., Tomé, A., Tsagkogeorgas, G., Vaattovaara, P., Viisanen, Y., Vrtala, A., Wagner, P. E., Weingartner, E., Wex, H., Wimmer, D., Carslaw, K. S., Curtius, J., Donahue, N. M., Kirkby, J., Kulmala, M., Worsnop, D. R., and Baltensperger, U. (2014). Oxidation Products of Biogenic Emissions Contribute to Nucleation of Atmospheric Particles. Science, 344:717–721.
- Seinfeld, J. H., and Pandis, S. N. (2006). Atmospheric Chemistry and Physics: From Air Pollution to Climate Change. John Wiley & Sons, Inc., New York.
- Shi, G., Seinfeld, J. H., and Okuyama, K. (1990). Transient Kinetics of Nucleation. Phys. Rev. A, 41:2101–2108, 10.1103/PhysRevA.41.2101.
- Sihto, S.-L., Vuollekoski, H., Leppä, J., Riipinen, I., Kerminen, V.-M., Korhonen, H., Lehtinen, K. E. J., Boy, M., and Kulmala, M. (2009). Aerosol Dynamics Simulations on the Connection of Sulphuric Acid and New Particle Formation. Atmos. Chem. Phys., 9:2933–2947, 10.5194/acp-9-2933-2009.
- Vehkamäki, H. (2006). Classical Nucleation Theory in Multicomponent Systems. Springer-Verlag, Berlin-Heidelberg.
- Vehkamäki, H., McGrath, M. J., Kurtén, T., Julin, J., Lehtinen, K. E. J., and Kulmala, M. (2012). Rethinking the Application of the First Nucleation Theorem to Particle Formation. J. Chem. Phys., 136:094107.
- Wang, J., McGraw, R. L., and Kuang, C. (2013). Growth of Atmospheric Nano-Particles by Heterogeneous Nucleation of Organic Vapor. Atmos. Chem. Phys., 13:6523–6531, 10.5194/acp-13-6523-2013.
- Warren, D. R., and Seinfeld, J. H. (1984). Nucleation and Growth of Aerosol from a Continuously Reinforced Vapor. Aerosol. Sci. Technol., 3:135–153.
- Yu, F. (2003). Nucleation Rate of Particles in the Lower Atmosphere: Estimated Time Needed to Reach Pseudo-Steady State and Sensitivity to H2SO4 gas Concentration. Geophys. Res. Lett., 30:33–1.
- Zeldovich, J. (1943). On the Theory of New Phase Formation; Cavitation. Acta Physiochim., URSS XVIII, 1/22/01.
- Zhang, R., Khalizov, A., Wang, L., Hu, M., and Xu, W. (2012). Nucleation and Growth of Nanoparticles in the Atmosphere. Chem. Rev., 112:1957–2011.
- Zhang, Y., Seigneur, C., Seinfeld, J. H., Jacobson, M. Z., and Binkowski, F. S. (1999). Simulation of Aerosol Dynamics: A Comparative Review of Algorithms Used in Air Quality Models. Aerosol. Sci. Technol., 31:487–514, 10.1080/027868299304039.