ABSTRACT
The ability of an atmospheric aerosol particle to impact climate by acting as a cloud condensation nucleus (CCN) or an ice nucleus (IN), as well as scatter and absorb solar radiation is determined by its physicochemical properties at the single particle level, specifically size, morphology, and chemical composition. The identification of the secondary species present in individual aerosol particles is important as aging, which leads to the formation of these species, can modify the climate relevant behavior of particles. Raman microspectroscopy has a great deal of promise for identifying secondary species and their mixing with primary components, as it can provide detailed information on functional groups present, morphology, and internal structure. However, as with many other detailed spectroscopic techniques, manual analysis by Raman microspectroscopy can be slow, limiting single particle statistics and the number of samples that can be analyzed. Herein, the application of computer-controlled Raman (CC-Raman) for detailed physicochemical analysis that increases throughput and minimizes user bias is described. CC-Raman applies automated mapping to increase analysis speed allowing for up to 100 particles to be analyzed in an hour. CC-Raman is applied to both laboratory and ambient samples to demonstrate its utility for the analysis of both primary and, most importantly, secondary components (sulfate, nitrate, ammonium, and organic material). Reproducibility and precision are compared to computer controlled-scanning electron microscopy (CCSEM). The greater sample throughput shows the potential for CC-Raman to improve particle statistics and advance our understanding of aerosol particle composition and mixing state, and, thus, climate-relevant properties.
© 2017 American Association for Aerosol Research
EDITOR:
1. Introduction
Atmospheric aerosol particles play an important role in climate by scattering and absorbing solar radiation and acting as cloud condensation nuclei (CCN) or ice nuclei (IN) (Prather et al. Citation2008; Ramanathan et al. Citation2001). Climate-relevant properties, such as hygroscopicity, ice nucleation ability, and light scattering, are dependent on the physical and chemical properties of individual aerosol particles, such as size, chemical composition, and morphology (Poschl Citation2005). Knowledge of the primary components in individual aerosols is often not sufficient to infer their climate properties (Ault and Axson Citation2017; Bauer et al. Citation2013; Prather et al. Citation2008), as a particle's chemical mixing state, the secondary chemical species present, and their internal spatial distribution influence behavior in the atmosphere, such as the ability to act as CCN or IN (Schill et al. Citation2015; Wang et al. Citation2010). For example, partitioning between phases and different types of internal structure, such as core-shell morphology, can inhibit heterogeneous reactivity and/or water uptake (Davies et al. Citation2013; McNeill et al. Citation2006; Ruehl and Wilson Citation2014; Ryder et al. Citation2014, Citation2015). Spectroscopic analysis has great potential to probe particle mixing state and provide important information on particle composition and structure (Lee and Allen Citation2012), while ideally being non-destructive, which can allow for further analysis at a future date.
Raman microspectroscopy has been increasingly applied to study chemical composition and mixing state by probing the functional groups present in individual aerosol particles (Ault et al. Citation2013b; Catelani et al. Citation2014; Craig et al. Citation2015; Deng et al. Citation2014; Ebben et al. Citation2013; Ivleva et al. Citation2007; Laskina et al. Citation2013; Rindelaub et al. Citation2016; Sobanska et al. Citation2012; Zhou et al. Citation2014). Raman microspectroscopy not only measures the vibrational frequencies of functional groups present, but also provides the spatial distribution of the measured functional groups within a particle through spectral mapping (Ault et al. Citation2014; Jung et al. Citation2014; Sobanska et al. Citation2014; Stefaniak et al. Citation2009). Raman is particularly sensitive to secondary chemical species, and spectra of the fingerprint region (500–1500 cm−1) (Smith and Dent Citation2005) can be used to identify organic functional groups and, thus, infer classes of organic compounds present based on the detected vibrational modes (Avzianova and Brooks Citation2013; De Gelder et al. Citation2007; McLaughlin et al. Citation2002). Additionally, elemental carbon (EC), especially graphitic species, have very large Raman scattering cross sections, making even small amounts of EC in particles easily detectable (Rosen et al. Citation1978; Rosen and Novakov Citation1977). Raman can also distinguish species, such as sulfate and bisulfate, which gravimetric and mass spectrometry-based methods cannot, allowing pH to be probed (Rindelaub et al. Citation2016). While various infrared spectroscopy techniques, such as attenuated total reflectance Fourier transform infrared spectroscopy (ATR-FT-IR), diffuse reflectance FT-IR, or optical microscopy coupled with FT-IR (micro-FT-IR), can also probe chemical composition and are sensitive to organic compounds, these methods must overcome a strong absorbance interference from water and are limited to bulk analysis or larger (>5 μm) particles with spatial resolution varying across the spectral window (Gaffney et al. Citation2015; Ghorai et al. Citation2011; Jung et al. Citation2014; Ryu and Ro Citation2009; Takahama et al. Citation2013).
In comparison, the diffraction limit for commonly used wavelengths for Raman analysis (532, 633, and 640 nm) allows for analysis of ≥ ∼0.8 μm particles (projected area diameter, for confocal Raman microscopy with a 100x objective). Methods such as surface enhanced Raman spectroscopy (SERS) (Craig et al. Citation2015) and tip enhanced Raman spectroscopy (TERS) (Ofner et al. Citation2016) have been extending the lower size limit for individual particle Raman analysis further into the submicron size range. Additional advantages of Raman microspectroscopy include non-destructive analysis at ambient temperature and pressure, as well as minimal sample preparation. Although Raman microspectroscopy is a powerful analytical technique, it can be time-intensive and difficult to perform uniform analysis for an entire particle population (Ault and Axson Citation2017). Very recently, work by Doughty and Hill has shown the potential of automated Raman analysis for field samples using a 40 × 1 μm beam and advancing tape (Doughty and Hill Citation2017). Development of an automated Raman microspectroscopic method that couples morphological measurements of individual submicron-sized particles with detailed Raman spectra has the potential to improve efficient and thorough characterization of aerosol particle physicochemical properties.
Due to the benefits of spectroscopic analysis, several microspectroscopy techniques have already been automated and applied to the study and characterization of individual aerosol particles. These methods include computer controlled scanning electron microscopy (CCSEM) with energy dispersive X-ray analysis (EDX) and scanning transmission X-ray microscopy coupled with near-edge X-ray absorption fine structure spectroscopy (STXM-NEXAFS) (Casuccio et al. Citation1983; Casuccio et al. Citation2004; Germani and Buseck Citation1991; Laskin and Cowin Citation2001; Laskin et al. Citation2006; Mamane et al. Citation2001; Moffet et al. Citation2010). SEM yields images with high spatial resolution that can be used to determine size and morphology of both coarse and fine mode particles (Casuccio et al. Citation2004; Jambers et al. Citation1995; Laskin and Cowin Citation2001; Laskin et al. Citation2006). When coupled with EDX, SEM can also provide information on elemental composition, and is particularly well-suited to particles containing metals (Ault et al. Citation2012; Guasco et al. Citation2013; Shen et al. Citation2016). However, detailed chemical characterization of particles, particularly mixed carbon-containing particles, is limited (Casuccio et al. Citation2004; Jambers et al. Citation1995; Laskin et al. Citation2006). Another microscopy technique coupled with electronic spectroscopy, TEM–EELS (transmission electron microscopy with electron energy loss spectroscopy) uses inelastically scattered electrons, which provides better chemical detail than EDX, such as the ability to distinguish between organic carbon and soot, but still cannot differentiate organic carbon species and has yet to be automated (Alexander et al. Citation2008; Katrinak et al. Citation1992). As organosulfates and organonitrates have been identified as a larger than previously realized portion of secondary organic aerosol (Darer et al. Citation2011; Glasius and Goldstein Citation2016; Hatch et al. Citation2011; Surratt et al. Citation2010), the inability of EDX to distinguish inorganic sulfate and nitrate from organosulfates and organic nitrates represents another limitation. An additional disadvantage of CCSEM is that it requires samples to be under vacuum, even the relatively less-intense vacuum used for environmental SEM (ESEM), which can result in transformation or loss of volatile or unstable compounds (Stefaniak et al. Citation2009).
Another automated microspectroscopic technique, STXM-NEXAFS, is a non-destructive x-ray technique that yields two-dimensional maps of particle composition and morphology with high spatial resolution, as well as information on bonding and oxidation state (Moffet et al. Citation2010, Citation2011; Takahama et al. Citation2007). This electronic spectroscopy technique is best for investigating oxidation state and evolution of aerosol mixing state, which can be difficult to study with SEM or other methods of microscopy (Moffet et al. Citation2011; Takahama et al. Citation2007). The automated aspect of these methods allows for individual particle analysis while also providing information on the overall particle population through characterization of thousands of particles (Jambers et al. Citation1995; Moffet et al. Citation2010). While both electronic spectroscopy methods (CCSEM-EDX and STXM-NEXAFS) can provide some chemical information (elemental and bonding/oxidation state, respectively), neither provides the detailed molecular information of vibrational spectroscopy methods, such as Raman microspectroscopy. Specifically Raman modes provide insight into functional groups within organic molecules and other secondary components (e.g., nitrate, sulfate), or bonding environment (aqueous versus solid, binding to different cations). This has led multiple studies to use coupled electron beam and Raman microspectroscopy measurements of the same samples, but the statistics for particles analyzed by Raman has typically been limited when compared with EDX or electron probe X-ray microanalysis (EPMA) (Ault et al. Citation2013b; Eom et al. Citation2016; Jung et al. Citation2014; Sobanska et al. Citation2012).
This study describes an automated method for single particle Raman microspectroscopic analysis. Computer-controlled Raman microspectroscopy (CC-Raman) is a method that can provide detailed chemical composition and size for a representative population of aerosol particles. Our initial publication of CC-Raman was validated by comparison with numerous other characterization techniques during the analysis of insoluble residues in snow from the California Sierra Nevada (Creamean et al. Citation2016), but has not been applied to atmospheric aerosols or quantitatively characterized. Herein, the potential of CC-Raman is demonstrated by analysis of laboratory generated aerosol particle standards and mixtures of varying chemical composition, as well as ambient particles collected during the Southern Oxidant and Aerosol Study (SOAS). CCSEM was also used to analyze the standard and ambient aerosol particle samples and is shown to be a valuable complementary technique to CC-Raman, which can provide a more detailed understanding of primary chemical species present for source apportionment. Overall, this study aims to highlight the unique detailed molecular characterization measurements possible with CC-Raman and to demonstrate the potential for CC-Raman to help improve understanding of the chemical composition, mixing state, and climate-relevant properties of atmospheric aerosol particles.
2. Materials and methods
2.1. Laboratory generated aerosol particle samples
Standard solutions were prepared from 18.3 MΩ Milli-Q water and the following chemicals: sodium nitrate (NaNO3), L-alanine (C3H7NO2), and sodium sulfate (Na2SO4) (Sigma-Aldrich, St. Louis, MO, USA); calcium sulfate (CaSO4) (Acros Organics, Fair Lawn, NJ, USA); ammonium sulfate (NH4)2SO4 (Alfa Aesar, Ward Hill, MA, USA); and magnesium sulfate (MgSO4) (Fisher Scientific, Fair Lawn, NJ, USA). All chemicals were >98.0% purity and used without further purification. The nitrate and sulfate salts were selected for this study as NO3− and SO42− are common inorganic species present in aerosol particles. L-alanine was selected as it contains organic functional groups corresponding to vibrational modes commonly observed in aerosol particles, including ν(C-H), δ(C-H), ν(C-C), ν(C-O), and ν(O-C-O). Particles were generated from 0.05 M solutions atomized using HEPA-filtered air. After atomization, particles were size selected at 0.791 μm (electrical mobility diameter) with an electrostatic classifier (Model 3080, TSI Corporation, Shoreview, MN, USA) equipped with a long differential mobility analyzer (Model 3082, TSI Corporation) at sample to sheath flow ratio of 1:8.3 (0.3 to 2.5 lpm) and then impacted onto quartz substrates or Formvar transmission electron microscopy (TEM) grids (Ted Pella, Inc., Redding, CA, USA) using a Microanalysis Particle Sampler (MPS-3, California Measurements, Inc., Sierra Madre, CA, USA). Stage 2 of the MPS (0.40–2.8 μm equivalent aerodynamic diameter cut-point) was analyzed for each of the following samples: (1) mixed standards (L-alanine, ammonium sulfate, and sodium nitrate) and (2) sulfate standards (ammonium sulfate, calcium sulfate, magnesium sulfate, and sodium sulfate). For each sample, particles of each standard type were generated one compound at a time and impacted onto the same quartz substrate or the same TEM grid to achieve an externally mixed distribution. All MPS samples were collected with low enough aerosol concentrations and short enough sampling times to ensure minimal particle overlap (<3% of particles had overlap).
2.2. Ambient aerosol particle samples
Samples of airborne particles were collected on quartz substrates and TEM grids during the SOAS field campaign in Centreville, AL in 2013 using a Micro-Orifice Uniform Deposit Impactor (MOUDI, Model 110, MSP Corp., Shoreview, MN, USA). Samples were frozen and stored prior to analysis. Stage 7 (aerodynamic size cut of 0.56 μm) for the following six samples were analyzed: 6 June, 8:00 PM–7:00 AM; 12 June, 12:00 PM–3:00 PM; 14 June, 12:00–3:00 PM; 20 June, 8:00 AM–7:00 PM; 26 June, 8:00 PM- 7:00 AM, and 8 July, 8:00 PM–7:00 AM.
2.3. Computer-controlled Raman microspectroscopy (CC-Raman)
This study used a Horiba LabRAM HR Evolution Raman spectrometer (Horiba Scientific, Edison, NJ, USA) coupled with a confocal optical microscope (100× long working distance Olympus objective, 0.9 numerical aperture) and equipped with a Nd:YAG laser source (50 mW, 532 nm) and CCD detector. A 600 groove/mm diffraction grating yielded spectral resolution of ∼1.8 cm−1. The instrument was calibrated against the Stokes Raman signal of pure Si at 520 cm−1 using a silicon wafer standard. Laser power was adjusted accordingly with a neutral density filter to prevent damage to the sample (incident laser power ∼35 mW). Spectra were collected for the range 500 to 4000 cm−1 with three accumulations at 5 s acquisition time for the standard samples and four accumulations at 15 s acquisition time for the ambient samples for each particle analyzed.
The CC-Raman method is facilitated by improved software, which applies the Particle Finder and Multivariate Analysis modules of LabSpec6 software (Horiba) that accompanies the spectrometer. An optical image was collected () and aerosol particles were identified based on specified contrast, size, and morphological features (). The spatial resolution of the image was about 9.7 pixels/μm, which allowed for easy identification of ∼1 μm particles. In terms of contrast, arbitrary light and dark thresholds that scaled the contrast of the image within a range of 1–100 were set to identify dark particles against a light background or light particles against a dark background. For size, the minimum particle area was set to 0.5 μm2 and morphological filters were applied to better detect particle edges. A Raman spectrum was collected for each identified particle, as well as measurements of projected area diameter, circularity, and other morphological characteristics. In comparison to standard Raman mapping techniques, the identified particles form an irregularly shaped map area with variable step size between points (particles) analyzed. Only the particles within the image are analyzed and as settings identifying particles can be controlled by the user, false positive and false negative particle identification is reduced, and if unavoidable, at least able to be taken into account. Automation of particle analysis increased the number of particles analyzed per hour by a factor of four in comparison to manual Raman analysis, with ∼80 and ∼20 particles per hour analyzed for the standard and ambient samples, respectively, by CC-Raman. Divisive clustering analysis (DCA), a K-means partitional clustering method, was used to cluster and classify particles based on distinct features in their Raman spectra ( and ). Spectra were not processed prior to clustering analysis and the number of clusters was arbitrarily chosen by the user after testing several numbers of clusters and determining when clusters began to be distinguished by differing intensity of spectral features, rather than the spectral features themselves. Multiple CC-Raman maps were collected for each sample. 323, 151, and 1391 particles were analyzed for the mixed standards sample, the sulfate standards sample, and the ambient samples, respectively. Further discussion of the spectra is provided in the Results section.
Figure 1. (a) Optical image of laboratory generated aerosol particles composed of L-alanine, (NH4)2SO4, and NaNO3. (b) The same image now with aerosol particles to be analyzed identified by the particle area (blue), particle perimeter (green), and the center of the particle where the Raman spectrum is collected (red). (c) DCA-clustered Raman spectra of the analyzed aerosol particles, showing individual and mixed particles including: L-alanine, L-alanine/(NH4)2SO4, (NH4)2SO4, and L-alanine/(NH4)2SO4/NaNO3. Vibrational modes are shown for NaNO3 (orange), (NH4)2SO4 (yellow), and L-alanine (green). (d) Optical image with some identified particle types with four identified clusters - L-alanine particles, L-alanine/(NH4)2SO4 mixed particles, (NH4)2SO4 particles, and L-alanine/(NH4)2SO4/NaNO3 mixed particles.
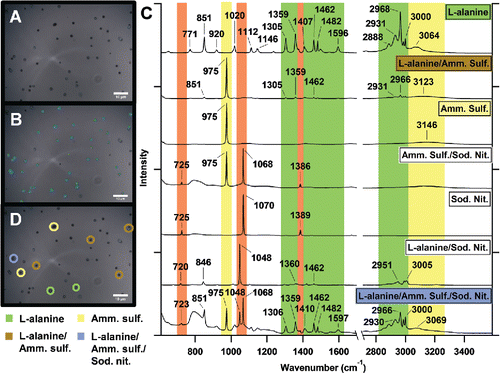
2.4. Computer-controlled scanning electron microscopy (CCSEM)
The laboratory-generated standards were analyzed at the Michigan Center for Materials Characterization (MC)2 at the University of Michigan using a FEI Helios 650 nanolab SEM/FIB equipped with a field emission gun operating at 10 kV and a high angle annular dark field (HAADF) detector. The instrument was equipped with an EDX spectrometer (EDAX, Inc., Mahweh, NJ, USA) which allowed for X-ray detection of elements with atomic numbers (Z) higher than Be. EDX spectra from individual particles were analyzed to determine the relative abundance of elements within each of the standard samples. 271 and 226 particles were analyzed for the mixed standard and sulfate standard samples, respectively. The elements quantified in the mixed standards sample were: C, N, O, Na, S and the elements quantified in the sulfate standards sample were: N, O, Na, Mg, S, and Ca. Single-particle data from the CCSEM analysis was analyzed in MATLAB R2013b (MathWorks, Inc., Natick, MA, USA) using k-means clustering of the elemental composition following the method described in Ault et al. (Citation2012). Clusters were identified as a single compound or mix of compounds based on their elemental composition and the number of clusters was arbitrarily chosen to minimize both cluster number and error. Clustering analysis was performed on the square root of the matrix of atomic percent to emphasize differences due to lower atomic percent elements.
The ambient samples were analyzed at the Environmental Molecular Sciences Laboratory (EMSL) at the Pacific Northwest National Laboratory (PNNL) using a FEI Quanta environmental SEM equipped with a field emission gun operating at 20 kV, a HAADF detector, and an EDX spectrometer (EDAX, Inc.). For the six ambient samples, a total of 4830 particles were analyzed. EDX spectra from individual particles were analyzed to determine the relative abundance of the following 15 elements: C, N, O, Na, Mg, Al, Si, P, S, Cl, K, Ca, Ti, Fe, and Zn. K-means clustering of the particles resulted in clusters that were grouped into source-based classes by elemental composition, including mineral dust, secondary organic aerosol (SOA), biomass burning aerosol, fly ash, biological particles, sea spray aerosol (SSA), and lake spray aerosol (LSA), identified by comparison with literature from previous studies (Allen et al. Citation2015; Ault et al. Citation2012, Citation2013a; Axson et al. Citation2016a,b; Coz et al. Citation2009; Edgerton et al. Citation2009; Hopkins et al. Citation2008; Laskin et al. Citation2006, Citation2012; Li et al. Citation2003; Moffet et al. Citation2013; Posfai et al. Citation2003; Shen et al. Citation2016; Sobanska et al. Citation2003; Utsunomiya et al. Citation2004).
2.5. Condensation particle counter (CPC)
Particle number concentration and counts for each atomized and size selected standard compound were measured using a condensation particle counter (CPC) (Model 3775, TSI Corporation). Samples were tested in triplicate. The particle number counts for each standard were summed over the sampling time to determine the total possible number of particles of the respective compound for the laboratory generated standard sample. The total particle number count for each compound in a sample was compared and used as a preliminary prediction of particle composition of the sample.
3. Results and discussion
3.1. Standard aerosol particle samples
To test the ability of the CC-Raman to distinguish particle types during rapid analysis, a laboratory-generated, mixed-standard aerosol particle sample of L-alanine, (NH4)2SO4, and NaNO3 was characterized (). The sample was compared with CCSEM characterization and the predicted composition of the sample based on CPC particle concentration measurements prior to impaction (). The CC-Raman method identified clusters of L-alanine particles, L-alanine/(NH4)2SO4 mixed particles, (NH4)2SO4 particles, (NH4)2SO4/NaNO3 mixed particles, NaNO3 particles, L-alanine/NaNO3 mixed particles, and L-alanine/(NH4)2SO4/NaNO3 mixed particles ( and ). These clusters were determined based on the functional groups identified by the different vibrational modes present in the Raman spectra (). NaNO3 was identified by the presence of the νs(NO3−) symmetric stretching mode at 1068 cm−1 (solid) or 1048 cm−1 (free, aqueous NO3− ion) (Deng et al. Citation2014; Jentzsch et al. Citation2013; Rousseau et al. Citation1968). νa(NO3−) asymmetric stretching modes were also often present at ∼725 cm−1 and ∼1386 cm−1, respectively (Ault et al. Citation2014; Jentzsch et al. Citation2013; Rousseau et al. Citation1968). (NH4)2SO4 was identified by the presence of the νs(SO42−) symmetric stretching mode at 975 cm−1 and the ν(N-H) stretching mode, a broad peak around 3000–3200 cm−1 (Ault et al. Citation2013b; Jentzsch et al. Citation2013; Sobanska et al. Citation2012; Venkateswarlu et al. Citation1975; Zhou et al. Citation2014). As the only organic compound present in this sample, L-alanine, was identified by the presence of peaks in the 1300–1600 cm−1 and 2800–3000 cm−1 regions. The 1300–1600 cm−1 region contains many different vibrational modes, such as carbon-carbon and carbon-oxygen stretches, including ν(C-C), ν(C-O), and ν(O-C-O), as well as carbon-hydrogen bending modes (δ(C-H)), and others (Ault et al. Citation2013b; Avzianova and Brooks Citation2013; De Gelder et al. Citation2007; Devillepin et al. Citation1982; Larkin Citation2011; Laskina et al. Citation2013; McLaughlin et al. Citation2002). Vibrational modes in the 2800–3000 cm−1 region more often represent symmetric and asymmetric ν(CH3) and ν(CH2) stretches (Ault et al. Citation2013b; Ebben et al. Citation2013; Larkin Citation2011; Laskina et al. Citation2013; McLaughlin et al. Citation2002). It is important to note that simple assignments relating each vibrational mode to a specific compound can be made for this laboratory generated standard sample, as the compounds dictating the chemical composition of the particles were controlled.
Figure 2. (a) Percent fraction of cluster types as determined using CC-Raman, CC-SEM, and CPC techniques. (b) Total fraction of particles containing each compound regardless of mixing state as determined by each method.
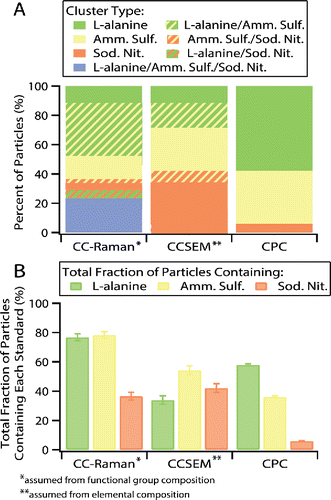
CCSEM analysis, which identified particles based on their elemental composition, resulted in similar clustering as CC-Raman. Particles of each individual compound and particles with mixtures of two compounds were distinguished, but CCSEM was not sensitive to particles containing all three compounds. The simple predicted sample composition based on CPC measured particle number counts did not account for multiple particles impacting on each other or any partitioning and thus did not include particles of mixed composition. For further comparison between the methods, the total fraction of particles containing each compound (L-alanine, (NH4)2SO4, and NaNO3) was calculated, regardless of whether the particle contained one compound or was mixed (meaning the total fraction for all the compounds will sum greater than 100% for the CC-Raman and CCSEM data due to the presence of mixed particles) (). Differences between CC-Raman and CCSEM identified cluster fractions with the CPC predicted cluster fractions can mainly be attributed to challenges from particle impaction. The CPC prediction was based on the total number of particles counted for each generated aerosol population prior to impaction onto substrates, while CC-Raman and CCSEM analysis was performed on particles after impaction, during which losses most likely occurred as some particle types impacted more efficiently than others, dependent on both particle composition and substrate material (Chang et al. Citation1999; Hinds Citation1999; Virtanen et al. Citation2011). Impaction of particles also led to mixed chemical composition, which the CPC method was unable to predict. CC-Raman was consistent with the CPC predictions in that there was significantly less NaNO3 (37%) than L-alanine (77%) and (NH4)2SO4 (78%) present overall in the sample. While CCSEM detected a similar fraction of NaNO3 (42%), it was much less sensitive towards L-alanine (34%) and (NH4)2SO4 (54%). This is most likely due to CCSEM sensitivity issues with low Z elements, such as H, C, O, and N (Mamane et al. Citation2001; Ro et al. Citation1999), interference with detection of C and O due to the Formvar TEM grid substrate, and poor stability of these compounds under the electron beam. In comparison, CC-Raman most likely had minimal issues with sensitivity towards certain particle types due to all compounds containing functional groups with easily detected Raman active vibrational modes and lack of interference from the quartz substrate. Differences in the size of particles analyzed by each technique could also contribute to discrepancies in the particle types observed by CC-Raman in comparison to CCSEM. As shown in the size distributions for particles analyzed by each technique (Figure S1), CC-Raman detected only supermicron particles, while CCSEM detected both sub- and supermicron particles. Cluster percent fraction and total fraction of particles containing each compound with standard error for each method for the mixed standards sample are included in Tables S1 and S2.
Limitations of manual Raman microspectroscopy include: user biases due to hand selecting particles for analysis and time expenses limiting particle statistics and samples analyzed. Both are essentially eliminated by the ability of the CC-Raman method to increase the number of individual particles analyzed under identical analysis conditions. Figures of merit are given in and , including accuracy and precision (reproducibility) of the CC-Raman method. illustrates the cluster percent fractions for three trials of the CC-Raman method in comparison with the cumulative total CC-Raman analysis that was shown in . Trials 1, 2, and 3 characterized 107, 111, and 105 particles, respectively. Cluster percent fraction values with standard error, with comparison to the cumulative total mixed standards CC-Raman analysis, are listed in Table S3. shows the total fraction of particles containing each compound, whether as a single or mixed component, for each trial and the cumulative total. Values with standard error for the total fraction of particles containing each compound are provided in . While there are some differences between the cluster percent fractions, most likely due to the particle variability within the sample, the total fraction of particles containing each compound are mostly within error for each trial and the cumulative total. The consistency between trials for the total fraction of particles containing each compound demonstrates the reliability of the CC-Raman method.
Figure 3. (a) Cluster percent fractions for three trials of ∼100 particles each and cumulative total particles analyzed. (b) Total fraction of particles containing each compound for the three trials and cumulative total for the mixed standards sample.
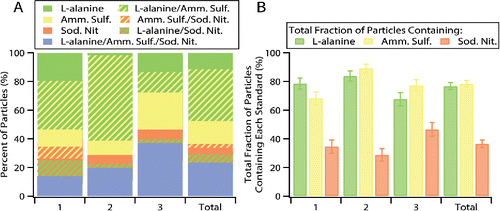
Table 1. Total fraction of particles containing each compound type with standard error for each trial and cumulative total for CC-Raman of the mixed standards sample.
As inorganic sulfate can be present in different bonding environments (liquid, solid, and mixed phase particles) in the atmosphere, the ability to distinguish the counter ion that sulfate is bonded to is important. CC-Raman was applied to characterize a laboratory-generated standard sample composed of different types of sulfate aerosol particles: (NH4)2SO4, calcium sulfate (CaSO4), magnesium sulfate (MgSO4), and sodium sulfate (Na2SO4). Raman can detect shifts in the νs(SO42−) frequency in the Raman spectrum that are caused by the corresponding cation (Jentzsch et al. Citation2013; Mabrouk et al. Citation2013). Raman is also capable of probing bonding environment present in the particle, such as by identifying the presence of a free SO42− ion based on the νs(SO42−) location. The ability to differentiate particles that contain the same anion is unique compared to other single particle analysis techniques, such as CCSEM, STXM-NEXAFS, and aerosol time-of-flight mass spectrometry (ATOFMS) that can only identify the presence of the anion and not the bonding cation, and will be valuable for investigations of particle reactivity, phase, and acidity of both laboratory generated and ambient aerosol particles.
Similar to the mixed aerosol particle standard sample, the results of this characterization were also compared to CCSEM characterization and CPC predicted aerosol population composition ( and ). CC-Raman analysis identified clusters of (NH4)2SO4 particles, (NH4)2SO4/CaSO4 mixed particles, CaSO4/MgSO4 mixed particles, CaSO4/MgSO4/Na2SO4 mixed particles, Na2SO4 particles, and (NH4)2SO4/CaSO4/MgSO4/Na2SO4 mixed particles (). The νs(SO42−) for (NH4)2SO4 is located at 975 cm−1 (Ault et al. Citation2013b; Jentzsch et al. Citation2013; Zhou et al. Citation2014), for Na2SO4 at 996 cm−1 (Jentzsch et al. Citation2013; Sobanska et al. Citation2012), for CaSO4 at 1008 cm−1 (Jentzsch et al. Citation2013; Sobanska et al. Citation2012), and for MgSO4 at 1014 cm−1 (Jentzsch et al. Citation2013). The ν(SO42−) mode located at 983 cm−1 is indicative of free, aqueous sulfate ion (Jentzsch et al. Citation2013). The peak location for each sulfate compound's νs(SO42−) mode was also confirmed by comparison with Raman spectra collected from individual particles from samples of each pure compound.
Figure 4. (a) Percent fraction of cluster types as determined by the CC-Raman, CCSEM, and CPC methods. (b) Total fraction of particles containing each compound regardless of mixing state as determined by each method. (c) Example spectra (focused on the spectral region where the νs(SO42−) vibrational mode is present) for each CC-Raman identified. The highlights represent regions where intensity in the Raman spectrum are indicative of the νs(SO42−) vibrational mode of ammonium sulfate (yellow), sodium sulfate (green), calcium sulfate (purple), and magnesium sulfate (orange).
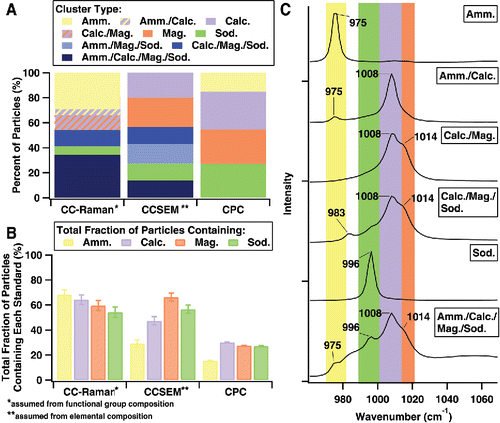
CCSEM is unable to differentiate different types of sulfates, except indirectly by identifying the cation element. CCSEM analysis yielded clusters of CaSO4 particles, MgSO4 particles, Na2SO4 particles, (NH4)2SO4/MgSO4/Na2SO4 mixed particles, CaSO4/MgSO4/Na2SO4 mixed particles, and (NH4)2SO4/CaSO4/MgSO4/Na2SO4 mixed particles. CCSEM did not detect any mixed particles containing only two components nor was CCSEM able to identify any free sulfate anions. Both the cluster percent fractions () and total fraction of particles containing each sulfate compound () show CCSEM's poor sensitivity for ammonium sulfate compared to CC-Raman. As with the previously discussed mixed standard sample, this is most likely due to the fact that NH4+ cation is composed solely of low Z elements and that it is an unstable compound under the electron beam (Mamane et al. Citation2001; Ro et al. Citation1999). Also similar to the mixed standard sample, discrepancy between the CC-Raman and CCSEM methods compared to the CPC predictions can mainly be attributed to inefficiencies in the particle impaction process (Chang et al. Citation1999; Hinds Citation1999; Virtanen et al. Citation2011) and differences in the size of particle analyzed by each technique (Figure S2). Cluster percent fraction and the total fraction of particles containing each compound with standard error for each method for the sulfate standards sample are included in Tables S4 and S5.
3.2. Ambient aerosol particle samples
To further demonstrate the potential of CC-Raman, the method was applied to study ambient aerosol particles collected during the SOAS field campaign in Centreville, AL in 2013. 1391 particles from six different samples were characterized, yielding clusters of trace organic particles, organic particles, organic/mineral mixed particles, particles containing graphitic soot, and particles that exhibited fluorescence (). The graphitic soot cluster also includes particles that exhibit artifacts of burning, due to the similarity of these spectral features to those of soot and graphitic carbon species (discussed further later). Cluster fraction values with standard error can be found in Table S6. A size distribution, ranging from 0.8 to 8.7 μm with a mode of 1.5 μm, based on the CC-Raman measured projected area diameter, is shown in Figure S1. The measured particle sizes are consistent with the expected particle sizes based on the MOUDI stage 7 nominal size cut of 0.56 µm when spreading upon impaction, which can be three or four times the aerodynamic diameter (Sobanska et al. Citation2014), is taken into account.
The trace organic cluster was composed of particles that yielded spectra with intensity in both the fingerprint and higher frequency regions of the Raman spectrum that would correspond to the vibrational modes of organic functional groups, but the intensity was too low to resolve specific peaks. The low intensity is most likely due to the organic components of the particle contributing only a small fraction to the overall particle composition, with the remaining components potentially being water, SSA, mineral (non-fluorescing), or other types that either cannot be detected or are difficult to detect with Raman. For example, the small fraction of organic/mineral particles exhibited strong peaks at 518 and 636 cm−1 indicative of either aluminum-containing and silicon-containing minerals (Frost et al. Citation1997; Laskina et al. Citation2013), but peaks corresponding to various unresolved organic vibrational modes were also present. The organic/mineral particle spectra can be found in Figure S4, along with example spectra of the different types of fluorescence and graphitic soot observed. With detailed post-analysis, useful information can be obtained from spectra that exhibit fluorescence (Doughty and Hill Citation2017) and fluorescence microscopy or other techniques can potentially be used in correlation with Raman analysis to identify soot, biological, and other types of particles. For the EC-containing particles, peaks around 1350 and 1600 cm−1 corresponding to the D “disorder” band of the breathing vibrational mode and the G “graphitic” band of the stretching and bending vibrational modes of honeycomb framework carbon atoms, respectively, can be used to identify soot and various types of ordered graphitic carbon (Catelani et al. Citation2014; Doughty and Hill Citation2017; Gaffney et al. Citation2015; Ivleva et al. Citation2007; Malard et al. Citation2009; Pimenta et al. Citation2007). A few optical images highlighting particles identified as graphitic soot are included in Figure S5.
The organic cluster, which was almost a quarter fraction of the particles detected, was composed of many particles with diverse and rich spectra. As seen in the example spectra shown in , these particles contained ν(SO42−), ν(NO3−), ν(N-H), and ν(O-H) stretching modes, as well as a variety of modes in the organic fingerprint region and different combinations of ν(CH2/CH3) symmetric and asymmetric stretching modes. The vibrational modes in the organic fingerprint region are difficult to identify with certainty due to the many different functional groups that exhibit Raman activity within that spectral window (Larkin Citation2011). However, based on the functional groups of carboxylic acids, long chain aliphatics, fatty acids, organonitrates, and other compounds common to SOA, these vibrational modes are most likely stretching modes, such as ν(C-C), ν(C=C), ν(C-O), ν(C=O), ν(CO2−), and ν(C-OH), and bending, wagging, and rocking modes, such as δ(CH2/CH3), δ(C-C), δ(O-H), and δ(O-C-O) (Ault et al. Citation2013b; Avzianova and Brooks Citation2013; De Gelder et al. Citation2007; Deng et al. Citation2014; Devillepin et al. Citation1982; Laskina et al. Citation2013; McLaughlin et al. Citation2002; Zhou et al. Citation2014). shows the further classification of the different types of organic particles based on their secondary components, with smaller clusters broken out on the right hand side of the figure for clarity. Cluster fraction values with standard error can be found in Table S7. A brief comparison of the CC-Raman analysis with CCSEM analysis for two days, June 14 and July 8, is included in Figure S6. This comparison shows that according to CCSEM, SOA comprises a large component of the SOAS aerosol population and CC-Raman can be used to further classify the SOA particles based on their secondary composition. As this work is focused on demonstrating the potential for CC-Raman to be applied to the study ambient aerosol particles, a forthcoming publication will provide further details on the chemical composition of the SOA, mixing state and atmospheric implications of the SOAS aerosol population. Just as there are challenges with directly comparing CC-Raman and CCSEM analysis due to the limitations and capabilities of each technique, direct comparison of CC-Raman analysis with other work characterizing SOA particles from SOAS, such as studies of organic nitrates (Lee et al. Citation2016), IEPOX derived SOA (Lopez-Hilfiker et al. Citation2016), and dust and sea spray aerosol (Allen et al. Citation2015) may be difficult due to differences in the size of particles analyzed and the specific properties characterized by each technique.
Figure 6. Example Raman spectra of the fingerprint region (<1600 cm−1) and higher energy region (2700–3600 cm−1) of particle types identified within the organic class of SOAS aerosol particles. Each spectrum was normalized to the highest intensity peak.
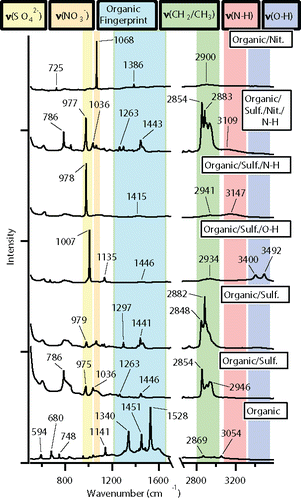
Figure 7. Further classification of the SOAS organic cluster based on the secondary components identified from the vibrational modes present in the Raman spectra.
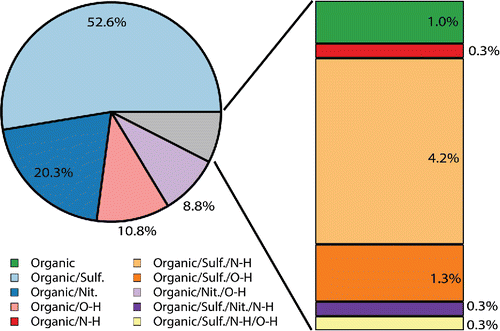
For the ambient samples, CC-Raman provided less information on the primary components and general classification of aerosol particle types. For example, sea spray aerosol (SSA) was difficult to identify by CC-Raman since key SSA components, such as NaCl and MgCl2, do not contain Raman-active vibrational modes. Additionally, while Raman is sensitive to many vibrational modes of mineral components, due to the high photon energy of the 532 nm laser, the Raman spectra of mineral-containing dust particles are often overwhelmed by fluorescence features (Bozlee et al. Citation2005; Doughty and Hill Citation2017). Other particle types also susceptible to fluorescence and/or burning interference include biological and biomass (Poehlker et al. Citation2012). As discussed above, soot particles typically have a distinct spectral signature but it can be challenging to distinguish that signature from the burning features of previously mentioned laser sensitive components (Catelani et al. Citation2014; Doughty and Hill Citation2017; Gaffney et al. Citation2015; Ivleva et al. Citation2007; Sadezky et al. Citation2005). Despite these limitations, there is great potential for CC-Raman to detect the secondary components of aerosol particles, especially those of secondary organic aerosol or other organic-containing particles, as demonstrated in and . In contrast to CCSEM, which can only identify the elemental composition, CC-Raman can distinguish between the functional groups present in a particle that might consist of similar elemental composition. For example, CC-Raman can differentiate between nitric acid (HNO3), nitrate (NO3−), nitrite (NO2−), or ammonium (NH4+) based on the different vibrational modes corresponding to each functional group, whereas CCSEM can only detect nitrogen and oxygen (and oxygen can be difficult to differentiate from signal due to the substrate). Probing secondary speciation with CC-Raman will allow for investigations to improve understanding of important physicochemical properties, including chemical mixing state and internal structure, as well as atmospheric processes such as water uptake and particle phase reactions.
4. Conclusions
This study demonstrates a new method, CC-Raman, for automated single particle aerosol analysis using information dense Raman microspectroscopy. CC-Raman maximizes the potential of Raman microspectroscopy for aerosol particle characterization, specifically in terms of identifying secondary chemical components in SOA and other organic-containing particles that can be difficult to detect. CC-Raman uses uniform, improved throughput analysis to provide information on the vibrational modes that can be used to identify the functional groups present in individual aerosol particles. In addition to identifying secondary species in SOA, CC-Raman can also be applied to study inorganic and non-fluorescing mineral components as well. Because of its ability to probe secondary species and properties of particles that other single particle techniques struggle to determine, CC-Raman can be used for investigations that will answer questions regarding the organic and inorganic molecular species present in aerosol particles, particle aging, and mixing of secondary species with primary components. Additional advantages of CC-Raman include minimal sample preparation and analysis at ambient temperature and pressure. CC-Raman, as a stand-alone technique or used in tandem with other methods, has the potential to be a powerful tool for studying important chemical and physical properties of aerosol particles to further understanding of their role in climate change and other atmospheric processes.
UAST_1337268_Supplemental_File.zip
Download Zip (252.7 KB)Acknowledgments and funding
University of Michigan startup funds supported this project. The Michigan Center for Materials Characterization (MC2) is acknowledged for assistance with CCSEM. Funding for SOAS sampling was provided by the Environmental Protection Agency (EPA). Professors Paul Shepson, Steve Bertman, Kerri Pratt, Ann Marie Carlton, as well as Manelisi Nhliziyo are thanked for assistance with SOAS sampling. The SOAS CCSEM analysis was performed at the Environmental Molecular Sciences Laboratory (EMSL), with assistance from Professor Alex Laskin and Dr. Bingbing Wang, a Department of Energy (DOE) national user facility sponsored by the Office of Biological and Environmental Research at Pacific Northwest National Laboratory (PNNL). PNNL is operated for DOE by Battelle Memorial Institute under Contract No. DE-AC06-76RL0 1830. The University of Michigan Graduate School and Office of the Provost provided travel funds to PNNL.
References
- Alexander, D. T. L., Crozier, P. A., and Anderson, J. R. (2008). Brown Carbon Spheres in East Asian Outflow and Their Optical Properties. Science, 321:833–836.
- Allen, H. M., Draper, D. C., Ayres, B. R., Ault, A., Bondy, A., Takahama, S., Modini, R. L., Baumann, K., Edgerton, E., Knote, C., Laskin, A., Wang, B., and Fry, J. L. (2015). Influence of Crustal Dust and Sea Spray Supermicron Particle Concentrations and Acidity on Inorganic NO3− Aerosol During the 2013 Southern Oxidant and Aerosol Study. Atmos. Chem. Phys., 15:10669–10685.
- Ault, A. P., and Axson, J. L. (2017). Atmospheric Aerosol Chemistry: Spectroscopic and Microscopic Advances. Anal. Chem., 89:430–452.
- Ault, A. P., Guasco, T. L., Baltrusaitis, J., Ryder, O. S., Trueblood, J. V., Collins, D. B., Ruppel, M. J., Cuadra-Rodriguez, L. A., Prather, K. A., and Grassian, V. H. (2014). Heterogeneous Reactivity of Nitric Acid with Nascent Sea Spray Aerosol: Large Differences Observed between and within Individual Particles. J. Phys. Chem. Lett., 2493–2500.
- Ault, A. P., Moffet, R. C., Baltrusaitis, J., Collins, D. B., Ruppel, M. J., Cuadra-Rodriguez, L. A., Zhao, D., Guasco, T. L., Ebben, C. J., Geiger, F. M., Bertram, T. H., Prather, K. A., and Grassian, V. H. (2013a). Size-Dependent Changes in Sea Spray Aerosol Composition and Properties with Different Seawater Conditions. Environ. Sci. Technol., 47:5603–5612.
- Ault, A. P., Peters, T. M., Sawvel, E. J., Casuccio, G. S., Willis, R. D., Norris, G. A., and Grassian, V. H. (2012). Single-Particle SEM-EDX Analysis of Iron-Containing Coarse Particulate Matter in an Urban Environment: Sources and Distribution of Iron within Cleveland, Ohio. Environ. Sci. Technol., 46:4331–4339.
- Ault, A. P., Zhao, D., Ebben, C. J., Tauber, M. J., Geiger, F. M., Prather, K. A., and Grassian, V. H. (2013b). Raman Microspectroscopy and Vibrational Sum Frequency Generation Spectroscopy as Probes of the Bulk and Surface Compositions of Size-Resolved Sea Spray Aerosol Particles. Phys. Chem. Chem. Phys., 15:6206–6214.
- Avzianova, E., and Brooks, S. D. (2013). Raman Spectroscopy of Glyoxal Oligomers in Aqueous Solutions. Spectrochim. Acta. A., 101:40–48.
- Axson, J. L., May, N. W., Colón-Bernal, I. D., Pratt, K. A., and Ault, A. P. (2016a). Lake Spray Aerosol: A Chemical Signature from Individual Ambient Particles. Environ. Sci. Technol., 50:9835–9845.
- Axson, J. L., Shen, H., Bondy, A. L., Landry, C. C., Welz, J., Creamean, J. M., and Ault, A. P. (2016b). Transported Mineral Dust Deposition Case Study at a Hydrologically Sensitive Mountain Site: Size and Composition Shifts in Ambient Aerosol and Snowpack. Aerosol Air Qual. Res., 16:555–567.
- Bauer, S. E., Ault, A., and Prather, K. A. (2013). Evaluation of Aerosol Mixing State Classes in the Giss Modele-Matrix Climate Model Using Single-Particle Mass Spectrometry Measurements. J. Geophys. Res.-Atmos., 118:9834–9844.
- Bozlee, B. J., Misra, A. K., Sharma, S. K., and Ingram, M. (2005). Remote Raman and Fluorescence Studies of Mineral Samples. Spectrochim. Acta. A., 61:2342–2348.
- Casuccio, G. S., Janocko, P. B., Lee, R. J., Kelly, J. F., Dattner, S. L., and Mgebroff, J. S. (1983). The Use of Computer Controlled Scanning Electron Microscopy in Environmental Studies. J. Air Pollut. Control Assoc., 33:937–943.
- Casuccio, G. S., Schlaegle, S. F., Lersch, T. L., Huffman, G. P., Chen, Y., and Shah, N. (2004). Measurement of Fine Particulate Matter Using Electron Microscopy Techniques. Fuel Process. Technol. 85:763–779.
- Catelani, T., Pratesi, G., and Zoppi, M. (2014). Raman Characterization of Ambient Airborne Soot and Associated Mineral Phases. Aerosol Sci. Technol., 48:13–21.
- Chang, M., Kim, S., and Sioutas, C. (1999). Experimental Studies on Particle Impaction and Bounce: Effects of Substrate Design and Material. Atmos. Environ., 33:2313–2322.
- Coz, E., Gómez-Moreno, F. J., Pujadas, M., Casuccio, G. S., Lersch, T. L., and Artíñano, B. (2009). Individual Particle Characteristics of North African Dust under Different Long-Range Transport Scenarios. Atmos. Environ., 43:1850–1863.
- Craig, R. L., Bondy, A. L., and Ault, A. P. (2015). Surface Enhanced Raman Spectroscopy Enables Observations of Previously Undetectable Secondary Organic Aerosol Components at the Individual Particle Level. Anal. Chem., 87:7510–7514.
- Creamean, J. M., Axson, J. L., Bondy, A. L., Craig, R. L., May, N. W., Shen, H., Weber, M. H., Pratt, K. A., and Ault, A. P. (2016). Changes in Precipitating Snow Chemistry with Location and Elevation in the California Sierra Nevada. J. Geophys. Res.-Atmos., 121:7296–7309.
- Darer, A. I., Cole-Filipiak, N. C., O'Connor, A. E., and Elrod, M. J. (2011). Formation and Stability of Atmospherically Relevant Isoprene-Derived Organosulfates and Organonitrates. Environ. Sci. Technol., 45:1895–1902.
- Davies, J. F., Miles, R. E. H., Haddrell, A. E., and Reid, J. P. (2013). Influence of Organic Films on the Evaporation and Condensation of Water in Aerosol. P. Natl. Acad. Sci. USA., 110:8807–8812.
- De Gelder, J., De Gussem, K., Vandenabeele, P., and Moens, L. (2007). Reference Database of Raman Spectra of Biological Molecules. J. Raman. Spectrosc., 38:1133–1147.
- Deng, C., Brooks, S. D., Vidaurre, G., and Thornton, D. C. O. (2014). Using Raman Microspectroscopy to Determine Chemical Composition and Mixing State of Airborne Marine Aerosols over the Pacific Ocean. Aerosol Sci. Technol., 48:193–206.
- Devillepin, J., Novak, A., and Bougeard, D. (1982). Alpha Phase and Beta Phase of Oxalic Acid, H2C2O4 - Vibrational Spectra, Normal Coordinate Calculations, and Intermolecular Forces. Chem. Phys., 73:291–312.
- Doughty, D. C., and Hill, S. C. (2017). Automated Aerosol Raman Spectrometer for Semi-Continuous Sampling of Atmospheric Aerosol. J. Quant. Spectrosc. Radiat. Transfer, 188:103–117.
- Ebben, C. J., Ault, A. P., Ruppel, M. J., Ryder, O. S., Bertram, T. H., Grassian, V. H., Prather, K. A., and Geiger, F. M. (2013). Size-Resolved Sea Spray Aerosol Particles Studied by Vibrational Sum Frequency Generation. J. Phys. Chem. A., 117:6589–6601.
- Edgerton, E. S., Casuccio, G. S., Saylor, R. D., Lersch, T. L., Hartsell, B. E., Jansen, J. J., and Hansen, D. A. (2009). Measurements of OC and EC in Coarse Particulate Matter in the Southeastern United States. J. Air Waste Manage. Assoc., 59:78–90.
- Eom, H.-J., Gupta, D., Cho, H.-R., Hwang, H. J., Hur, S. D., Gim, Y., and Ro, C.-U. (2016). Single-Particle Investigation of Summertime and Wintertime Antarctic Sea Spray Aerosols Using Low-Z Particle EPMA, Raman Microspectrometry, and Atr-Ftir Imaging Techniques. Atmos. Chem. Phys., 16:13823–13836.
- Frost, R. L., Thu Ha, T., and Kristof, J. (1997). FT-Raman Spectroscopy of the Lattice Region of Kaolinite and Its Intercalates. Vib. Spectrosc, 13:175–186.
- Gaffney, J. S., Marley, N. A., and Smith, K. J. (2015). Characterization of Fine Mode Atmospheric Aerosols by Raman Microscopy and Diffuse Reflectance FTIR. J. Phys. Chem. A., 119:4524–4532.
- Germani, M. S., and Buseck, P. R. (1991). Automated Scanning Electron Microscopy for Atmospheric Particle Analysis. Anal. Chem., 63:2232–2237.
- Ghorai, S., Laskin, A., and Tivanski, A. V. (2011). Spectroscopic Evidence of Keto−Enol Tautomerism in Deliquesced Malonic Acid Particles. J. Phys. Chem. A., 115:4373–4380.
- Glasius, M., and Goldstein, A. H. (2016). Recent Discoveries and Future Challenges in Atmospheric Organic Chemistry. Environ. Sci. Technol., 50:2754–2764.
- Guasco, T. L., Cuadra-Rodriguez, L. A., Pedler, B. E., Ault, A. P., Collins, D. B., Zhao, D., Kim, M. J., Ruppel, M. J., Wilson, S. C., Pomeroy, R. S., Grassian, V. H., Azam, F., Bertram, T. H., and Prather, K. A. (2013). Transition Metal Associations with Primary Biological Particles in Sea Spray Aerosol Generated in a Wave Channel. Environ. Sci. Technol., 48:1324–1333.
- Hatch, L. E., Creamean, J. M., Ault, A. P., Surratt, J. D., Chan, M. N., Seinfeld, J. H., Edgerton, E. S., Su, Y., and Prather, K. A. (2011). Measurements of Isoprene-Derived Organosulfates in Ambient Aerosols by Aerosol Time-of-Flight Mass Spectrometry - Part 1: Single Particle Atmospheric Observations in Atlanta. Environ. Sci. Technol., 45:5105–5111.
- Hinds, W. C. (1999). Aerosol Technology: Properties, Behavior, and Measurement of Airborne Particles. John Wiley & Sons, Inc., New York.
- Hopkins, R. J., Desyaterik, Y., Tivanski, A. V., Zaveri, R. A., Berkowitz, C. M., Tyliszczak, T., Gilles, M. K., and Laskin, A. (2008). Chemical Speciation of Sulfur in Marine Cloud Droplets and Particles: Analysis of Individual Particles from the Marine Boundary Layer over the California Current. J. Geophys. Res.-Atmos., 113.
- Ivleva, N. P., McKeon, U., Niessner, R., and Poeschl, U. (2007). Raman Microspectroscopic Analysis of Size-Resolved Atmospheric Aerosol Particle Samples Collected with an ELPI: Soot, Humic-Like Substances, and Inorganic Compounds. Aerosol Sci. Technol., 41:655–671.
- Jambers, W., Bock, L. D., and Grieken, R. V. (1995). Recent Advances in the Analysis of Individual Environmental Particles: A Review. Analyst, 120:681–692.
- Jentzsch, P. V., Kampe, B., Ciobota, V., Roesch, P., and Popp, J. (2013). Inorganic Salts in Atmospheric Particulate Matter: Raman Spectroscopy as an Analytical Tool. Spectrochim. Acta. A., 115:697–708.
- Jung, H.-J., Eom, H.-J., Kang, H.-W., Moreau, M., Sobanska, S., and Ro, C.-U. (2014). Combined Use of Quantitative ED-EPMA, Raman Microspectrometry, and ATR-FTIR Imaging Techniques for the Analysis of Individual Particles. Analyst, 139:3949–3960.
- Katrinak, K. A., Rez, P., and Buseck, P. R. (1992). Structural Variations in Individual Carbonaceous Particles from an Urban Aerosol. Environ. Sci. Technol., 26:1967–1976.
- Larkin, P. (2011). Infrared and Raman Spectroscopy; Principles and Spectral Interpretation. Elsevier, Waltham, MA.
- Laskin, A., and Cowin, J. P. (2001). Automated Single-Particle SEM/EDX Analysis of Submicrometer Particles Down to 0.1 μm. Anal. Chem. 73:1023–1029.
- Laskin, A., Cowin, J. P., and Iedema, M. J. (2006). Analysis of Individual Environmental Particles Using Modern Methods of Electron Microscopy and X-Ray Microanalysis. J. Electron. Spectrosc. Relat. Phenom., 150:260–274.
- Laskin, A., Moffet, R. C., Gilles, M. K., Fast, J. D., Zaveri, R. A., Wang, B., and Nigge, P., Shutthanandan, J. (2012). Tropospheric Chemistry of Internally Mixed Sea Salt and Organic Particles: Surprising Reactivity of NaCl with Weak Organic Acids. J. Geophys. Res.-Atmos., 117.
- Laskina, O., Young, M. A., Kleiber, P. D., and Grassian, V. H. (2013). Infrared Extinction Spectroscopy and Micro-Raman Spectroscopy of Select Components of Mineral Dust Mixed with Organic Compounds. J. Geophys. Res.-Atmos., 118:6593–6606.
- Lee, B. H., Mohr, C., Lopez-Hilfiker, F. D., Lutz, A., Hallquist, M., Lee, L., Romer, P., Cohen, R. C., Iyer, S., Kurtén, T., Hu, W., Day, D. A., Campuzano-Jost, P., Jimenez, J. L., Xu, L., Ng, N. L., Guo, H., Weber, R. J., Wild, R. J., Brown, S. S., Koss, A., de Gouw, J., Olson, K., Goldstein, A. H., Seco, R., Kim, S., McAvey, K., Shepson, P. B., Starn, T., Baumann, K., Edgerton, E. S., Liu, J., Shilling, J. E., Miller, D. O., Brune, W., Schobesberger, S., D'Ambro, E. L., and Thornton, J. A. (2016). Highly Functionalized Organic Nitrates in the Southeast United States: Contribution to Secondary Organic Aerosol and Reactive Nitrogen Budgets. P. Natl. Acad. Sci., 113:1516–1521.
- Lee, S.-H., and Allen, H. C. (2012). Analytical Measurements of Atmospheric Urban Aerosol. Anal. Chem., 84:1196–1201.
- Li, J., Posfai, M., Hobbs, P. V., and Buseck, P. R. (2003). Individual Aerosol Particles from Biomass Burning in Southern Africa: 2, Compositions and Aging of Inorganic Particles. J. Geophys. Res.-Atmos., 108.
- Lopez-Hilfiker, F. D., Mohr, C., D'Ambro, E. L., Lutz, A., Riedel, T. P., Gaston, C. J., Iyer, S., Zhang, Z., Gold, A., Surratt, J. D., Lee, B. H., Kurten, T., Hu, W. W., Jimenez, J., Hallquist, M., and Thornton, J. A. (2016). Molecular Composition and Volatility of Organic Aerosol in the Southeastern U.S.: Implications for IEPOX Derived SOA. Environ. Sci. Technol., 50:2200–2209.
- Mabrouk, K. B., Kauffmann, T. H., Aroui, H., and Fontana, M. D. (2013). Raman Study of Cation Effect on Sulfate Vibration Modes in Solid State and in Aqueous Solutions. J. Raman. Spectrosc., 44:1603–1608.
- Malard, L. M., Pimenta, M. A., Dresselhaus, G., and Dresselhaus, M. S. (2009). Raman Spectroscopy in Graphene. Phys. Rep., 473:51–87.
- Mamane, Y., Willis, R., and Conner, T. (2001). Evaluation of Computer-Controlled Scanning Electron Microscopy Applied to an Ambient Urban Aerosol Sample. Aerosol Sci. Technol., 34:97–107.
- McLaughlin, R. P., Bird, B., and Reid, P. J. (2002). Vibrational Analysis of Isopropyl Nitrate and Isobutyl Nitrate. Spectrochim. Acta. A., 58:2571–2580.
- McNeill, V. F., Patterson, J., Wolfe, G. M., and Thornton, J. A. (2006). The Effect of Varying Levels of Surfactant on the Reactive Uptake of N2O5 to Aqueous Aerosol. Atmos. Chem. Phys., 6:1635–1644.
- Moffet, R. C., Henn, T., Laskin, A., and Gilles, M. K. (2010). Automated Chemical Analysis of Internally Mixed Aerosol Particles Using X-Ray Spectromicroscopy at the Carbon K-Edge. Anal. Chem., 82:7906–7914.
- Moffet, R. C., Roedel, T. C., Kelly, S. T., Yu, X. Y., Carroll, G. T., Fast, J., Zaveri, R. A., Laskin, A., and Gilles, M. K. (2013). Spectro-Microscopic Measurements of Carbonaceous Aerosol Aging in Central California. Atmos. Chem. Phys., 13:10445–10459.
- Moffet, R. C., Tivanski, A. V., and Gilles, M. K. (2011). Chapter 17: Scanning Transmission X-Ray Microscopy: Applications in Atmospheric Research, in Fundamentals and Applications in Aerosol Spectroscopy, R. Signorell, and J. P. Reid, eds., Taylor & Francis Group, Boca Raton, FL.
- Ofner, J., Deckert-Gaudig, T., Kamilli, K. A., Held, A., Lohninger, H., Deckert, V., and Lendl, B. (2016). Tip-Enhanced Raman Spectroscopy of Atmospherically Relevant Aerosol Nanoparticles. Anal. Chem., 88:9766–9772.
- Pimenta, M. A., Dresselhaus, G., Dresselhaus, M. S., Cancado, L. G., Jorio, A., and Saito, R. (2007). Studying Disorder in Graphite-Based Systems by Raman Spectroscopy. Phys. Chem. Chem. Phys., 9:1276–1290.
- Poehlker, C., Huffman, J. A., and Poeschl, U. (2012). Autofluorescence of Atmospheric Bioaerosols—Fluorescent Biomolecules and Potential Interferences. Atmos. Meas. Tech., 5:37–71.
- Poschl, U. (2005). Atmospheric Aerosols: Composition, Transformation, Climate and Health Effects. Angew. Chem. Int. Ed., 44:7520–7540.
- Posfai, M., Simonics, R., Li, J., Hobbs, P. V., and Buseck, P. R. (2003). Individual Aerosol Particles from Biomass Burning in Southern Africa: 1. Compositions and Size Distributions of Carbonaceous Particles. J. Geophys. Res.-Atmos., 108.
- Prather, K. A., Hatch, C. D., and Grassian, V. H. (2008). Analysis of Atmospheric Aerosols. Annu. Rev. Anal. Chem., 1:485–514.
- Ramanathan, V., Crutzen, P. J., Kiehl, J. T., and Rosenfeld, D. (2001). Aerosols, Climate, and the Hydrological Cycle. Science, 294:2119–2124.
- Rindelaub, J. D., Craig, R. L., Nandy, L., Bondy, A. L., Dutcher, C. S., Shepson, P. B., and Ault, A. P. (2016). Direct Measurement of Ph in Individual Particles Via Raman Microspectroscopy and Variation in Acidity with Relative Humidity. J. Phys. Chem. A., 120:911–917.
- Ro, C.-U., Osán, J., and Van Grieken, R. (1999). Determination of Low-Z Elements in Individual Environmental Particles Using Windowless Epma. Anal. Chem., 71:1521–1528.
- Rosen, H., Hansen, A. D. A., Gundel, L., and Novakov, T. (1978). Identification of the Optically Absorbing Component in Urban Aerosols. Appl. Opt., 17:3859–3861.
- Rosen, H., and Novakov, T. (1977). Raman Scattering and the Characterisation of Atmospheric Aerosol Particles. Nature, 266:708–710.
- Rousseau, D. L., Miller, R. E., and Leroi, G. E. (1968). Raman Spectrum of Crystalline Sodium Nitrate. J. Chem. Phys., 48:3409–&.
- Ruehl, C. R., and Wilson, K. R. (2014). Surface Organic Monolayers Control the Hygroscopic Growth of Submicrometer Particles at High Relative Humidity. J. Phys. Chem. A., 118:3952–3966.
- Ryder, O. S., Ault, A. P., Cahill, J. F., Guasco, T. L., Riedel, T. P., Cuadra-Rodriguez, L. A., Gaston, C. J., Fitzgerald, E., Lee, C., Prather, K. A., and Bertram, T. H. (2014). On the Role of Particle Inorganic Mixing State in the Reactive Uptake of N2O5 to Ambient Aerosol Particles. Environ. Sci. Technol., 48:1618–1627.
- Ryder, O. S., Campbell, N. R., Morris, H., Forestieri, S., Ruppel, M. J., Cappa, C., Tivanski, A., Prather, K., and Bertram, T. H. (2015). Role of Organic Coatings in Regulating N2O5 Reactive Uptake to Sea Spray Aerosol. J. Phys. Chem. A., 119:11683–11692.
- Ryu, J., and Ro, C.-U. (2009). Attenuated Total Reflectance Ft-Ir Imaging and Quantitative Energy Dispersive-Electron Probe X-Ray Microanalysis Techniques for Single Particle Analysis of Atmospheric Aerosol Particles. Anal. Chem., 81:6695–6707.
- Sadezky, A., Muckenhuber, H., Grothe, H., Niessner, R., and Pöschl, U. (2005). Raman Microspectroscopy of Soot and Related Carbonaceous Materials: Spectral Analysis and Structural Information. Carbon, 43:1731–1742.
- Schill, S. R., Collins, D. B., Lee, C., Morris, H. S., Novak, G. A., Prather, K. A., Quinn, P. K., Sultana, C. M., Tivanski, A. V., Zimmermann, K., Cappa, C. D., and Bertram, T. H. (2015). The Impact of Aerosol Particle Mixing State on the Hygroscopicity of Sea Spray Aerosol. ACS Central Sci., 1:132–141.
- Shen, H., Peters, T. M., Casuccio, G. S., Lersch, T. L., West, R. R., Kumar, A., Kumar, N., and Ault, A. P. (2016). Elevated Concentrations of Lead in Particulate Matter on the Neighborhood-Scale in Delhi, India as Determined by Single Particle Analysis. Environ. Sci. Technol., 50:4961–4970.
- Smith, E., and Dent, G. (2005). Modern Raman Spectroscopy: A Practical Approach. John Wiley & Sons Ltd, West Sussex, England.
- Sobanska, S., Coeur, C., Maenhaut, W., and Adams, F. (2003). SEM-EDX Characterisation of Tropospheric Aerosols in the Negev Desert (Israel). J. Atmos. Chem., 44:299–322.
- Sobanska, S., Falgayrac, G., Rimetz-Planchon, J., Perdrix, E., Bremard, C., and Barbillat, J. (2014). Resolving the Internal Structure of Individual Atmospheric Aerosol Particle by the Combination of Atomic Force Microscopy, ESEM-EDZ, Raman and Tof-SIMS Imaging. Microchem. J., 114:89–98.
- Sobanska, S., Hwang, H., Choel, M., Jung, H. J., Eom, H. J., Kim, H., Barbillat, J., and Ro, C. U. (2012). Investigation of the Chemical Mixing State of Individual Asian Dust Particles by the Combined Use of Electron Probe X-Ray Microanalysis and Raman Microspectrometry. Anal. Chem., 84:3145–3154.
- Stefaniak, E. A., Buczynska, A., Novakovic, V., Kuduk, R., and Van Grieken, R. (2009). Determination of Chemical Composition of Individual Airborne Particles by Sem/Edx and Micro-Raman Spectrometry: A Review. Journal of Physics: Conference Series 162:012019.
- Surratt, J. D., Chan, A. W. H., Eddingsaas, N. C., Chan, M. N., Loza, C. L., Kwan, A. J., Hersey, S. P., Flagan, R. C., Wennberg, P. O., and Seinfeld, J. H. (2010). Reactive Intermediates Revealed in Secondary Organic Aerosol Formation from Isoprene. P. Natl. Acad. Sci. USA., 107:6640–6645.
- Takahama, S., Gilardoni, S., Russell, L. M., and Kilcoyne, A. L. D. (2007). Classification of Multiple Types of Organic Carbon Composition in Atmospheric Particles by Scanning Transmission X-Ray Microscopy Analysis. Atmos. Environ., 41:9435–9451.
- Takahama, S., Johnson, A., and Russell, L. M. (2013). Quantification of Carboxylic and Carbonyl Functional Groups in Organic Aerosol Infrared Absorbance Spectra. Aerosol Sci. Technol., 47:310–325.
- Utsunomiya, S., Jensen, K. A., Keeler, G. J., and Ewing, R. C. (2004). Direct Identification of Trace Metals in Fine and Ultrafine Particles in the Detroit Urban Atmosphere. Environ. Sci. Technol., 38:2289–2297.
- Venkateswarlu, P., Bist, H. D., and Jain, Y. S. (1975). Laser Excited Raman Spectrum of Ammonium Sulfate Single Crystal. J. Raman. Spectrosc., 3:143–151.
- Virtanen, A., Kannosto, J., Kuuluvainen, H., Arffman, A., Joutsensaari, J., Saukko, E., Hao, L., Yli-Pirilä, P., Tiitta, P., Holopainen, J. K., Keskinen, J., Worsnop, D. R., Smith, J. N., and Laaksonen, A. (2011). Bounce Behavior of Freshly Nucleated Biogenic Secondary Organic Aerosol Particles. Atmos. Chem. Phys., 11:8759–8766.
- Wang, J., Cubison, M. J., Aiken, A. C., Jimenez, J. L., and Collins, D. R. (2010). The Importance of Aerosol Mixing State and Size-Resolved Composition on CCN Concentration and the Variation of the Importance with Atmospheric Aging of Aerosols. Atmos. Chem. Phys., 10:7267–7283.
- Zhou, Q., Pang, S.-F., Wang, Y., Ma, J.-B., and Zhang, Y.-H. (2014). Confocal Raman Studies of the Evolution of the Physical State of Mixed Phthalic Acid/Ammonium Sulfate Aerosol Droplets and the Effect of Substrates. J. Phys. Chem. B, 118:6198–6205.