ABSTRACT
Aerosol particles are formed by chemical transformations in diverse systems including the atmosphere, fossil fuel combustors, aerosol synthesis reactors, and semiconductor processing equipment. This article discusses solutions to the aerosol population balance equations that account for nucleation, coagulation, wall deposition, scavenging by preexisting particles, and dilution with particle-free air in spatially homogeneous systems when a condensing species is produced by gas phase reactions at a constant rate. Two nucleation mechanisms are considered: classical nucleation due to competing rates of condensation and evaporation, and chemical nucleation due to acid-base reactions. The equations, which apply to a single component system (two components, the acid and base, are included for acid-base nucleation), are cast in a dimensionless form. This leads to dimensionless parameters (dimensionless rate constants) that characterize the importance of each process. When these parameters are sufficiently small, the corresponding process (scavenging by pre-existing particles, wall deposition, dilution, and cluster evaporation) has an insignificant effect and nucleation approaches the collision-controlled limit. Because the dimensionless parameters vary inversely with the square root of the reaction rate, the collision-controlled limit is reached for any chemical system provided the reaction rate is high enough. The numerical solutions quantify the effects of each process for low rates of gas-to-particle conversion where the dimensionless parameters become sufficiently large. They also illustrate how data for sub 10 nm number distributions can provide insights into the nucleation process.
© 2017 American Association for Aerosol Research
EDITOR:
Introduction
Certain gases react to produce nonvolatile particulate products. For example, in the atmosphere, sulfur dioxide (SO2) reacts with hydroxyl to form sulfates, and complex reaction pathways involving many organic precursors can also lead to particle formation (Kirkby et al. Citation2016; Seinfeld and Pandis Citation2016; Tröstl et al. Citation2016). Silane and other gases react to form contaminant particles in semiconductor processing equipment (Swihart and Girshick Citation1999), and titanium tetrachloride is used to produce catalytic particles in flame synthesis reactors (Koirala et al. Citation2016). In such systems, particles can nucleate from trace gas phase constituents formed by chemical reaction and can subsequently grow by condensation and coagulation. Other processes, such as loss to preexisting particles, deposition on reactor walls, entrainment of particle free air, cluster evaporation, etc., can also affect the number distributions of the nucleated aerosol. This article discusses solutions to the aerosol population balance equations pertinent to such processes.
There is a rich and growing body of work aimed at understanding the detailed chemical processes responsible for aerosol formation in reacting systems. This work shows that multiple species often contribute, and that particle mass can be formed by reactions that occur in the gas phase and on or within particles. Rather than attempting to describe the behavior of a particular system in all its complexity, the objective of this article is to obtain general solutions constrained by well-defined simplifying assumptions. In particular, we assume that only a single chemical species contributes to particle formation (two species, sulfuric acid and a basic gas, are considered for acid-base nucleation), and that this species is formed at a constant rate R . These may be good assumptions for aerosol formation in some chemical systems, such as the nucleation of sulfuric acid with a strong basic gas. For chemically more complex systems, such as atmospheric secondary organic aerosols, deviations between observations and our theoretical predictions may provide some insight into types of processes that are important.
This article builds on early work by the senior author (PHM), beginning with McMurry (Citation1980). That work focused on interpreting the pioneering measurements done by William Clark (Clark Citation1972; Clark and Whitby Citation1975), a doctoral student of the late Prof. Kenneth T. Whitby. Using the Whitby Aerosol Analyzer (WAA) (Whitby and Clark Citation1966), Clark measured time-dependent size distributions of 4 − 400 nm aerosol particles formed photochemically from SO2 in a 17.7 m3 Teflon film reactor. Although the air used in these experiments almost certainly contained unmeasured organic contaminants that contributed to aerosol formation, sulfates probably dominated since the concentrations of SO2 were very high (49–2,880 ppb). It probably also contained significant concentrations of stabilizing compounds, such as ammonia or amines. McMurry's analysis assumed that a single chemical species was responsible for nucleation and particle growth. While McMurry (Citation1980) accounted for all possible cluster and particle coagulation interactions, he assumed that evaporation rates were negligible relative to condensation rates (i.e., that nucleation was “collision-controlled”). Furthermore, he showed that if a single condensing species is formed at a constant rate R, the population balance equations can be cast in a nondimensional form that is independent of . With these simplifications McMurry was able to predict observed number distributions and concentrations with to within about 50%. This “zero activation energy” approach (referred to as “spinodal decomposition” by the nucleation community) greatly simplifies nucleation modeling, since the primary uncertainties in nucleation theory involve modeling cluster stability (evaporation rates). Recent work from the CLOUD consortium involving the photooxidation of SO2 in the presence of dimethyl amine (DMA) showed that “neutral clusters containing up to 14 SA (sulfuric acid) and 16 DMA molecules form at or close to the kinetic limit, limited only by the collision rate of SA molecules” (Kürten et al. Citation2014), confirming McMurry's Citation1980 hypothesis. Atmospheric observations also show that particle formation rates often vary in proportion to [H2SO4]2 (Weber et al. Citation1996; Kuang et al. Citation2008), a result consistent with collision-controlled nucleation. Sihto et al. (Citation2006) and Riipinen et al. (Citation2007) found that particle formation rates vary as [H2SO4]p (1<p<2), which led to their conclusion that nucleation may either be limited by collisions of sulfuric acid with itself (p = 2) or with an organic compound (p = 1). Our analysis in this article does not address the latter possibility.
Rao and McMurry (Citation1989) incorporated wall losses of sulfuric acid “monomer” and nanometer-sized particles in the nondimensional framework described above. Diffusion-limited wall deposition rates of these small particles were determined using the theory developed by Crump and Seinfeld (Citation1981) and widely used by others including Kürten et al. (Citation2014). They also accounted for cluster evaporation, assuming that evaporation rates could be predicted with the Kelvin equation (Friedlander Citation2000). This allowed them to study the transition from collision-controlled to condensation/evaporation-controlled nucleation. In this article, our treatment of wall losses and classical condensation/evaporation-controlled nucleation is identical to that introduced by Rao and McMurry (Citation1989), although our analysis is expanded to include other processes that might occur simultaneously. Independently, Lushnikov and Kulmala (Citation1995) described a nondimensional solution for “source-enhanced” classical nucleation that accounted for the chemical production of condensing vapor. Their analysis was formulated to account for time-dependent vapor formation rates, so it follows that their analytical solutions are also applicable to constant rate systems. Their analysis also assumes constant condensation coefficients, which is not applicable to atmospheric nucleation, and does not account for cluster coagulation or other cluster removal processes.
Since about 1990, we and others have focused on developing new instruments for measuring clusters and particles in the 1 nm range together with their gas phase precursors (especially sulfuric acid vapor). These instruments have been used for atmospheric observations and laboratory experiments. This work has shown that acid-base nucleation is one process that contributes to nucleation in the atmosphere. Also, in some studies (e.g., CLOUD) experiments are done using constant volume reactors with sampled air continuously replenished with particle-free flow. This article incorporates these processes into the dimensionless framework from the 1980s and systematically compares the effects of each process on the dynamic behavior of the nucleated aerosols. This work complements the detailed understanding of cluster chemistry that has been the focus of much recent work, as it provides a means to confirm closure between predicted and observed number distributions of larger particles. It is those larger particles that determine the properties or effects of the nucleated aerosol.
Theory
We assume that a single condensing species is produced by gas phase chemical reactions at a constant rate R , and we consider two nucleation scenarios: (i) single component nucleation accounting for cluster evaporation using the classical liquid droplet model, and (ii) chemical nucleation by reactions of sulfuric acid vapor with a basic gas (e.g., ammonia or an amine). For both scenarios, species concentrations are assumed to be spatially uniform, all possible coagulation interactions are included, particles are assumed spherical, and time-dependent (rather than steady-state) solutions are obtained. We begin with the dimensional equations for both nucleation scenarios followed by their dimensionless analogs. The reader is referred to the nomenclature for definitions and some important relationships.
(i) | Classical condensation-evaporation nucleation: the dimensional equations |
Monomer ():
[1]
Clusters ():
[2]
In Equation (Equation2[2] ), k corresponds to the number of vapor molecules (monomer) in a cluster, and is used both as a subscript and a variable (third term on r.h.s.). This formulation implicitly assumes two populations of particles: those formed by nucleation, which are included in the coagulation terms, and those present initially (AFuchs). The coagulation terms in these equations are discussed in textbooks (Friedlander Citation2000; Seinfeld and Pandis Citation2016). The evaporation rate constants, Ej, are derived using assumptions of the classical liquid droplet model, and are expressed in a discretized form that accounts for the evaporation of one molecule from a size j cluster to form a size j-1 cluster as well as the relative motion of the monomer and evaporating clusters (see nomenclature). In the limit of large j,
and the cluster size dependence in our expression for Ej (see nomenclature) collapses to the usual Kelvin equation expression. The Kelvin equation is derived assuming that the Gibbs free energy change for droplet formation is a continuous function of droplet radius, an approximation that becomes increasingly inaccurate as j decreases below 10. Our discrete formulation does nothing to ensure the validity of the underlying physical assumptions that lead to the liquid droplet model and has only a small effect on calculated results. The wall loss term is of the form used by Kürten et al. (Citation2014). The relationship between this and earlier notation is shown in the footnote to . The empirical constant, Cw, is independent of particle size, but depends on the reactor dimensions and operating conditions (i.e., convection, temperature, etc.). The dilution term accounts for the entrainment of particle-free air, and assumes that mixing is instantaneous so that concentrations remain spatially uniform. The terms that describe loss to pre-existing particles account for transport to particles in the transition regime; the Fuchs surface area concentration, AFuchs, is assumed constant and is based on the approximate expression given by Fuchs and Sutugin (Citation1971) and discussed by Davis et al. (Citation1981). The relationship between AFuchs and the condensation sink (CS) used by (Kerminen and Kulmala Citation2002) to describe cluster scavenging by pre-existing particles is (McMurry et al. Citation2005; Kuang et al. Citation2010):
[3]
Table 1. Dimensionless parametersFootnote‡.
so these approaches are equivalent. This approach for scavenging by preexisting particles was first discussed by McMurry and Friedlander (Citation1979); McMurry (Citation1983) obtained accurate numerical solutions to quantify its effects. Rao and McMurry (Citation1989) studied evaporation and wall losses using the mathematical framework discussed above.
(ii) | Acid-base nucleation: the dimensional equations |
The analysis uses the acid-base nucleation model proposed by Jen et al. (Citation2014) (scheme 2 with E3 = 0). This model involves simplifying assumptions about the multitude of acid-base reactions that contribute to cluster formation, as exemplified by the ACDC model proposed by McGrath et al. (Citation2012), and is consistent with laboratory measurements reported by Jen and coworkers. The reader is referred to Jen's paper for details. The model for acid-base reactions does not explicitly account for temperature or for the role of water. Instead, the model assumes that dependencies on those variables would be reflected primarily in the monomer (N1 = AB) evaporation rate constant (EAB). Furthermore, this analysis assumes that the base concentration, [B], remains constant during nucleation. With these assumptions, Jen's scheme 2 leads to the following conservation equations for free acid, monomer, and clusters:Free Acid (A)[4] Monomer (N1 = AB)
[5]
Clusters (Nk, k ≥ 2)[6]
For a constant rate system (R = constant), the above conservation equations for both nucleation scenarios collapse to a dimensionless form that is independent of R and of the condensing species' properties (molecular volume, surface tension, mass, etc.) provided that the collision frequency function is a homogeneous function of those properties. Particles formed in chambers can easily grow into the transition regime, and the transition regime collision frequency function is not a homogeneous function of condensing species properties. However, the free molecular collision frequency function is homogeneous. Therefore, prior to nondimensionalizing the equations, is replaced with
, thereby ensuring that solutions to the dimensionless equations are independent of species properties. One might guess that this would significantly limit the applicability of our results. However, as is shown in , simulations performed using free molecular and transition regime collision frequency functions are nearly indistinguishable at t = 0.5 h and have only a minor difference at t = 2 h. Two approaches were used for calculating transition-regime coagulation rates: the widely-used expression developed by Sutugin and Fuchs and described by Fuchs (Citation1964), and the approach developed by Gopalakrishnan and Hogan (Citation2011). Results obtained using the free molecular and transition regime collision frequency functions probably agree so well because most coagulation involves the smallest particles, which are present in much higher concentrations than particles in the transition regime. The results in were obtained assuming a monomer formation rate
and a monomer volume of
. This reaction rate is high enough to ensure that aerosol grows into the transition regime during the course of an hour or two; the monomer volume corresponds to a monomer containing one dimethylamine and one sulfuric acid molecule with an assumed density of
We conclude therefore, that for the chemical nucleation problems addressed in this article, sufficiently accurate results are obtained with the free molecular expression.
Figure 1. Transition regime vs. free molecular regime solutions to the dimensional equations for collision-controlled nucleation ( all set equal to zero) with the initial condition
for all j. Note that results obtained using the free molecular and transition regime collision frequency functions are nearly indistinguishable at 0.5 h and differ by only a small degree at 2 h.
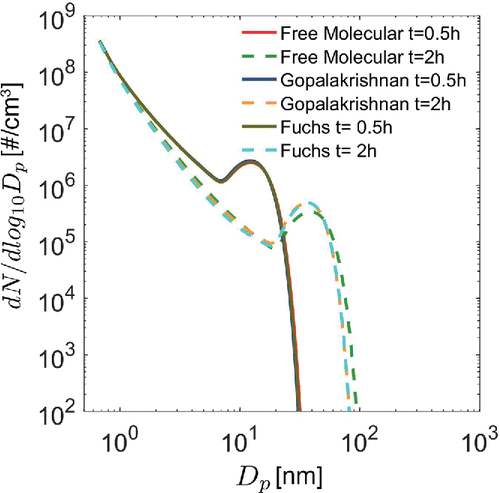
The following expressions transform species concentrations, time, and collision frequency functions, to their dimensionless analogs:[7]
Furthermore, for free molecular transport, species diffusivities have the following dependence on mass:[8]
With these transformations the above population balance equations assume the dimensionless forms given below. Values of the dimensionless parameters and
or
enable one to easily determine whether or not a given process (scavenging by preexisting particles, wall deposition, dilution, cluster evaporation) plays a significant role. (See for the mathematical definitions of these parameters.) Transforming the dimensionless solutions back to dimensional form is done simply using Equations (Equation7
[7] ), which requires knowing the applicable value of R together with thermodynamic or condensing species material properties needed to calculate
.
(iii) | Classical condensation-evaporation nucleation: the dimensionless equations |
Monomer (Ñ1)[9]
Clusters ()
[10]
(iv) | Acid-base Nucleation: the dimensionless equations |
Free acid (Ã)[11]
Monomer ()
[12]
Clusters ()
[13]
Note that for both classical and acid-base nucleation, the dimensionless parameters determine the importance of processes that compete with coagulation. They can be interpreted as dimensionless first-order rate constants. In addition, classical nucleation depends on the dimensionless surface energy
. As is shown in , this term is mathematically equal to the “free energy of a monomer surface” divided by the mean translational energy (
). It is more physically valid to interpret
as the proportionality constant for the change in the normalized surface energy upon the evaporation of one molecule from a cluster. The dimensionless quasi-first-order rate constant for the A+B reaction,
, incorporates the constant concentration of the basic gas and is equal to
. Because
, it follows that
. In the following discussion
will be used in place of
for simplicity. The dimensionless rate constants are all ratios of the corresponding dimensional rate constant to
, which also has dimensions of inverse time. The significance of
can be understood by considering the equation for monomer formation and monomer-monomer collisions alone:
[14] which leads to:
[15]
For a characteristic time equal to ,
. Thus,
is a measure of the rate at which monomer would reach its steady state concentration if monomer concentrations were affected only by chemical formation and monomer-monomer collisions. A given process (scavenging by preexisting particles, wall deposition, dilution, or evaporation) will be significant when its effect on monomer concentrations significantly exceeds that due to monomer collisions alone. The numerical solutions discussed below confirm this expectation. As
approach unity, the corresponding process becomes significant. For the nucleation processes, monomer concentrations are affected by two parameters (
or
), both of which influence whether or not evaporation has a significant effect. Significantly, all of the dimensionless rate constants vary in proportion to
. Thus, at a sufficiently high reaction rate, all of these processes become insignificant.
For classical nucleation, the solution to Equations (Equation9[9] ) and (Equation10
[10] ) depends on values of
and
, with larger values of
leading to a higher surface energy barrier for nucleation. Assuming the weight fraction of sulfuric acid in an aqueous droplet is the same as that in equilibrated aqueous solutions at the RH and temperature in Clark's chamber experiment, the surface tension of sulfuric acid aqueous droplets is estimated to be 0.075 J m−2, corresponding to an Ω value of 18.35. As an approximation, we choose to use Ω = 16 for consistency with results discussed by Rao and McMurry (Citation1989). Similarly, for acid-base nucleation the solutions to Equations Equation(11)–(13) depend on both
and
.
Discussion of numerical solutions
The dimensionless population balance equations were solved numerically to compare the effects of the different cluster sink processes. Calculations were done by varying a single dimensionless parameter, assuming that other processes can be neglected. Thus, to study the impact of wall deposition, the dimensionless parameter, W, was varied from zero to a value much larger than unity, while the other dimensionless rate constants (), were set equal to zero. In an actual system, of course, more than one process could play a significant role, and the equations would need to be solved to account for their combined effects. The discrete-sectional algorithm applied in this study is similar to that described by Rao and McMurry (Citation1989), though finer sections are utilized to improve simulation accuracy. In total, 100 discrete sizes and 250 sections are used, with a geometric volume amplification factor of 1.0718 for neighboring sections.
Effect of different sink processes on distribution
First we examine the influence of each of the processes on particle number distribution, using the collision-controlled limit as a baseline. displays the sensitivity of dimensionless number distributions to ,
,
,
and
at
. To allow concentrations of discrete sizes and distribution functions to be shown on the same plot, discrete concentrations were converted to distribution functions using the following transformation:
[16]
Figure 2. Effects of ,
,
,
and
on dimensionless number distributions at
. Note that results approach the collision-controlled limit for the smallest values of
,
,
,
and
except when
= 0.1.
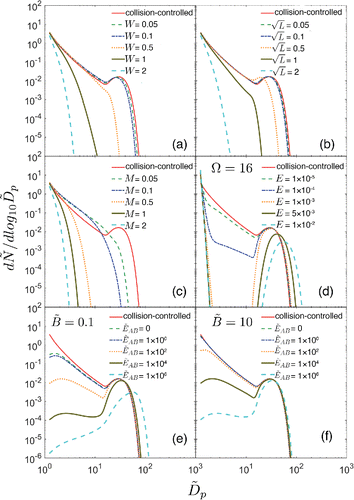
The minimum size plotted () corresponds to the monomer. Wall deposition (
, scavenging by pre-existing particles (
) and dilution (
) () have qualitatively similar effects on the distribution: as the rate constants increase, particle concentrations decrease for all particle sizes with the second peak in the distribution eliminated. However, for the same value of a rate constant (i.e., dimensionless parameter), dilution has a bigger effect than scavenging by preexisting particles or wall deposition since the impacts of the latter processes decrease with increasing particle size, while dilution affects all sizes equally. The influence of evaporation () is different in that total particle volume concentrations are redistributed rather than lost. For both nucleation scenarios, concentrations of
particles decrease sharply as the evaporation rate constants increase.
Solutions for two base concentrations ( are shown in and .
corresponds, for example, to
molecules cm−3 s−1 and a base concentration of 0.05 pptv. This value of
would occur if 1 ppb SO2 were oxidized at a rate of 1 % hr−1, a characteristic midday value for the continental atmosphere. While there remains uncertainty about concentrations of basic gases such as amines, mixing ratios would likely exceed 0.05 pptv in the terrestrial boundary layer but might be below this in other regions of the atmosphere. shows that for
the distribution does not converge to the collision-controlled limit even if the A + B reaction is collision-controlled (i.e,
). This is because the amount of available base is insufficient to react with all of the chemically-produced acid to form monomers (AB). For
, however, the base is high enough to ensure that nucleation reaches the collision-controlled limit when
. Acid-base nucleation leads to less pronounced valleys than those presented in since only monomers evaporate in this model while all particles undergo evaporation in the classical nucleation model.
Figure 3. (a) Effects of on time-dependent total concentrations
for k = 10 and 3000, and (b)
vs.
for several values of k. Results for
are qualitatively similar to those for
and are therefore not included.
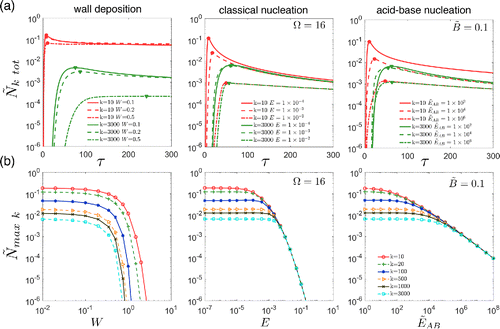
also illustrates how measurements of sub 10 nm number distributions (corresponds to a dimensionless diameter of about 18 when ) could provide insights into processes that occur during nucleation. For both classical and acid-base nucleation, the number distributions of the smallest particles are significantly suppressed relative to distributions that would occur if clusters do not evaporate. Information on sub 10 nm number distributions would also allow one to distinguish between these nucleation processes. While significant progress on such measurements has been made over the past decade, measurement uncertainties need to be reduced to allow such measurements to reach their full potential.
Particles larger than cut-off sizes
Aerosol instruments such as CPCs often have cut-off sizes below which detection efficiencies drop sharply. Therefore, it is sometimes useful to compare the predicted and observed number concentrations of particles greater than a specified size, k. shows the impact of on the time-dependent number concentration of particles larger than k = 10 and 3000. For a monomer volume of
, these correspond to diameters of 1.5 and 10 nm, respectively.
is defined by:
[17]
In each case, one rate constant () is varied to show its effect on
with the other rate constants all set to zero. The effects of
and
are not shown here as they are qualitatively similar to that of
. In each case,
increases from zero to a maximum value,
, before decreasing due to coagulation and other removal processes. The maxima are indicated by circles (k = 10) and triangles (k = 3000). Note that wall deposition leads to
, while significantly smaller decreases in
are observed for both nucleation scenarios. This is because wall deposition causes a significant fraction of nucleated particles to be lost before they reach size 3000, while in the absence of wall deposition particles tend to survive once they pass size 10 for both nucleation scenarios.
displays as a function of the rate constants (
,
, and
) for six values of
ranging from 10 to 3000. Again, there's an obvious distinction between wall deposition and both nucleation scenarios. For wall deposition, lines for different
values do not converge as W increases, while values converge towards a single asymptotic limit as E (classical) and
(acid-base) increase. These asymptotic limits occur because (i) fewer particles are formed as the evaporation parameters increase, decreasing the effects of coagulation, and (ii) particles that do form tend to survive.
drops rapidly with W and E, but less rapidly with
. The more gradual decrease with
is due, in part, to the constant base concentration assumed in the model and the stability of clusters larger than monomer. The model implies that classical nucleation tends to produce particles in a single burst, while nucleation resulting from chemical processes (such as acid-base reactions) tend to be more sustained.
Evolution of particle size distribution
Contour plots can be utilized to conveniently visualize the development of particle distributions for the entire time span of simulation. displays contour plots of time-dependent number distributions for the collision-controlled limit and for selected values of . The particle number distributions are represented by a logarithmic color scale with red representing the highest concentration and blue the lowest. Due to the wide range of particle concentration, dimensionless concentrations lower than
are artificially set to
. Values of the rate constants are chosen such that
would be half of its value reached in the collision-controlled limit. The horizontal line at
corresponding to k = 100 is shown for reference.
Figure 4. Contour plots showing the effects of on time-dependent size distributions. Values of the rate constants are chosen so that
is half of its value in the collision-controlled limit. The dimensionless particle concentration is indicated by a logarithmic color scale.
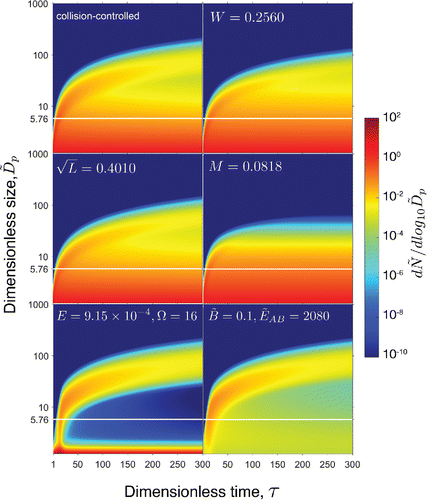
The contour plots for and
are similar to that of the collision-controlled limit although particle concentrations are somewhat lower for all sizes and the average particle size is somewhat smaller. In contrast, dilution (
) leads to distributions that reach a steady state at approximately
.
,
and
all lead to permanent removal of particulate mass from the nucleated aerosol, but with different size dependencies. Losses by wall deposition and scavenging by preexisting particles decrease with increasing size
and
, while
affects all particle sizes equally and at steady state newly generated particle volume compensates for sampling losses.
For both nucleation scenarios, new particles are formed in the early stage of the simulation. These particles serve as condensation sinks and continue to grow and become more polydisperse as time progresses. Such widening of the distribution for these particles is due partly to coagulation and partly to numerical diffusion, as discussed by Warren and Seinfeld (Citation1985).
Conclusions
We used a discrete-sectional model to simulate the dynamic behavior of aerosols in chemically reacting systems. The population balance equations were cast in a nondimensional form that reveals the dimensionless parameters that quantify the effects of various sink processes. Based on our simulations, we draw the following conclusions:
1. | The use of the free molecular collision frequency function, rather than the transition regime expression, is justified for simulations described in this article. This is because most coagulation occurs among the smallest particles. | ||||
2. | For every sink process except acid-base nucleation, the collision-controlled limit is reached provided the corresponding dimensionless rate constant approaches zero. For acid-base nucleation, the collision-controlled limit is reached only when the base concentration is sufficiently high. | ||||
3. | Different sink processes target particles of different sizes. Specifically, dilution has the same effect on all particle sizes. Evaporation, either in the classical nucleation model or acid-base nucleation model, preferentially affects the smallest sizes. Wall deposition and loss to preexisting particles affect particles of all sizes, although at rates that decrease with increasing size. These size-dependencies impact the time dependent size distributions that would be observed in a chamber experiment or the atmosphere. | ||||
4. | Dimensionless rate constants embody the dependence of sink processes on material properties and experimental conditions, and are straightforward to calculate. Care must be taken to avoid errors due to dilution or wall deposition when calculating monomer formation rates, R. |
Our analysis leads to dimensionless parameters () that determine whether or not a given process significantly affects size distributions of nucleated particles. When the parameters are sufficiently large, effects become significant. We have formulated the theory to include all of the processes that were considered, but for simplicity we have shown numerical results that consider the effects of only an individual process. In practice, multiple processes are likely to occur simultaneously, and in that case the equations must be solved to account for their simultaneous effects. For example, wall deposition and dilution always occur in chamber experiments reported by CLOUD, and cluster evaporation occurs for some chemical systems. Collectively, these processes will have a greater effect than any individual process, so deviations from the collision-controlled limit will occur at smaller values of the dimensionless parameters than are reported here for individual processes.
Nomenclature
AFuchs | = | aerosol surface area concentration, corrected to account for transition regime condensation |
[A] | = | concentration of free acid |
= | dimensionless concentration of free acid | |
[B] | = | concentration of free base |
[B˜] | = | dimensionless concentration of free base |
βij | = | collision frequency function for clusters that contain i and j monomer |
βij fm | = | free molecular expression for i-j colisions |
cij | = | dimensionless free molecular collision function for i-j clusters |
ciA | = | |
Cw | = | Chamber wall loss constant as defined by Kürten et al. (Citation2014) |
dp | = | particle diameter |
= | dimensionless particle diameter | |
Ds | = | diffusion coefficient for species s |
Ej | = | Monomer evaporation rate constant from size j clusters |
kA+B | = | forward rate constant for the reaction A+B→AB, assumed equal to the A + B collision rate |
EAB | = | evaporation rate constant for the reaction AB→A+B |
KAB | = | equilibrium constant for A+B⇔AB |
kb | = | Boltzmann's constant |
Kn | = | Knudsen Number = |
ms | = | mass of species s |
n(dp) | = | number distribution function of preexisting aerosol |
Nj | = | concentration of clusters that contain j monomer |
= | dimensionless concentration of clusters that contain j monomer | |
Nsat | = | saturation vapor concentration of condensing monomer |
R | = | rate of monomer formation by gas phase reaction |
Qdil | = | volumetric flow rate of particle free air entering a rigid reactor |
t | = | time |
T | = | absolute temperature |
v1 | = | monomer volume = m1/ρ |
Vchamber | = | volume of rigid chamber |
Greek
δ2j | = | Kronecker delta function ( = 1 for j = 2, and = 0 for j≠2) |
λ | = | mean free path |
ρ | = | particle density |
σ | = | surface tension |
τ | = | dimensionless time |
Funding
This research was supported by the US Department of Energy's Atmospheric System Research, an Office of Science, Office of Biological and Environmental Research program, under grant number DE-SC0011780.
References
- Clark, W. E. (1972). Measurements of Aerosols Produced by the Photochemical Oxidation of SO2 in Air. Mechanical Engineering. University of Minnesota, Minneapolis, MN.
- Clark, W. E., and Whitby, K. T. (1975). Measurements of Aerosols Produced by the Photochemical Oxidation of SO2 in Air. J. Colloid Interface Sci., 51:477–490.
- Crump, J. G., and Seinfeld, J. H. (1981). Turbulent Deposition and Gravitational Sedimentation of an Aerosol in a Vessel of Abritrary Shape. J. Aerosol Sci., 12(5):405–415.
- Davis, J., Ravindran, P., and Ray, A. K. (1981). Single Aerosol Particle Studies. Adv. Colloid Interface Sci., 15:1–24. doi: 10.1016/0001-8686(81)80001-2.
- Friedlander, S. K. (2000). Smoke, Dust, and Haze: Fundamentals of Aerosol Dynamics. Oxford University Press, New York.
- Fuchs, N. A. (1964). The Mechanics of Aerosols. Pergamon Press, Oxford.
- Fuchs, N. A., and Sutugin, A. (1971). High Dispersed Aerosols. in Topics in Current Aerosol Research (Part 2), G. M. Hidy, and J. R. Brock, eds., Pergamon, New York, pp. 1–60.
- Gopalakrishnan, R., and Hogan Jr, C. J. (2011). Determination of the Transition Regime Collision Kernel from Mean First Passage Times. Aerosol Sci. Technol., 45(12):1499–1509. doi: 10.1080/02786826.2011.601775.
- Jen, C., McMurry, P. H., and Hanson, D. R. (2014). Stabilization of Sulfuric Acid Dimers by Ammonia, Methylamine, Dimethylamine, and Trimethylamine. J. Geophys. Res., 119:7502–7514. doi: 10.1002/2014JD021592.
- Kerminen, V. M. and Kulmala, M. (2002). “Analytical formulae connecting the ”real” and the “apparent” nucleation rate and the nuclei number concentration for atmospheric nucleation events. J. Aerosol Sci., 33(4):609–622.
- Kirkby, J., Duplissy, J., Sengupta, K., Frege, C., Gordon, H., Williamson, C., Heinritzi, M., Simon, M., Yan, C., Almeida, J., Tröstl, J., Nieminen, T., Ortega, I. K., Wagner, R., Adamov, A., Amorim, A., Bernhammer, A.-K., Bianchi, F., Breitenlechner, M., Brilke, S., Chen, X., Craven, J., Dias, A., Ehrhart, S., Flagan, R. C., Franchin, A., Fuchs, C., Guida, R., Hakala, J., Hoyle, C. R., Jokinen, T., Junninen, H., Kangasluoma, J., Kim, J., Krapf, M., Kürten, A., Laaksonen, A., Lehtipalo, K., Makhmutov, V., Mathot, S., Molteni, U., Onnela, A., Peräkylä, O., Piel, F., Petäjä, T., Praplan, A. P., Pringle, K., Rap, A., Richards, N. A. D., Riipinen, I., Rissanen, M. P., Rondo, L., Sarnela, N., Schobesberger, S., Scott, C. E., Seinfeld, J. H., Sipilä, M., Steiner, G., Stozhkov, Y., , S. F., Tomé, A., Virtanen, A., Vogel, A. L., Wagner, A. C., Wagner, P. E., Weingartner, E., Wimmer, D., Winkler, P. M., Ye, P., Zhang, X., Hansel, A., Dommen, J., Donahue, N. M., Worsnop, D. R., Baltensperger, U., Kulmala, M., Carslaw, K. S., and Curtius, J. (2016). Ion-induced nucleation of pure biogenic particles. Nature, 533(7604):521–526.
- Koirala, R., Pratsinis, S. E., and Baiker, A. (2016). Synthesis of Catalytic Materials in Flames: Opportunities and Challenges. Chem. Soc. Rev., 45:3053–3068. doi: 10.1039/c5cs00011d.
- Kuang, C., McMurry, P. H., McCormick, A. V., and Eisele, F. L. (2008). Dependence of Nucleation Rates on Sulfuric Acid Vapor Concentration in Diverse Atmospheric Locations. J. Geophys. Res.-Atmospheres, 113(D10):D10209. doi: 10.1029/2007JD009253.
- Kuang, C., Riipinen, I., Sihto, S.-L., Kulmala, M., McCormick, A. V., and McMurry, P. H. (2010). An Improved Criterion for New Particle Formation in Diverse Atmospheric Environments. Atmos. Chem. Phys., 10(17):8469–8480. doi: 10.5194/acp-10-8469-2010.
- Kürten, A., Jokinen, T., Simon, M., Sipilä, M., Sarnela, N., Junninen, H., Adamov, A., Almeida, J., Amorim, A., Bianchi, F., Breitenlechnerg, M., Dommen, J., Donahue, N. M., Duplissy, J., Ehrhart, S., Flagan, R. C., Franchin, A., Hakala, J., Hanselg, A., Heinritzi, M., Hutterli, M., Kangasluom, J., Kirkby, J., Laaksonen, A., Lehtipalo, K., Leiminger, M., Makhmutov, V., Mathot, S., Onnela, A., Petäjä, T., Praplan, A. P., Riccobono, F., Rissanen, M. P., Rondo, L., Schobesberger, S., Seinfeld, J. H., Steiner, G., Tomé, A., Tröstl, J., Winkler, P. M., Williamson, D., Wimmer, D., Ye, P., Baltensperger, U., Carslaw, K. S., Kulmala, M., Worsnop, D. R., and Curtius, J. (2014). Neutral Molecular Cluster Formation of Sulfuric Acid–Dimethylamine Observed in Real Time Under Atmospheric Conditions. Proc. Nat. Acad. Sci., 111(42):15019–15024. doi: doi/10.1073/pnas.1404853111.
- Lushnikov, A. A., and Kulmala, M. (1995). Source-Enhanced Condensation in Monocomponent Disperse Systems. Phys. Rev. E, 52(2):1658–1668. doi: 10.1103/PhysRevE.52.1658.
- McGrath, M. J., Olenius, T., Ortega, I. K., Loukonen, V., Paasonen, P., Kurtén, T., Kulmala, M., and Vehkamäki, H. (2012). Atmospheric Cluster Dynamics Code: A Flexible Method for Solution of the Birth-Death Equations. Atmos. Chem. Phys., 12:2345–2355. doi: 10.5194/acp-12-2345-2012.
- McMurry, P. H. (1980). Photochemical Aerosol Formation from SO2: A Theoretical Analysis of Smog Chamber Data. J. Colloid Interface Sci., 78:513–527.
- McMurry, P. H. (1983). New Particle Formation in the Presence of an Aerosol: Rates, Time Scales and sub-0.01 µm Size Distributions. J. Colloid Interface Sci., 95(1):72–80.
- McMurry, P. H., Fink, M. A., Sakurai, H., Stolzenburg, M. R., Mauldin, L., Moore, K., Smith, J. N., Eisele, F. L., Sjostedt, S., Tanner, D., Huey, L. G., Nowak, J. B., Edgerton, E., and Voisin, D. (2005). A Criterion for New Particle Formation in the Sulfur-Rich Atlanta Atmosphere. J. Geophys. Res. - Atmospheres, 110:D22S02. doi: 10.1029/2005JD005901.
- McMurry, P. H., and Friedlander, S. K. (1979). New Particle Formation in the Presence of An Aerosol. Atmos. Environ., 13:1635–1651.
- McMurry, P. H., and Rader, D. J. (1985). Aerosol Wall Losses in Electrically Charged Chambers. Aerosol Sci. and Technol., 4:249–268.
- Rao, N. P., and McMurry, P. H. (1989). Nucleation and Growth of Aerosol in Chemically Reacting Systems: A Theoretical Study of the Near- Collision-Controlled Regime. Aerosol Sci. Technol., 11:120–132.
- Riipinen, I., Sihto, S.-L., Kulmala, M., Arnold, F., Dal Maso, M., Birmili, W., Saarnio, K., Teinila, K., Kerminen, V.-M., Laaksonen, A., and Lehtinen, K. E. J. (2007). Connections Between Atmospheric Sulphuric Acid and New Particle Formation During QUEST III - IV Campaigns in Hyytiala and Heidelberg.” Atmos. Phys. Chem., 7:1899–1914.
- Seinfeld, J. H., and Pandis, S. N. (2016). Atmospheric Chemistry and Physics: From Air Pollution to Climate Change, 3rd Edition. Wiley Interscience, New York.
- Sihto, S. L., Kulmala, M., Kerminen, V. M., Dal Maso, M., Petaja, T., Riipinen, I., Korhonen, H., Arnold, F., Janson, R., Boy, M., Laaksonen, A., and Lehtinen, K. E. J. (2006). Atmospheric Sulphuric Acid and Aerosol Formation: Implications from Atmospheric Measurements for Nucleation and Early Growth Mechanisms. Atmos. Chem. Phys., 6:4079–4091.
- Swihart, M. T., and Girshick, S. L. (1999). Thermochemistry and Kinetics of Silicon Hydride Cluster Formation During Thermal Decomposition of Silane. J. Phys. Chem., 103B:64–73.
- Tröstl, J., Chuang, W. K., Gordon, H., Heinritzi, M., Yan, C., Molteni, U., Ahlm, L., Frege, C., Bianchi, F., Wagner, R., Simon, M., Lehtipalo, K., Williamson, C., Craven, J. S., Duplissy, J., Adamov, A., Almeida, J., Bernhammer, A.-K., Breitenlechner, M., Brilke, S., Dias, A., Ehrhart, S., Flagan, R. C., Franchin, A., Fuchs, C., Guida, R., Gysel, M., Hansel, A., Hoyle, C. R., Jokinen, T., Junninen, H., Kangasluoma, J., Keskinen, H., Kim, J., Krapf, M., Kürten, A., Laaksonen, A., Lawler, M., Leiminger, M., Mathot, S., Möhler, O., Nieminen, T., Onnela, A., Piel, F. M., Miettinen, P., Rissanen, M. P., Rondo, L., Sarnela, N., Schobesberger, S., Sengupta, K., Sipilä, M., Smith, J. N., Steiner, G., Tomè, A., Virtanen, A., Wagner, A. C., Weingartner, E., Wimmer, D., Winkler, P. M., Ye, P., Carslaw, K. S., Curtius, J., Dommen, J., Kirkby, J., Kulmala, M., Riipinen, I., Worsnop, D. R., Donahue, N. M., and Baltensperger, U. (2016). The Role of Low-Volatility Organic Compounds in Initial Particle Growth in the Atmosphere. Nature, 533(7604):527–531.
- Warren, D. R., and Seinfeld, J. H. (1985). Simulation of Aerosol Size Distribution Evolution in Systems with Simultaneous Nucleation, Condensation, and Coagulation. Aerosol Sci. Technol., 4:31–43.
- Weber, R. J., Marti, J., McMurry, P. H., Eisele, F. L., Tanner, D. J., and Jefferson, A. (1996). Measured Atmospheric New Particle Formation Rates: Implications for Nucleation Mechanisms. Chem. Eng. Comm., 151:53–64.
- Whitby, K. T., and Clark, W. E. (1966). Electrical Aerosol Particle Counting and Size Distribution Measuring System for the 0.015 to 1.0 µm Size Range. Tellus, 13:573–586.