ABSTRACT
Flames generate a large amount of chemically and thermally ionized species, which are involved in the growth dynamics of particles formed in flames. However, existing models predicting particle formation and growth do not consider particle charging, which may lead to bias in the calculated size distribution of particles. In this study, Fuchs' charging theory was coupled with a monodisperse particle growth model to study the simultaneous charging and coagulation of nanoparticles during combustion. In order to quantify the charging characteristics of nanoparticles, a high-resolution DMA was used to measure the mobilities of ions generated from a premixed flat flame operated at various conditions. The effect of temperature on ion–particle and particle–particle combination coefficients was further examined. The proposed model showed that the influence of charging on particle growth dynamics was more prominent when the ion concentration was comparable to or higher than the particle concentrations, a condition that may be encountered in flame synthesis and solid fuel-burning. Simulated results also showed that unipolar ion environments strongly suppressed the coagulation of particles. In the end, a simplified analysis of the relative importance of particle charging and coagulation was proposed by comparing the characteristic time scales of these two mechanisms.
© 2017 American Association for Aerosol Research
EDITOR:
1. Introduction
Combustion is a major source of particulate matter (PM) generation (Biswas and Wu Citation2005). The precise control of aerosol formation during combustion is equally crucial for both the applications of flame-generated particles (Liu et al. Citation2015; Hu et al. Citation2016; Li et al. Citation2016b) and their environmental implications (Wang et al. Citation2013; Li et al. Citation2016a). However, a detailed understanding of the particle dynamics in flames is lacking due to the fast reaction rate of combustion, making it difficult to control the particle formation and growth processes. Due to the limitations of instruments, the particle nucleation and growth at initial stages during combustion, especially below 3 nm, still remain to be a knowledge gap (Wang et al. Citation2014). At the same time, because of chemical ionization and thermal ionization mechanisms (Fialkov Citation1997), flames generate large numbers of ions and charged clusters with concentrations as high as 1010/cm3. A recent study conducted with a series of enhanced particle detectors showed that the fraction of charged species can be high in a premixed flame (Wang et al. Citation2017a). The presence of these ions makes the flame a quasi-neutral plasma that may notably affect the property of the produced particles. The highly concentrated ions and charged clusters actively collide with the formed nanoparticles, adding electrostatic potentials to the system, and further altering the properties of the generated particles, such as their size, shape, and crystallinity (Jiang et al. Citation2007a; Zhang et al. Citation2012). This effect is significantly enhanced when manipulated ion environments (such as unipolar-ion environments and concentrated ion environments) are imposed (Vemury and Pratsinis Citation1995; Citation1996; Vemury et al. Citation1997; Zhang et al. Citation2011; Zhang et al. Citation2012; Xiong et al. Citation2016; Ren et al. Citation2017).
According to Fuchs' charging theory, the particle charging probability is determined by the mass and mobility of the surrounding ions (Fuchs Citation1963). Although it neglects the effect of particle morphology and absolute ion concentrations, Fuchs' charging theory provides a quantitative match with the experimentally measured charging probabilities of particles between 3 nm to 1 μm (Hoppel and Frick Citation1986; Reischl et al. Citation1996; Jiang et al. Citation2007a; Gopalakrishnan et al. Citation2015). Therefore, direct measurement of ion mass and mobility can shed light upon particle charging probabilities in flames. Previously, this type of measurement was difficult due to the low resolution of instruments determining the mobility of highly diffusive ions below 3 nm. With the development of differential mobility analyzers (DMAs) with high sheath flow rates, the residence time for particle diffusion in the instrument is greatly reduced, enhancing the resolution of ion mobility measurements (Fang et al. Citation2014; Wang et al. Citation2014; Wang et al. Citation2015; Kangasluoma et al. Citation2016a; Carbone et al. Citation2016). The coupling of a high-resolution DMA with an atmospheric pressure-inlet mass spectrometer (MS), i.e., tandem DMA-MS, enables the simultaneous measurement of ion mobility and mass, through which the accurate calculation of the interaction between ions and particles becomes achievable (Gopalakrishnan et al. Citation2015; Maißer et al. Citation2015). Apart from creating the ion-particle interactions, the presence of ions in particle systems also modifies the charge distributions of particles, changing the electrostatic potentials among the flame-generated particles and altering the coagulation growth of flame-generated nanoparticles. In existing models predicting particle formation in flames, the influence of particle charging is not evaluated, although the attractive or repulsive forces among particles can vary the coagulation coefficients greatly (Friedlander Citation2000).
In order to study how ions affect particle formation in flames, one needs to solve the particle general dynamic equation by coupling particle charging and coagulation (Jiang et al. Citation2007b). Existing studies have attempted to solve these two mechanisms separately. By assuming particle size distributions remain constant, kinetic equations tracking the concentrations of ions and particles with different charging states were solved based on the ion-particle combination coefficients (Fuchs Citation1963; Reischl et al. Citation1996; López-Yglesias and Flagan Citation2013; Jean et al. Citation2015). There is a plethora of modeling methods predicting particle formation dynamics where particle charging is not considered, including the unimodal model, bimodal model, method of moments, and discrete-sectional model (Wu and Flagan Citation1988; Landgrebe and Pratsinis Citation1990; Tsantilis et al. Citation2002). These models are applicable when there is a large difference between the characteristic times for particle charging and coagulation. However, when the two time scales are similar to each other, such assumptions ignoring certain mechanisms cannot be made, especially in flame systems, where the particle coagulation time may have a broad range depending on the particle concentrations in different scenarios. Few studies to date have investigated simultaneous charging and coagulation during particle formation and growth. Alonso et al. (Citation1998) studied the transient charging and coagulation of nanoparticles in an aerosol charger with constant ion concentration, in order to examine the effect of mean aerosol residence time on the output concentration of charged particles. Fujimoto et al. (Citation2003) calculated the unipolar ion charging and particle coagulation during aerosol formation from titanium isopropoxide thermal decomposition at temperatures below 500°C, and predicted that the particles' average size, standard deviation, and concentration were affected greatly. Recently, Kim et al. (Citation2016) simulated the charging and coagulation of radioactive and non-radioactive particles in the atmosphere and found that the mutual effects of the two mechanisms indeed alter the particle size distribution. These studies demonstrate that simultaneous particle charging and coagulation may be different from particle dynamics predicted by commonly used models.
In this article, the simultaneous charging and coagulation of flame-generated particles by coupling Fuchs' charging theory with a monodisperse model predicting particle growth dynamics was simulated. In order to quantify the particle charging mechanism, a high-resolution DMA was used to measure the mobility distributions of ions generated from a premixed flat flame. Different operating conditions of the flame were applied to study their influence on ion generation. The obtained ion mobilities were used as inputs to the proposed model for calculating particle growth dynamics in the presence of charging, where the effects of initial particle concentration and the polarity of the ions were specifically investigated.
2. Methods
2.1. Experimental setup
shows a schematic diagram of the experimental setup. A premixed flat flame was used in this study to measure ion mobility distributions, due to its high stability, uniformity, and broad usage. A detailed description of the setup can be found elsewhere (Wang et al. Citation2017b). The combustion mixture was composed of methane (CH4, Airgas Inc., Radnor, PA, USA), oxygen (O2, Airgas Inc.), and nitrogen (N2, Airgas Inc.) whose flow rates were controlled by mass flow controllers (MKS Inc., Andover, MA, USA). Before their mobility distributions were examined, the flame-generated ions were sampled with a dilution probe operated at a dilution ratio of 180, with N2 (Airgas Inc.) as the dilution gas. A high-resolution DMA (half mini-type; Fernández de la Mora and Kozlowski Citation2013) was used to measure the mobility of the flame-generated ions. Details of the DMA setting and calibration method (Ude and de la Mora Citation2005) can be found in the online supplemental information (SI). The ion mobility distributions in both polarities can be obtained by scanning the DMA voltage from 0 to ±5 kV. The mobility size of the ions () was then calculated by
[1] where
is the Cunningham slip correction factor,
is the number of charges on the particle,
is the electronic charge,
is the air viscosity, and
is the ion mobility size. It should be noted that the
value calculated with this equation is larger than its actual value (volumetric size) due to the ion-induced dipole created on the surrounding gas molecules (Larriba et al. Citation2011). However, since size information is not needed in calculating the charging characteristics of flame-generated particles, Equation (Equation1
[1] ) is still used to qualitatively show the size of the ions. In this study, it was assumed that all the flame-generated ions carry one charge only, which is typical in combustion systems (Fialkov Citation1997). The concentration of charged clusters was calculated based on the current recorded by the electrometer, as introduced in the SI.
Figure 1. Schematic diagram of the experimental setup, including a premixed flat flame and a high-resolution DMA system.
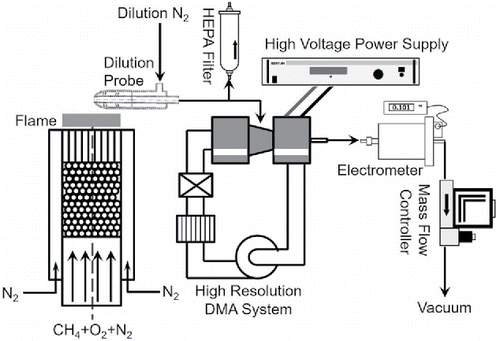
A series of flow rates in the flat flame was used to study the effects of the flame equivalence ratio on flame-generated ions. Additional measurements at different heights above the burner (HAB) were conducted to elucidate the ion properties as a function of residence time after their formation. The detailed flow rates, flame equivalence ratio (), and HAB combinations are listed in . The flow rate settings achieved
values of 0.60, 0.85, 1.00, and 1.10 in Tests 1 through 4. Since changing
can result in different flame speeds and flame positions, N2 flow was adjusted accordingly to maintain a constant height of the flame sheet. also lists the adiabatic flame temperatures under different flow rate settings calculated using the major–minor species model (Turns Citation1996). Note that due to temperature and dilution effects, the sampling at the same HAB does not imply equal residence time in different flames. The ion mobility distributions were measured in a broad range of HAB (5–20 mm), where different characteristics of positive and negative ions were observed. The effect of sampling height on ion size and mobility distribution is discussed in the SI due to the page limit.
Table 1. Experimental plan showing the combination of flow rates, adiabatic flame temperatures, and sampling heights.
2.2. Simulation method
2.2.1. Ion–particle and particle–particle combination coefficients
In order to track simultaneous particle charging and coagulation, combination rates among the ions and particles, which are determined by the ion–particle and particle–particle combination coefficients were calculated.
According to Fuchs' charging theory (1963), the only unknown parameters in calculating the ion–particle combination coefficient are the positive and negative ion mobilities and masses. Ideally, the mobility and mass of an ion should be measured with a tandem DMA-MS setup, where these values are obtained at the same time (Maißer et al. Citation2015; Wang et al. Citation2017b). Due to the instrument limitation, ion mobility was the only parameter measured in this study. Approximate mass values of 140 amu and 70 amu for positive and negative ions were used, which are in the range of existing measurement results obtained with mass spectrometers (Fialkov Citation1997). These atomic mass values also agree with a recent study on the measurement of premixed flat flame-generated ions with an atmospheric pressure-inlet time-of-flight mass spectrometer, where the dominant positive charge carriers were hydrocarbons (100–250 amu) and dominant negative charge carriers were nitrate-related ions (62 amu and 125 amu; Wang et al. Citation2017a). As pointed out by the study of the sensitivity analysis in Fuchs' charging model, the main influential variable is the difference between the positive and negative ion mobilities, which were measured accurately in this study (Tigges et al. Citation2015). Because the DMA-measured flame-generated ions had distributed mobilities, the number-weighted mean mobilities of the positive and negative ions were calculated. Furthermore, since the mobility values were measured at 298 K, which was the sheath flow temperature of the DMA, one needs to convert the measured mobility values to those at other temperatures. Here, a mobility-temperature dependence of was used (Larriba et al. Citation2011). The influence of other mobility-temperature dependences on calculating the ion–particle combination coefficient was discovered to be small (Figure S1). The ion–particle combination coefficient
of positive (+) and negative (−) ions with a particle carrying i elementary units of charge can be derived as
[2]
The particle–particle combination coefficient for the Brownian collision of spherical particles in the free molecular and continuum regime (Seinfeld and Pandis Citation2012) is given by[3]
In the presence of electrostatic interaction between charged particles, the combination coefficient is modified by a correction factor (W) (Friedlander Citation2000), which is given by[4]
Detailed explanations and calculation results of Equations Equation(2)–(4) are included in the SI.
2.2.2. Calculation of simultaneous particle charging and coagulation
Using the ion–particle and particle–particle combination coefficients, the detailed equations describing the concentrations of ions and particles with different charging states can be written. The following differential equations track the ion number concentration (), particle number concentration (
), and total particle volume concentration (
) at any time:
[5]
In this equation, k is the number of charges carried by each particle, is the generation rate of ions,
is the ion recombination coefficient, and
is the ion-particle combination coefficient calculated in Equation (Equation2
[2] ). The three terms on the right-hand side (RHS) of Equation (Equation5
[5] ) correspond to the rate of ion generation, the loss of ions due to recombination, and the loss of the ions due to collision with particles, respectively. When solving Equation (Equation5
[5] ), for simplicity, the rate of ion generation was set to 0 due to the thinness of the reaction zone (flat flame sheet) in combustion, and the initial concentration of ions was set to be 1010/cm3, based on a summary of existing measurements of ion concentrations in flames (Fialkov Citation1997; Kim et al. Citation2005; Alquaity et al. Citation2016). As illustrated in previous studies, the change of fuel type and combustion equivalence ratio will affect the ion concentrations, however, the influence was not strong, where the ion concentrations were in the range of 109 to 1011/cm3. It reveals that the ion concentrations in the flame may be an intrinsic value, which may not be very sensitive to flame conditions due to combustion chemistries. Regarding the ion recombination, theoretically, since the masses and mobilities of electrons and negatively charged clusters were significantly different from each other, a complete model should consider this difference in recombination coefficients. However, due to the difficulty in retrieving the data on recombination coefficients between positive ions and elections, in this model, the recombination was considered to take place between positively and negatively charged clusters only, and a constant value of
cm3/s obtained in various combustion studies was used (Fialkov Citation1997).
increases as temperature decreases, since the attractive electrostatic potential start to dominate over kinetic movement of ions with opposite polarities. In this study, the influence of using larger
values at lower temperatures was determined to be minimal. The number concentration and total volume of particles carrying k charges are described by
[6]
[7]
[8]
In these equations, is the volume of each particle with charge
,
is the particle–particle combination coefficient calculated with Equations (Equation2
[2] ) and (Equation4
[4] ), and
is the mobility of particles with
charges. In both equations, the first and second terms on the RHS together account for the gain of particles by ion–particle collision. The third and fourth terms together account for loss of particles by ion–particle collision. The fifth term represents the gain of particles through the coagulation of charged particles. The sixth term accounts for the loss of particles through coagulation, and the seventh term accounts for the loss of particles due to electrostatic dispersion. Note that m, the highest charging state of particles, is assumed to take values between −4 and +4 in this study.
The simulation assumes a constant temperature environment (300, 600, 1200, or 2400 K) synthesizing TiO2 nanoparticles, and records the particle size and the concentrations of ions and particles as a function of time. It should be noted that in flame systems, the flow field and temperature field are not uniform, so the assumption of a constant temperature may not be valid. However, in premixed flat flames, the one-dimensionality and uniformity of the flame makes it similar to a plug flow reactor, where investigating particle charging and coagulation at specific temperatures is meaningful.
Equations Equation(5)–(8) represent a population balance on the ion concentration, particle number concentration, and particle volume concentration, respectively. This system of coupled ordinary differential equations is non-dimensionalized and solved using the VODE solver in Python with backward differentiation formulas (BDF). At any time , this monodisperse model assumes one size mode for each charge,
, calculated by Equation (Equation8
[8] ), instead of a particle size distribution (Vemury et al. Citation1997). The schematic of the model is shown in . This model is found to be in good agreement with the predictions of highly accurate aerosol dynamics model. The slight error that is contributed by the overestimation of mean particle diameter and underestimation of the particle number concentration is inconsequential (Landgrebe and Pratsinis Citation1990). Both bipolar and unipolar ion environments were simulated, since flame synthesis under these conditions can be achieved (Vemury and Pratsinis Citation1995; Citation1996; Vemury et al. Citation1997). A baseline group that calculates particle dynamics with coagulation only was also conducted for comparing the results. A total simulated time of 1 s was used, since in flame systems, the residence time of particles in a specific temperature zone is less than 1 s. For the simulations performed, the total number concentration of particles at any time
,
is the sum of all the positively charged, negatively charged, and neutral particles:
[9]
Figure 2. Schematic diagram of the monodisperse model for charge () from −2 to +2. For each charge there is one mode represented by
and
. The arrow pointing toward right indicates that as time goes by coagulation leads to increase in size for all the modes. Note that the size (
) may be different for different values of
.
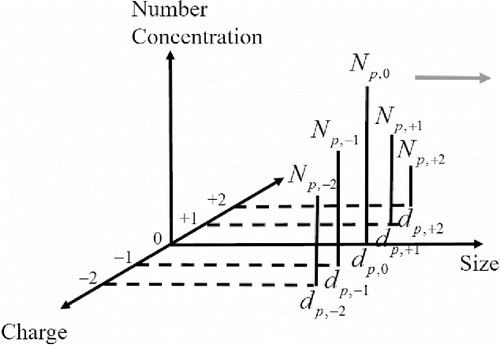
The average diameter of particles at any time ,
is defined as
[10]
The average charge on the particles at any time ,
is calculated as
[11]
lists the parameters and their values used in the simulation. Different initial particle concentrations were used to study influence of initial particle-to-ion concentration ratio on particle growth, since in real situations, the initial particle concentration in a flame can be easily changed by adjusting the precursor concentration. The initial particle size was assumed to be 0.4 nm, which is the diameter of a TiO2 molecule (Tsantilis et al. Citation2002). Furthermore, particles were set to be neutral at the initial time.
Table 2. Parameters used in the simulation of particle simultaneous charging and coagulation.
3. Results and discussion
3.1. Size and mobility distribution of flame-generated ions
displays the size and mobility distributions of positive and negative ions generated from flames operated at different equivalence ratios (). The high-resolution DMA detected characteristic peaks at 1.04, 1.10, and 1.27 nm in positive ions and at 1.01 and 1.06 nm in negative ions. These charged particles were believed to come from the intensive chemical ionization in the studied flames, where the temperatures were not high enough to generate charged species through thermal ionization (Fialkov Citation1997; Jiang et al. Citation2007b; Wang et al. Citation2017). One can also notice that the measured ion sizes are larger than those expected from clusters composed of a few atoms. The mobility size shown in the size distributions is inherently larger than the volumetric size of a charged cluster due to the ion-induced dipole effect, which pose a stronger drag force on charged cluster. Recent studies (Wang et al. Citation2017a,b) also indicate that the major peak (1.01 nm) in the negative ion size distributions is mainly composed of nitrate ions (
), which has an ionic radii of 0.179 nm (Volkov et al. Citation1997). Hence, further study with a mass spectrometer is needed in order to determine the exact chemical composition of the measured ions and charged clusters. On the other hand, the relatively large mobility size might be caused by the limitation of the sampling system, where the rapid quenching not only stopped particle dynamics, but also altered the chemical equilibriums, introducing new species to the measurement (Carbone et al. Citation2016). During CH4 combustion, the chemically produced positively and negatively charged species mainly come from two reactions:
[12]
[13] where the formed ions and electrons collide with other molecules to form more stable charged species. According to recent work by Jones and Hayhurst (Citation2016), the reaction shown in Equation (Equation12
[12] ) dominates in fuel-lean combustion of methane (C/O ≤ 0.4). A pool of positive ions is then formed rapidly, mainly through proton transfer from
to other intermediates and final products of combustion. The average mobility of negative ions was higher than that of positive ions, possibly because the positive ions were composed of organic species, which had higher proton affinities. As the flame transited from fuel-lean to fuel-rich, the concentration of the detected ions first increased and then decreased. The increase in ion concentration was caused by the stronger chemical ionization processes in the flame, while the decrease was a result of depletion of free oxygen atoms in the reaction zone (Jones and Hayhurst Citation2016). It also shows that the number concentration of positive ions is higher than that of negative ions. According to charge neutrality, this result suggests that electrons may serve as a major species of negative charge carriers. The presence of highly mobile electrons can lead to a higher fraction of particles being negatively charged. The chemical species of these stable intermediates did not change as a function of
(as indicated by the same peak location), except that the relative magnitude of these charged species differed. For example, at
, the concentration of the larger positive ions (1.10 and 1.27 nm) was higher than that at
, while the concentration of 1.04 nm positive ions was lower than that at
, implying a conversion from smaller to larger ions as
increased. However, as indicated in existing studies conducted with molecular beam mass spectrometry, these charged species may alter as
further increases to sooting conditions (Jones and Hayhurst Citation2016). Based on the measurement results, the positive and negative ion mobilities used for calculating ion–particle combination coefficients were 1.54 cm2/Vs and 1.97 cm2/Vs, by averaging the mobility distributions measured at
and HAB = 5 mm.
3.2. Simultaneous particle charging and coagulation
Using the calculated ion–particle and particle–particle combination coefficients (Figures S2 and S3), particle and ion concentrations are analyzed with the population balance equations (Equations Equation(5)–(8)). The results of simultaneous particle charging and coagulation in the unipolar and bipolar ion environments are shown as follows.
3.2.1. Unipolar ion environment
and show the change of particle average size and concentration as a function of time in a unipolar ion environment with different initial particle-to-ion ratios. The effect of the unipolar ion environment on particle growth is very prominent. Due to particle charging, the coagulation among unipolarly charged particles is significantly suppressed. In all the calculated cases, the average particle size first increases and then reaches a plateau where particle size stops increasing (). Similar results were observed in existing experimental studies when unipolar ions were introduced into flames synthesizing nanoparticles: The electrostatic repulsion dispersed the synthesized particles, and a monodisperse product was obtained (Vemury and Pratsinis Citation1995). The point where particle size stopped increasing was determined by the relative concentrations of particles and ions, which is shown more directly in . The particle concentration stops decreasing when it is comparable to the initial ion concentration ( and ). Hence, creating unipolar ion environments during flame synthesis of nanoparticles can effectively suppress particle growth, and the size of the synthesized particles can be controlled by manipulating the relative concentrations of unipolar ions and particles.
Figure 4. Evolution of average particle size as a function of time at different temperatures, with and without charging effects in a unipolar ion environment. Subplots represent initial particle-to-ion concentration ratios of (a) 0.01, (b) 1, (c) 100, and (d) 1000. Note the different scales of x-axes and y-axes.
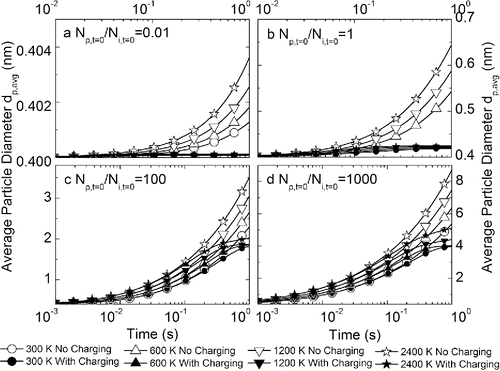
Figure 5. Evolution of normalized particle number concentration as a function of time at different temperatures, with and without charging effects in a unipolar ion environment. Subplots represent the cases with initial particle-to-ion concentration ratios of (a) 0.01, (b) 1, (c) 100, and (d) 1000. Note the different scales of x-axes and y-axes.
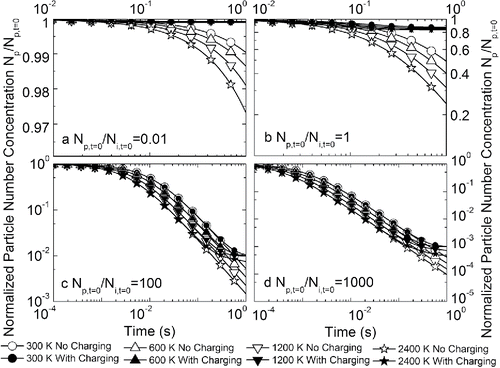
3.2.2. Bipolar ion environment
shows the evolution of average particle size as a function of time in a bipolar ion environment. When the initial particle concentration is low (, ), minimal particle growth is observed after a simulated time of 1 s. The bipolar ion environment is found to enhance particle growth, especially at higher temperatures. This finding also agrees with atmospheric studies on ion-induced nucleation, where the participation of ions plays a more significant role when the molecular cluster concentration is lower (Laakso et al. Citation2002; Kangasluoma et al. Citation2016b). The effect of a bipolar ion environment is less important or even negligible when the initial particle concentration is comparable to or higher than the initial ion concentration (). The limited effect of bipolar ions is a result of ion recombination, which quickly consumes all the available ions. As the initial particle concentration increases, the grown particle size also becomes larger, reaching 9 nm at a temperature of 2400 K after 1 s. The evolution of normalized particle concentration as a function of time with different initial particle-to-ion concentration ratios is displayed in Figure S5, which corresponds well with the results on particle size, showing that the initial particle-to-ion concentration determines the influence of bipolar ion environment.
Figure 6. Evolution of average particle size as a function of time at different temperatures, with and without charging effects in a bipolar ion environment. Subplots represent initial particle-to-ion concentration ratios of (a) 0.01, (b) 1, (c) 100, and (d) 1000. Note the different scales of x-axes and y-axes.
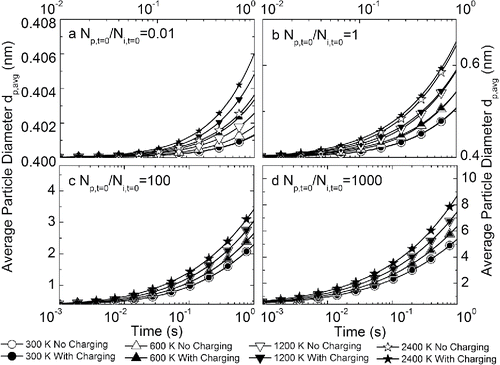
Due to the continuous chemical ionization in certain flames, the ion concentrations may remain relatively constant during a period of time before the quick consumption of ions through recombination. To simulate such a system, it was assumed that the ion concentrations stay constant (1010/cm3) during a period of 10 ms. Different initial particle concentrations in the flame were simulated to understand the effect of a constant ion presence on the particle dynamics. In , the ratio of final particle sizes obtained with and without charging (), and the average charge on each particle (
) are plotted against the initial particle-to-ion concentration ratios. As particle concentration increases, initially,
increases due to the similar concentrations of positively and negatively charged particles at small sizes, resulting in attractive forces among particles. Note that an average negative charge is developed, due to the higher negative ion–particle combination coefficients caused by the larger mobilities of negative ions. As the initial particle concentration further increases, the particle size becomes larger through coagulation, and the difference between negatively and positively charged particle concentrations also becomes larger. Hence, the average negative charge increases to a higher value, causing a decrease of
. This result indicates that, during simultaneous particle charging and coagulation with a constant ion concentration, initially, the attractive forces between oppositely charged particles play a more important role in influencing the particle size. Gradually, as the average charge increases, repulsive forces dominate, and therefore the average particle size drops compared to the coagulation-only case. This phenomenon is, to some extent, similar to the screening effect in aquatic environments. Similar results were obtained in a bipolar ion environment with higher ion concentrations, as shown in Figure S6, where a constant ion concentration of 1012/cm3 is applied.
3.3. Time scale analysis of particle charging and coagulation
The competition between particle charging and coagulation can be simplified by comparing the characteristic time scales for these two processes. According to Equation (Equation6[6] ), initially, since all particles are neutral and have the same size, the decrease in neutral particle concentration is mainly caused by ion collisions with particles and particle self-coagulation. Additionally, due to the similar magnitude of the positive and negative ion–particle combination coefficients, Equation (Equation6
[6] ) can be simplified as
[14]
Hence, two characteristic time scales can be derived, where the characteristic charging time can be derived as , and the characteristic coagulation time can be derived as
. The ratio of these two time scales (
) further determines the relative sequence of the two physical processes.
is plotted as a function of particle size with different initial particle-to-ion concentration ratios (
) in . As particle size increases, the collision-cross-section area of particles also increases, leading to a faster charging process, but the effect of the enlarged collision-cross-section area on particle coagulation is counteracted by the smaller particle diffusion coefficient. Hence, introducing ions to particles with larger sizes can cause a stronger influence on particle growth dynamics. The particle-to-ion concentration ratio significantly affects the temporal sequence of charging and coagulation, especially when particle size is smaller than 10 nm, which has already been shown in to . When the particle-to-ion concentration ratio is 1, charging takes place significantly earlier than coagulation, which can induce or suppress particle growth, depending on the ion environment. When the particle-to-ion concentration ratio is 104, particle coagulation is much faster than particle charging, so particles can grow to a relatively larger size before the electrostatic potential begins to play a role. This effect is more evident in a unipolar ion environment, where particles grow until the concentration of ions and particles are comparable (). No noticeable difference in
is observed when system temperature increased from 300 K to 2400 K, since as temperature increases, both the ion–particle and particle–particle combination coefficients increase. By comparing the relative magnitudes of the characteristic charging and coagulation time, it can be concluded that particle size and the initial particle-to-ion concentration determine the influence of flame-generated ions on the simultaneous charging and coagulation of nanoparticles, and this influence becomes more significant when particle size or ion concentration is larger.
Figure 8. Ratio of characteristic charging time over coagulation time as a function of particle size at particle-to-ion concentration ratios of 1, 102, and 104. The ratio of the characteristic time determines the sequence of the two mechanisms. When , coagulation takes place much earlier than charging. When
, charging is faster than coagulation.
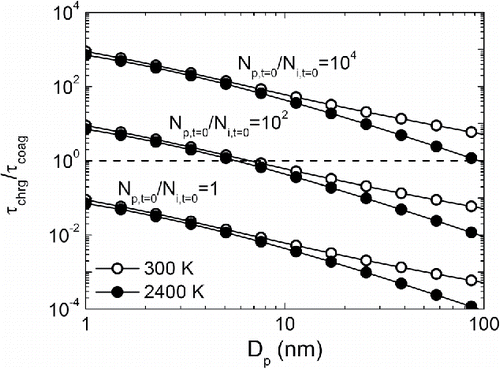
4. Conclusions
By coupling Fuchs' charging theory with a monodisperse particle dynamics model, the simultaneous charging and coagulation of nanoparticles was simulated at various temperatures. As input values for Fuchs' charging theory, the mobility distributions of flame-generated ions were measured with a high-resolution differential mobility analyzer (DMA). Measurements showed that positive ions had smaller mobilities than negative ions, which resulted in higher probabilities of nanoparticles carrying negative charges. Characteristic peaks at 1.04, 1.10, and 1.27 nm in positive ions and at 1.01 and 1.06 nm in negative ions were detected by the high-resolution DMA. The modeling of particle dynamics with simultaneous charging and coagulation showed that the effect of ion interaction was more prominent in unipolar ion environments, where particle coagulation is significantly suppressed. The presence of bipolar ions promoted particle growth only when the particle concentration was lower than the ion concentrations. A simplified model using time scale analysis was proposed, suggesting that the initial particle-to-ion concentration and particle size were two important factors that determine the relative importance of particle charging and coagulation.
UAST_1304635_Supplementary_File.zip
Download Zip (1.1 MB)Funding
This work is supported by the Solar Energy Research Institute for India and the United States (SERIIUS), funded jointly by the U.S. Department of Energy (Office of Science, Office of Basic Energy Sciences, and Energy Efficiency and Renewable Energy, Solar Energy Technology Program, under Subcontract DE-AC36-08GO28308 to the National Renewable Energy Laboratory, Golden, Colorado) and the Government of India, through the Department of Science and Technology under Subcontract IUSSTF/JCERDC-SERIIUS/2012. GS would also like to acknowledge the McDonnell International Academy at Washington University in St. Louis for their support.
References
- Alonso, M., Hashimoto, T., Kousaka, Y., Higuchi, M., and Nomura, T. (1998). Transient Bipolar Charging of a Coagulating Nanometer Aerosol. J. Aerosol Sci., 29:263–270.
- Alquaity, A. B., Han, J., Chahine, M., Selim, H., Belhi, M., Sarathy, S. M., Bisetti, F., and Farooq, A. (2016). Measurements of Positively Charged Ions in Premixed Methane-Oxygen Atmospheric Flames. Combust. Sci. Technol., 189(4):575–594.
- Biswas, P., and Wu, C.-Y. (2005). Nanoparticles and the Environment. J. Air Waste Manage. Assoc., 55:708–746.
- Carbone, F., Attoui, M., and Gomez, A. (2016). Challenges of Measuring Nascent Soot in Flames as Evidenced by High-resolution Differential Mobility Analysis. Aerosol Sci. Technol., 50:740–757.
- Fang, J., Wang, Y., Attoui, M., Chadha, T. S., Ray, J. R., Wang, W.-N., Jun, Y.-S., and Biswas, P. (2014). Measurement of sub-2 nm Clusters of Pristine and Composite Metal Oxides During Nanomaterial Synthesis in Flame Aerosol Reactors. Anal. Chem., 86:7523–7529.
- Fernández de la Mora, J., and Kozlowski, J. (2013). Hand-held Differential Mobility Analyzers of High Resolution for 1-30nm Particles: Design and Fabrication Considerations. J. Aerosol Sci., 57:45–53.
- Fialkov, A. B. (1997). Investigations on Ions in Flames. Prog. Energy Combust. Sci., 23:399–528.
- Friedlander, S. K. (2000). Smoke, Dust, and Haze: Fundamentals of Aerosol Behavior. Oxford University Press, New York, USA.
- Fuchs, N. (1963). On the Stationary Charge Distribution on Aerosol Particles in a Bipolar Ionic Atmosphere. Geofis. Pura Appl., 56:185–193.
- Fujimoto, T., Kuga, Y., Pratsinis, S. E., and Okuyama, K. (2003). Unipolar Ion Charging and Coagulation During Aerosol Formation by Chemical Reaction. Powder Technol., 135:321–335.
- Gopalakrishnan, R., McMurry, P. H., and Hogan Jr, C. J. (2015). The Bipolar Diffusion Charging of Nanoparticles: A Review and Development of Approaches for Non-spherical Particles. Aerosol Sci. Technol., 49:1181–1194.
- Hoppel, W. A., and Frick, G. M. (1986). Ion-Aerosol Attachment Coefficients and the Steady-State Charge Distribution on Aerosols in a Bipolar Ion Environment. Aerosol Sci. Technol., 5:1–21.
- Hu, Y., Jiang, H., Li, Y., Wang, B., Zhang, L., Li, C., Wang, Y., Cohen, T., Jiang, Y., and Biswas, P. (2016). Engineering the Outermost Layers of TiO2 Nanoparticles Using in Situ Mg Doping in a Flame Aerosol Reactor. AIChE J, 63:870–880.
- Jean, L., Swanson, J. J., and Boies, A. M. (2015). Unsteady Bipolar Diffusion Charging in Aerosol Neutralisers: A Non-dimensional Approach to Predict Charge Distribution Equilibrium Behaviour. J. Aerosol Sci., 86:55–68.
- Jiang, J., Hogan Jr., C. J., Chen, D.-R., and Biswas, P. (2007a). Aerosol Charging and Capture in the Nanoparticle Size Range (6–15nm) by Direct Photoionization and Diffusion Mechanisms. J. Appl. Phys., 102:034904.
- Jiang, J., Lee, M.-H., and Biswas, P. (2007b). Model for Nanoparticle Charging by Diffusion, Direct Photoionization, and Thermionization Mechanisms. J. Electrostat., 65:209–220.
- Jones, H. R., and Hayhurst, A. N. (2016). Measurements of the Concentrations of Positive and Negative Ions Along Premixed Fuel-Rich Flames of Methane and Oxygen. Combust. Flame, 166:86–97.
- Kangasluoma, J., Attoui, M., Korhonen, F., Ahonen, L., Siivola, E., and Petäjä, T. (2016a). Characterization of a Herrmann Type High Resolution Differential Mobility Analyzer. Aerosol Sci. Technol., 50:222–229.
- Kangasluoma, J., Samodurov, A., Attoui, M., Franchin, A., Junninen, H., Korhonen, F., Kurtén, T., Vehkamäki, H., Sipilä, M., and Lehtipalo, K. (2016b). Heterogeneous Nucleation onto Ions and Neutralized Ions: Insights into Sign-Preference. J. Phys. Chem. C, 120:7444–7450.
- Kim, S. H., Woo, K. S., Liu, B. Y. H., and Zachariah, M. R. (2005). Method of Measuring Charge Distribution of Nanosized Aerosols. J. Colloid Interf. Sci., 282:46–57.
- Kim, Y.-H., Yiacoumi, S., Nenes, A., and Tsouris, C. (2016). Charging and Coagulation of Radioactive and Nonradioactive Particles in the Atmosphere. Atmos. Chem. Phys., 16:3449–3462.
- Laakso, L., Mäkelä, J. M., Pirjola, L., and Kulmala, M. (2002). Model Studies on Ion-Induced Nucleation in the Atmosphere. J. Geophys. Res., 107:4427.
- Landgrebe, J. D., and Pratsinis, S. E. (1990). A Discrete-Sectional Model for Particulate Production by Gas-phase Chemical Reaction and Aerosol Coagulation in the Free-molecular Regime. J. Colloid Interf. Sci., 139:63–86.
- Larriba, C., Hogan, C. J. Jr, Attoui, M., Borrajo, R., Garcia, J. F., and de la Mora, J. F. (2011). The Mobility-Volume Relationship Below 3.0 nm Examined by Tandem Mobility-Mass Measurement. Aerosol Sci. Technol., 45:453–467.
- Li, Q., Li, X., Jiang, J., Duan, L., Ge, S., Zhang, Q., Deng, J., Wang, S., and Hao, J. (2016a). Semi-Coke Briquettes: Towards Reducing Emissions of Primary PM2. 5, Particulate Carbon, and Carbon Monoxide from Household Coal Combustion in China. Sci. Rep., 6:19306.
- Li, S., Ren, Y., Biswas, P., and Stephen, D. T. (2016b). Flame Aerosol Synthesis of Nanostructured Materials and Functional Devices: Processing, Modeling, and Diagnostics. Progress Energy Combust. Sci., 55:1–59.
- Liu, P., Arnold, I. J., Wang, Y., Yu, Y., Fang, J., Biswas, P., and Chakrabarty, R. K. (2015). Synthesis of Titanium Dioxide Aerosol Gels in a Buoyancy-Opposed Flame Reactor. Aerosol Sci. Technol., 49:1232–1241.
- López-Yglesias, X., and Flagan, R. C. (2013). Ion–Aerosol Flux Coefficients and the Steady-State Charge Distribution of Aerosols in a Bipolar Ion Environment. Aerosol Sci. Technol., 47:688–704.
- Maißer, A., Thomas, J. M., Larriba-Andaluz, C., He, S., and Hogan, C. J. (2015). The Mass-Mobility Distributions of Ions Produced by a Po-210 Source in Air. J. Aerosol Sci., 90:36–50.
- Reischl, G., Mäkelä, J., Karch, R., and Necid, J. (1996). Bipolar Charging of Ultrafine Particles in the Size Range Below 10 nm. J. Aerosol Sci., 27:931–949.
- Ren, Y., Li, S., Cui, W., Zhang, Y., and Ma, L. (2017). Low-Frequency AC Electric Field Induced Thermoacoustic Oscillation of a Premixed Stagnation Flame. Combust. Flame., 176:479–488.
- Seinfeld, J. H., and Pandis, S. N. (2012). Atmospheric Chemistry and Physics: From Air Pollution to Climate Change. John Wiley & Sons, New York.
- Tigges, L., Jain, A., and Schmid, H.-J. (2015). On the Bipolar Charge Distribution used for Mobility Particle Sizing: Theoretical Considerations. J. Aerosol Sci., 88:119–134.
- Tsantilis, S., Kammler, H., and Pratsinis, S. (2002). Population Balance Modeling of Flame Synthesis of Titania Nanoparticles. Chem. Eng. Sci., 57:2139–2156.
- Turns, S. R. (1996). An Introduction to Combustion. McGraw-hill, New York.
- Ude, S., and De la Mora, J. F. (2005). Molecular Monodisperse Mobility and Mass Standards from Electrosprays of Tetra-Alkyl Ammonium Halides. J. Aerosol Sci., 36:1224–1237.
- Vemury, S., and Pratsinis, S. E. (1995). Corona-Assisted Flame Synthesis of Ultrafine Titania Particles. Appl. Phys. Lett., 66:3275–3277.
- Vemury, S., and Pratsinis, S. E. (1996). Charging and Coagulation During Flame Synthesis of Silica. J. Aerosol Sci., 27:951–966.
- Vemury, S., Pratsinis, S. E., and Kibbey, L. (1997). Electrically Controlled Flame Synthesis of Nanophase TiO2, SiO2, and SnO2 Powders. J. Mater. Res., 12:1031–1042.
- Volkov, A., Paula, S., and Deamer, D. (1997). Two Mechanisms of Permeation of Small Neutral Molecules and Hydrated Ions Across Phospholipid Bilayers. Bioelectroch. Bioener., 42:153–160.
- Wang, X., Williams, B., Tang, Y., Huang, Y., Kong, L., Yang, X., and Biswas, P. (2013). Characterization of Organic Aerosol Produced During Pulverized Coal Combustion in a Drop Tube Furnace. Atmos. Chem. Phys., 13:10919–10932.
- Wang, Y., Fang, J., Attoui, M., Chadha, T. S., Wang, W.-N., and Biswas, P. (2014). Application of Half Mini DMA for Sub 2 nm Particle Size Distribution Measurement in an Electrospray and a Flame Aerosol Reactor. J. Aerosol Sci., 71:52–64.
- Wang, Y., Kangasluoma, J., Attoui, M., Fang, J., Junninen, H., Kulmala, M., Petäjä, T., and Biswas, P. (2017a). The High Charge Fraction of Flame-generated Particles in the Size Range below 3 nm Measured by Enhanced Particle Detectors. Combust. Flame., 176:72–80.
- Wang, Y., Kangasluoma, J., Attoui, M., Fang, J., Junninen, H., Kulmala, M., Petäjä, T., and Biswas, P. (2017b). Observation of Incipient Particle Formation During Flame Synthesis by Tandem Differential Mobility Analysis-Mass Spectrometry (DMA-MS). Proc. Combust. Inst., 36(1):745–752.
- Wang, Y., Liu, P., Fang, J., Wang, W.-N., and Biswas, P. (2015). Kinetics of sub-2 nm TiO2 Particle Formation in an Aerosol Reactor During Thermal Decomposition of Titanium Tetraisopropoxide. J. Nanopart. Res., 17:1–13.
- Wu, J. J., and Flagan, R. C. (1988). A Discrete-Sectional Solution to the Aerosol Dynamic Equation. J. Colloid Interf. Sci., 123:339–352.
- Xiong, G., Kulkarni, A., Dong, Z., Li, S., and Stephen, D. T. (2016). Electric-Field-Assisted Stagnation-Swirl-Flame Synthesis of Porous Nanostructured Titanium-Dioxide Films. Proc. Combust. Inst., 36(1):1065–1075.
- Zhang, Y., Li, S., Yan, W., and Stephen, D. T. (2012). Effect of Size-Dependent Grain Structures on the Dynamics of Nanoparticle Coalescence. J. Appl. Phys., 111:124321.
- Zhang, Y., Li, S., Yan, W., Yao, Q., and Stephen, D. T. (2011). Role of Dipole-Dipole Interaction on Enhancing Brownian Coagulation of Charge-neutral Nanoparticles in the Free Molecular Regime. J. Chem. Phys., 134:084501.