ABSTRACT
Fibrous filters are commonly used for aerosol purification and sampling. The filtration efficiency has been extensively studied using standard aerosol generators, yet the literature on experimental data and theoretical study concerning the filtration of agglomerates from real engines remains scarce. A filtration efficiency test system was developed to determine the filtration efficiency of two types of filters (uncoated and fluorocarbon coated) loaded by particulate matter (PM) emissions from a gasoline direct injection (GDI) engine. The experimental results showed that the filtration efficiency in terms of PM mass and number increased over time for both types of filters. The fractional efficiency (penetration efficiency) curves for the test fibrous filters rendered a U-shaped curve for particle sizes from 70 to 500 nm, and the most penetrating particulate size (MPPS) decreased over time. A small fraction of accumulation mode particles with the size between 70 nm to 500 nm penetrated the filters while almost all nucleation mode particles with the size below 50 nm were captured by the filters. The filtration efficiency derived from an empirical model based on classical single-fiber theory for laden filters generally agreed with the experimental data for the first 500 s, but suffered a significant deviation by approximately one order of magnitude at 948 s. A better estimate of the filtration efficiency trend with the maximum deviation of about 20% (except for large particles at the high end of the measurement spectra) was obtained by using a revised model which incorporates the effects of the increase in filter solidity, local velocity, dynamic shape factor and effective total length of fibers during the filtration process.
© 2017 American Association for Aerosol Research
EDITOR:
1. Introduction
Vehicular exhaust has been considered a significant source of ambient PM emissions (EPA Citation2009), and a number of emission regulations, particularly more stringent benchmarks for particulate matter (PM) mass and number concentrations have been mandated worldwide. With the introduction of clean engine design and diesel particulate filters (DPFs), the limit of detection of the gravimetric method is no longer sufficiently sensitive to record PM mass concentrations under existing regulations (Giechaskiel et al. Citation2014; Chen, et al. Citation2017). A detailed analysis of PM filtration process and filtration efficiency is needed to improve the accuracy of PM mass and number concentrations.
Particle counting methods (PCM) are commonly used to evaluate filter filtration efficiency in which the pre- and post-filter PM number concentrations are measured and compared. Glass fiber uncoated filters are less affected by moisture and age than cellulose fiber filters. Liu and Lee (Citation2002) introduced an improved filter test method based on the PCM method, and various types of filters were evaluated by mono-dispersed standard particles with a mobility diameter of 0.03–1.3 μm. Previous studies (Liu and Lee Citation2002; Kasper et al. Citation2009; Viswanathan et al. Citation2015) mainly utilized mono-dispersed and spherical particles to evaluate the filtration efficiency, while the filtration of aggregates from real engines (poly-dispersed and non-spherical) was rarely investigated. Therefore, it is of interest to quantify the filtration efficiencies of the filter (uncoated and fluorocarbon coated) for poly-disperse and non-spherical agglomerates from real engine-out emissions.
A classical single fiber (SF) filtration theory has been widely used for a variety of scenarios (Hinds Citation1999; Kulkarni et al. Citation2011). The shape of aerosol particles, filtration condition and filter parameters appeared to have a significant influence on the filter performance (Yang et al. Citation2009). The aforementioned filtration models were normally verified by experiments using spherical particles or metal agglomerates with uniform particle size (Kasper et al. Citation2009; Kim et al. Citation2009; Viswanathan et al. Citation2015). It would be desirable to assess the applicability of the filtration models for non-spherical agglomerates from a real engine with a wide range of particle size.
Another main difficulty is how to relate the change of particle deposition on filters to a change in filtration efficiency for particle-laden filters (Kasper et al. Citation2009; Liu et al. Citation2011; Ardkapan et al. Citation2014; Viswanathan et al. Citation2015). Kasper et al. (Citation2009) reviewed the models for dust loaded filters, and reported that researchers tried a number of empirical approaches to improve the SF model (Lajos Citation1986; Kanaoka Citation1989; Lehmann Citation2005; Kasper et al. Citation2009) considering the computational challenges of filtration kinetics pursued by Payatakes and Chi (Citation1976) and Payatakes and Gradoń (Citation1980). One practical difficulty is how to relate the changes in the shape of agglomerate particles to a change in single fiber drag coefficient and filtration efficiency. Another difficulty is how to “distribute” the accumulated dust mass on the filter, and how to determine the effects of the loaded particles on the parameters of filters. Thus, there remains a need for checking how well the established empirical model predicts the filtration efficiency trends for agglomerates from engines, and it might be necessary to revise the classical model for predicting agglomerates loaded filters.
The objective of the study was to evaluate the filtration performance of two filter types (uncoated and fluorocarbon coated) by using non-spherical and poly-disperse agglomerates (5–1000 nm) emitted from a GDI engine. The particle mass and number penetration efficiency, the MPPS and different deposition characteristics for nucleation mode (<50 nm) and accumulation mode (>50 nm) were determined by measuring the particle size distributions at pre- and post-filter locations. A comprehensive filtration model has been developed to predict the filtration efficiency for non-spherical particles from a GDI engine during the filtration process by incorporating a number of effects largely ignored in the classical single fiber model.
2. Experimental apparatus and methodology
2.1. Experimental set-up
The particle size distributions were generated from a GDI engine under a specific steady-state condition. An in-house filtration efficiency testing system was used to perform filtration experiments on two types of filters (uncoated and fluorocarbon coated). A Cambustion DMS500 fast particle spectrometer was used to measure the particle size distributions upstream and downstream of test filters.
2.1.1. Test engine and filtration efficiency testing system
The test engine was operated with stratified injection, so that a large portion of accumulation mode particles was generated. This operation point was an adaptation of a cold start operation point that is known to generate high levels of particle matter (PM) emissions. The test engine was equipped with a Three Way Catalyst (TWC), but only pre-catalyst samples were measured in this study.
A partial-flow dilution system was developed to provide a more realistic representation of actual PM emissions by simulating the process of the plume discharge to the atmosphere. The filtration efficiency testing system is shown in . The pure air, after heating and filtration, mixed completely with exhaust gas in a mixing tank. The pre- and post-filter particle size-resolved number concentrations were measured by a Cambustion DMS500 fast particle spectrometer. The DMS500 used in this study was specially calibrated for a GDI engine which takes into account the different particle charging characteristics between agglomerates and spheres and thus the uncertainties caused by multi-charging could be mitigated. The GDI-derived PM emissions are a mixture of soot and its coating of volatile organic compounds (VOCs), and the VOC mass percentage could be up to 90% of the overall PM emissions (Chen and Stone. 2011; Chen et al. Citation2012).
The upstream and downstream particles number concentrations were measured under the iso-kinetic sampling conditions, and the same dilution ratios were maintained throughout the experiments by adjusting the volume of hot dilution air. Mass Flow Rate 1 (MFM1) is the “dilution” air mass flow rate when the DMS500 took pre-filter PM sample. MFM*1 is the “dilution” air mass flow rate when the DMS500 took post-filter PM sample. The penetration efficiency (P) of particles through filters can be obtained using Equation (Equation1[1] ):
[1] where c(n)post-filter and c(n)pre-filter are the particle number concentrations at the downstream and upstream of the test filters, respectively.
Previous research suggested that the face velocity of the filters could significantly affect the filtration efficiency (Viswanathan et al. Citation2015). Thus, care was taken during the design of the system to achieve a constant face velocity of the test filters. A quantity of gas flow (corresponding to that taken by the DMS500) was bypassed the filter when sampling downstream of the filters, so that the same face velocity was achieved when sampling upstream of the filters. The bypass loop was closed when sampling upstream of the filter. The formulas used to calculate the mass flow rate of the filter are listed below (Equations (Equation2[2] ) and (Equation3
[3] )). A needle valve was then fitted in the bypass loop to set the bypass mass flow rate (
) equal to that of the mass flow rate of filter sampling system (MFRDMS500) to retain the face velocity of the filter constant (
). The face velocity of exhaust for the current experiment was maintained at approximately 5 cm/s.
When the DMS500 took pre-filter PM sample:[2]
When the DMS500 took post-filter PM sample:[3]
Particle losses and re-nucleation are highly dependent on the dilution parameters (Wei et al. Citation2001). Therefore, polished stainless steel pipe was used in the whole system to mitigate particle electrostatic loss. Raw samples from the exhaust stream passed through a heated pipe, and the sampling flow was heated to 200°C prior to mixing with low temperature air. The temperature of the heater was controlled to keep the filter temperature at 47°C. The formula used to calculate the dilution ratio (DR) is shown as Equations (Equation4[4] ) and (5). Because the bypass kept the total mass flow rate (or pressure) of diluted exhaust stable, the DR had little variation when changing the sample position from upstream to downstream of the test filter. The DR was kept constant by slightly adjusting the air flow rate. The DR of Whatman® GF/A filter was kept at 8.4 for both upstream and downstream sampling, and the DR of coated Pallflex® filter was also constant at 6.3. All the results of the PM emissions have been corrected by the dilution ratios.
[4]
[5]
2.1.2. Test filters
Fibrous filters consist of a mat of individual fibers. Generally, filter porosity is relatively high, ranging from about 0.6 to 0.999 (Kulkarni et al. Citation2011). Two types of filters (uncoated and fluorocarbon coated) were used in the experiments. The main parameters for the test filters are listed in .
Table 1. Specifications of the filters and main parameter for calculating the filtration efficiency.
The solidity is the volume ratio of total fibers to the filter, and it can be calculated by the weight and volume of filters (Thomas et al. Citation2001). The gas flowing through the glass fiber filter was kept constant at 47°C during the sampling process by using a dilution system with closed-loop control. The same filter holder was used for bracing both types of test filters. According to Davies' law (Citation1973), the effective fiber mean diameter df can be calculated using Equation (Equation6[6] ) with the pressure drop (△P) of a clean fibrous filter, the viscosity of the gas η and the face velocity of the filter U∞:
[6]
The pressure drop was monitored by measuring the pressures at pre- and post-filter locations. The mass of test filters was measured by an electronic balance (XPE26, METTLER-TOLEDO with a resolution of 1 μg) to calculate the solidity of the filter. A density bottle method was used to determine the fiber density with the resolution of 0.007 g/cm3 (Turnbull Citation2010; Xu Citation2000). For the density bottle method, the test fiber is put into a full bottle of water. By carefully determining the overflown water mass and the fiber mass, the fiber density can be calculated assuming that the volume of the overflown water is equal to the fiber volume.
2.2. Modeling of particle penetration through a filter
2.2.1. The filtration efficiency model for non-spherical particles
The classical filtration model for spherical particles through a fibrous filter was revised to incorporate the non-spherical effect of real agglomerates. The dynamic shape factor χ was adopted to correct the friction factor of the agglomerates in this work.
The deposition mechanisms for sub-micron ultrafine particles include diffusion (ED), interception (ER), impaction (EI), and enhanced interception for a diffusing particle (EDR). The expression for the filtration efficiency with the fiber diameter df, solidity of the filter α, filter thickness T, and total single fiber collection efficiency E1 was given by Brown (Citation1993):[7] where
[8]
The expressions of ED, ER, EI, and EDR are provided in the online supplementary information (SI). A modified model for non-spherical particles through a fibrous filter was given by Kim et al. (Citation2009) based on the classical single fiber model, and the results of the model generally agreed with the experimental results. Particles from engines are mostly agglomerates of many fine nanoparticles (primary particles) with a size between 5 and 50 nm (Friedlander et al. Citation2004), and the agglomerates are of about 100 nm to several micrometers with typical fractal dimensions Df = 2.1–2.7 (Maricq and Xu. 2004; Olfert et al. Citation2007; Friedlander et al. Citation2008). Therefore, the dynamic shape factor χ needs to be adopted to revise the drag force of mass spherical sphere of agglomerates, and it is defined as the ratio of the drag force of the agglomerate to the drag force of a mass equivalent sphere, as shown in Equations (Equation9[9] ) and (Equation10
[10] ) based on Stokes' law (Brockmann and Rader Citation1990; Kelly and McMurry Citation1992). If the dynamic shape factor and mass equivalent particle diameter (dm) are determined, the behavior of the agglomerates can be predicted as they are influenced by Brownian diffusion, interception and inertial impaction mechanisms (Kulkarni et al. Citation2011). The dm is defined as the diameter of a nonporous sphere composed of the bulk particle material that has the same mass as the particle.
[9]
[10]
F*d is the drag force on a non-spherical particle; Fd is the drag force on that particle's mass equivalent sphere; fd is defined as the friction factor of the mass equivalent sphere of the agglomerate with a unit kg/s, which reflects the drag force on a particle at the same speed; fd* is the friction factor of the agglomerate; Cc is the Cunningham slip correction factor; η is the viscosity of the gas; U∞ is face velocity of the air incident on the filter.
It has been shown by Kelly and McMurry (Citation1992) that χ can be calculated as function of the mobility diameter, dP, and the dm which can be obtained from the agglomerate material density and its mass:[11]
The corrected friction factor for agglomerates was given in Equation (Equation12[12] ) by combining Equations (Equation10
[10] ) and (Equation11
[11] ), and the mobility diameter is used in all filtration mechanisms instead of mass equivalent diameter of agglomerates to calculate the precise friction factor, which have also been determined by Brownian dynamics method.
[12] where the Cunningham slip correction factor Cc with dm is:
[13] where θ is the mean free path of the gas molecules. The particle mass (m) had been experimentally determined using a Couette CPMA (centrifugal particle mass analyser) on a GDI (gasoline direct injection) engine by previous researches (Chen et al. Citation2012), as shown in Equation (Equation14
[14] ). The agglomerate fractal dimension form the GDI engine is 2.65.
[14]
The effective density, ρe is defined here as the mass of a particle divided by the volume of a spherical particle having the same mobility diameter, and that witnessed a declining trend from about 1.15 g/cm3 at 20 nm to 0.29 g/cm3 at 1000 nm because smaller agglomerates (i.e., 20 nm) were more compact than large agglomerates. The similar trend had been found by Olfert et al. (Citation2007) and Maricq and Xu (Citation2004). The size of primary particles of agglomerates from engines is between 20 and 50 nm, so the material (mass) density of agglomerates adopted the value (ρp = 1.15 g/cm3) at dp = 20 nm. The dimensionless number Pe was revised using the modified friction factor, since Pe was the appropriate criterion for description of the Brownian diffusion deposition, and the dimensionless number Stk was used to describe the deposition efficiency of larger particles, which was revised using the modified friction factor as well. Therefore, the mobility diameter has been adopted in the diffusion, interception and impact mechanisms in this work. The expressions of the dimensionless numbers Pe and Stk are shown as follows:[15]
[16] where the relaxation time
is determined by the drag force and field force (e.g., gravitational force in gravity field); D is the revised diffusion coefficient of agglomerates; T is the absolute temperature.
2.2.2. The revised model for particle-laden filters
It should be mentioned that the filtration models adopted and proposed herein were all based on the filtration efficiency model for non-spherical agglomerate particles on clean filters (Equations Equation(7)–(16)), and may not applicable to heavily laden filters. In this study, a revised model for particle-laden filters was proposed to evaluate the increase of filtration efficiency incorporating the effects of the increase in filter solidity, local flow rate inside the filter and effective total length of fibers on filtration efficiency.
An empirical expression (Equation (Equation17[17] )) with a mass loading term was proposed as a revised version for particles-laden filters by Kanaoka (Citation1989), departing completely from any a-priori assumptions about deposit shape or growth patterns:
[17] where E1 is the total single-fiber filtration efficiency without laden particles; E1(m) is the total single-fiber filtration efficiency with soot loading; λ0 is an empirical ‘collection efficiency raising factor’, which was found to depend on E1 as well as Stk and the interception parameter R; cm is the accumulated mass per filter volume in kg/m3. If a linear regression λ0 = (E1)−a is performed the exponential index ‘a’ will be in the range of 1.1–1.3 (Kasper et al. Citation2009).
There is a challenge to determine the distribution of the accumulating dust mass in the filter, and the changes of the filter parameters due to particle load. In particular, the change in local velocity and effective filter solidity has a pronounced influence on the filtration efficiency as shown in simulation results (see the SI). Considering the aforementioned challenges which are largely neglected in the classical theory, a revised model is proposed to estimate the required change in local velocity and effective filter solidity given the fact that both parameters increase with the deposition of particles, assuming the dust mass was distributed uniformly inside the filters and the deposited particles-dendrites were non-permeable to newly arrived particles. Therefore, E1(m) can be calculated by adding Equations (Equation18[18] ) and (Equation19
[19] ) to the classical single-fiber filtration efficiency model for non-spherical agglomerate particles (Equations Equation(7)–(16)):
[18]
[19] where A is the cross-sectional area of the filter exposed to the entering air stream; t is the time; α0 is the solidity of the bare filter; α(m) is the effective solidity of filter with soot loading; V is the volume of the filter; c(m) is the mass concentration of particles of emissions; mtotal is total accumulated particle mass; U∞ is face velocity of the bare filter; U0(m) is the effective local velocity of filter with soot loading, which is larger than U∞ because the area for air passage is blocked by the volume of fibers and laden particles.
The E1(m) can then be expanded to overall filtration efficiency of a whole particles-laden filter E(m) using Equation (Equation7[7] ), assuming that trapped particles result in a change in filter solidity which is accounted for as a change in total effective length of the fibers in a unit volume of filters, l(m) using the Equation (Equation20
[20] ). Because the physical fiber diameter in a real fibrous filter is diverse and the size of deposited particle dendrites may be comparable to fiber diameter, the effective fiber diameter is essentially an average value and the laden particles were assumed as a part of fibers to calculate l(m). L(m) is defined as total effective length of the fibers of a filter and can be calculated by multiplying l(m) and the filter volume.
[20]
3. Results and discussion
3.1. Filter penetration efficiency in relation to PM number and mass
The filtration efficiency was analyzed based on the measurement of particle size-resolved number concentrations measured by the DMS500 at pre- and post-filter locations.
shows the averaged size spectra measured at the upstream and downstream of the test filters over different sampling times (t < 1401 s). The particle number concentration at the upstream of filters was largely stable throughout the experiment for both coated and uncoated filter tests. In particular, the variation of pre-filter particle concentrations was within 5% for 5 – 300 nm region, while the variation increased slightly (but still <10%) for 300–500 nm region. The sensitivity of DMS500 for particles with size from 10 to 300 nm was exhibited in the according to Table S1 in the SI. Uncertainty curves were also depicted and both ends of the post-filter data of low significance were truncated. When the number concentration was less than 1000 #/cm3 (dp < 70 nm or dp > 500 nm), the relative error was larger than 10%. Especially for smaller particles than 70 nm, the error could be more than 20%. Therefore, only the 70–500 nm data with the relative error being less than 10% were illustrated. The data between 100–300 nm had an even lower relative error (<1%). The pre-filter spectra exhibited a large portion of accumulation mode particles with the peak of the spectra at approximately 200 nm, which is typical for combustion with stratified injection (Chen et al. Citation2015; Badshah et al. Citation2016; Chen et al. Citation2017).
Figure 2. Size resolved number concentrations at the upstream and downstream of test filters (top: uncoated Whatman® GF/A glass microfiber filters; bottom: coated Pallflex® fiberfilm filters) over different sampling times (U∞ = 5 cm/s).
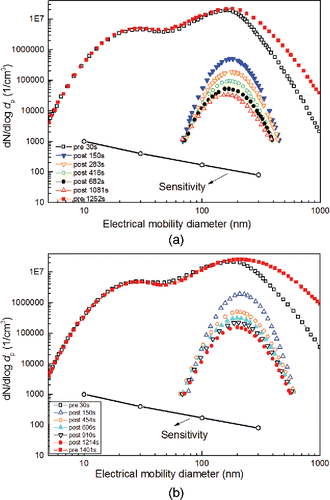
The number-based penetration efficiencies shown in were calculated by comparing the pre- and post-filter particle number concentrations over the particle size range of 70–500 nm. As mentioned previously, the uncertainty in calculating the filtration efficiency was less than 10% when the particle size was between 70–500 nm and it was less than 1% for 100–300 nm data. The penetration efficiency curves for the fibrous filters rendered an inverted U-shaped collection efficiency curve for particle sizes from 70 to 500 nm. Similar results can be found elsewhere in literatures (Sharma et al. Citation2007; Viswanathan et al. Citation2015). Moreover, the penetration efficiency for agglomerates from 70 nm to 500 nm decreased gradually for both fluorocarbon coated and uncoated filters with sampling time. The decrease in penetration efficiency was more pronounced from beginning to 416 s (trapped particles mass: 1.45 mg) than from 416 s to 948 s for Whatman® GF/A filters (uncoated), and from 0 to 454 s (trapped particles mass: 1.44 mg) than from 454 s to 910 s for Pallflex® (fluorocarbon coated) filters. The peak of penetration efficiency decreased from 2% to 0.2% for Whatman® GF/A filters, and from 9% to 0.6% for Pallflex® filters over a sampling time of 900s. Larger thickness and smaller effective fiber diameter are the main reasons for higher penetration efficiency of Pallflex filters compared to the Whatman filters (Podgórski et al. Citation2006). For low or high particle diameters, the number concentration measurement is subject to high noise to signal ratio so that there was occasional abnormal rise (e.g., the end of the 948 s curve), and the inter-section of curves could occur.
Figure 3. Number-based filter penetration efficiencies versus particle size with different sampling times (top: uncoated Whatman® GF/A glass microfiber filters; bottom: coated Pallflex® fiberfilm filters). Arrow indicates the decrease of the MPPS over the sampling process (U∞ = 5 cm/s).
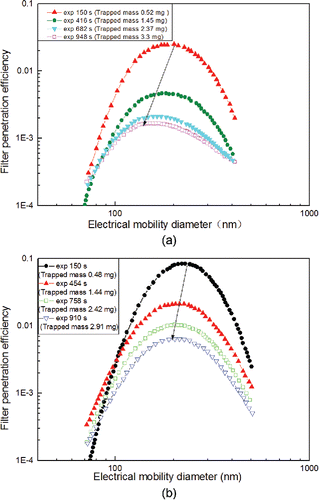
Another feature for particle-laden filters is the change in the most penetration particle size (MPPS), which were located at approximately 200 nm for both types of the filters at the beginning. The MPPS of micro-glass filter media is usually in the range of 120 nm to 250 nm (Sharma et al. Citation2007). This is because when the particle size dp is between 100 and 200 nm, both diffusion and the interception have weak effects on filtration efficiency. The MPPS decreased from 200 nm to about 150 nm for uncoated filters, and from 220 nm to 200 nm for fluorocarbon coated filters. This was illustrated by arrows in . The observed shift in the MPPS was a result of a larger increase in interception than diffusion with sampling time. Furthermore, an increase in effective local velocity will decrease the diffusion filtration efficiency and increase interception filtration efficiency (Viswanathan et al. Citation2015), which provide an explanation for the shift of MPPS. The solidity also increased (Figure S2 in the SI) with filter soot loading, which resulted in a faster reduction in penetration efficiency for large particles than for small particles.
3.2. Comparison of experimental and modeled results
The filter efficiencies were calculated based on the theoretical analysis of the filtration mechanisms to further understand the decrease of penetration efficiency and the decrease of MPPS. shows the comparison of the theoretical predictions and experimental results for an initial phase (t < 948 s) using the empirical single-fiber filtration efficiency model and the revised model for particles-laden filters. Since Whatman filter is the pure glass fiber filter without any binder and fluorocarbon coating, the two models only simulate the Whatman filter. The pressure drop of the filter increased slowly (by ∼200 Pa) from beginning to 948 s (see the SI), which implies that this period was still in the depth filtration stage without soot cake on the surface of the filter (Thomas et al. Citation2001). shows the change of penetration efficiency, which was estimated by using the empirical single-fiber filtration efficiency model for particle-laden filters (Equations Equation(7)–(17)), whilst exhibits the change of penetration efficiency, which was estimated by using the revised model (Equations Equation(7)–(16) and Equation(18)–(20)). In general, the simulated data was consistent with experimental trends at the beginning. Both the empirical model and the revised model showed that the decrease in penetration efficiency was accompanied by a corresponding decrease of the MPPS.
Figure 4. Comparison of the Whatman® GF/A Glass Microfiber filter efficiency curves from the modeled results and experimental data (U∞ = 5 cm/s).
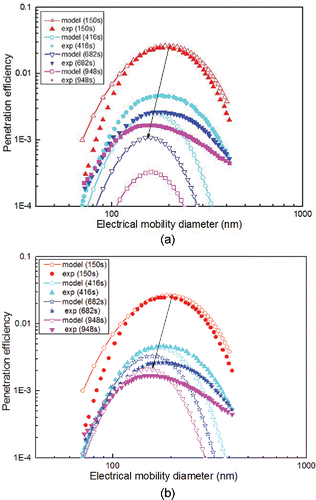
The empirical model was consistent with the experimental results before 416 s, but a deviation of nearly one order of magnitude thereafter was noted over time. The simulated values of λ0 were significantly greater compared with experimental data (Lehmann Citation2005; Kasper et al. Citation2009), and therefore the deviations were gradually increased. The revised model could provide a better estimate of the filtration efficiency without increasing deviation over time. It can be noted that the simulated data from the new model agreed with the experimental data until 948 s, and the new model was able to predict the filtration efficiency trends consistently with the maximum deviation of about 20% except for large particles at the high end of the measurement spectra, which might have been caused by the high noise to signal ratio of the DMS500 for large particles. The MPPS decreased from 200 nm to around 150 nm for both the empirical model and the revised model, which was consistent with experimental trends. The filter weight and pressure drop have been showed in the SI (Table S3).
3.3. Different deposition characteristics for nucleation mode and accumulation mode PM emissions
showed the total number concentration of nucleation mode (dp < 50 nm) and accumulation mode (50 nm < dp < 1000 nm) for both pre- and post-filter. High concentrations of particulate matter emissions in the accumulation mode were observed upstream of the filter, which was typical for the GDI engine operating conditions. The number concentrations of the nucleation mode particles were close to the noise level of the DMS500 after the filters. Almost all the nucleation mode (below 50 nm) PM emissions were captured by the two kinds of filters whilst a small fraction of accumulation mode with a size between 70 nm to 500 nm PM emissions could pass through both the coated and uncoated filters, which might lead to errors (0.5%–6%) in terms of total particle number when fiber filters are adopted to investigate the characteristics of particle emissions.
4. Conclusion
The filtration efficiency for agglomerate emissions from a GDI engine using two types of glass fiber filters was measured and analyzed experimentally and theoretically, and the main conclusions are as follows:
1. | The fractional efficiencies for both coated and uncoated filters exhibited a U-shaped curve for particle size of 70 to 500 nm when loaded with PM emissions from a GDI engine. For both fluorocarbon coated and uncoated filters, the filtration efficiency in terms of particle mass and particle number increased with sampling time. The most penetrating particulate size decreased over a sampling period of 900 s for both filters, which may be attributed to the increase of local velocity inside the filter and the increase in solidity for PM laden filters. | ||||
2. | A small fraction of accumulation mode particles with a size between 70 nm to 500 nm penetrated both fluorocarbon coated and uncoated the filters whilst almost all nucleation mode particles with a size below 50 nm were captured by the two filters. | ||||
3. | A revised single-fiber filtration efficiency model quantitatively incorporating the effects of the increasing local velocity, filter solidity, effective total length of fiber and the dynamic shape factor was developed that could provide a better estimate of the filtration efficiency trends without increasing deviation over time compared with the empirical model. |
Nomenclature
AFR | = | air fuel ratio |
ATDC | = | after top dead center |
BTDC | = | before top dead center |
CA | = | crank angle |
CPMA | = | centrifugal particle mass analyzer |
DPF | = | diesel particulate filter |
DR | = | dilution ratio |
EVC | = | exhaust valve close |
EVO | = | exhaust valve open |
GDI | = | gasoline direct injection |
IVC | = | intake valve close |
IVO | = | intake valve open |
MFM1 | = | the “dilution” air mass flow rate |
MFR | = | mass flow rate |
MPPS | = | most penetrating particulate size |
PCM | = | particle counting methods |
PAH | = | polycyclic aromatic hydrocarbon |
PM | = | particulate matter |
SF | = | single fiber |
VOCs | = | volatile organic compounds |
TWC | = | three way catalyst |
T | = | the thickness of filters (µm) |
Cc | = | the Cunningham slip correction factor |
Cc, dm | = | the Cunningham slip correction factor based on mass equivalent particle diameter |
Cc, dp | = | the Cunningham slip correction factor based on mobility diameter |
cm | = | the accumulated mass per filter volume (kg/m3) |
c(m) | = | the mass concentration of particles of emissions |
ED | = | Brownian diffusion efficiency (%) |
ER | = | interception efficiency (%) |
EI | = | inertial impaction efficiency (%) |
EDR | = | interception of the diffusing particles (%) |
E1 | = | total single-fiber efficiency for single-fiber (%) |
E1(m) | = | total single fiber efficiency with soot loading (%) |
E | = | the total filtration efficiency of a filter (%) |
E(m) | = | the total filtration efficiency of a filter with soot loading in the revised model (%) |
m | = | the particle mass |
P(m) | = | the overall penetration efficiency of a whole filter for particle laden filters (%) |
Df | = | fractal dimensions[−] |
χ | = | dynamic shape factor [−] |
fd | = | friction factor of mass equivalent sphere for the agglomerate [−] |
fd* | = | friction factor of the agglomerate [−] |
Pe | = | Peclet number |
R | = | interception parameter |
Stk | = | Stokes number |
α | = | solidity (%) |
α0 | = | the solidity of the bare fiber (%) |
α(m) | = | the effective solidity of filter with soot loading (%) |
U∞ | = | face velocity of the bare fiber (cm/s) |
U0(m) | = | the effective local velocity of filter with soot loading (cm/s) |
l(m) | = | the total effective length of fiber in a unit volume of the particles laden filters (1/m2) |
L(m) | = | the total effective length of the fibers of a filter (m) |
mtotal | = | total accumulated particle mass |
D | = | the revised diffusion coefficient of agglomerates |
λ0 | = | collection efficiency raising factor |
ρe | = | the effective density of particles |
θ | = | the mean free path of the gas molecules |
dm | = | the mass equivalent particle diameter |
df | = | the effective fiber mean diameter |
dp | = | electrical mobility diameter |
η | = | the gas viscosity |
= | the relaxation time |
UAST_1331293_Supplemental_File.zip
Download Zip (721.2 KB)Acknowledgments
The authors would also like to express gratitude to Mr. Jianhua Xiao, a senior engineer in the State Key Laboratory of Automotive Safety and Energy, for his great support in the test bench setup.
Funding
This study was funded by the National Natural Science Foundation of China (Grant No. 91641119 and Grant No. 51306011). Financial support from the Shenzhen Science and Technology Innovation Committee (No. JCYJ20160318094727251) is also acknowledged.
References
- Ardkapan, S., Johnson, M., Yazdi S., et al. (2014). Filtration Efficiency of an Electrostatic Fibrous Filter: Studying Filtration Dependency on Ultrafine Particle Exposure and Composition. J. Aerosol Sci., 72(3):14–20.
- Badshah, H., Kittelson, D., and Northrop W. (2016). Particle Emissions from Light-Duty Vehicles during Cold-Cold Start. SAE Int. J. Engines, 9(3):1775–1785.
- Brown, R. C. (1993). Air Filtration: An Integrated Approach to the Theory and Applications of Fibrous Filters. Pergamon Press, New York.
- Brockmann, J., and Rader, D. (1990). APS Response to Nonspherical Particles and Experimental Determination of Dynamic Shape Factor. Aerosol Sci. Technol., 13(2):162–172.
- Cambustion website, Gasoline Direct Injection Particulate Measurement with the DMS Series. Available at: http://www.cambustion.com/sites/default/files/applications/DMS/dms08v01.pdf
- Chen, L., Liang, Z., Zhang, X, et al. (2017). Characterizing Particulate Matter Emissions from GDI and PFI Vehicles Under Transient and Cold Start Conditions. Fuel, 189:131–140.
- Chen, L., and Stone, R. (2011). Measurement of Enthalpies of Vaporization of Isooctane and Ethanol Blends and Their Effects on PM Emissions from a GDI Engine. Energy Fuels, 25(3):1254–1259.
- Chen, L., Stone, R., and Richardson, D. (2012). A Study of Mixture Preparation and PM Emissions Using a Direct Injection Engine Fuelled with Stoichiometric Gasoline/Ethanol Blends. Fuel, 96(1):120–130.
- Chen, L., Stone, R, and Richardson, D. (2012). Effect of the Valve Timing and the Coolant Temperature on Particulate Emissions from a Gasoline Direct Injection Engine Fuelled with Gasoline and with a Gasoline–Ethanol Blend. Proc. IMechE, Part D: J. Automobile Eng., 226(D10):1419–1430.
- Chen, L., Zhang, Z., Gong, W., et al. (2015). Quantifying the Effects of Fuel Compositions on GDI-Derived Particle Emissions Using the Optimal Mixture Design of Experiments. Fuel, 154:252–260.
- Chen, L., Ma, Y., Guo, Y., et al. (2017). Quantifying the Effects of Operational Parameters on the Counting Efficiency of a Condensation Particle Counter Using Response Surface Design of Experiments (DoE). J. Aerosol Sci., 33:27–39.
- Davies, C. (1973). Air Filtration. Academic Press, London and New York.
- EPA US. (2009). Integrated Science Assessment for Particulate Matter (Final Report). U.S. Environmental Protection Agency, Washington, D.C.
- Friedlander, S., and Marlow, W. (2008). Smoke, Dust and Haze: Fundamentals of Aerosol Behavior. Phys. Today, 1(9):58–59.
- Friedlander, S., and Pui, D. (2004). Emerging Issues in Nanoparticle Aerosol Science and Technology. J. Nanopart. Res., 6(2):313–320.
- Giechaskiel, B., Maricq, M., Ntziachristos, L., et al. (2014). Review of Motor Vehicle Particulate Emissions Sampling and Measurement: From Smoke and Filter Mass to Particle Number. J. Aerosol Sci., 67(1):48–86.
- Hinds, W. (1999). Aerosol Technology: Properties, Behavior and Measurements of Airborne Particles, 2nd ed. John Wiley, New York.
- Kanaoka, C., Emi, H., and Myojo, T. (1980). Simulation of the Growing Process of a Particle Dendrite and Evaluation of a Single Fiber Collection Efficiency with Dust Load. J. Aerosol Sci., 11(4):377–389.
- Kanaoka, C. (1989). Time Dependency of Air Filter Performance. Earozoru Kenkyu, 4:256–264.
- Kasper, G., Schollmeier, S., Meyer, J., et al. (2009). The Collection Efficiency of a Particle-Loaded Single Filter Fiber. J. Aerosol. Sci., 40(12):993–1009.
- Kelly, W., and McMurry, P. (1992). Measurement of Particle Density by Inertial Classification of Differential Mobility Analyzer–Generated Monodisperse Aerosols. Aerosol Sci. Technol., 17(3):199–212.
- Kim, S., Wang, J., Emery, M., et al. (2009). Structural Property Effect of Nanoparticle Agglomerates on Particle Penetration through Fibrous Filter. Aerosol Sci. Technol., 43(4):344–355(12).
- Kirsch, A. A., and Stechkina, I. B. (1978). The Theory of Aerosol Filtration with Fibrous Filters. John Wiley &Sons, New York.
- Kulkarni, P., Baron, P., and Willeke, K. (2011). Aerosol Measurement: Principles, Techniques, and Applications, Third Edition. John Wiley & Sons, Inc., Hoboken, New Jersey.
- Lajos, T. (1986). Model for Calculation of Clogging Process in Filter Mats, Proceedings world filtration congress on particle technology. 175–188.
- Lee, K. W. (1981). Maximum Penetration of Aerosol Particles in Granular Bed Filters. J. Aerosol Sci., 12(1):79–87.
- Lehmann, M. (2005). Investigation of Structure and Loading Kinetics of Depth Filters. Ph.D. thesis, Universitatsverlag Karlsruhe (in German).
- Liu, B., and Lee, K. (2002). Efficiency of Membrane and Nuclepore Filters for Submicrometer Aerosols. Environ Sci. Technol., 10(4):345–350.
- Liu, J., Pui, D., and Wang, J. (2011). Removal of Airborne Nanoparticles by Membrane Coated Filters. Sci. Total Environ., 409(22):4868–4874.
- Maricq, M., and Xu, N. (2004). The Effective Density and Fractal Dimension of Soot Particles from Premixed Flames and Motor Vehicle Exhaust. J. Aerosol Sci., 35(10):1251–1274.
- Olfert, J., Symonds, J., and Collings, N. (2007). The Effective Density and Fractal Dimension of Particles Emitted from a Light-Duty Diesel Vehicle with a Diesel Oxidation Catalyst. J. Aerosol Sci., 38(1):69–82.
- Payatakes, A., and Chi, T. (1976). Particle Deposition in Fibrous Media with Dendrite-Like Pattern: A Preliminary Model. J. Aerosol Scie., 7(2):85–100.
- Payatakes, A., and Gradoń, L. (1980). Dendritic Deposition of Aerosol Particles in Fibrous Media by Inertial Impaction and Interception. Chem. Eng. Sci., 35(5):1083–1096.
- Podgórski, A., Bałazy, A, and Gradoń, L. (2006). Application of Nanofibers to Improve the Filtration Efficiency of the Most Penetrating Aerosol Particles in Fibrous Filters. Chem. Eng. Sci., 61(20):6804–6815.
- Sharma, A., Wallin, H., and Jensen, K. A. (2007). High Volume Electrostatic Field-Sampler for Collection of Fine Particle Bulk Samples. Atmos Environ., 41(2):369–381.
- Thomas, D., Penicot, P., Contal, P., et al. (2001). Clogging of Fibrous Filters by Solid Aerosol Particles Experimental and Modelling Study. Chem. Eng. Sci., 56(11):3549–3561.
- Turnbull, J. C. (2010). Separation of Inhomogeneities in Bottle Glass by Density Differences. J. Am. Ceram. Soc., 24(2):37–43.
- Viswanathan, S., Rothamer, D., Sakai, S., et al. (2015). Effect of Particle Size Distribution on the Deep-Bed Capture Efficiency of an Exhaust Particulate Filter. J. Eng. Gas Turb Power, 137:1–11.
- Wei, Q., Kittelson, D., and Watts, W. F. (2001). Single-Stage Dilution Tunnel Design. SAE Technical Paper, 01–0207.
- Xu, J. (2000). The Analysis and Comparison to the Two Methods of Times Density Measurement. Drilling Fluid & Completion Fluid, 17(6):21–24 (In Chinese).
- Yang, J., Stewart, M., Maupin, G., Herling, D., and Zelenyuk, A. (2009). Single Wall Diesel Particulate Filter (DPF) Filtration Efficiency Studies Using Laboratory Generated Particles. Chem. Eng. Sci., 64(8):1625–1634.
- Yeh, H., and Liu, B. (1974). Aerosol filtration by fibrous filters—I. theoretical. J. Aerosol Sci., 5(2):191–204.